Introduction
Once the genetic code was deciphered, it was quickly recognized that the code matrix was decidedly nonrandom. Elucidation of the underlying causes of this surprising regularity has been described as ‘The Universal Enigma’. Thought leaders such as Francis Crick, Manfred Eigen, Ed Trifonov, and others (see reviews/overviews and references cited therein) (Crick, Reference Crick1968; Eigen and Winkler-Oswatitsch, Reference Eigen and Winkler-Oswatitsch1992; Trifonov, Reference Trifonov2000, Reference Trifonov2004; Koonin and Novozhilov, Reference Koonin and Novozhilov2009), proposed fundamental, physio-chemical frameworks to explain the origin and evolution of the code, including error minimization (Freeland et al., Reference Freeland, Wu and Keulmann2003; Novozhilov and Koonin, Reference Novozhilov and Koonin2009), stereochemical (Yarus et al., Reference Yarus, Caporaso and Knight2005; Polyansky and Zagrovic, Reference Polyansky and Zagrovic2013; de Ruiter and Zagrovic, Reference de Ruiter and Zagrovic2015), and coevolution theories (Di Giulio, Reference Di Giulio2004; Wong, Reference Wong2005). They sought to explain the near singularity of the code across most living organisms, out of 1084 alternative possibilities (Koonin and Novozhilov, Reference Koonin and Novozhilov2009). To this end, a thermodynamic perspective/framework helps rationalize the origins and evolution of the genomic energy landscape, in which differential energy profiles correlate with differential biological outcomes.
Biophysical origins and evolution of the genetic code
Within the context of an energy-based perspective for the origin and evolution of the genetic code, one can hypothesize that the original ancestral codons were comprised of a family of ‘prebiotic’ duplexes of sufficient stability to avoid dissociation from their antiparallel, complementary codons. One might reasonably envision that such codon couplets would code for the most ancient amino acids (Miller, Reference Miller1953; Miller and Urey, Reference Miller and Urey1959; Miller et al., Reference Miller, Urey and Oro1976; Trifonov and Bettecken, Reference Trifonov and Bettecken1997; Trifonov, Reference Trifonov2000, Reference Trifonov2004) if given the proper translational machinery. This core of ‘prebiotic’ ‘codon duplexes’ could have produced the rest of the code through a sequential series of transition and transversion mutations, the order of which is controlled/regulated/influenced via families of interlocking energy cycles. In this way, one can converge to a nearly singular genetic code out of the potential of more than 1084 alternative code tables (Novozhilov et al., Reference Novozhilov, Wolf and Koonin2007; Koonin and Novozhilov, Reference Koonin and Novozhilov2009). Such energy-driven convergence of an astronomical number of potential alternative ‘states/codes’ into a single, unique ensemble ‘state/code’ is reminiscent of the energy landscape funnel associated with the protein folding phenomenon (Dill and Chan, Reference Dill and Chan1997). Alternatively, one could argue that evolution was ‘stuck with’ whatever code developed by chance in the primordial environment. From that code, sequential mutations may have worked to drive the code toward evolutionarily traceable changes. Thus, once organisms started using such codes to connect replication (and transcription) to translation, they evolved more rapidly toward a thermodynamically driven code. We prefer the former perspective of an early stage, energy-driven, nonrandom evolutionary shaping of the genetic code through a series of mutations within particularly stable, prebiotic ‘codon duplexes’, all controlled via families of interlocking energy cycles.
Toward a thermodynamic contribution to the genetic code and to molecular Darwinism
In the classic view of Darwinian evolution (Darwin, Reference Darwin1859), a phenotypical characteristic of a species that imparts a survival advantage in a given environment persists through generations. By contrast, the population of those species variants that lack such an advantageous characteristic disappears over time. This ‘survival of the fittest’ phenomenon is what gives rise to what Darwin referred to as ‘natural selection’.
A necessary correlate to such a view of evolution is that the selective survival of the phenotypically advantaged form of a species yields enrichment within the species of genotypic signatures that correspond to production of (coding for) the advantageous phenotypical characteristics. Within such a framework, one could envision the generational persistence of certain species' characteristics that not only provide selective advantage for survival within a given environment, but which also are associated with particularly stable genetic signatures (codes) that resist alterations and thus enable generational persistence. As such, evolution may result from a mixture of contributions from classic, natural selection, Darwinian theory, as well as from what can be called ‘molecular Darwinism’ (Eigen, Reference Eigen1976), or ‘Watson–Crick Darwinism’. In the latter context, some phenotypical characteristics might persist since they are coded for by more stable domains in the genome, even if such characteristics do not maximize species survival. In the classic evolutionary context, characteristics that provide a survival advantage will generationally persist, with no consideration for the stability of the genotypical signature. The net outcome of species evolution may well reflect contributions from both phenotypical (classic Darwinism) and genotypical (molecular Darwinism) influences.
To gain insight into such a DNA-based perspective, one can map the iconic chemical genetic code in terms of an energy code. Perhaps early evolution of the genetic code can be viewed in terms of the differential stabilities of codon/complementary codon couplets that form antiparallel trimeric duplexes, as opposed to exclusively in terms of the functionality of any gene product.
The genetic code as an energy code
In Table 1, the classic Crick genetic code matrix (Crick, Reference Crick1968) is elaborated by also listing the stabilizing free energy values for each fully paired codon/complementary codon antiparallel trimeric duplex. These stability parameters were calculated using calorimetrically-derived free energy values previously reported by our labs (Breslauer et al., Reference Breslauer, Frank, Blocker and Marky1986). The decision to map the genetic code in terms of codon/antiparallel complementary codon energetics is justified on multiple physio-chemical levels. First, trimeric duplexes formed from association of fully complementary, antiparallel, codon pairs, using up to four ‘letters’, reflect the minimum molecular information units required to code for the diversity of amino acids. Second, as demonstrated by Porschke and Eigen (Reference Porschke and Eigen1971), codon/complementary codon, antiparallel, trimeric duplexes possess the minimum stability required to form a complex of sufficient strength such that the associated species do not spontaneously dissociate into their constituent single-stranded components; a circumstance that makes them susceptible to degradation and thereby loss of coding information. On the other hand, making these interactions still more stable, as in a tetramer or higher chain length code, may well work against optimal rates of translation (see Greive and von Hippel, Reference Greive and von Hippel2005). In other words, in the current context, increased thermodynamic stability might work against the use of more stable (e.g. longer) codon–complementary codon interactions in ‘molecular evolution’. Third, it has been suggested that in primitive primordial molecular machines, translocation events in steps of 3 monomer units (i.e. the size of the codon) correspond to local energy minima that are favored over other translocation step sizes (Aldana et al., Reference Aldana, Cázarez-Bush, Cocho and Martı́nez-Mekler1998; Martinez-Mekler et al., Reference Martinez-Mekler, Aldana, Cazarez-Bush, Garcia-Pelayo and Cocho1999; Aldana-González et al., Reference Aldana-González, Cocho, Larralde and Martinez-Mekler2003). It is within this context, that stability data for each antiparallel, codon/complementary codon interaction are shown in Table 1. In this format, one can explore relationships between the differential trimeric duplex stabilities formed from codons and their corresponding complementary codons and biological observations/outcomes.
Table 1. Genetic code matrix annotated with trimeric duplex stabilities formed between codons and their corresponding, antiparallel complementary codons
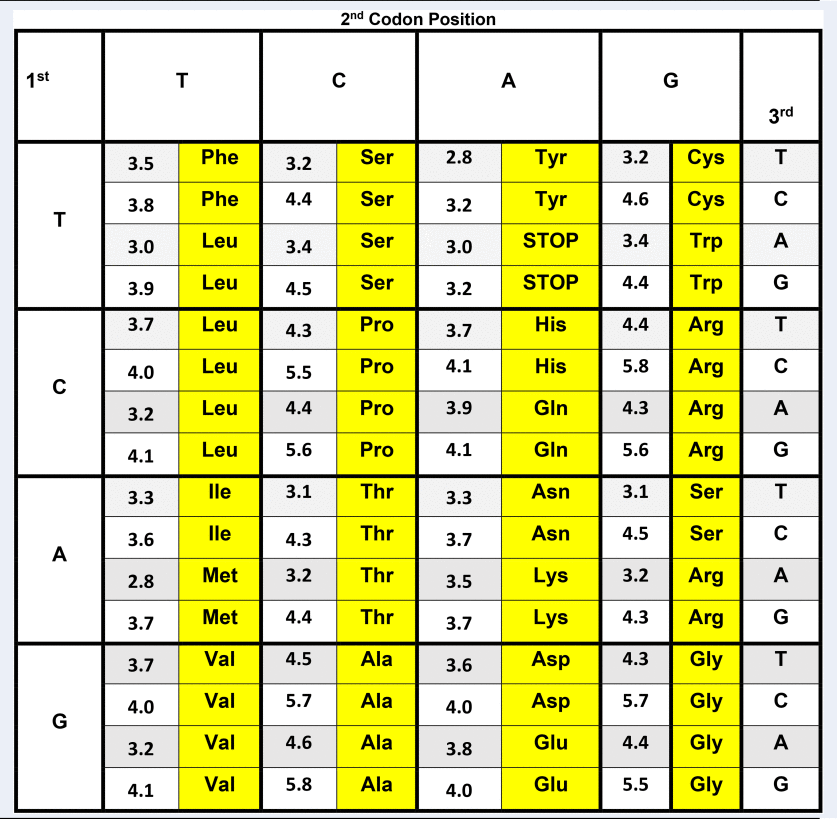
Genetic code matrix for human mitochondrial DNA annotated with stabilizing free energy values for the trimeric duplexes formed by antiparallel, complementary codons. The free energy values were calculated based on the calorimetrically determined nearest-neighbor dataset reported by Breslauer et al. (Reference Breslauer, Frank, Blocker and Marky1986). To reduce the impact of end effects, the normalized, weighted average of the duplex free energies were calculated, with each terminus hypothetically ‘sealed’ by all possible base pairs/stacks. The net impact of this end effect correction is to dampen the variability between codons, and to reduce the dominance of the central base in the codon trimer caused by it being the only base with two neighbors. Significantly, however, aside from modest compression of the stability range, the rank order of the differential codon duplex stabilities listed, as well as the general correlations noted here remain unaltered, even when no end effect ‘correction’ is applied. Compilations of the codon free energy data employing any of the other commonly used nearest-neighbor databases results in some numerical and rank order differences, reflective of subtle differences in the numerical values assigned to nearest-neighbors in these different databases (e.g. Delcourt and Blake, Reference Delcourt and Blake1991; Doktycz et al., Reference Doktycz, Goldstein, Paner, Gallo and Benight1992; SantaLucia et al., Reference SantaLucia, Allawi and Seneviratne1996; SantaLucia, Reference SantaLucia1998). That said, the nearest-neighbor data of Ritort and Bustamante, derived from force stretching experiments, exhibit the most concurrence with the relative trends reported here; specifically in terms of the free energy rank ordering of the codons, as well as the codon usage patterns (Huguet et al., Reference Huguet, Bizarro, Forns, Smith, Bustamante and Ritort2010). Given Ritort's subsequent assessment of the differential impact of magnesium ion on the nearest-neighbor data, future studies should also consider measurements in a variety of counterion/cation environments (Huguet et al., Reference Huguet, Ribezzi-Crivellari, Bizarro and Ritort2017).
The genetic code matrix shown in Table 1 corresponds to the human mitochondria code (Anderson et al., Reference Anderson, Bankier, Bankier, Barrell, de Bruijn, Coulson, Drouin, Eperon, Nierlich, Roe, Sanger, Schreier, Smith, Staden and Young1981; Breitenberger and RajBhandary, Reference Breitenberger and RajBhandary1985). Note that the smallest interaction free energy (least stable), ΔG, of 2.8 kcal per mole triplet is between the alternating sequences ATA and TAT, and the highest (most stable) ΔG value is 5.8 kcal per mole triplet for the duplex formed by the alternating sequences GCG and CGC.
Energy dispersion of the codon/complementary codon, trimeric duplexes
The 64 possible DNA triplet codons collectively constitute the minimal ‘words’ of the genetic code. When evaluated as bound to their fully complementary, antiparallel codons, they form 32 trimeric duplexes, each with a calculated stability. As summarized in Scheme 1 heat map, this process reveals a broad stability dispersion of the trimeric duplexes formed by the codon/complementary antiparallel codon. Such significant energy dispersion makes these differential energy profiles information-rich.
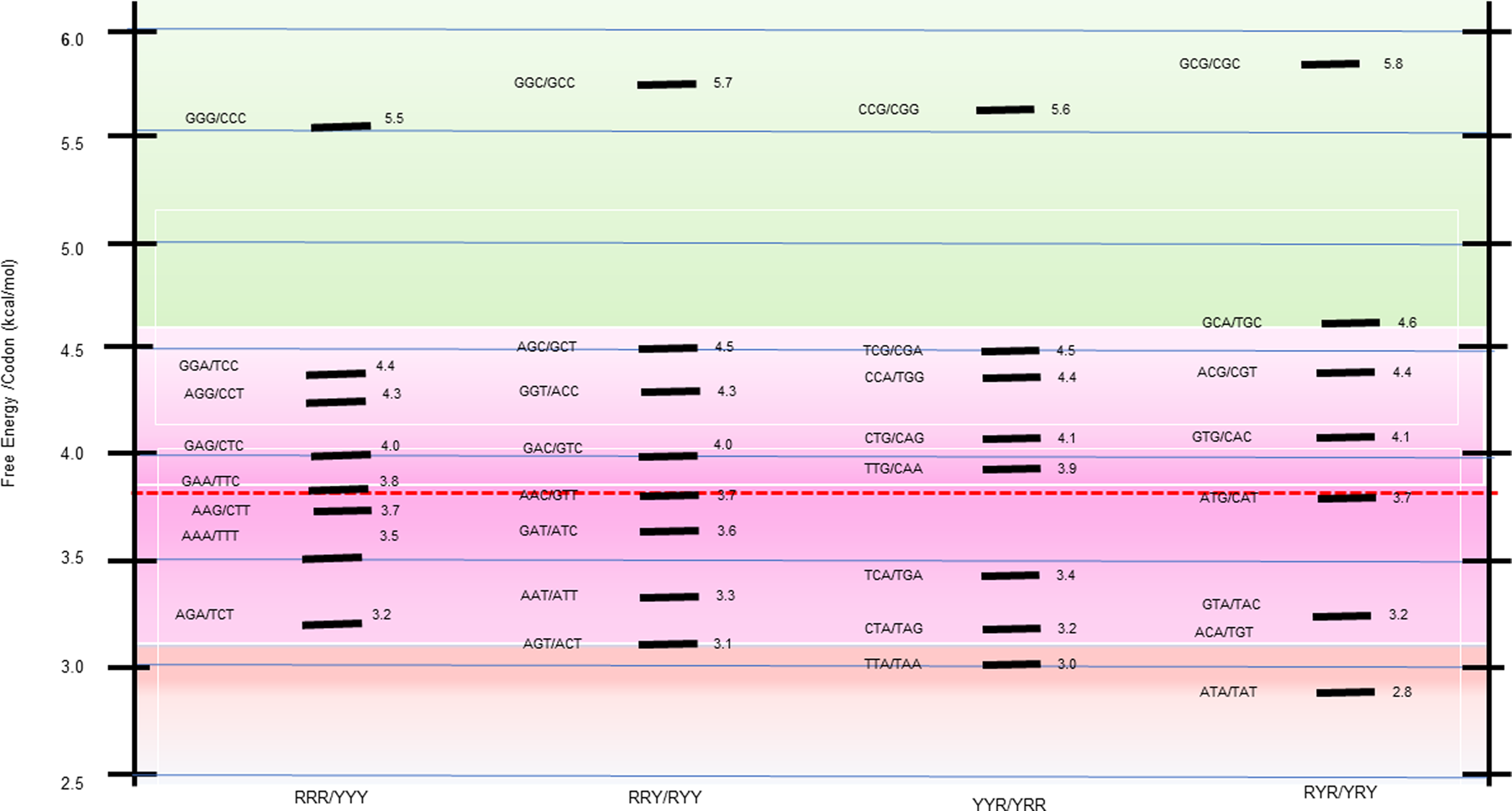
Scheme 1. Free energy distribution spectrum for the 32 trimeric duplexes formed by all 64 complementary codons. The stability distribution is color coded as a ‘heat map’, with the GC-rich most stable family (highest free energy of trimeric duplex formation) highlighted toward the top of the scheme in light green; the next most stable family is highlighted in light purple; and the less stable duplexes relative to the mean are highlighted in light red. The energy spectrum is formatted within four columns that reflect the purine (R)/pyrimidine (Y) sequence patterns designated at the bottom of the scheme.
Correlations between codon usage frequencies and the stabilities of the duplexes formed by each codon and its antiparallel complementary codon
Comparisons between the codon/complementary codon free energy data and the whole genome codon usage frequencies reported by Futcher and coworkers (Gardin et al., Reference Gardin, Yeasmin, Yurovsky, Cai and Futcher2014) for yeast Saccharomyces cerevisiae reveal an intriguing coupling of properties. By combining the differential stabilities and Futcher's datasets, one observes a near linear correlation between the frequency with which a given codon is used for a particular amino acid and the corresponding codon/complementary codon free energy. To be specific, save for isoleucine, of the 17 out of 20 amino acids for which Futcher reports sufficient data density, we observe that codons with lower free energies (less stable) are used more frequently than codons for the same amino acid with higher free energies (more stable). This coupling of a fundamental physio-chemical property with the outcome of a complex biological process is illustrated for several amino acids in the plots shown in Fig. 1. Such empirical correlations reinforce reductionists' efforts to rationalize complex biology in terms of fundamental chemical principles.
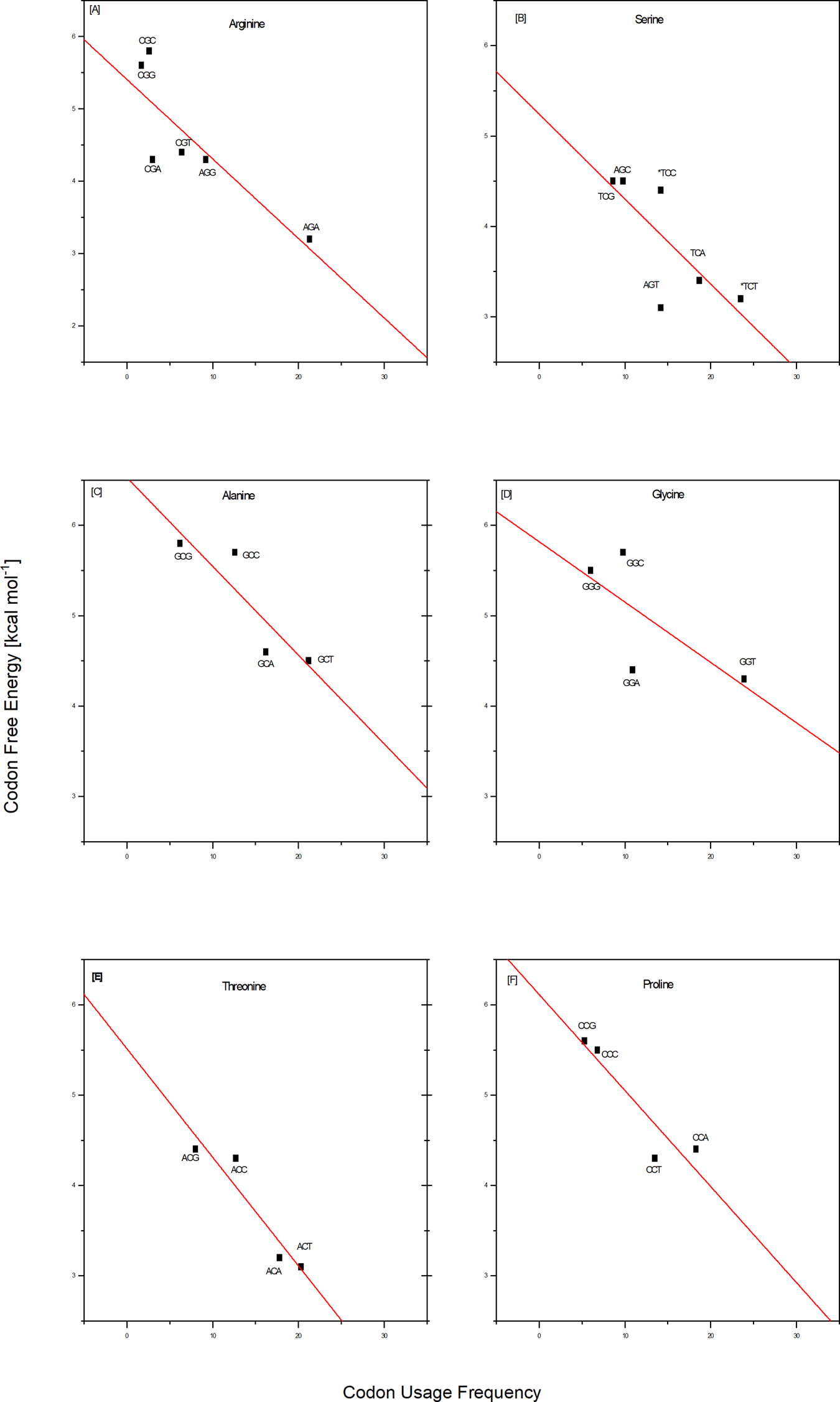
Fig. 1. Empirical correlations between whole genome codon usage frequencies in S. cerevisiae taken from the work of Futcher and coworkers (Gardin et al., Reference Gardin, Yeasmin, Yurovsky, Cai and Futcher2014) and the corresponding codon/complementary codon free energies of this study. Each red line represents a best fit to the equation for a straight line of these two independently derived data sets. The result shown here are for two of the three amino acids encoded by six codons, and for four of the five amino acids encoded by four codons. This selection corresponds to that subset of the amino acids judged most ancient, based on a meta-analysis reported by Trifonov (Reference Trifonov2000, Reference Trifonov2004). With the exception of isoleucine, and the insufficient data density for methionine and tryptophan, all of the amino acids encoded by only two codons also show a preference for higher codon usage frequency that correlates with lower codon free energy. For a thermodynamic argument, one strictly should use a log scale plot for the usage frequency. However, over the small data range assessed here, we have confirmed that one cannot distinguish between linear and log linear, with the log plot simply compressing the data.
Based on this coupling, one might speculate, that the degeneracy associated with the use of multiple codons of differential stabilities to code for the same amino acid reflects a form of thermodynamic selection; one in which codon energetics is more determinatory of usage frequency than a codon's chemical syntax alone. It will be instructive to probe the extent to which this empirical correlation between codon stabilities and codon usage frequencies is universal across all organisms and genes, as well as to define the biological implications. For now, this correlation provides an example of insights that can be gained by parsing the iconic genetic code in terms of energy differentials. Conversely, one also might posit that the usages of codons reflect biologically relevant features of those DNA sequences containing a statistical overabundance of energetically favorable or unfavorable codons. The altered energy profiles of such DNA sequences relative to a statistically expected distribution of codons/energies may reflect the existence of biological constraints that do not apply to an average sequence. In other words, codon usage that deviates from the average expected distribution (either positive or negative) may reflect altered biological constraints.
‘Evolving’ all codons from each other through sequential series of transitions and transversions
To illustrate the cycles associated with interconverting codons for the entire genetic code, the 64 codons presented in Table 1 and Scheme 1 can be arranged into a total of eight ‘octets’. Each octet is composed of codons located at one of the eight apices of a cube. Each cube corresponds to one of the purine (R)/pyrimidine (Y) sequence patterns designated in Scheme 1. The resultant eight octet cubes are then positioned at the corners of a master scaffolding cube to create a ‘hypercube’ as shown in Fig. 2. This hypercube illustrates the full cascade of all of codon interconversions via sequential site changes over all codon sequence space.
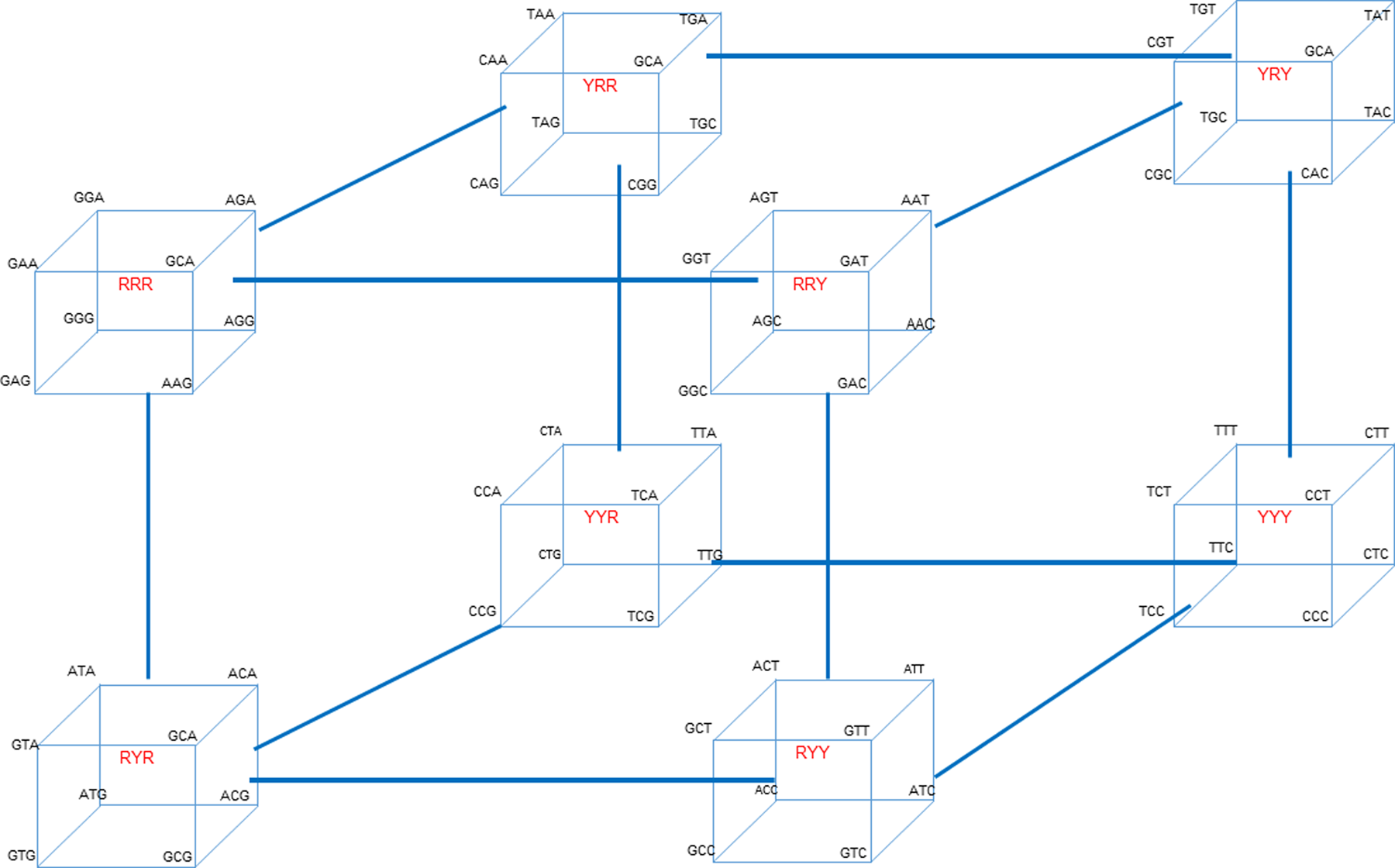
Fig. 2. The hypercube of all eight cube octet sequence classes (shown in red) located at each apex of the hypercube, illustrating the interconnectedness of the cycles associated with the full cascade of codon interconversions via sequential site changes. Transition mutations occur within a cube, while transversion mutations link one cube to another.
Note that the eight octet cubes shown in the hypercube of Fig. 2 are inter-cube related by codon transversion mutations, whereas codons within a given octet cube are intra-cube related by transition mutations. These stepwise interconversions create interlocking cycles that allow one to traverse/”evolve” the entire genetic code.
This stepwise generation/”evolution” of all 64 codons via sequential transition and transversion mutations, starting from any codon, may reflect a differential, energy-modulated evolution of the genetic code. As such, it might correspond to a biophysical basis for what Eigen referred to as ‘molecular Darwinism’. At the heart of molecular Darwinism is the generation of genetic variation. Genetic variants can result from DNA sequence alterations at local levels; from rearrangement of DNA segments intragenomically; and by gene transfer of foreign DNA. The hypercube illustrates how local sequence changes can interconvert all 64 codon variants via sequential cascades of transition and transversion steps that Table 1 shows exhibit differential free energies, thereby creating codon variants; a characteristic at the heart of molecular Darwinism.
Correlating trimeric duplex stability with amino acid coding properties
Inspection of the data in Table 1 and Scheme 1 reveals eight exceptionally stable all-GC codons, as defined by the relative stability of the trimeric duplex they each form with their antiparallel, complementary codon. By the same criteria, a second group of significantly stable codons of the form, GCX, CGX, GGX, and CCX, also can be identified, although less resolved, where X is either T or A. It is noteworthy that collectively the GCX, CGX, GGX, and CCX families of stable codons code for Ala, Arg, Gly, and Pro, which are among the most abundant amino acids, and, save for Arg, also are considered ancient amino acids (Trifonov, Reference Trifonov2000). Furthermore, when X = A or T, the complementary codons to this second group are XCG, XGC, XCC, and XGG. Except for Trp, these codons code for the amino acids Cys, Ser, and Thr, which, like Ala, Gly, and Pro noted above all are defined as ancient amino acids (Trifonov, Reference Trifonov2000). This empirical correspondence between the stabilities of codons and the abundance as well as age of the amino acids for which they code raises the intriguing possibility of a stability-modulated, evolutionary shaping of the code.
These stable codon groups, and their corresponding, antiparallel codons, each occupy three positions within each of the eight cubes that make up the hypercube scaffolding that interconnect all 64 codons. This set of 24 ‘high-stability’, codon groupings may have been energetically favored ‘prebiotically’. As such, it is intriguing to note that all the other 40 codons can be generated/“evolved” from these most stable codons by, at most, three transition mutations; again suggestive of a stability-modulated, evolutionary shaping of the code.
To test the robustness of our conclusions, we conducted the same analyses for a dataset in which we reversed the polarities of the codon–complementary codon interactions to yield parallel codon couplets. This assessment yielded an altered energy spectrum, as well as changes in the stability rank order for the complementary codon couplets; particularly for the RRR/YYY and the YYR/RRY families of trimeric duplexes (columns 1 and 3 in Scheme 1). Further comparison of the antiparallel and parallel datasets also revealed differences in the energy changes associated with the sequential transition and transversion mutations. In the aggregate, these differential outcomes underscore the robustness of the correlations noted here between the stabilities of the antiparallel couplets and the shaping of the genetic code.
Correlations between larger domain DNA energy profiles and higher-order biological functions
One long-term goal is to define correlations between functional domains of the genome and the energy profiles of such domains. Some initial trends, that require further validation, include the suggestion by Klump and coworkers that protein-coding sequences predominately consist of codon domains of relatively uniform stability (Klump and Maeder, Reference Klump and Maeder1991). By contrast, Klump proposes that signal sequences exhibit less uniform and less stable domains, while also being more sensitive to local changes in cellular and sequence/structural environments, thereby allowing them to amplify a perturbation in a localized sequence. This biophysical behavior is what one would expect for a biological signal transducer. Coding sequences, by contrast, are less sensitive to environmental conditions, also consistent with their biological function to faithfully code for a protein. Much more research is required to establish a robust biophysical map of genomes to test such hypotheses. However, these intriguing early correlations should motivate such efforts, including a parallel analysis for RNA.
Concluding remarks
We have reviewed, presented, and integrated evidence that the iconic chemical genetic code also can be viewed as a differential energy code that influences biological outcomes. This perspective includes implications for differential, energy-based, molecular contributions to classic Darwinian evolutionary theory. In short, evolutionary pressures may well derive from the optimization of fundamental biophysical properties, as well as from the classic perspective of being driven to yield a functionally adaptive advantage for either a biopolymer or an organism.
Darwinian evolution, when proceeding over sufficiently long timeframes, leaves only the evolutionary ‘winners’ behind. This reality makes it difficult, if not impossible, to deduce, with any certitude, the precursors or evolutionary pathways that ultimately culminated in the current, evolved ‘winners’. However, by evoking the laws of thermodynamics, it becomes possible via considerations of thermodynamic selection and linkages, as illustrated in the hypercube of Fig. 2, to speculate on what may have preceded these ‘left behind winners’. It is precisely the beauty of thermodynamics that follows universal laws, under all conditions and times, that allows one to extrapolate backward from the ‘left behind winners’ to make informed speculations as to how these winners may have evolved from earlier remnants (molecular fossils).
Consistent with this perspective is our hypothesis that the evolution of the genetic code was shaped and modulated by the differential stabilities of complementary codons; a feature reflective of ‘molecular Darwinism’. As thermodynamic fingerprints of such an evolutionary influence, we found correlations between the free energies of formation of antiparallel, complementary DNA trimers and their codon usage frequency. We also noted correlations between the stabilities of complementary codon couplets and those that code for ‘ancient’ amino acids. Collectively, our observations are consistent with a scenario in which the genetic code, driven by differential codon stabilities, evolved under the influence and regulation of a series of interlocking thermodynamic cycles. We proposed that these coupled energy cycles controlled the transition and transversion mutations of a group of the 24 most ancient (‘prebiotic’) and stable codon pairs, ultimately yielding the complete 64 codon code; via a form of ‘thermodynamic selection’. As such, we suggested that the evolution of the genetic code exhibits contributions from both stability-driven ‘molecular’/genotypic Darwinism as well as the more traditional, phenotypic Darwinism. As we stated in the Abstract, yet worth repeating, it is not surprising that evolution of the code was influenced by differential energetics, as thermodynamics is the most general and universal branch of science that operates over all time and length scales.
Going forward
Given the correlative examples noted here, going forward it seems justified to create a comprehensive energy map of the human genome; or for that matter, the genome of any organism of interest. The differential energy domains so characterized may correlate with known functionalities; or may reveal and yield insights into regions of yet defined function. Such profiling to create an ‘energy genome’ would yield a thermodynamic bridge between sequence, structure, and biological function.
Postscript: Shortly after the beginning of the 20th century, Albert Einstein (Schilpp and Einstein, Reference Schilpp and Einstein1949) declared:
‘A theory is the more impressive the greater the simplicity of its premises, the more different kinds of things it relates, and the more extended its area of applicability. Therefore the deep impression that classical thermodynamics made upon me. It is the only physical theory of universal content which I am convinced will never be overthrown, within the framework of applicability of its basic concepts’.
As biophysical chemists, the authors consider the ultimate exemplar/test of this assertion to be the demonstration that the molecular language and complexities of biology embedded in the genetic code can be rationalized in terms of fundamental thermodynamic principles.
Financial support
This research was supported by grants from the NIH GM23509, GM34469, and CA47995 (to K.J.B.) and NRF (Pretoria, RSA) grant GUN 61103 to H.H.K.
Conflict of interest
The authors declare no conflict of interest.