1. Introduction
Language massive open online courses (LMOOCs) are web-based online courses that offer unlimited access to language learning for a limited period primarily by higher education institutions catering to individuals seeking to learn a foreign language (Bárcena & Martín-Monje, Reference Bárcena, Martín-Monje, Martín-Monje and Bárcena2014; Gimeno-Sanz, Reference Gimeno-Sanz, Beaven and Rosell-Aguilar2021). LMOOCs have been growing steadily in popularity since their inception in 2012 (Sallam, Martín-Monje & Li, Reference Sallam, Martín-Monje and Li2022; Xue & Dunham, Reference Xue and Dunham2023; Zhang & Sun, Reference Zhang and Sun2023). However, concerns about their low completion rates (Duru, Sunar, White, Diri & Dogan, Reference Duru, Sunar, White, Diri and Dogan2019; Friðriksdóttir, Reference Friðriksdóttir2021; Wang, An & Wright, Reference Wang, An and Wright2018) and low levels of learning autonomy (Agonács, Matos, Bartalesi-Graf & O’Steen, Reference Agonács, Matos, Bartalesi-Graf and O’Steen2020) have called the quality of LMOOC teaching into question and spurred many researchers to study LMOOC learning behavior (e.g. Doğan, Sunar, Duru & White, Reference Doğan, Sunar, Duru and White2018; Martín-Monje, Castrillo & Mañana-Rodríguez, Reference Martín-Monje, Castrillo and Mañana-Rodríguez2018; Wang, Chen, Tai & Zhang, Reference Wang, Chen, Tai and Zhang2021; Zeng, Zhang, Gao, Xu & Zhang, Reference Zeng, Zhang, Gao, Xu and Zhang2022; Zhang & Sun, Reference Zhang and Sun2023). Mac Lochlainn, Nic Giolla Mhichíl and Beirne (Reference Mac Lochlainn, Nic Giolla Mhichíl and Beirne2021) recently called for LMOOC research to identify “which specific behaviours, both L2 oriented and not, are prevalent among LMOOC learners, to allow us to scrutinise and reflect on what types of specific, L2-directed patterns of participation might be established” (pp. 113–114).
However, research to date has focused on what might be called a “canon” of LMOOC learning behaviors that includes watching videos, reading class slides, taking quizzes, and participating in discussions in forums (e.g. Duru et al., Reference Duru, Sunar, White, Diri and Dogan2019; Martín-Monje et al., Reference Martín-Monje, Castrillo and Mañana-Rodríguez2018; Read & Bárcena, Reference Read and Bárcena2021), but broadly ignores learning check-ins. Learning check-ins denotes a relatively new type of learning behavior in which an LMOOC learner posts/shares part or all of their own learning record or information about their learning-related experience (Chen, Sun, Wu & Song, Reference Chen, Sun, Wu and Song2019). Although learning check-in behavior itself has become popular in many technology-assisted language-learning applications, and is a demonstrably effective aid to English learning (Nie et al., Reference Nie, Zheng, Zeng, Zhou, Lei and Wang2020), little is known about the effects of such behavior in LMOOC contexts.
Methodologically, when examining data produced by LMOOC learners, researchers frequently employ questionnaire-based surveys to assess learning behavior (e.g. Xu, Zhu & Chan, Reference Xu, Zhu and Chan2023). Compared to this indirect method, the use of learning analytics has greater potential to capture a more comprehensive picture of such behavior. Such an approach is perhaps especially well suited to analyzing MOOC data, insofar as MOOCs’ learning platforms naturally generate large datasets comprising authentic traces of online learning by their users (Beaven, Codreanu & Creuzé, Reference Beaven, Codreanu, Creuzé, Martín-Monje and Bárcena2014).
Learning check-ins have not to our knowledge been studied in previous LMOOC research. Our decision to adopt learning analytics to study this novel behavior was linked not only to our aim of painting a broad picture of it in a MOOC but also to our desire to test the relationships between such behavior, on the one hand, and, on the other, learning outcomes including both final exam grade and unit grades.
In addition, due to the exceptional diversity of LMOOC learners, some have argued that course-completion rates are not appropriate as the sole metric of LMOOC learning success, as long as learners achieve what they want to achieve (e.g. Jitpaisarnwattana, Darasawang & Reinders, Reference Jitpaisarnwattana, Darasawang and Reinders2022; Mac Lochlainn et al., Reference Mac Lochlainn, Nic Giolla Mhichíl and Beirne2021). Accordingly, the present study will include those who have engaged with the sampled LMOOC, regardless of their completion status. We expect that our results will help answer questions about the effectiveness of specific behaviors by LMOOC learners (Mac Lochlainn et al., Reference Mac Lochlainn, Nic Giolla Mhichíl and Beirne2021), thereby helping such learners make the most of their LMOOC experience (Appel & Pujolà, Reference Appel and Pujolà2021) and facilitating LMOOC educators’ provision of more innovative, engaging, and rewarding LMOOC learning to their students (Duru et al., Reference Duru, Sunar, White, Diri and Dogan2019; Friðriksdóttir, Reference Friðriksdóttir2021; Godwin-Jones, Reference Godwin-Jones2014; Hsu, Reference Hsu2023; Mac Lochlainn et al., Reference Mac Lochlainn, Nic Giolla Mhichíl and Beirne2021; Zhang & Sun, Reference Zhang and Sun2023).
2. Literature review
2.1 Learning behavior and autonomous learning in LMOOCs
Learning behaviors have always been a major topic of the LMOOC-development literature (Mac Lochlainn et al., Reference Mac Lochlainn, Nic Giolla Mhichíl and Beirne2021), and strong links have been found between particular behaviors and particular learning outcomes (e.g. Martín-Monje et al., Reference Martín-Monje, Castrillo and Mañana-Rodríguez2018). For example, Jitpaisarnwattana, Reinders and Darasawang (Reference Jitpaisarnwattana, Reinders and Darasawang2021) found that working in groups and making study plans were associated with completion rate in an LMOOC, while Zeng et al. (Reference Zeng, Zhang, Gao, Xu and Zhang2022) reported that LMOOC learners’ attention to the learning unit distinguished high performers from low ones. At the heart of learning behaviors in MOOC contexts is learner autonomy – that is, learners taking an active role in controlling and making choices and decisions regarding their own learning process, such as setting learning goals, deciding how, when, and where to engage with learning materials or support from teachers or peers as needed, etc. (Benson, Reference Benson2011; Huang & Benson, Reference Huang and Benson2013; Little, Reference Little1991). The massiveness and openness of MOOCs afford learners greater autonomy in directing their own learning path and deciding how to engage with a MOOC primarily at their own will, as indicated by diverse learning behaviors documented in the literature (e.g. Ding & Shen, Reference Ding and Shen2022; Hood, Littlejohn & Milligan, Reference Hood, Littlejohn and Milligan2015).
According to the relevant literature, when learning autonomously, a person remains attentive, motivated, and engaged by course-related materials or activities (Chacón-Beltrán, Reference Chacón-Beltrán2018; Ding & Shen, Reference Ding and Shen2022; Mac Lochlainn et al., Reference Mac Lochlainn, Nic Giolla Mhichíl and Beirne2021; Xu et al., Reference Xu, Zhu and Chan2023). For example, Read and Bárcena (Reference Read and Bárcena2021) showed how highly autonomous learners in a Spanish LMOOC studied diligently, submitted activities with very high rates of correctness, viewed almost all course videos, and remained active in discussion forums. In addition, learners showed their own wills in controlling and making decisions regarding their own learning process. For example, the students sampled by Agonács and Matos (Reference Agonács and Matos2019) actively sought opportunities to use the Italian language outside of their existing LMOOC context, and showed autonomy in engaging with different learning behaviors such as watching Italian movies or reading Italian newspapers and magazines. Taken together, these findings imply that design features and pedagogical practices aimed at encouraging LMOOC learning autonomy (i.e. learners’ control of their own learning) would tend to improve LMOOC learners’ outcomes.
2.2 The potential of check-in behavior to enhance language learning
Check-in behavior first came to prominence when certain mobile-phone applications allowed their users to share location-based information, mainly for social-networking purposes (Kim, Reference Kim2016). Gradually, people came to share not only location-based information but also sports records, learning records, pictures, and other types of personal news, motivated by desires including self-expression, information sharing, providing and seeking social support, and enjoyment (Luarn, Yang & Chiu, Reference Luarn, Yang and Chiu2015). Eventually, check-ins were formally adopted by some English-language mobile-learning applications to enable their users to share their English-learning progress. Such apps generate screenshots of each user’s learning record (e.g. learning content, learning duration, or learning achievement) at regular intervals, at which point the learner in question can decide to click the share button to make the learning record appear on either the learning app or their other social networking sites (Nie et al., Reference Nie, Zheng, Zeng, Zhou, Lei and Wang2020). Additionally, some users choose to check in by sharing their records to their social networks without any such prompting.
To the best of our knowledge, however, only one prior study – by Nie et al. (Reference Nie, Zheng, Zeng, Zhou, Lei and Wang2020) – has explored the role of check-in behavior in language learning. It concluded that learners with positive attitudes toward check-in behavior and high levels of perceived behavioral control were more likely than others to check in with their English-learning records, suggesting a positive relation between autonomous learning and check-in behavior.
Though no prior study appears to have explored the role of check-in behavior in LMOOCs explicitly, the learning activity described by Jitpaisarnwattana et al. (Reference Jitpaisarnwattana, Reinders and Darasawang2021) was relatively similar. The language learners in their study took a MOOC with the aim of developing their English presentation skills and were given recommendations by the learning system once they had uploaded their first video assignment. Then, they created individual learning plans (ILPs), taking account of specific system-recommended activities. We believe that the process of creating and discussing an ILP is fundamentally similar to check-in behavior, in that it involves learners’ (1) setting of personal learning goals based on their known language strengths and weaknesses; (2) autonomous cognitive engagement with learning resources that were not assigned; and (3) sharing evidence of having accomplished such extra work. However, the use of ILPs in Jitpaisarnwattana et al.’s study also differs from the check-in behavior in a typical LMOOC in terms of the formats used for sharing (i.e. uploading one’s final product vs. uploading one’s learning record), restrictions on language skills (i.e. presentation skills only vs. no skills restriction), and the format of the MOOC in question (i.e. cMOOC vs. MOOC in any other format, especially xMOOC). Among the format categories mentioned in the preceding sentence, cMOOCs emphasize strong community connection and extensive content contributions from the learners themselves, whereas xMOOCs focus on the transmission of large amounts of informational content and feature few chances for direct interaction between individuals (Fidalgo-Blanco, Sein-Echaluce & García-Peñalvo, Reference Fidalgo-Blanco, Sein-Echaluce and García-Peñalvo2016). This warrants further study of the effects of check-in behavior, defined in the present study’s LMOOC context as students’ spontaneous uploading and sharing of their own learning records.
2.3 The use of learning analytics in LMOOC studies
Aided by advancements in information technology, today’s learning management systems provide extensive new opportunities for researchers to discern patterns of online learning (Gašević et al., Reference Gašević, Dawson and Siemens2015). By enabling accurate interpretation of such data, learning analytics help to identify predictors of learning success and is increasingly used for decision-making about both instructional design and resource allocation (Gelan et al., Reference Gelan, Fastré, Verjans, Martin, Janssenswillen, Creemers, Lieben, Depaire and Thomas2018; Leitner, Khalil & Ebner, Reference Leitner, Khalil, Ebner and Peña-Ayala2017). In LMOOC research specifically, there is a similar interest in taking advantage of learning platforms’ rich stores of data to analyze learning patterns, behaviors, and outcomes. However, the number of studies that have used learning analytics to study effective learning within LMOOCs remains quite limited.
Within that small body of work, three key approaches can be identified. The first is the adoption of descriptive learning-analytics metrics to explore various forms of learning behaviors (e.g. Martín-Monje et al., Reference Martín-Monje, Castrillo and Mañana-Rodríguez2018; Read & Bárcena, Reference Read and Bárcena2021; Zeng et al., Reference Zeng, Zhang, Gao, Xu and Zhang2022). This answers the question of “what is happening in distance language learning,” via “the provision of more accurate quantitative analysis of online language learning behavior” (Gelan et al., Reference Gelan, Fastré, Verjans, Martin, Janssenswillen, Creemers, Lieben, Depaire and Thomas2018: 313). The second key approach is to use learning-platform data to examine the effects of certain behaviors on learning outcomes. For example, Duru et al. (Reference Duru, Sunar, White, Diri and Dogan2019) and Jitpaisarnwattana et al. (Reference Jitpaisarnwattana, Reinders and Darasawang2021) used log data to build statistical models of the relationship between course completion and various types of learning behaviors, including completion of particular learning activities, contribution to discussions, and student–student interactions, among others. The third approach consists of identifying variance in the learning-behavior patterns of different groups of students (e.g. Duru et al., Reference Duru, Sunar, White, Diri and Dogan2019; Zeng et al., Reference Zeng, Zhang, Gao, Xu and Zhang2022). For example, Martín-Monje et al. (Reference Martín-Monje, Castrillo and Mañana-Rodríguez2018) found statistically significant differences in the incidence of all log behaviors between successful and non-successful students.
To gain a better understanding of learners’ check-in behavior, the present study used learning analytics to look into all three of the research topics mentioned above. Our research questions were as follows:
-
RQ1: How and how often did learners autonomously check in about their learning progress while taking an intermediate-level English LMOOC?
-
RQ2: Did those learners who engaged in language-learning check-in behavior have a significantly higher course-completion rate than those who did not? And if so, to what extent can such behavior be said to encourage course completion?
-
RQ3: Among those learners who successfully completed the course, were there significant differences between the check-in and no-check-in groups in (a) learning outcomes (i.e. final exam grade and eight quiz grades) and/or (b) language-learning behaviors (i.e. total number of clicks on slides and announcements; total and per-unit times spent watching assigned videos)?
-
RQ4: Among all learners who engaged with the course in any discernible way, were there significant differences between the check-in and no-check-in groups in (a) learning outcomes (i.e. final exam grade and eight quiz grades) and/or (b) language-learning behaviors (i.e. the total number of clicks on slides and announcements; total and per-unit times spent watching assigned videos)?
3. Methodology
3.1 Research context
This study’s focal LMOOC was University English for Academic Purposes, as provided on XuetangX over a six-month period in 2019. Aimed at helping university students to master academic skills and join in academic conversations, it encouraged them to read, write and share their ideas while studying. The course was designed following an xMOOC format, focused on transmission of knowledge through embedded video lectures, computer-marked assignments, supplementary materials, and a discussion forum, among other features. As of May 25, 2023, various iterations of this LMOOC had provided learning services to 283,446 people worldwide. At the time of our data collection, it comprised eight learning units, each organized around a different research topic: cultural studies, life science, art, ecology, social issues, engineering and technology, psychology, and economics and management. Within each unit, the learning content was divided into 10 parts, including an introduction, listening, group discussion, topic and text, vocabulary, academic writing, a “skills power station,” student research projects, summary, and discussion.
As well as engaging in the above series of learning activities, there were discussion boards where learners could record and share their views with peers. They were also expected to complete relevant exercises and quizzes. After learning all eight units, students had the option of taking a two-hour online final exam that covered the language knowledge taught in the course. (This was required of those who wished to earn a course certificate.) The final exam score and the scores from the eight units were collected and used as indicators of learning outcomes in our further analyses.
3.2 Check-in behavior
At the start of the course, the teacher posted announcements encouraging the students to post about their learning records and about their learning progress in the discussion forum, regardless of whether the learning in question had been undertaken in or out of the LMOOC. Specifically, the teacher said, “This is totally voluntary work, and it depends on your time and learning interest. You are welcome to show your learning progress or learning record, either in this MOOC course or outside this MOOC course, in the discussion forum. When doing this, you might first want to think carefully about your own strengths and weaknesses in English and make your own check-in plan accordingly. The purpose of doing these check-ins is that I want to encourage you to learn English, and I also want to create a good learning environment for this course. This is not graded work, but I still hope to see your participation.” The teacher also set some rules for learning check-ins: students could either upload screenshots they felt were representative of their learning progress or write textual reports on how much and/or what they had learned.
3.3 Data mining
The initial learning-analytics data that we obtained from the learning platform took the form of a six-sheet Excel workbook that documented the respective logs of all 11,293 students. Each of its spreadsheets represented a separate data theme. We used Python software for data-mining processing (van Rossum & Drake, Reference van Rossum and Drake2009), and created a new dataset containing all 809,049 of the enrolled learners’ learning behaviors, learning outcomes, and check-in statuses.
Based on this first dataset, we created a second one relating specifically to those students who checked in. This was accomplished in the first instance via manual screening of all 2,224 posts in the discussion forum and extracting those that could be identified as check-ins (coded as 1; non-check-ins, as 0). The results were then triangulated by the researchers.
3.4 Measurement
3.4.1 Learning outcomes
A total of nine variables relating to the final exam and the eight unit-specific quizzes were used as learning-outcome indicators. In each unit, there were nine quiz questions aimed at assessing students’ understanding of the content covered in that unit. The maximum total quiz score for any one unit was therefore nine. The maximum total score for the final exam was 100. All exam questions were multiple choice.
3.4.2 Learning completion
Following Zeng et al. (Reference Zeng, Zhang, Gao, Xu and Zhang2022), we labeled learners as having completed the LMOOC if their final exam score was equal to or greater than 60 out of 100. Our data showed that among the LMOOC’s 11,293 learners, 1,046 completed it.
3.4.3 Learning behavior
A total of 11 variables were used as indicators of learning behaviors. They were grouped into three types: (1) total number of clicks on course slides, (2) total number of views of course announcements, and (3) overall and per-unit times spent watching course videos. The total length of the video provided by the course was 8 hours 48 minutes and 33 seconds (i.e. 31,713 seconds).
3.5 Data analysis
We used content analysis and descriptive statistics to answer our first research question. After extracting the posts that pertained to check-in messages, one of the authors categorized them based on whether their check-in content focused on in-LMOOC or out-of-LMOOC learning. In addition, within the out-of-LMOOC learning category, we categorized posts based on the language skills they mentioned: listening, reading, etc. The results were then triangulated by the other authors.
To answer our second research question, a chi-square test of independence was used to analyze inter-group differences in LMOOC completion and logistic regression applied to establish the extent to which check-in behavior encouraged learners to complete the LMOOC.
To answer our third research question, we first checked the distribution of the data. Specifically, we used Shapiro–Wilk tests of normality (Shapiro & Wilk, Reference Shapiro and Wilk1965) to check the distribution of the two independent groups’ final exam and unit scores, and the results indicated that all the tested variables significantly deviated from normal distribution (all p < .001). Therefore, a non-parametric technique, the Mann–Whitney U test (Mann & Whitney, Reference Mann and Whitney1947), was used in our subsequent analyses relating to this question.
To answer our final research question, we first distinguished between a group of learners who had engaged with the LMOOC in any discernible way and a group that had not obviously done so. Specifically, this was done by checking all students’ knowable learning behaviors (i.e. video-watching during each unit, slide accessing, announcement viewing, quiz-taking, and exam-taking) and classified those individuals who had not demonstrated any of them as not having engaged in the LMOOC at all. Among the 11,293 enrolled learners, 2,314 engaged. Within those 2,314, we further examined differences in learning behaviors across the check-in and non-check-in groups. We again conducted Shapiro–Wilk tests of normality and, as the results indicated that all tested variables significantly deviated from normal distribution (all p < .001), we then conducted Mann–Whitney U testing.
4. Results
4.1 An overview of learners’ check-in behavior
Of the 11,293 learners in our sample, 699 exhibited language-learning check-in behavior on the platform, representing a per capita check-in rate of 6.2%. Those learners who checked in did so a total of 1,633 times (M = 2.34). In terms of frequency, 663 of the 699 learners checked in 10 times or fewer; 21 of them did so 11–20 times; eight, 21–30 times; three, 31–40 times; one, 41–50 times; two, 51–60 times; and one, more than 60 times.
Figure 1 illustrates the content of these students’ check-in behaviors. Among the 1,633 check-in posts, the most frequently seen content type was self-reported LMOOC learning progress (n = 699, 42.8%). When posting check-in messages in this category, the students either posted a screenshot showing their current progress, generated by the learning platform, or simply reported their progress textually, such as by saying, “[Name] has completed Unit 1.”
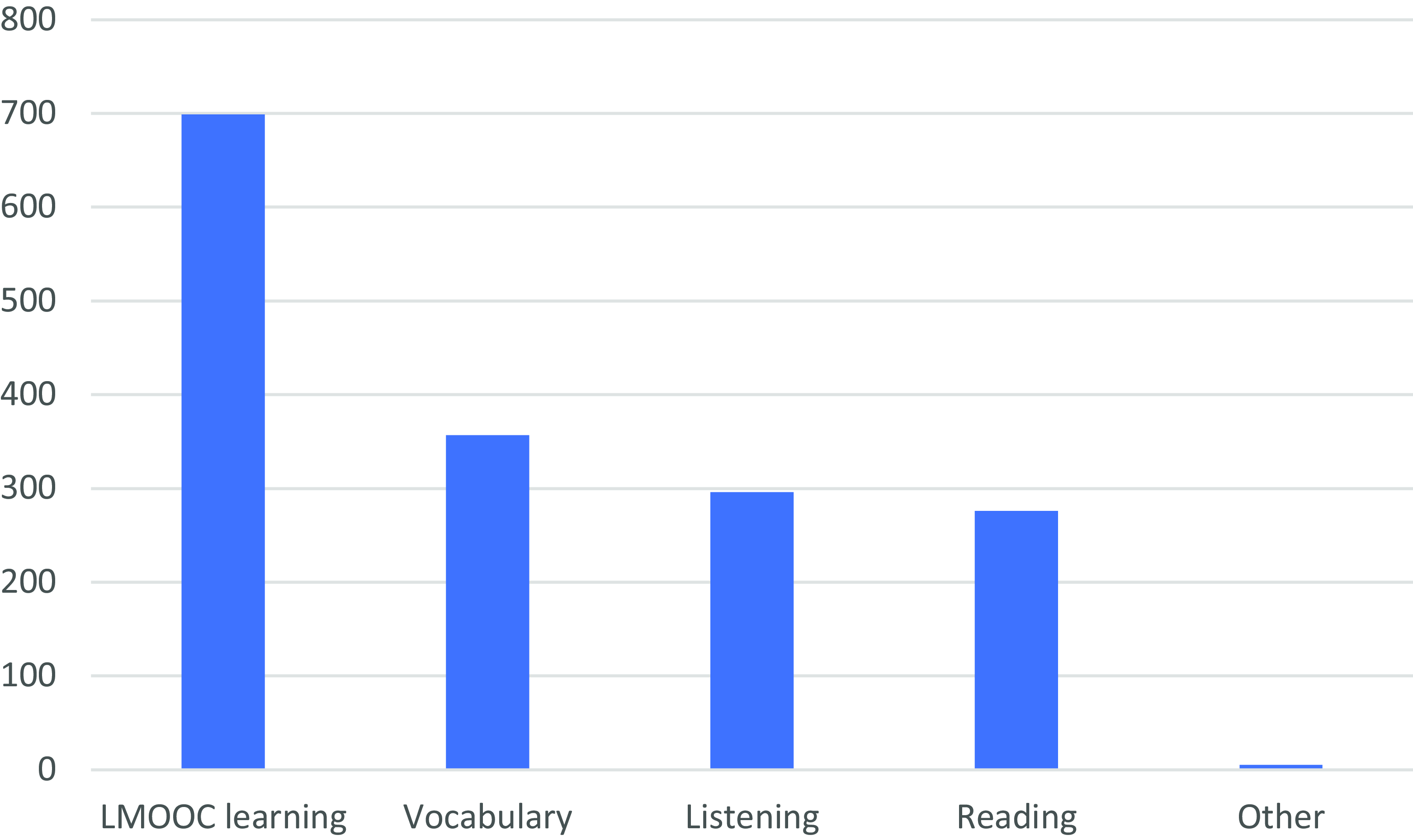
Figure 1. Learners’ check-in behavior by content type.
The second most common type of check-in content was vocabulary learning (n = 357, 21.9%). In this category, learners reported new words that they had learnt either in or out of the LMOOC, either by providing a screenshot from their vocabulary-learning software or by typing the new words in question into the forum.
This was followed by listening (n = 296, 18.1%). In this category, we found posts highlighting the completion of listening materials or the length of time that learners had spent engaged in listening practices.
The fourth category was reading (n = 276, 16.9%), which included posts about which materials the students had read and summaries of such materials. A typical example was, “Today’s reading: Tuesday with Morrie, p158-p180”.
The fifth and final category, “other” (n = 5, 0.3%), included all material that did not fit into any of the previous four categories – for example, posts noting that a student had learned English but without further elaboration.
4.2 Differences in and prediction of completion rate
Table 1 shows frequency counts for students’ completion and check-in statuses. The completion rate was 9.3% for all 11,293 learners, 93.8% for the check-in group, and 3.7% for the non-check-in group (χ2 = 6,333, df = 1, p < .001).
Table 1. Frequency counts by completion status and check-in status

As Table 2 shows, check-in behavior was a significant predictor of course completion (p < .001). Specifically, the odds of completing the course increased by a factor of more than 43 if the learners checked in on their progress. When other independent variables were held at their means, the predicted probability of completing the course was .71 for learners from the check-in group and .05 for others. In addition, the length of viewing slides, announcements and certain units (i.e. Units 5 and 8) also increased the odds of completing the course significantly, at p < .001. On the other hand, as the length of time spent viewing Unit 6 slides increased, the odds of completing the course decreased significantly, at p < .05. The model fit of this analysis was calculated as χ2 = 5,306, df = 11 (p < .001), indicating that the model with predictors fit the data significantly better than the one with just an intercept.
Table 2. Logistic regression, learning behaviors on course completion
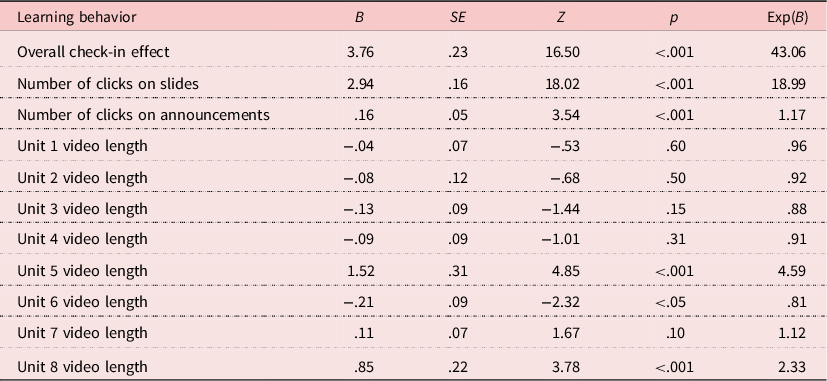
4.3 Differences in learning outcomes and behaviors among those who successfully completed the course
Table 3 presents the means of the learning outcomes of the check-in and non-check-in subgroups of those students who successfully completed the course. Of the two, the check-in subgroup’s mean scores were lower on all eight quizzes, but its mean final exam grade was higher (M = 97.69 vs. M = 94.98).
Table 3. Learning outcomes of the check-in and non-check-in subgroups of students who completed the LMOOC
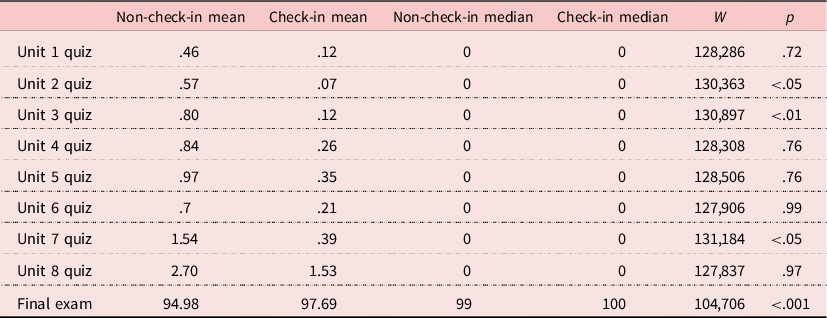
Mann–Whitney U tests were then applied to establish whether learning outcomes differed significantly between those who checked in and those who did not (Table 3). When we checked their quiz scores on a unit-by-unit basis, we found that the check-in subgroup of LMOOC completers scored significantly lower than its non-check-in counterpart on three units – that is, Unit 2 (p < .05), Unit 3 (p < .01), and Unit 7 (p < .05). In the final exam score, the check-in group outperformed the non-check-in group significantly (p < .001).
Turning now to learning behaviors, Table 4 first presents the mean incidence of the 11 captured behaviors across the same two subgroups. Not surprisingly, the means of the incidence of all learning behaviors by those LMOOC completers who checked in on their learning progress were higher than among those who did not check in.
Table 4. Learning behaviors of the check-in and non-check-in subgroups of students who completed the LMOOC
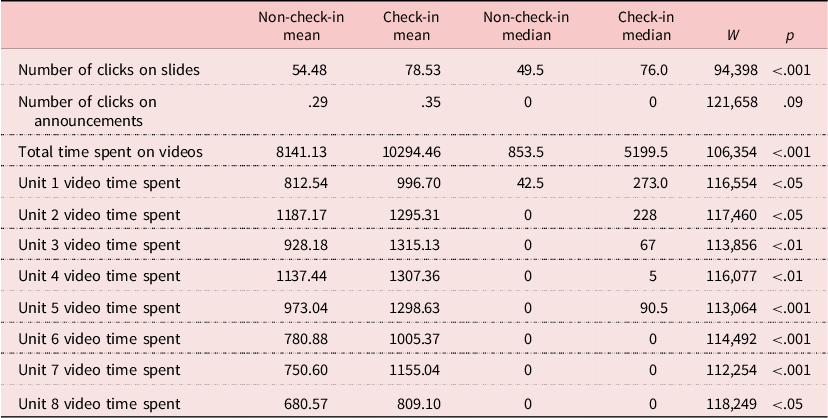
In terms of learning-behavior differences, the check-in subgroup of LMOOC completers watched videos for significantly longer (p < .001) and viewed significantly more class slides (p < .001) than the non-check-in subgroup did. However, we found no significant difference in those two subgroups’ total views of course announcements. Across all eight units, the check-in subgroup of completers watched significantly more videos than its non-check-in counterpart (Table 4).
4.4 Difference in learning outcomes and behaviors among those who engaged in the course
Of the 20.5% of students (n = 2,314) who were demonstrably engaged in the LMOOC, irrespective of their completion status, 29.4% (n = 680) exhibited check-in behavior and the remaining 70.6% (n = 1,634) did not. As compared to its non-check-in counterpart, the check-in subgroup of engaged students earned lower mean quiz scores on the first three units but higher ones starting from Unit 4 (with the exception of Unit 7). The mean final exam score for the check-in subgroup of engaged students (M = 94.59) was also markedly higher than that of the non-check-in subgroup (M = 24.60) (Table 5).
Table 5. Learning outcomes of the check-in and non-check-in subgroups of students who engaged with the LMOOC
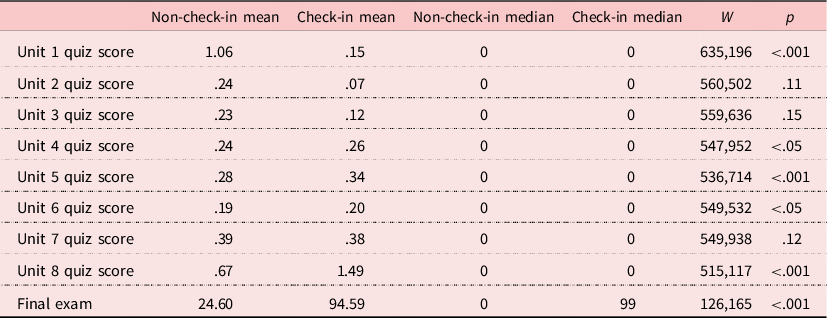
When we examined inter-subgroup quiz-score differences by unit, we found that the check-in subgroup of engaged students scored significantly lower on Unit 1 (p < .001). However, the same subgroup began to earn significantly higher scores than its counterpart starting from Unit 4 – that is, Unit 4 (p < .05), Unit 5 (p < .001), Unit 6 (p < .05), and Unit 8 (p < .001). In terms of final exam score, the check-in group outperformed the non-check-in group significantly (p < .001) (Table 5).
We then checked the means of the same two subgroups’ learning behaviors. Those for all learning behaviors exhibited by the check-in subgroup were higher than the parallel means for the non-check-in subgroup. Mann–Whitney U tests confirmed that the check-in subgroup accessed more class slides (p < .001) and class announcements (p < .001), and watched more video footage, both in total (p < .001) and for all eight units (p < .001) (Table 6).
Table 6. Learning behaviors of the check-in and non-check-in subgroups of students who engaged with the LMOOC
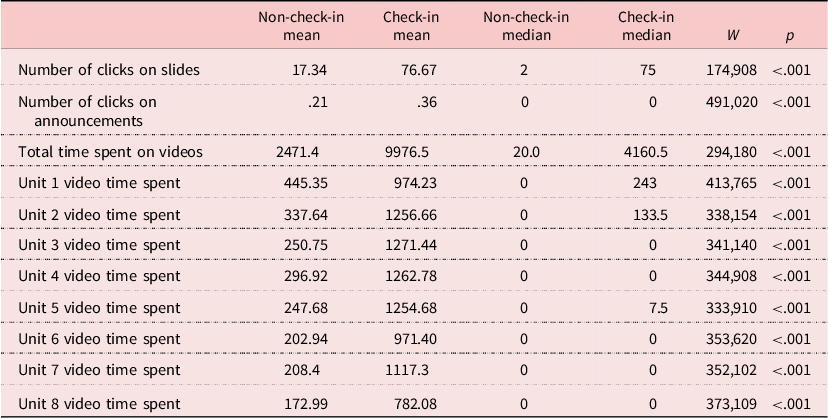
5. Discussion
This study aimed to develop a better understanding of learning check-in behavior. Its results suggest that the use of check-ins greatly benefited LMOOC learning, probably by enabling learners to practice autonomous self-directed learning behavior (Agonács & Matos, Reference Agonács and Matos2019; Agonács, Matos, Bartalesi-Graf & O’Steen, Reference Agonács, Matos, Bartalesi-Graf and O’Steen2019; Agonács et al., Reference Agonács, Matos, Bartalesi-Graf and O’Steen2020; Appel & Pujolà, Reference Appel and Pujolà2021; Ding & Shen, Reference Ding and Shen2022; Fuchs, Reference Fuchs2020; Read & Bárcena, Reference Read and Bárcena2021; Wang et al., Reference Wang, An and Wright2018).
Our first key finding, regarding overall patterns of check-in behavior, was that just 6.2% of the 11,293 sampled LMOOC learners chose to check in even once. The content of their check-in behavior was not prescribed by the instructor, and this may have driven the remarkable diversity of such content, with around 43% of it relating to learning progress on course topics, and the rest to students’ out-of-class learning progress in reading, vocabulary and listening. It seems likely that learners’ check-in behavior both reflects and facilitates autonomous online language learning, as it allows learners to control their own learning and decide how to engage with a MOOC at their own wills (Agonács & Matos, Reference Agonács and Matos2019; Agonács et al., Reference Agonács, Matos, Bartalesi-Graf and O’Steen2019; Chacón-Beltrán, Reference Chacón-Beltrán2018; Ding & Shen, Reference Ding and Shen2022; Fuchs, Reference Fuchs2020; Mac Lochlainn et al., Reference Mac Lochlainn, Nic Giolla Mhichíl and Beirne2021; Xu et al., Reference Xu, Zhu and Chan2023). Based on previous studies of the nature of autonomous learning in LMOOCs, such learning is “non-linear [… and] informal, being unconstricted by the formal educational context” (Agonács et al., Reference Agonács, Matos, Bartalesi-Graf and O’Steen2020: 1164). The check-in behavior we observed indeed fit this description, as evidenced by both its spontaneity and the great variety of its content, with some learners choosing to report the progress of their current LMOOC learning in terms of the number of units, videos, quizzes, etc., that they had completed up to that point, and others casting their eyes beyond the course’s content to report on their learning progress in off-class reading, vocabulary and listening, often using authentic novels, movies, and newspaper articles. It is also important to bear in mind that learners were free to adjust their check-in behavior at any time during the course. Perhaps more importantly, however, the check-in behavior observed in this study confirms that LMOOC students have the potential to grow beyond reactive autonomy, in which they regulate the direction of their learning after such a direction is set by others, and achieve proactive autonomy – that is, regulate the direction of learning for themselves (Ding & Shen, Reference Ding and Shen2022). None of the check-in behaviors were set, required, regulated or even monitored by the instructor; rather, while checking in, the sampled students took full responsibility for regulating not only the direction but also the whole process of their learning.
The relationship between check-in behavior and course completion was found to be very close indeed: the predicted probability of completing the course was 0.71 for learners for the check-in group, as compared to just .05 for the non-check-in group. Previous research has (1) shown that a better understanding of learner engagement can help educators minimize dropout rates (Duru et al., Reference Duru, Sunar, White, Diri and Dogan2019); (2) identified lack of motivation as one of the chief obstacles to learners’ completion of LMOOCs (Friðriksdóttir, Reference Friðriksdóttir2021); and (3) confirmed that engagement – with course materials, tasks, and assessments – significantly predicts LMOOC completion (Duru et al., Reference Duru, Sunar, White, Diri and Dogan2019; Martín-Monje et al., Reference Martín-Monje, Castrillo and Mañana-Rodríguez2018). Resonating with such findings, our study not only confirmed the importance of engaging with in-LMOOC learning activities, such as viewing slides, announcements, and videos, but also highlighted the unique role of learning check-ins in LMOOC completion. Although no previous research seems to have tested the effects of check-in behavior per se on LMOOC completion, our findings are consistent with those of Jitpaisarnwattana et al. (Reference Jitpaisarnwattana, Reinders and Darasawang2021), who reported that learners who had ILPs were more likely to complete than those who did not. The present study also extends Jitpaisarnwattana et al.’s findings in that (1) it did not limit language skills to presentation skill; rather, learners were given free rein to decide what language skill(s) they wished to improve; and (2) the LMOOC in Jitpaisarnwattana et al.’s study was cMOOC, which emphasized community-building and knowledge-sharing. Although our sampled LMOOC itself looked more like an xMOOC than a cMOOC – that is, followed a traditional mode of teaching that focused on the transmission of knowledge (Fidalgo-Blanco et al., Reference Fidalgo-Blanco, Sein-Echaluce and García-Peñalvo2016) – the addition of the check-in activity was arguably a community aspect. Thus, pedagogically, the inclusion of check-in elements can be treated as a new design feature that encourages engagement and participation, and therefore could be seen as bridging the gap between cMOOCs and xMOOCs, at least to some extent (Godwin-Jones, Reference Godwin-Jones2014).
Turning now to the relationship between check-in behavior and learning outcomes other than LMOOC completion, it was interesting to see that the check-in group earned lower quiz scores than its non-check-in counterpart, especially on quizzes administered in earlier stages of the course. Such results raise the important possibility that those who chose to check in on their progress had relatively low English proficiency at the beginning of the course. As Read, Sedano and Bárcena (Reference Read, Sedano and Bárcena2018) pointed out, LMOOCs are promising for at-risk learners, but it is nevertheless important to set a combined mind and action plan to facilitate engagement and learning by this group. Through careful course design, diligent work and active engagement with various course activities, vulnerable language learners can improve their language competency (Read & Bárcena, Reference Read and Bárcena2021). A similar positive result was observed in our study, with the check-in group earning significantly higher final exam scores than other students who took the exam. In our specific study context, we suspect that this was because their relatively low initial proficiency in English prompted some learners to make greater efforts to seek and utilize various learning resources, devote more time to learning, and check in on their learning progress more spontaneously and more actively than their initially more-proficient peers. And crucially, these efforts – including ones outside the assigned material – paid off in terms of their final exam scores.
Admittedly, the significantly lower final exam grades of the non-check-in group could have been because doing well in the exam was not their primary reason for taking the course. After all, some LMOOC users have been found to treat such courses differently from regular ones: in effect, as resource banks (Hsu, Reference Hsu2023; Mac Lochlainn et al., Reference Mac Lochlainn, Nic Giolla Mhichíl and Beirne2021). We do not deny this possibility, especially in the case of those students who engaged with the focal LMOOC at least minimally. However, among those who successfully completed it, there were still significant differences in final exam grades between those who checked in and those who did not. This was perhaps because those students who completed the course regarded their final exam with more or less equal seriousness, irrespective of whether they checked in; and, if that was the case, then the observed link between check-in behavior and academic improvement is still meaningful.
Regarding the relationship between the check-in behavior and other course-related learning behaviors, those who checked in generally engaged significantly more with in-LMOOC learning components than those who did not. This probably reflects the open nature of the LMOOC environment, in which language knowledge is learned not in isolation but in a connected and non-linear way (Agonács et al., Reference Agonács, Matos, Bartalesi-Graf and O’Steen2020). In a broader sense, the act of checking in belongs to what Ushioda (Reference Ushioda2009) conceptualized as a wider system of language-learning activities and experiences, in which language acquisition occurs not only while taking the LMOOC but also in an ecological, open-ended manner that renders the boundary between the course and the rest of one’s life effectively meaningless (see also Chacón-Beltrán, Reference Chacón-Beltrán2018; Mac Lochlainn et al., Reference Mac Lochlainn, Nic Giolla Mhichíl and Beirne2021). When LMOOC learners maximize their opportunities to use a variety of learning resources, especially external ones (Agonács et al., Reference Agonács, Matos, Bartalesi-Graf and O’Steen2019), they become more dedicated, motivated, and successful language learners (Chacón-Beltrán, Reference Chacón-Beltrán2018; Ding & Shen, Reference Ding and Shen2022; Mac Lochlainn et al., Reference Mac Lochlainn, Nic Giolla Mhichíl and Beirne2021; Xu et al., Reference Xu, Zhu and Chan2023). However, one should not disregard the slight but potentially important differences we observed in specific learning behaviors. For example, among those who completed the sampled LMOOC, we found significant inter-group differences in total time spent checking course slides and videos, but not in the checking of class announcements. This seems to indicate that these particular behavioral differences are content-related rather than logistics-related.
Based on this discussion so far, it seems reasonable to regard check-in behavior as closely related to LMOOC course completion. Noting that our third and fourth research questions differ in terms of the population data used to answer them (i.e. those who successfully completed the course vs. those who merely engaged with it), it is worth asking whether check-in behavior is actually necessary among people for whom course completion is not a primary goal. In other words, in light of prior findings about various learner profiles, especially those who only treat LMOOCs as resource banks or learning networks (Hsu, Reference Hsu2023; Mac Lochlainn et al., Reference Mac Lochlainn, Nic Giolla Mhichíl and Beirne2021), are check-ins really helpful for all learners?
Our answer to this question is yes, for the reason that, unlike in some MOOCs in other disciplines, the learning materials in LMOOCs are not merely “a way of lecturing” (Ding & Shen, Reference Ding and Shen2022: 3). Rather, they are sources of authentic linguistic and cultural input with which learners are expected to engage actively (Sokolik, Reference Sokolik, Martín-Monje and Bárcena2014). And ungraded learning check-in behavior, despite seeming superficially to be an optional add-on or even wholly irrelevant to courses’ positive impact on their students, should in fact be treated as an integral part of LMOOC learning from the moment a learner starts to exhibit this behavior.
Some limitations of this study should be noted. First, because of the design of the learning platform, we were unable to collect learners’ demographic information, such as their genders, ages, and educational backgrounds. This problem was also raised by Martín-Monje et al. (Reference Martín-Monje, Castrillo and Mañana-Rodríguez2018), who noted that there was a disconnection between different data-storage venues in LMOOC studies. This further points to a need for seamless linkages between MOOC platforms and learning-analytics infrastructure. Second, the current study did not include students’ self-reported data on their learning progress or their own perceptions of their check-in behaviors, due to the difficulty of collecting such data. Third, this study included data drawn directly from the learning platform to indicate the time students spent watching videos. Although this could have accurately reflected the actual time spent watching, it would be impossible to prove or disprove this, except insofar as the platform-recorded time spent is an upper bound for such time; and the students’ levels of attention to the videos they played remain unknown. Lastly, our study was undertaken within a single intermediate-level English-language LMOOC. Thus, it is particularly important to try to replicate our findings about check-in behavior, and in particular, to test whether the effects we observed also exist in LMOOCs (1) with other target languages, especially less-spoken ones; (2) at other levels; (3) with different enrollment numbers; (4) delivered from different countries; and/or (5) used only as an add-on component of regular classroom language learning.
Given the importance of providing LMOOC learners with innovative assignments and instruction (Hsu, Reference Hsu2023; Zhang & Sun, Reference Zhang and Sun2023), future LMOOC educators should consider encouraging check-in behavior explicitly. Such encouragement should also take account of learners’ unique strengths and weaknesses in language-specific knowledge, as well as their respective learning contexts (Read & Bárcena, Reference Read and Bárcena2021; Zhang & Zhang, Reference Zhang and Zhang2017). Teachers should give special encouragement to participate in this activity to those learners with limited language proficiency. In addition, because promoting autonomy is crucial to the design of LMOOC platforms (Ding & Shen, Reference Ding and Shen2022; Zhang & Sun, Reference Zhang and Sun2023), future designers of such platforms should consider embedding check-in functions in their system architecture.
Conclusion
The current study used learning analytics to investigate a relatively new learning behavior in LMOOCs – learning check-ins – and studied the differences between the in-platform behavior of those who chose to engage in it and those who did not. Its findings show that check-ins varied with context and with which language skills learners were seeking to acquire. We also found that a positive role appeared to be played by check-in behavior. First, it was a significant predictor of LMOOC-completion status. Second, those who checked in earned relatively low scores on assessments near the start of their LMOOC learning journeys, but significantly higher final exam scores at the end of the six-month course. And third, students who chose to check in also engaged in 11 other measured learning behaviors significantly more often than those who did not. These findings add considerably to our knowledge of the complex learning elements in LMOOCs (Agonács et al., Reference Agonács, Matos, Bartalesi-Graf and O’Steen2020) and enable us to infer the types of activities that are effective in LMOOC learning (Mac Lochlainn et al., Reference Mac Lochlainn, Nic Giolla Mhichíl and Beirne2021; Zhang & Sun, Reference Zhang and Sun2023). Therefore, we confidently anticipate that support for and encouragement of student check-ins will be embedded in LMOOCs by designers and educators in the near future.
Acknowledgements
This study is funded by the Ministry of Education in China Humanities and Social Science Project, Grant/Award Number: 20YJC880126 and the Graduate Education Teaching Reform Project of Tsinghua University, 202303J032.
Ethical statement and competing interests
This study did not involve human subjects, human material, human tissues, or human data. The authors declare no competing interests and no use of generative AI.
About the authors
Yining Zhang is an associate professor in the Department of Foreign Languages and Literatures at Tsinghua University. Her research interests include online language teaching and learning, individual differences in language learning, and learning analytics in language teaching and learning.
Fang Yang is an associate professor in the Language Centre at Tsinghua University. Her research interests include applied linguistics and online language teaching and learning.
Haolin Yang is an undergraduate student in the Department of Foreign Languages and Literatures at Tsinghua University. His research interests are network analysis and graph neural networks.
Shuyuan Han is an undergraduate student in the Department of Foreign Languages and Literatures at Tsinghua University. Her research interest lies in sustainable development.