As the world’s population of adults aged 60 years or older is projected to more than double between 2015 and 2050(1), identifying modifiable risk factors of age-related chronic disease can help reduce the public health burden of population ageing. The association between diet quality and age-related chronic disease is well established(Reference Prince, Wu and Guo2–Reference Everitt, Hilmer and Brand-Miller4) with a growing focus on overall dietary intake as opposed to specific nutrients and foods, acknowledging potential interactions between nutrients and bioavailability of foods(Reference Anderson, Harris and Tylavsky5). Classifications of overall diet quality are either defined a priori, consisting of indices used to score and rank diet quality, or are estimated a posteriori, using data-reduction techniques such as factor or cluster analysis(Reference Newby and Tucker6). Knowledge-based dietary classifications including the Mediterranean diet, the Healthy Eating Index and the Dietary Approaches to Stop Hypertension score have been associated with numerous chronic disease outcomes(Reference Reedy, Wirfalt and Flood7–Reference McCullough, Feskanich and Stampfer12), although predefined dietary indices have limitations including various definitions of a ‘healthy’ diet based on current nutritional guidance and the inability to describe heterogeneous dietary patterns that may exist among distinct population subgroups(Reference Newby and Tucker6).
Empirical approaches to describing dietary intake rely on statistical modelling to identify common dietary patterns in the population of interest and do not depend on pre-established dietary indices(Reference Newby and Tucker6). Dietary patterns identified through data-reduction techniques have been associated with a host of health behaviours and chronic diseases(Reference Anderson, Harris and Tylavsky5,Reference Newby and Tucker6,Reference Van de Rest, Berendsen and Haveman-Nies11,Reference Kerver, Yang and Bianchi13) although most existing studies are based on smaller, non-representative samples or come from studies that lack robust measurement of factors associated with diet including socio-economic status (SES), food insecurity, and health outcomes such as chronic disease and physical disability. Existing research that has applied empirical classification to diet among older adults has been conducted in countries such as Australia and Spain(Reference Thorpe, Milte and Crawford14,Reference Machón, Mateo-Abad and Vrotsou15) or draws from non-generalizable samples of older Americans(Reference Reedy, Wirfalt and Flood7,Reference Wirfält, Midthune and Reed16) . Finally, the statistical methods commonly used to classify dietary patterns among older adults are typically limited to factor analytic methods that do not identify mutually exclusive groups that can be easily compared, or clustering approaches with strict assumptions that do not allow formal tests of model fit(Reference Sotres-Alvarez, Herring and Siega-Riz17).
As the understanding of current dietary patterns among ageing Americans is limited, the objective of our study was to identify common dietary patterns among older Americans and investigate associations between dietary habits, demographic and socio-economic characteristics, health behaviours, chronic disease, and intake of macronutrients and micronutrients known to be associated with chronic disease outcomes. We draw from recently collected dietary data linked to rich contextual data representative of America’s community-dwelling older adults and use latent profile analysis, a form of finite mixture modelling, to overcome limitations common to a posteriori methods of dietary classification.
Methods
Observations were drawn from the Health and Retirement Study (HRS), a biennial national panel survey of older Americans beginning in 1992 and funded by the National Institute on Aging (NIA) and the Social Security Administration (grant number NIA U01AG009740)(Reference Hauser and Weir18). The 2013 Health Care and Nutrition Study (HCNS), an off-year mail-out HRS supplement conducted by the Survey Research Center at the Institute for Social Research at the University of Michigan (grant number NIA U01AG009740), collected information about food consumption using the Harvard FFQ originally proposed by Willett and colleagues(Reference Willett, Sampson and Stampfer19,20) with calculated energy and nutrient totals based on Harvard School of Public Health nutrient tables(21). The University of Michigan’s institutional review board approved the HRS protocol and participants were read a confidentiality statement and provided oral or implied consent when first contacted, and signed a written informed consent form at each interview(Reference Weir, Langa and Ofstedal22). All measures were collected through participant self-report and proxy response to the HRS and HCNS surveys was allowed when the respondent was unable to complete the interview. The HRS sample is a multistage, area-clustered and stratified sampling design representative of all age-eligible non-institutionalized individuals in the US population(Reference Ofstedal, Weir and Chen23).
The original HCNS contained 8073 observations with complete information on consumption of 164 food items. Individuals under the age of 65 years at time of completing the HCNS (n 3792) were removed. To reduce the impact of measurement error on our estimates of interest, we excluded respondents who had daily energy intakes falling outside the commonly used allowable range of 2092–14 644 kJ/d (500–3500 kcal/d) for women and 3347–16 736 kJ/d (800–4000 kcal/d) for men (n 286)(Reference Willett24), or who had either the 2012 HRS or 2013 HCNS survey completed by a proxy respondent (n 437), resulting in an analytic sample of 3558. Participant characteristics were measured in 2012 and were primarily drawn from the RAND HRS data file (Version P)(Reference Chien, Campbell and Chan25), with indicators of cognitive status taken from the core HRS files. Items used to identify food insecurity were drawn from the HCNS. The simple response rates for the HRS and HCNS were 89·1 and 65·0 %, respectively(20,26) .
Measures
Respondents were asked to indicate average total consumption of each specified food item and amount over the past 12 months. Measures of average consumption of 164 food items were converted to responses reflecting average servings per day. Food items were grouped and five food items were excluded from analysis based on recommendations provided by the Food Patterns Equivalence Database by the US Department of Agriculture(Reference Bowman, Clemens and Friday27). The remaining 159 food items were grouped based on nutritional similarity, then summed to represent daily intake of thirty-five separate food groups. Table 1 describes the food groups and lists excluded food items. For clarity, we use the term ‘food groups’ to describe the thirty-five separate foods and food groups used to identify dietary profiles. Scores were log-transformed with an offset of 0·01 to improve normality and allow inclusion of individuals reporting non-intake of a given food group.
Table 1 Food groupings used in the dietary profile analysis, 2012 Health and Retirement Study/2013 Health Care and Nutrition Study*
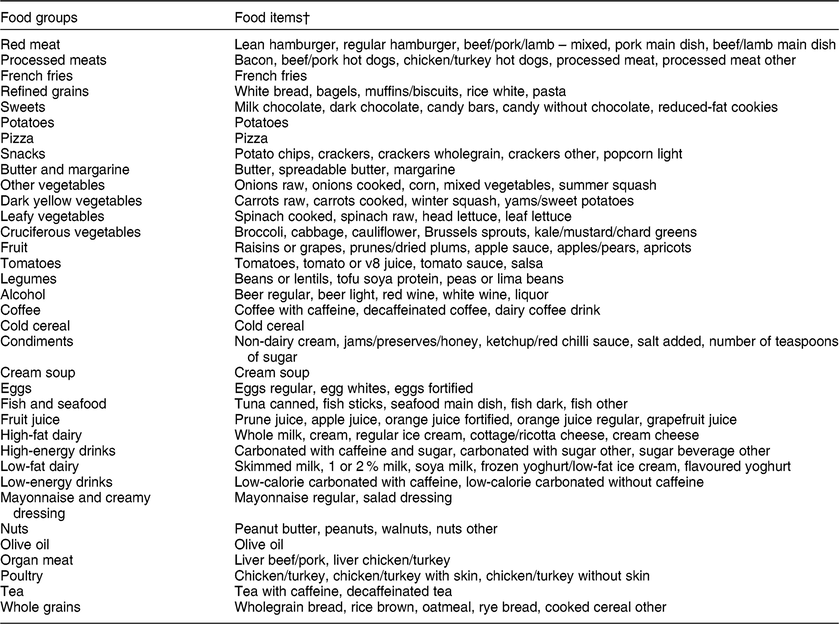
* Food items excluded from analysis: Splenda, artificial sweetener, garlic, low-carbohydrate bars, plain water.
† Some individual food items were not collapsed into groups due to the combination of multiple food items used to produce the food (i.e. pizza) or having varying preparation techniques (i.e. potatoes).
Demographic and socio-economic characteristics, health behaviours and health outcomes were compared across dietary profiles. Demographic measures included respondents’ age, gender (1 = female, 0 = male), race/ethnicity (White, Black, Hispanic, Other), marital status (1 = partnered or married, 0 = single, divorced or widowed) and retirement status (1 = retired, 0 = not retired). Education (<12 years of education, 12 years of education, >12 years of education), longest occupational tenure (white-collar, blue-collar, female homemaker, other occupational tenure), and log-transformed household income and assets were included as indicators of SES. Additionally, food insecurity was assessed using the US Household Food Security Survey Module six-item short form(28). Responses to the six items were summed then dichotomized to reflect either food security (raw score of 0–1) or low/very low food security (raw score of 2–6; 1 = food insecure, 0 = food secure).
Health behaviours included BMI (underweight (BMI < 18·5 kg/m2), normal weight (18·5 kg/m2 ≤ BMI< 25 kg/m2), overweight (25 kg/m2 ≤ BMI < 30 kg/m2), obese (BMI ≥ 30 kg/m2)), vigorous physical activity (participation in activities such as sports and heavy housework, or a job that involves physical labour; no vigorous physical activity; vigorous physical activity less than 1 time/week; vigorous physical activity more than 1 time/week), current smoking status (1 = current smoker, 0 = not current smoker) and alcohol consumption (non-drinkers; moderate drinkers (men drinking between 1 and 14 drinks/week, women drinking between 1 and 7 drinks/week); heavy drinkers (men drinking more than 14 drinks/week, women drinking more than 7 drinks/week)).
Health status was measured using self-rated health status (1 = poor, 5 = excellent) and a sum of doctor-diagnosed chronic conditions (high blood pressure, cancer, diabetes, lung disease, heart problems, stroke, psychiatric problems, arthritis). Early physical disability was assessed with a sum of eleven indicators of limitation in physical mobility (any difficulty in each of the following activities: stooping or crouching, climbing one flight of stairs without resting, climbing several flights of stairs without resting, moving large objects, sitting in a chair for two hours, getting up from a chair after sitting for long periods, lifting weights of more than 4·5 kg (10 lb), raising arms above shoulder level, walking one block, walking several blocks, picking up a dime from a table). Advanced physical disability was based on report of any limitation in activities of daily living (ADL; some difficulty in bathing activities, getting dressed, eating, getting in and out of bed, or walking across a room; 1 = any ADL limitation, 0 = no ADL limitation). Cognitive status was assessed with a multidimensional cognitive measure based on the Telephone Interview for Cognitive Status (TICS) and included tests of immediate and delayed word recall, a serial 7 counting task, backwards counting task, date naming, word recognition and general knowledge questions (range 0–35)(Reference Brandt, Spencer and Folstein29,30) .
Energy intake, macronutrient density, macronutrient intake and micronutrient intake were compared across dietary patterns. Estimated daily energy intake was measured as average daily total kilocalorie intake. Macronutrient density for daily intake of carbohydrate, protein, fat and saturated fat was calculated as the estimated percentage of daily energy coming from each macronutrient source. Macronutrients examined included fibre, n-3 fatty acids, EPA, DHA, alcohol, added sugar, n-6 fatty acids, trans fat and cholesterol. Micronutrients included Ca, Fe, K, folate and vitamins B12, C, D and E. To assess dietary diversity, we included a count of the number of different food groups each participant reported consuming. Finally, RMR was estimated using the Mifflin–St Jeor(Reference Mifflin, St Jeor and Hill31) equation, identified as the most accurate equation to estimate RMR in older populations(Reference Reidlinger, Willis and Whelan32). RMR was also used to calculate the ratio of daily energy intake to RMR.
Statistical methods
Latent profile analysis (LPA) was used to classify older adults into mutually exclusive dietary patterns across the thirty-five observed food groups. LPA is a form of the more general latent class analysis (also described as finite mixture modelling), allowing identification of unobserved heterogeneity in multiple continuous response variables(Reference Collins and Lanza33). The best-fitting latent profile model was determined using information criteria-based metrics including the Akaike information criterion, Bayesian information criterion and sample-size-adjusted Bayesian information criterion, and the Vuong, Lo, Mendell and Rubin likelihood ratio test allowed a significance test of whether the inclusion of an additional profile contributed to a significantly better-fitting model (P < 0·05 was used as the α level for nested model-fit testing). To ensure convergence on global maxima through several replications of the best log-likelihood for each model, 10 000 random sets of starting values with ten final-stage optimizations were used. When random starting values and final-stage optimizations were doubled, log-likelihoods were replicated for all reported LPA models. For reporting purposes, exploratory factor analysis was used to identify meaningfully correlated food groups. Although exploratory factor analysis with orthogonal rotation identified nine factors with eigenvalues >1, only two factors had three or more items with loadings >0·40. Mplus version 8.1(Reference Muthén and Muthén34) was used to conduct the LPA and exploratory factor analysis using maximum-likelihood estimation with robust se and adjustments for missing data and complex survey design.
Once the optimal number of latent dietary profiles was identified, respondent characteristics and indicators of energy, macronutrient density and macronutrient and micronutrient intakes were compared across dietary profile using the statistical software package SAS version 9.4(35). Overall differences in continuous measures were compared across dietary profile using ANOVA with bivariate follow-up through least-squares mean differences. Differences in categorical measures across dietary profile were tested using contingency tables with the Rao–Scott χ 2 test. Significant overall χ 2 tests were partitioned into 2 × 2 contingency tables with Rao–Scott χ 2 tests used to test statistical significance and OR were used to identify the direction the observed cell frequency departed from the expected cell frequency. To reduce the likelihood of type I error due to multiple comparisons, the significance level for each follow-up test was adjusted using the Bonferroni correction. All statistical tests adjusted for complex survey design.
Results
Latent profile analysis
Table 2 presents model fit statistics for LPA models estimating between one and five latent profiles. As the number of estimated profiles increased, the Akaike information criterion, Bayesian information criterion and sample-size-adjusted Bayesian information criterion generally decreased, while entropy remained consistently above 0·80. The Vuong, Lo, Mendell and Rubin likelihood ratio test indicated that the four-class model fit the data significantly better than the three-class solution (P = 0·038), but the five-class solution did not significantly improve model fit over the four-class solution (P = 0·426). Based on model fit tests and the goal of parsimony, the four-class solution was identified as the best description of latent dietary profiles.
Table 2 Model fit statistics for latent profile analysis by number of estimated profile, 2012 Health and Retirement Study/2013 Health Care and Nutrition Study*

LL, log-likelihood; AIC, Akaike information criterion, BIC, Bayesian information criterion; SS-BIC, sample-size-adjusted Bayesian information criterion; VLMR, Vuong–Lo–Mendell–Rubin likelihood ratio test.
* All estimates and statistical tests account for the complex survey design.
Average servings per day of the thirty-five food groups estimated separately for each latent profile are presented in Table 3. Two factors were identified through exploratory factor analysis which we describe as ‘vegetables, fruits and legumes’, containing seven food groups, and ‘red meat and processed foods’, containing nine food groups. Nineteen food items were not meaningfully associated with intake of other foods and are ordered alphabetically. The four latent dietary profiles were characterized by either the types of foods being consumed or the amounts of foods being consumed. Of the estimated 35 035 196 US adults aged 65 years or over in 2013 based on the HCNS population adjustments for our analytic sample, 15·5 % (n 552; N 5 436 762) were classified as eating a ‘Healthy’ diet, 42·1 % (n 1495; N 14 740 699) were classified as consuming a ‘Western’ diet, 29·0 % (n 1058; N 10 170 789) were classified as having ‘High Intake’ and 13·4 % (n 453; N 4 686 946) were described as having ‘Low Intake’. The US Census Bureau reports an estimated population of US adults aged 65 years or older in 2013 as 44 672 695, indicating that our analyses underestimate the US resident population of older adults by about 22 %(36).
Table 3 Average servings per day of food items by latent dietary profile for American adults aged 65 years or older, 2012 Health and Retirement Study/2013 Health Care and Nutrition Study*

a,b,c,d Mean values within row with unlike superscript letters were significantly different (α level for statistical significance identified with Bonferroni correction).
* All estimates and statistical tests excluding sd account for the complex survey design.
† Mean and sd reported for non-log-transformed food groups, statistical tests based on log-transformed food groups.
Older adults identified as having a ‘Healthy’ diet had relatively high intake of vegetables, fruits and legumes, low intake of red meat and processed foods, low intake of condiments and energy drinks, and high intake of low-fat dairy, nuts, olive oil, tea and whole grains. Those with a ‘Western’ diet were typified by low intake of vegetables, fruits and legumes, relatively high intake of red meat and processed foods, as well as comparatively high intake of coffee, condiments, high-fat dairy and high-energy drinks. Older adults classified as ‘High Intake’ consumed the greatest amount of vegetables, fruits and legumes, red meat and processed foods, and nearly all other foods. Finally, those identified in the ‘Low Intake’ profile had the lowest intake of vegetables, fruits and legumes of all identified profiles, relatively low intake of red meat and processed foods, and generally the lowest reported intake of all other food groups excluding non-nutrient-dense items such as condiments and energy drinks.
Respondent characteristics by dietary profile
Table 4 describes how demographic characteristics, SES, health behaviours and health indicators were associated with dietary profile membership. Age did not appear to differ meaningfully across dietary profile. Members of the ‘Healthy’ dietary pattern were more likely to be female, less likely to be White, and less likely to be married/partnered and retired than expected. Household income and assets were relatively high among ‘Healthy’ dietary pattern members, as was the likelihood of earning greater than a high-school education and reporting white-collar occupational tenure. Older adults in the ‘Healthy’ dietary profile were likely not smokers, tended towards normal BMI, reported regular vigorous activity more often than those in other profiles, and generally had the best physical and cognitive health of all profiles. The ‘Western’ dietary profile had significantly fewer women than expected by chance and had household income and assets similar to those in the ‘Healthy’ profile. Members of the ‘Western’ profile were less likely to report earning greater than a high-school degree than expected and had a relatively high likelihood of reporting blue-collar occupational tenure. There were relatively high proportions of smokers and respondents reporting no vigorous physical activity in the ‘Western’ profile, and members of this group appeared to have on average worse health than members of the ‘Healthy’ profile but health similar to members of the ‘High Intake’ profile.
Table 4 Respondent characteristics by latent dietary profile for American adults aged 65 years or older, 2012 Health and Retirement Study/2013 Health Care and Nutrition Study*

TICS, Telephone Interview of Cognitive Status; HS, high school; ADL, activities of daily living.
a,b,c,d,e Mean values within row with unlike superscript letters were significantly different (α level for statistical significance identified with Bonferroni correction).
* All estimates and statistical tests excluding sd account for the complex survey design.
† Mean and sd reported for non-log-transformed food groups, statistical tests based on log-transformed food groups.
‡ Mean and sd reported for non-log-transformed household income and assets, statistical tests based on log-transformed household income and assets. Mean and sd for household income and assets are reported in thousands of $US.
§ Overall sample size for TICS was 3394 owing to some items used in the summary score not being asked of all Health and Retirement Study participants.
║ Observed cell frequency significantly greater than expected.
¶ Observed cell frequency significantly less than expected.
Older adults in the ‘High Intake’ dietary profile had the greatest likelihood of being White, being married or partnered, and had the greatest reported household income of all profiles, but otherwise had SES similar to those in the ‘Healthy’ profile. Those in the ‘High Intake’ profile were relatively unlikely to report currently smoking, were the most likely to report moderate alcohol consumption, and had a relatively high likelihood of reporting vigorous physical activity. Members of the ‘High Intake’ profile had lower self-rated health and TICS cognitive scores, more chronic health conditions, and greater likelihood of reporting an ADL limitation than those in the ‘Healthy’ profile. Finally, the ‘Low Intake’ dietary profile had a greater proportion of Hispanic and Other race/ethnicity respondents than expected, and members had a relatively low likelihood of being married. ‘Low Intake’ older adults also reported the fewest monetary resources, were the most likely to report not having a high-school degree, and the least likely to report white-collar occupational tenure of all dietary profiles. The ‘Low Intake’ profile was also characterized by the greatest likelihood of food insecurity, smoking and reporting no physical activity. ‘Low Intake’ older adults also generally had the worst health status observed, with 20·0 % of respondents reporting at least one limitation in ADL.
Energy, macronutrient and micronutrient intakes by dietary profile
Table 5 presents estimated daily energy intake, macronutrient density, macronutrient intake, micronutrient intake, and both RMR and the ratio of estimated daily energy intake to RMR by dietary profile. Energy intake differed significantly across all profiles with the ‘High Intake’ group having an average energy intake 2768·1 kJ (661·6 kcal) greater than next highest group, the ‘Western’ profile. Carbohydrate and protein macronutrient density were generally similar across dietary profile and members of the ‘Western’ and ‘High Intake’ profiles had the greatest saturated fat energy density. Older adults in the ‘High Intake’ profile had the greatest consumption of fibre, overall n-3 fatty acids, added sugar, n-6 fatty acids, trans fat and cholesterol. Compared with the ‘High Intake’ group, ‘Healthy’ profile members consumed significantly less n-6 fatty acids, trans fat and cholesterol. Individuals with a ‘Western’ diet had relatively high intake of added sugar, trans fat and cholesterol considering that their average energy intake was similar to members of the ‘Healthy’ profile. The ‘Low Intake’ profile had on average the lowest intake of macronutrients although members consumed more added sugar than those in the ‘Healthy’ profile. Members of the ‘High Intake’ profile had the greatest micronutrient intake while members of the ‘Low Intake’ profile generally had the lowest intake of micronutrients. Across all dietary patterns, intakes of Ca, K, folate, vitamin D and vitamin E were below Dietary Reference Intakes. Members of the ‘Western’ and ‘High Intake’ profiles reported consuming a similar number of foods, with those in the ‘Low Intake’ profile having the least diverse food intake. Estimated RMR was lower in the ‘Healthy’ profile than in other profiles, and regarding the energy intake to RMR ratio, members of the ‘High Intake’ profile had estimated energy intake about 70 % greater than RMR. The energy intake to RMR ratio for members of the ‘Healthy’ and ‘Western’ profiles was about 1·2 (sd 0·5), and that for members of the ‘Low Intake’ group was 0·9 (sd 0·4).
Table 5 Energy intake, macronutrient density, macronutrient intake and micronutrient intake by latent dietary profile for American adults aged 65 years or older, 2012 Health and Retirement Study/2013 Health Care and Nutrition Study*

%E, percentage of energy.
a,b,c,d Mean values within row with unlike superscript letters were significantly different (α level for statistical significance identified with Bonferroni correction).
* All estimates and statistical tests excluding sd account for the complex survey design.
Discussion
Using a sample of community-dwelling US adults aged 65 years or older, we identified four latent dietary profiles that were characterized by either type or quantity of foods being consumed. Profile membership was associated with the respondents’ demographic and socio-economic characteristics, health behaviours and indicators of health status. Macronutrient and micronutrient intakes varied as expected across dietary profile, although intake of several key nutrients fell below acceptable ranges for all groups. Our findings support the use of empirical approaches to identify dietary patterns in diverse populations and call for further analyses of the association between empirically derived dietary patterns, socio-economic context and health outcomes among older adults using the HRS and HCNS.
The latent dietary patterns we identified in the HCNS reflect two distinct taxonomies of dietary intake. The first is based on preferential selection of certain food groups over others. Members of the ‘Healthy’ dietary profile appeared to selectively consume foods known to support health and well-being, while members of the ‘Western’ dietary profile displayed low intake of vegetables and fruits and high intakes of red meat and processed foods. SES and health behaviours were associated with dietary pattern membership, with ‘Healthy’ profile members reporting relatively high SES and favourable health behaviours compared with ‘Western’ profile members. The ‘Western’ dietary pattern contained the greatest proportion of respondents, a concerning outcome given established associations between ‘Western’ dietary habits and increased risk of chronic disease(Reference Zhang, Shu and Si37–Reference Gougeon, Payette and Morais39).
The remaining dietary profiles were characterized by the overall amount of food being consumed. The ‘High Intake’ dietary profile contained the second-largest proportion of older adults and was characterized by the highest daily consumption of almost all food groups. The ‘High Intake’ group had relatively high SES and reported generally healthful behaviours, but also reported lower subjective health, greater chronic disease burden, lower cognitive scores, and had a greater likelihood of reporting an ADL limitation than those in the ‘Healthy’ profile.
The final and potentially most problematic dietary pattern identified was characterized by overall low food intake, likely reflecting a population at risk of nutritional deficiencies and poor health. ‘Low Intake’ older adults consumed the fewest vegetables, fruits and legumes of all profiles, reported relatively low consumption of red meat and processed foods, and intake of other foods was also generally low. The dietary habits and health behaviours of those in the ‘Low Intake’ profile occurred in a setting that reflected restricted access to socio-economic resources, which were coupled with the worst self-rated health, highest levels of physical disability and lowest cognitive scores of all dietary profiles. Notably, the average rate of food insecurity in this profile was 18·6 %, substantially greater than estimates drawn from comparably aged US populations(Reference Goldberg and Mawn40,Reference Strickhouser, Wright and Donley41) . Under-reporting of dietary intake may contribute to the low intake of foods observed in this group, although we believe older adults in the ‘Low Intake’ dietary profile appear particularly vulnerable and likely represent those who would most benefit from nutritional interventions.
Dietary profile membership was associated with differing macronutrient and micronutrient intakes that may place individuals at varying risk of chronic disease. The ‘Western’ and ‘High Intake’ patterns had the highest intakes of saturated fat, trans fat and cholesterol, likely related to high intake of red meat and processed foods. High intake of trans fat has been associated with increased risk of CVD(Reference Li, Hruby and Bernstein42,43) , and although the relationship between SFA and CVD is inconsistent, research suggests replacing SFA with PUFA may reduce the risk of CVD(Reference Li, Hruby and Bernstein42,43) . Fibre is associated with prevention or treatment of chronic diseases such as CVD, diabetes, colon cancer and obesity through health benefits that include weight control, regulation of blood glucose and lowering of cholesterol(Reference Timm and Slavin44). Daily fibre intake was below the Dietary Reference Intake in the ‘Western’ and ‘Low Intake’ patterns, reflecting insufficient consumption of whole grains, fruits and vegetables(45).
Although the consumption of nutrients varied across profile, important similarities reflecting inadequate intakes of key nutrients emerged. All dietary patterns identified fell within the Acceptable Macronutrient Distribution Ranges for protein and carbohydrate(43), although micronutrient adequacy is dependent on the nutrient density of the foods consumed. All dietary patterns were below Ca (1200 mg/d) and vitamin D (15–20 µg/d (600–800 IU/d)) recommendations for older adults(45,46) . The risk for osteoporotic fractures increases with age and inadequate amounts of either Ca or vitamin D can increase fracture risk(Reference Weaver, Alexander and Boushey47). In addition, vitamin D deficiency has been shown to increase the risk for cognitive decline and dementia in older adults(Reference Miller, Harvey and Beckett48,Reference Annweiler, Dursun and Féron49) . All profiles had low intakes of dairy products and few other foods contain Ca or vitamin D; therefore, adequate intakes of these nutrients may be difficult to achieve in older adults. Like other national estimates(Reference Zhang, Cogswell and Gillespie50), all dietary profiles had inadequate K intakes.
Strengths and limitations
The strengths of our study include the empirical identification of dietary profiles in a large sample of community-dwelling older Americans and analysis of an under-utilized data source allowing the linkage of dietary information with diverse indicators of SES and health. Compared with predefined dietary scores such as the Mediterranean diet or Healthy Eating Index, we identified dietary patterns reflecting both nutritional quality and overall quantity; outcomes that may be conflated when using predetermined dietary indices. Particularly important was the identification of ‘Low Intake’ older adults who appear to be at the greatest risk of nutritional deficits, low SES and poor health.
Our study also has important limitations to consider. The use of an FFQ to estimate dietary intake relies on the respondent to accurately report dietary intake, which could introduce measurement error through incorrect recall or different interpretations of the FFQ. Excluding proxy responses to the HRS and HCNS as well as removing observations with estimated daily energy intake falling outside the commonly used acceptable range have helped reduce the possible influence of measurement error on our findings, although our results may still be subject to bias due to under/over-reporting of dietary intake. In this context, the use of LPA to identify heterogeneous mixtures of dietary intake in the HCNS is an effective means of separating dietary responses distinguished by preferential consumption of certain types of foods (i.e. members of the ‘Healthy’ and ‘Western’ profiles) from those reporting either low or high intake of all foods. Those identified as members of dietary patterns distinguished by low or high intake should be further examined to differentiate those providing biased reports of dietary intake from those truly at risk of diseases related to excessive dietary intake or malnutrition. In particular, a combination of education, race/ethnicity, and lower cognitive and physical function appear to be salient in identifying members of the ‘Low Intake’ group. Members of this group may have had different interpretations of the FFQ, difficulties in accurately completing the questionnaire, or potentially found the foods included in the FFQ less representative of their dietary intake.
In addition to the issue of measurement error, longitudinal measures of dietary intake would allow clearer identification of associations between dietary patterns, socio-economic context and health, and the ability to examine co-occurring changes in dietary habits and health over time would provide useful information about the efficacy of nutritional interventions. Also, being representative of only community-dwelling older Americans, the HRS and HCNS do not reflect the experiences of institutionalized older adults who are likely to be at the greatest risk of nutritional deficiencies and poor health. Exclusion of proxy responses likely magnifies the fact that our results are reflective of healthier, less-disabled older Americans. Additional studies are required to provide support for the representativeness of the dietary profiles we identified.
Our identification of dietary patterns among members of the rapidly expanding population of ageing Americans is a useful contribution for those who study the convergence of dietary habits, socio-economic constraints and health outcomes among older adults. The LPA approach to identifying heterogeneous dietary patterns proved useful in our research and should be applied to other sources of dietary data representing the diverse settings where older adults around the world live. Finally, the ability to link dietary information from the HCNS to longitudinal measures of SES and health in the HRS provides a unique opportunity to investigate the role of dietary intake in the changing health of America’s ageing population.
Acknowledgements
Financial support: This research was supported by the Texas State University Research Enhancement Program. The Texas State University Research Enhancement Program had no role in the design, analysis or writing of this article. Conflict of interest: There are no conflicts of interest. Authorship: N.J.B. and K.E.Z. designed the research and N.J.B., K.E.Z. and C.M.R. wrote the paper; N.J.B. analysed the data and N.J.B. has primary responsibility for final content. All authors read and approved the final manuscript. Ethics of human subject participation: This study was conducted according to the guidelines laid down in the Declaration of Helsinki and all procedures involving human subjects were approved by the University of Michigan’s institutional review board. Verbal informed consent was obtained from all subjects and was witnessed and formally recorded.
Author ORCID
Nicholas J Bishop, 0000-0002-1253-892X.