1. Introduction
Automating spoken conversation practice in computer-assisted language learning (CALL) of a second language (L2) is a challenging task, both pedagogically and technologically. The primary pedagogical challenge is to create a practice that is both stimulating – motivating learners to interact with a chatbot, a computer-animated character or a robot – and realistic – allowing the practice outcome to be transferred to real-world situations. The main technological challenge is to autonomously interpret conversational non-native learner utterances, since these may deviate semantically and phonetically from the standard. Previous CALL systems for conversation practice have often restricted the conversations to specific role-playing scenarios with computer-animated agents in virtual environments, in which the learner input is either provided by the system for the learner to read or is more easily predicted by a narrow task-based context. This allows the system to correctly interpret the learners’ input and possibly provide corrective feedback using comparisons to the expected input. Examples for adult L2 learners include the 3D virtual reality environment and task scenarios such as “at the restaurant” created by Morton and Jack (Reference Morton and Jack2005) for beginner learners of French, Italian, Japanese and English, and the role-play dialogues with a virtual shopkeeper at a flea market that Wik and Hjalmarsson (Reference Wik and Hjalmarsson2009) used to investigate conversation practice of Swedish. Johnson and Valente (Reference Johnson and Valente2008) and Hautopp and Hanghøj (Reference Hautopp and Hanghøj2014) created similar 3D computer-animated scenarios in which the learners, respectively US army recruits and immigrants to Denmark, could interact in freer spoken conversation with a virtual version of their target community to practise the L2 (Arabic or Danish) and cultural norms (in Iraq and Denmark, respectively). Such conversation practice is intended to be transferable to real-life situations. However, the screen-based virtual environments lack realism regarding the physical rapport of situated, often multiparty interaction that is common in real-life conversations. Educational robots may therefore improve conversation practice by strengthening social aspects, regarding, for example, turn-taking, non-verbal signals, and mutual peer learner support in multi-learner settings.
The extensive reviews by van den Berghe, Verhagen, Oudgenoeg-Paz, van der Ven and Leseman (Reference van den Berghe, Verhagen, Oudgenoeg-Paz, van der Ven and Leseman2019) and Randall (Reference Randall2019) of, respectively, 33 and 79 studies on using social robots to practise vocabulary, grammar, reading, speaking and sign language show that robots may contribute to effective learning by, for example, increasing learner motivation, time on practice or self-confidence, or by introducing alternative methods for learning. However, as pointed out by Neri, Cucchiarini and Strik (Reference Neri, Cucchiarini and Strik2002), there is a risk and tendency that CALL development is technology driven rather than pedagogically founded; that is, implementation is based on what is technologically possible instead of what is most beneficial for learning. This study therefore uses data triangulation of several different learning-focused data sources to answer the research question: What recommendations for robot-led social L2 conversation practice for adult learners at intermediate level do learners, teachers and researchers give based on experience and a user test?
To do so, a literature review of previous work first identifies important aspects to consider for robot-led L2 conversations (Section 2). These aspects guide a questionnaire and interviews with L2 teachers and learners (Section 3) to determine pedagogical requirements and expectations on robot-led conversation practice similar to that at language cafés. These are informal gatherings in which L2 speakers meet first language (L1) speakers to practise listening skills and fluency through social small talk (e.g. about home countries, languages, society, and hobbies). The identified requirements are in turn considered in the implementation of a framework for robot-led L2 conversations (Section 4). A user experiment is conducted (Section 5), after which the interaction is evaluated by interviews with learners (Section 6.1), ratings of the robot’s behaviour by teachers (Section 6.2) and interaction analysis of the video-recorded conversations to identify current shortcomings in the robot’s interaction (Section 6.3). Evaluation by both students and teachers is used because it has been shown that students are not always aware of what is most beneficial for their learning (e.g. Kirschner & van Merriënboer, Reference Kirschner and van Merriënboer2013). Together, these evaluations provide a more complete pedagogical perspective of strengths, weaknesses and required future work. Many aspects are relevant for CALL-based conversation practice in general (Section 7), regardless of whether the intelligent tutor is a spoken chatbot, an animated conversational character or a robot.
2. Literature review: Previous work on L2 conversation practice with robots
Almost all previous studies of conversation practice with robots are with children, and our review therefore includes studies with children even though the present work is with adults. A summary of differences related to age for how different robots, robot roles and teaching strategies may be combined is presented in Engwall and Lopes (Reference Engwall and Lopes2020). The review concluded that adult learners require real-life practice to increase their intrinsic motivation. This can be achieved with teaching strategies such as communicative language teaching, collaborative language learning, or task-based language teaching.
To our knowledge, the only other previous robot-led conversation practice study with adults is Khalifa, Kato and Yamamoto (Reference Khalifa, Kato and Yamamoto2017), who used a three-party setting with one Nao robot posing similar questions to another robot and to a human student to find out to what extent the student learned new English expressions from the robot. Engwall, Lopes and Åhlund (Reference Engwall, Lopes and Åhlund2021) presented the background and implementation of robot-led L2 conversation practice for adult L2 learners of Swedish, inspired by language café interactions. From that study, four different areas to investigate further are identified. First, that language café interactions provide a social context that allows for realistic practice. Second, that the conversational interaction of language cafés is quite specific and mixes different types of interaction. An important reason for this is to address different aspects of language learning, such as adapting to the differences in linguistic level of heterogeneous learner groups and distributing feedback in a constructive manner during conversation practice. User tests with robot-led conversation practice showed that aspects that are specific for robot moderation – including text-to-speech synthesis (TTS), automatic speech recognition (ASR) and visual appearance – influenced the learners’ interaction with the robot and their perception of how personal and human-like it was.
These four dimensions will be used throughout this article and previous findings in relation to each are presented below.
2.1 Language learning
Motivation is one of the most important aspects for learning, and Shin and Shin (Reference Shin and Shin2015) showed that participation and satisfaction were significantly higher for a Korean middle-school class working with a robot than for another class using traditional CALL. Learner engagement may, however, be lost if the practice is too hard or too easy, as shown by Baxter, Ashurst, Read, Kennedy and Belpaeme (Reference Baxter, Ashurst, Read, Kennedy and Belpaeme2017) using robot tutors practising math and history material with preschool children. Van den Berghe et al. (Reference van den Berghe, Verhagen, Oudgenoeg-Paz, van der Ven and Leseman2019) and Randall (Reference Randall2019) further found that results in previous studies disagree on how robots’ social behaviour affects language learning, as some find positive results and others negative. One reason for this may be a mismatch between the robot’s social capabilities and the learners’ expectations, which may cause social interaction to interfere with learning. Randall (Reference Randall2019) therefore argued that the robot role should be that of peer, not teacher, because limitations in analysis and feedback capabilities make it difficult for the robot to meet expectations on how teachers should interact with learners.
2.2 Social context
Belpaeme et al. (Reference Belpaeme, Vogt, van den Berghe, Bergmann, Göksun, de Haas and Pandey2018) highlighted the importance of familiar and engaging overall themes in their analysis of requirements for using robots in L2 tutoring of preschool children. The social context further includes the relation to peers: Wang, Young and Jang (Reference Wang, Young and Jang2013), who used robot puppets to practise greetings and self-presentations in English conversations and singing with Taiwanese fifth graders, found that collaborative interaction between peers with the robot (co-discovery) was more effective for learning than sequential individual interaction, in which each learner was instructed by the preceding (peer tutoring). The post-test showed that the co-discovery group improved more than the peer tutoring group. In addition, both robot groups had a more positive attitude, less anxiety, and higher motivation and confidence in learning English than a group learning without a robot. Westlund, Martinez, Archie, Das and Breazeal (Reference Westlund, Martinez, Archie, Das and Breazeal2016) similarly observed that learners were less anxious about interacting with the robot if they had familiarised themselves with it together with peers.
2.3 Conversational interaction
Engwall et al. (Reference Engwall, Lopes and Åhlund2021) found that the 105 language café moderators who answered a questionnaire responded that they mainly interview one or several participants about a topic, answer participants’ questions, narrate (e.g. about the country), facilitate by initiating interaction between participants, or assist in their conversation. The actual strategy use was then analysed in 14 short human-led conversations with one moderator and two L2 learners. Based on the observations, four robot interaction strategies (interviewer, narrator, facilitator and interlocutor) were implemented for a user test aimed at investigating the learners’ linguistic level, gender, cultural origin and familiarity with the peer influence preferences for how the robot should structure the conversation. In the present study, a new user test is conducted to gain additional insights on pedagogical and technological challenges.
2.4 Robot moderation
Studies by Lee et al. (Reference Lee, Noh, Lee, Lee, Lee, Sagong and Kim2011), In and Han (Reference In and Han2015) and Hong, Huang, Hsu and Shen (Reference Hong, Huang, Hsu and Shen2016) have shown that TTS may pose problems for robot-assisted language learning. Lee et al. (Reference Lee, Noh, Lee, Lee, Lee, Sagong and Kim2011) found that TTS was not of sufficient quality for practising listening skills in role-play scenarios between Korean middle-school students and a robot. In the post-tests, learners had improved their speaking skills, but not their oral comprehension. Hong et al. (Reference Hong, Huang, Hsu and Shen2016), on the contrary, found that fifth-grade Taiwanese students interacting in various teaching activities with a humanoid robot had improved their L2 listening skills more than the control group, but not their L2 speaking skills. In and Han (Reference In and Han2015) let a robot teach declarative versus interrogative intonation to 10-year-old Korean children but concluded that the paralinguistic features of the TTS were not distinct enough to teach prosody.
Starting from these insights from previous work, an exploratory pre-study was conducted.
3. Pre-study: Requirements for robot-led L2 conversation practice
The pre-study consisted of two parts: one questionnaire, including free-text questions sent out to a larger group of L2 Swedish teachers, and two sets of semi-structured interviews with teachers, researchers, and learners. This combination was selected to gather both broad (questionnaire) and deeper (interview) perspectives. The questionnaire, answered by 27 L2 Swedish teachers and language café moderators, focused on the use of robots in conversation practice. The first set of interviews was with two L2 teachers organising language cafés and two researchers, respectively specialised in language and social interaction, specifically how language café moderators and participants co-create the learning environment (Ali Reza Majlesi, Stockholm University), and Swedish as an L2, specifically in pedagogy for spoken practice (Elisabeth Zetterholm, Stockholm University). The relevant questions are summarised in the left part of Table 1. The second set was with 13 language café participants (seven female, six male, median age 25 years, representing a variety of L1-L2 pairs [Mauritian, Somali, Kyrgyz]–Swedish, [Spanish, English]–German, [Norwegian, French]–French, Swedish–Chinese, with both beginner- to intermediate-level learners and native speaker hosts). It focused on the questions shown in the right part of Table 1. The answers are listed below, with indications if they were mentioned by teachers (T), researchers (R) or language café participants (L) and the number of times. The data were analysed inductively, with all answers being summarised and categorised, before being grouped theme-wise post-analysis.
Table 1. Thematically reordered important questions for the pre-study interviews
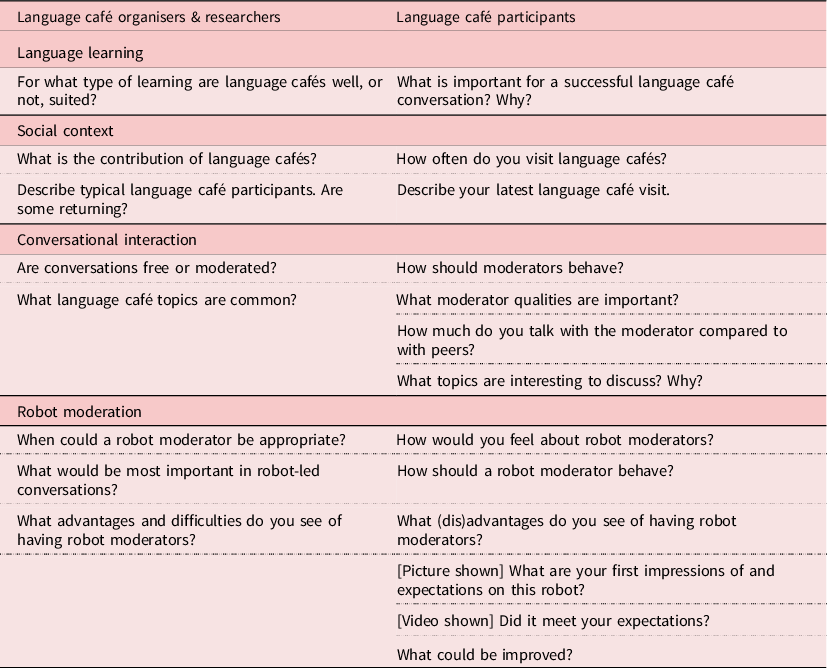
3.1 Language learning
Finding an appropriate level is a challenge in language café settings since the learners’ levels differ (1L), making it difficult to create practice adequate for all (1L). This is often mitigated by encouraging more proficient peers to assist lower-level learners (8L). Corrective feedback is expected (1R, 5L), at least when it benefits the whole group (1L) or if the moderator uses recasts to reformulate erroneous learner utterances rather than explicit corrections (1L). However, some learners did not want to give or receive feedback in conversation settings (2L), and it was estimated that human moderators correct only about 20% of the errors (1R+1T). Regarding using robots as moderators, opinions were divided between those who saw no advantages (6T+2L) and those who were positive (22T+2R+4L), because robots could be motivating to work with (3T+4L), they could increase training time and availability (10T+3L), they would be objective when correcting learner errors (3T+3L) or would be less intimidating to practise with (3T+2L). Robots should adapt to the learners’ linguistic level (2T+1L) but still challenge them (3T+2L) and assist them with linguistic problems (1L). Their spoken utterances should be linguistically correct (1L) and clearly produced as a pronunciation target (6T+2L), and be easily understandable. They should make sure that everyone understands or else reformulates utterances (4T+1L).
3.2 Social context
The focus should be first on personal and familiar topics (11L), to promote the social atmosphere and to allow learners to concentrate on utterance formulation (1L), and second on societal content, since learning about the society the learners live in is as important as language practice (1T+1R+7L). Commonly occurring and appreciated topics include culture and cultural differences (7L), everyday life (6L), self-presentations and own experiences (6L), the news (3L) and languages (2L). It was further stressed that diversity in topics is a key feature (2L). Social interaction with peers is the most important aspect of language cafés (2T+3L), and the lack of social interaction was deemed to be the largest disadvantage of using robot moderators (14T+8L). Robots do not allow learners to meet native speakers who assist them with matters outside the language café, such as paperwork and homework (1R+1L), or who engage in social activities (e.g. baking together) (2L). Since respondents stated that robots could never replace human moderators (2T+2L) or that it had to be better than a human moderator (1L), it is important to clearly present robot-led conversations as a complement (e.g. in case of low availability of L1 speakers or to gain confidence and L2 proficiency). Moreover, whereas some learners stated that they would prefer to use the robot individually as a personal coach (2L), more respondents stressed that human–human interaction is required to establish a social atmosphere (12T+9L). This suggests that a multiparty setting, with one robot and several learners, should be used and that learners should be encouraged to also interact with each other. The multiparty setting could further facilitate familiarisation with the robot, as some learners stated that they would need getting used to the robot before engaging with it in conversation practice (3L), while some would be comfortable directly (2L).
Other mentioned social disadvantages of using robots were that they would not have cognition and feelings (3T+4L) and that they would be impersonal (4T+2L) and lack cultural knowledge (1L). Because cognition may be hard to achieve in robots, it is important that they should establish a personal relationship by remembering participants (1L), their names and previous input (2L) and by providing their own stories and opinions.
3.3 Conversational interaction
Language café interactions are different from the L2 classroom (2T+2R+2L) since it depends on the learners’ level (2T+2L), if they are first-time attendees or returning (2L), and on the moderator (1L). In general, standard, basic questions are used the first time, but at subsequent meetings, interaction develops into freer and deeper discussions (2L) in which learners get the opportunity to talk as much as possible (2L). This development was stressed as important, since questions-and-answers practice becomes too constrained (2T+3L).
The L1 speakers’ role at language cafés varies substantially: some have no moderator responsibilities (4L), others are more active in initiating discussions when needed (9L), and still others play a formal role in leading and supporting conversations (2L). A good moderator should be helpful and provide linguistic support (4L), be patient (3T+1R+3L), present topics and ask questions around them (1T+3L) but know when to leave the initiative to learners (4L) and distribute turns to involve less active participants (1L).
3.4 Robot moderation
Robot moderators should be human-like (4T+1L), but after having been shown a video of robot–human interaction, learners were divided regarding whether the synthetic speech was adequate (2L) or monotonous (3L) and whether the facial expressions were good (2L) or unnerving (4L) concerning the appearance of the eyes (2L) and head movements (1L). Fears were that the robot would not understand learners (2T+4L) because of a lack of contextual knowledge (1L) or learners’ non-standard pronunciation (2T+1R).
When summarising the expectations for robot moderators, some appear currently beyond state of the art (constituting a clearer pronunciation target than humans, being able to correct all learner errors and provide linguistic support for learner difficulties) but others are within reach. In fact, after seeing the video, many respondents stated that the robot met (11T+1L) or exceeded (1T+6L) their expectations.
4. Implementation in robot-led conversation practice
Based on the findings of Sections 2–3, we summarise implementation choices for the present study as follows.
4.1 Language learning
To improve adaptation to the learners’ level, the robot is controlled by a semi-automated Wizard-of-Oz set-up (cf. Section 5), allowing the wizard to monitor learner problems and change topic or addressee when problems occur. The choice of using a wizard is based on the current limitations of ASR for L2 conversational speech.
All robot utterances are pre-generated, which means that the robot cannot provide linguistic support or reformulate utterances that were not understood.
Learner errors are not corrected, both for pedagogical reasons of not interrupting the conversation to promote fluency and self-confidence in the L2, and for the technological challenges of automatically generating corrective feedback in free conversations. Instead, the robot offers encouraging, positive feedback.
4.2 Social context
To ensure that the practice is familiar and transferable to human–human interaction, conversations are focused on typical everyday social topics, such as presenting oneself (background, occupation, hobbies, etc.), talking about Sweden and Swedish and comparing to the learners’ home countries and languages. To establish personal relationships, the robot provides (factual or fictional) information about itself and refers to learners, their home countries, and native languages by name.
A set-up with learner pairs is used to achieve pedagogical advantages (that the peers may support each other linguistically) as well as technological (that peer interaction may save the practice in situations if technology fails) and social (that peer interaction may improve the conversation and reduce learner anxiety of talking with a robot) benefits. The robot encourages peer interaction by asking questions that both learners need to provide input to (e.g. “Do you live close to each other?”), requesting peer comments (“Do you also like that movie?”), enticing collaboration (“Do you two know which the four largest cities in Sweden are?”) or encouraging peer assistance (“Could you help explaining?”).
4.3 Conversational interaction
The robot initiates and drives the conversation in the three-party interaction by asking questions directed to one or both learners and providing its own views. Limited interaction adjustments are made depending on the participants’ activity. Compared to Engwall et al. (Reference Engwall, Lopes and Åhlund2021), the interaction contains more individual follow-up questions to each learner and robot answers to counter-questions by learners, since individual interviewing was the most appreciated by learners and learner questions were frequent.
4.4 Robot moderation
Default TTS synthesis, with a Swedish voice from CereProc, is used for the robot’s vocal utterances, lip movements and facial expressions on the computer-animated face. This means that no manual adaptations are made of the robot’s voice (speed, intonation), facial expressions (emotional, word emphasis), or eye-gaze patterns (other than turning towards the addressed learner).
The above implementation choices were tested in the experiments described in Section 5.
5. Experimental set-up
We conducted 12 robot-led conversations of approximately 10 minutes each with 12 pairs of adult L2 learners (17 women, seven men) attending a Swedish for Immigrants course at level B2–C1. As is normally the case in such courses, the learners varied widely in L1 background (three Arabic, two Chinese, two Polish, Russian, Ukrainian, Belarussian, Azerbaijan, Armenian, Serbian, Bosnian, German, Greek, Italian, Indonesian, Tamil, Hindi, Dari, Urdu, Persian, Spanish), in age (24–60 years) and in how long they had been studying Swedish (between 1 month and 4 years). The robot Furhat (Al Moubayed, Beskow, Skantze & Granström, Reference Al Moubayed, Beskow, Skantze, Granström, Esposito, Esposito, Vinciarelli, Hoffmann and Müller2012) was placed on a table facing the learners, who were seated on a sofa (Figure 1). An informed consent form was used to describe the aim of the study and the use of collected data, but learners were not otherwise instructed regarding how to interact with the robot. To avoid influencing later learner pairs’ expectations, the Wizard-of-Oz set-up was not disclosed until all conversations had been conducted. The wizard controlled the dialogue flow using predefined utterances that were automatically updated at each robot turn, based on the previous robot utterance, so that the wizard could choose the next robot utterance from general statements (Yes/No/I do not really know), backchannels and feedback (Mm/Mhm/Interesting) and up to nine utterances with follow-up questions, topic transitions and robot answers to counter-questions. The wizard was seated in the same room, but hidden behind an office divider, so that he could hear the learners’ input and, based on this, choose the most appropriate next robot utterance using shortcut keys. To allow for post-session analysis, the conversations were recorded using a GoPro video camera capturing the scene (as shown in Figure 1) and one headset microphone per participant.
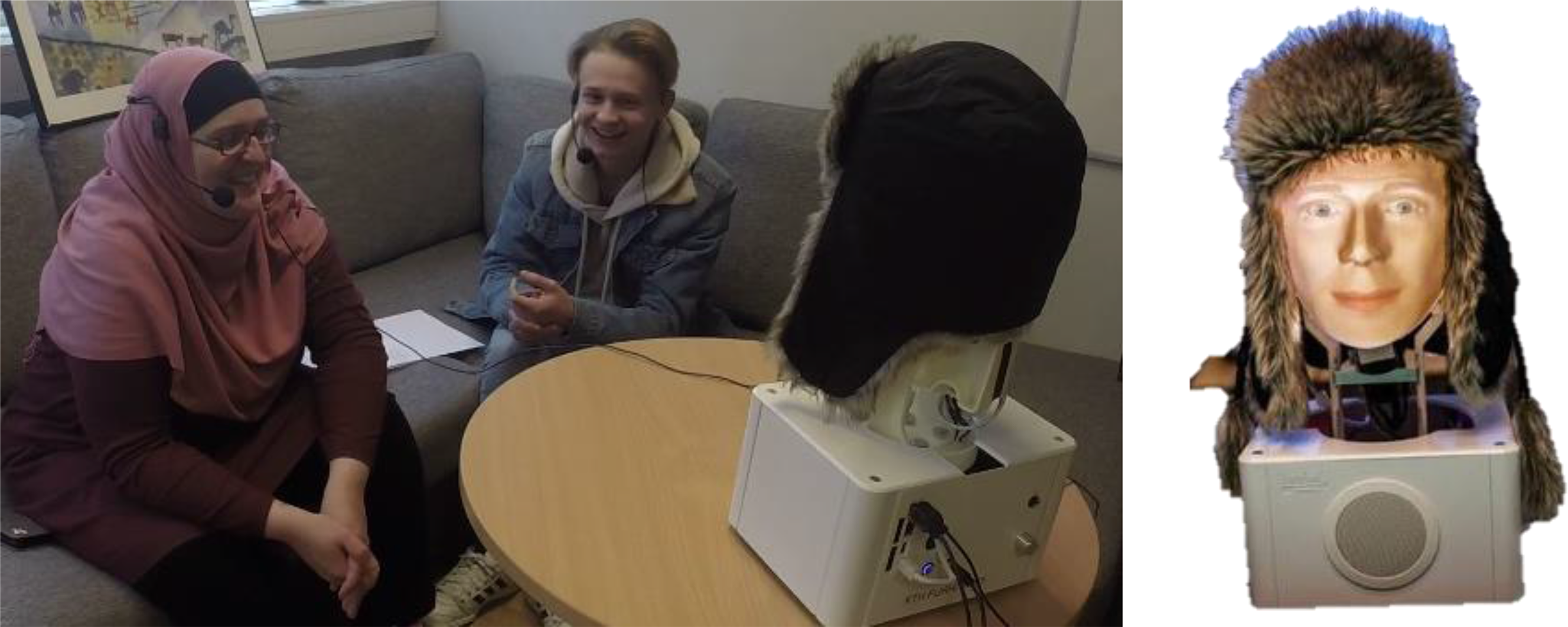
Figure 1. Left: Set-up of the robot-led conversation. Right: Furhat robot, with computer-animated face back-projected on 3D face mask
6. Evaluation
The 12 conversations were transcribed and analysed in three ways: (a) through semi-structured interviews with the 24 learners (Section 6.1), (b) via teacher assessment of the robot’s interaction (Section 6.2) and (c) by an interaction analysis of the video recordings (Section 6.3).
6.1 Post-interaction interviews with learners
After each conversation, learner pairs were interviewed for 10–20 minutes about the session in Swedish and/or English, depending on their preferences. Table 2 lists questions of the semi-structured interview reordered thematically post-analysis for this article, together with common answers. An inductive data analysis was employed; that is, all interviews were recorded and thereafter analysed as a set to identify common responses and count their frequency. Some responses emanate directly from one question (e.g. “Were there any unnatural pauses?”), whereas others were offered to more general questions, as indicated by Table 2. To quantify the learner responses, the number of learners providing positive or negative feedback on each aspect was counted. Using a binary scale for learner feedback (positive: +1, negative: −1), mean numbers were calculated per aspect (row-wise means, used below to quantify the quality of different aspects). As responses were given in free form to open questions, not all aspects were covered in each interview. Moreover, the binary scale does not indicate how strong the learners’ opinions were. The relative numbers of positive and negative opinions nevertheless indicate strong and weak aspects of the interaction.
Table 2. Post-session interview questions (reordered thematically), common responses and heatmap summarising responses per learner pair (sorted from negative to positive)
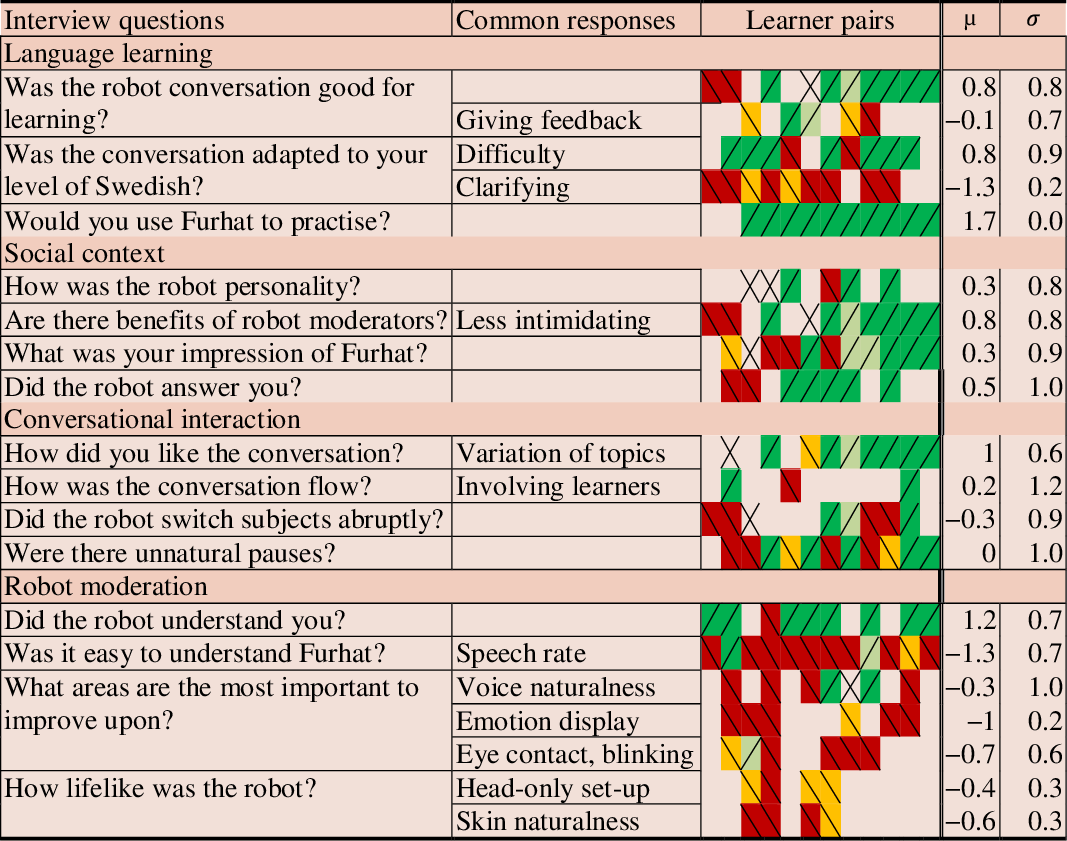
Note. Diagonal up signifies that one (light green) or both (green) learners were positive; diagonal down, that one (orange) or both (red) learners were negative; crossed diagonals, that they disagreed; and empty cells, that no feedback was provided. The last two columns show mean and standard deviation for each aspect.
6.1.1 Language learning
The most positive responses were that participants would like to practise again with Furhat (+1.7) and that a majority stated that the conversation was good for learning (+0.8). Feedback was discussed by nine participants who correctly noticed that learner errors were not corrected. Four participants found this to be positive, as they became more relaxed than speaking with a human teacher who would correct them, but five wanted feedback and felt that the robot was not paying enough attention (−0.1). The level of the robot’s utterances was adequate (+0.8) but many criticised that the robot did not clarify its utterances when the participants did not understand (−1.3).
6.1.2 Social context
The robot’s personality (+0.3), role as an L1 speaking peer (+0.8) and its ability to reply to within-topic questions (+0.5) were positively received, but it was criticised for not responding to more general questions.
6.1.3 Conversational interaction
The variation and duration of topics was adequate (+1.0), but the robot sometimes changed topic too abruptly (−0.3). Involving both learners was successful with some pairs, but not with all (+0.2). Regarding response times, negative learners either found that the robot took too long to respond, or, on the contrary, that the robot sometimes responded unnaturally quickly, without having to reflect before answering.
6.1.4 Robot moderation
The robot was perceived as understanding participants (+1.2), but the TTS speech rate was criticised for being too high (−1.3). Another problem concerned mispronunciations of participant names and unusual words, which could be confusing or detrimental for learning correct pronunciation. Opinions were divided regarding voice naturalness (−0.3): five learners found the synthetic speech good, whereas nine were negative, because it made understanding more difficult. Six learners mentioned that Furhat’s responses to participant input were repetitive (in particular, “Interesting!”). The robot’s visual behaviour suffered from lack of emotions (−1.0), eye contact (−0.7) and natural-looking skin (−0.6).
6.2 Assessment of the robot-led conversation by L2 Swedish teachers
In the next evaluation, 27 teachers of L2 Swedish and language café moderators (same respondents as for the pre-study questionnaire) rated the robot’s interaction. Rating was done in two steps with a 5-point Likert scale, using the four areas of our analytical framework and statements in the upper part of Figure 2. First, respondents graded the importance of one to four aspects within each category (1 = “unimportant”, 5 = “essential”). In the second stage, they viewed a video of one robot-led conversation and rated how well the statements described the robot’s performance (1 = “strongly agree”, 5 = “strongly disagree”). The results are summarised per area below, with ns/*/** respectively indicating if the difference between the two ratings was non-significant or significant at p < 0.05 or p < 0.01 using a non-parametric Mann–Whitney U test.
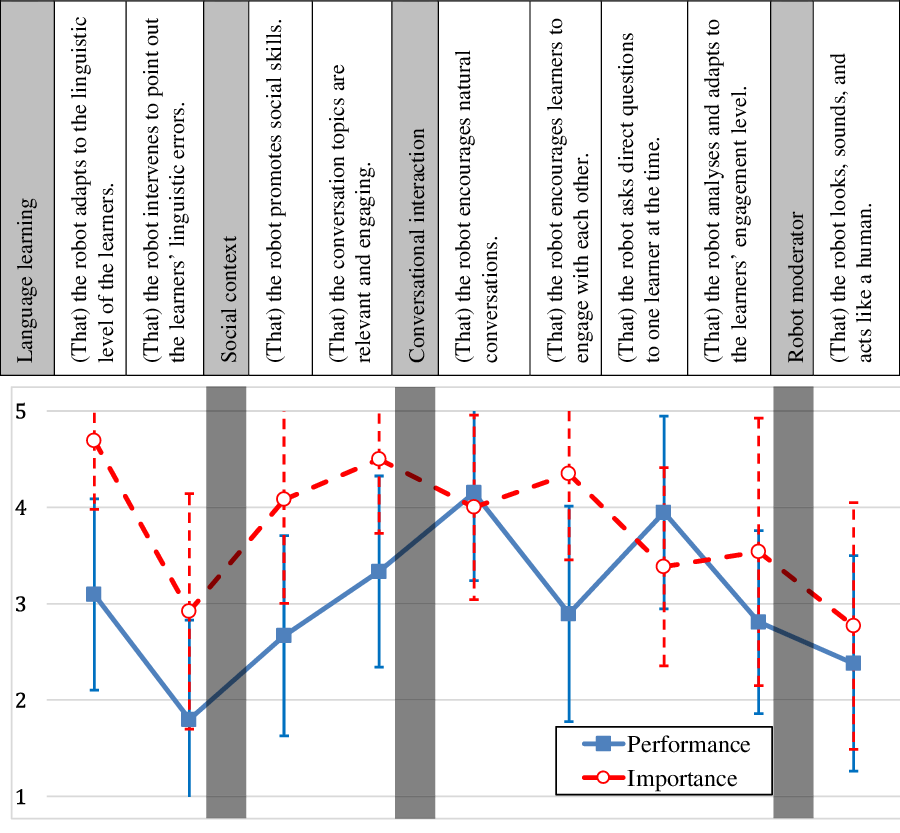
Figure 2. L2 teachers’ description of the current robot behaviour (solid blue line; excluding “That” in the statement) of different aspects and their importance (dashed red line), based on the statements above the graph. Error bars show standard deviation
6.2.1 Language learning
The robot did not adapt to the learners’ level as much as the teachers requested (performance: 3.1 vs. importance: 4.7**), but the fact that the robot did not correct linguistic errors was perceived as less problematic, since correction was viewed as less crucial (1.8 vs. 2.9**).
6.2.2 Social context
Topics were assessed as moderately relevant and engaging, which should be improved, as this is important (3.3 vs. 4.5**). The same applies to promoting social skills, where the gap is also large (2.7 vs. 4.1**).
6.2.3 Conversational interaction
The interaction was found to be natural (4.2 vs. 4.0ns), but the robot should encourage the learners to interact with each other more (2.9 vs. 4.3**) rather than interviewing one learner at a time (3.9 vs. 3.4ns) and should adapt more to the learners’ engagement (2.8 vs. 3.5*).
6.2.4 Robot moderation
The robot was not judged as sounding and looking like a human, but this was also not considered an important feature (2.4 vs. 2.7ns).
In the free-form answers in the questionnaire, 12 teachers stated that the interaction was appropriate, regarding, for example, the type of questions and the robot’s speech quality, three were more hesitant and three were negative. The criticism focused on how human-like and personal the robot was, its pronunciation and speech rate, the level of difficulty of some questions and the robot’s inability to handle comprehension problems or to adapt to unforeseen conversation.
The teachers were further remarkably divided on the utility of the current implementation in actual conversation practice, with eight stating that it was already almost acceptable, seven that it could be useful in some situations and seven that it was far from ready.
6.3 Interaction analysis of robot-L2 conversations
In the final level of analysis in this study, the 12 video-recorded sessions were examined to identify challenges, assess how they are currently handled and suggest improvements. The observations were made through inductive coding of interaction problems in the videos, illustrated in the extract in the Appendix (see supplementary material).
6.3.1 Language learning
The level of the practice overall seems appropriate for most, albeit not all learners. The robot sometimes had to repeat questions, but this appears to be linked to speech rate and pronunciation of specific words, rather than to too complex utterances, since the learners were eventually mostly able to respond after the robot had repeated the question. If repetition did not help, a different robot utterance that changes the topic was used, but a more suitable strategy would be to lower the TTS speed or rephrase the utterance.
Lack of learner responses may also be caused by difficulties formulating an answer. Linguistic help to find the correct words is common in human-led conversation practice but is challenging for a robot and the most realistic would be to encourage the peer to assist.
6.3.2 Social context
Using participant names, countries, and native languages may improve the interaction, but as shown in the Appendix, it introduces problems if the robot’s information about learner placement is incorrect. Updating the robot’s information or removing personalisation of robot utterances if a mismatch is detected would solve the problem. The use of predetermined robot utterances for each state was found to limit the robot’s ability to reply to personal questions.
6.3.3 Conversational interaction
Turn distribution is important to promote equal interaction from the learners, since one learner may be much more active than the other due to language level or personality. At present, there is no automatic tracking of the share of the conversation for each of the two learners, but it has been shown (Gillet et al., Reference Gillet, Cumbal, Pereira, Lopes, Engwall and Leite2021) that the robot may use gaze to achieve more even distribution.
The robot’s topic transitions are currently explicit (e.g. “Let’s talk about something else.”), whereas human conversations more seamlessly transition from one topic to another. A data-driven approach trained on human conversations could result in more natural topic changes.
6.3.4 Robot moderation
Lack of appropriate robot responses, or difficulties interpreting learner utterances, may be handled by involving the other learner (e.g. “What do you think about this?”). A particular case is learner input in another language, which would require the peer to assist, if the ASR is unable to recognise the intent from a partial transcription.
As generic feedback from the robot was found to be repetitive, the set of verbal and visual acknowledgement repertoire needs to be expanded from the five fixed responses currently used.
7. Discussion and conclusions
We conclude the article by discussing the study compared to the state-of-the-art, future work, its limitations, and its contributions to the CALL field.
7.1 Progress against state-of-the-art and future work
The present study extends previous work by demonstrating the possibility of using robots for more complex learning material (free social conversations, rather than practising basic vocabulary), a more advanced learner group (intermediate-level adults, rather than children) and a more demanding interaction with two learners (rather than one-to-one or robot-to-whole-group interaction). According to the evaluation of the robot-led conversations, several common expectations (such as varying topics, having an appropriate level, involving both learners and providing valuable, less intimidating practice) are at least partially met. However, others need to be addressed further for smooth conversation, as discussed below.
7.1.1 Language learning
Adaptation to learner level was highlighted in Section 6.2 as the aspect for which the difference between its importance and the robot performance was the largest, and we will attempt adapting to learner level during sessions by using different sets of conversation topics and utterance complexity for different proficiency levels. A lower-level set will be used if a need is detected through verbal (e.g. many requests for clarification and repetition) or visual cues of confusion (Cumbal, Lopes & Engwall, Reference Cumbal, Lopes and Engwall2020) and a higher one if the learners are more proficient (e.g. produce more words per minute or longer utterances).
Feedback requires more attention, both from a pedagogical and a technological perspective. Pedagogically, opinions of respondents were divided regarding whether the robot should provide corrective feedback, and if so, how much. Technologically, it is currently beyond the state of the art to robustly detect participant errors in free L2 conversations and provide adequate, explicit feedback. We will instead explore whether the same robot responses could be used for both confirmation and as a recast of incorrect formulations (e.g. “So you have been in Sweden for a long time” as a response to both “I have been in Sweden for three years” and “I be in Sweden three years”).
7.1.2 Social context
The focus on societal content, which was highlighted in Section 3 as being important for adult learners, is quite unique compared to previous work in robot-assisted language learning. The robot provides facts about Sweden and asks the participants quiz questions about the country, but a more complex discussion about culture and society would have been beyond the capacity of most participants in these conversations. Such interaction would be interesting to explore with more proficient learners, but this requires that the robot be able to automatically create new utterances using methods for question generation based on information extraction (e.g. Duan, Tang, Chen & Zhou, Reference Duan, Tang, Chen and Zhou2017) instead of manually created utterances.
The robot’s personality was mostly rated positively by the learners, which is promising, since adult learners generally have higher expectations regarding social abilities than children, who accept stereotypic, toy-like robots (Engwall & Lopes, Reference Engwall and Lopes2020). However, the robot’s personality can be enhanced further by enabling the use of all robot utterances in the database at every stage of the conversation instead of only when the learner question had been anticipated. Another improvement would be that the robot remembers and integrates participant answers into ongoing conversations, using a learner database (e.g. Verpoorten, Glahn, Kravcik, Ternier & Specht, Reference Verpoorten, Glahn, Kravcik, Ternier, Specht, Cress, Dimitrova and Specht2009).
7.1.3 Conversational interaction
The importance of the robot’s role to monitor and distribute conversation turns when one learner dominates is clearly illustrated in the Appendix. Determining the engagement level of the speaker, using textual and acoustic analysis of vocal features, and the non-active listener, using video analysis of facial expressions is valuable to decide when a topic or addressee shift is needed (Engwall, Cumbal, Lopes, Ljung & Månsson, Reference Engwall, Cumbal, Lopes, Ljung and Månsson2022).
Tests with state-of-the-art ASR (Cumbal, Moell, Lopes & Engwall, Reference Cumbal, Moell, Lopes and Engwall2021) on robot-led conversation recordings show important challenges for ASR to correctly transcribe spontaneous non-native speech, but Engwall, Lopes and Cumbal (Reference Engwall, Lopes and Cumbal2022) also demonstrate that the impact of these problems may be minimised by selecting the most probable next robot utterance, given statistics of what utterance the wizard chose in previous, similar conversations. We will explore employing follow-up utterances that do not rely on fully understanding the learner input and, in case of low ASR reliability, avoid problems by involving the peer learner to continue the conversation.
7.1.4 Robot moderation
Future work is required on TTS quality, which received many negative comments both in terms of naturalness and intelligibility. To improve naturalness, which is important in L2 practice for understanding and as learning targets (e.g. In & Han, Reference In and Han2015), we will investigate switching to a deep learning TTS, since development over the past few years has led to remarkable improvement in naturalness. To improve intelligibility, the TTS speaking rate will be lowered for more difficult utterances. To determine which these are, a data-driven approach will be used, so that an utterance that is not understood by a learner will not only be repeated at slower speed for this learner but will also have reduced speed in subsequent conversations with other learners.
Eye contact and emotion display were not implemented in the present study and were two of the aspects that received the most negative comments. Matching gaze has been demonstrated in an earlier study using Furhat with an eye-tracker (Zhang, Beskow & Kjellström, Reference Zhang, Beskow, Kjellström, Kheddar, Yoshida, Ge, Suzuki, Cabibihan, Eyssel and He2017), and because the robot’s face is computer animated, visual display of emotions and feedback can readily be added if robot utterances are manually or automatically coded for sentiment. Both eye contact and emotion display will be studied further in our future work.
7.2 Limitations
A major limitation is that the study intentionally does not evaluate the learning effectiveness of the practice, and this needs to be investigated. The studied interaction is moreover short (10 minutes), made once for a limited number (24) and one category (adults at intermediate level) of learners. The results are hence diagnostic with the aim of providing guidelines on how to improve this, and similar, L2 conversation practice. Future evaluations, after addressing aspects identified in this study, should study longer, repeated learner–robot interactions with a fully autonomous robot. Using repeated sessions is important, because learner preferences for the robot’s interaction may change over time (Engwall et al., Reference Engwall, Lopes and Åhlund2021). Interaction with an autonomous robot needs to be investigated, since it may be different compared with a Wizard-of-Oz set-up. This study presents conversation practice in Swedish, but in almost all respects, pedagogical requirements and analysis of interaction aspects would be similar regardless of language (except that English TTS and ASR may be more robust).
7.3 Contributions of the study
The study explored four important areas for development of conversational practice that to large extents are valid also for other types of conversation practice, led by a computer-animated intelligent tutor in CALL software or even a human moderator, except for aspects purely related to (robot) technology. Learners and teachers highlight the importance of using topics that are familiar and relevant, of establishing a personal relationship between the moderator and the learners, and of the moderator being active and adaptive to different learners, regarding moderating the conversation, its level of complexity and feedback given to learners. These requirements are natural for a human moderator but require additional efforts to fulfil with educational robots and intelligent tutors in CALL. This study has shown that some requirements for robot-led conversation practice are rather close to being fulfilled, such as motivating adult learners to engage in spoken practice and defining a suitable robot interaction strategy based on a social interviewing scheme inspired by language cafés. Other aspects, such as automatically adapting the linguistic complexity and the topics to the level of individual learners or providing corrective feedback and pedagogical support, will be more difficult to achieve. However, with additional development, robot-led conversation practice could serve as a complement to human-led lessons already with the current state of the art, since the learners found the practice to be valuable, engaging and less intimidating than speaking with a human moderator.
Supplementary material
To view supplementary material referred to in this article, please visit https://doi.org/10.1017/S0958344022000027
Acknowledgements
This work was supported by the Swedish Research Council under Grant 2016-03698.
Author contributions
The article was written by the first three authors, partly based on material (interviews and surveys in Sections 3 and 6.2, data collection in Section 6.1) gathered by authors 4–11 as part of their BSc theses supervised by the first author.
Ethical statement and competing interests
The authors have no conflicts of interest. All participants in the experiment signed an informed consent form.
About the authors
Olov Engwall is a professor in speech communication at KTH. He received his PhD from KTH in 2002 and has since led several research projects on computer-animated tutors, for which he received the Christian Benoît Award in 2004, and robot-assisted language learning. He has chaired several international workshops on the use of speech technology in language learning.
José David Lopes is a research associate at Heriot-Watt University. He has a PhD from Instituto Superior Técnico, Lisbon, Portugal, and has worked with spoken dialogue systems as a postdoctoral researcher at KTH.
Ronald Cumbal is PhD student at KTH under the supervision of Engwall and Lopes.
Gustav Berndtson, Ruben Lindström, Patrik Ekman, Eric Hartmanis, Emelie Jin, Ella Johnston, Gara Tahir and Michael Mekonnen are MSc students of the Industrial Engineering and Management programme, with a specialisation in Computer Science.
Author ORCIDs
Olov Engwall, https://orcid.org/0000-0003-4532-014X
José David Lopes, https://orcid.org/0000-0002-8773-9216
Ronald Cumbal, https://orcid.org/0000-0003-4472-4732