Background
Controversies related to the categorical classification of mental disorders and related morbidity have led to several initiatives aimed at reorganising diagnostic systems. Based on biobehavioural studies investigating common mechanisms of different mental disorders, the National Institute of Mental Health's Research Domain Criteria project was established to classify mental problems along dimensions of observable behaviour and neurobiological markers.Reference Insel, Cuthbert, Garvey, Heinssen, Pine and Quinn1 These findings are also reflected in the conceptualisation of the latest version of DSM-5, which includes additional dimensional classification (e.g. the internalising and externalising dimension). From this point of view, it has proven advantageous to not simply focus on individual mental disorders when examining associations with biological markers, but rather, to jointly consider several disorders from the same dimension.
Internalising disorders encompass mood and anxiety disorders, such as depression and anxiety, which show high rates of comorbidity indicating a common latent liability.Reference Gorman2 Additionally, previous studies demonstrated that the transdiagnostic internalising perspective improves prediction for future outcomes (e.g. all-cause mortality) compared with diagnosis-based considerations.Reference Kim, Turiano, Forbes, Kotov, Krueger and Eaton3 Furthermore, there is a growing body of literature showing empirical evidence of shared genetic aetiology for closely related psychiatric disorders from one dimension using analysis of common genetic variants.Reference Ward, Tunbridge, Sandor, Lyall, Ferguson and Strawbridge4 For the internalising dimension, the assumption of a shared genetic basis is supported by previous studies showing high genetic correlation between the single disorders.Reference Yilmaz, Halvorsen, Bryois, Yu, Thornton and Zerwas5
Vitamin D
Vitamin D comprises a group of lipid-soluble compounds with neurosteroid characteristics. Vitamin D can be generated by sunlight exposure and food intake. Vitamin D status is measured using the level of 25-hydroxy vitamin D (25OHD), which is a metabolite and the major circulating form of vitamin DReference Holick6 in the body. Besides playing an important role in bone metabolism, vitamin D is involved in brain processing, neuromodulation, regulation of neurotrophy, neuroplasticity and brain development.Reference de Abreu, Eyles and Feron7 In this context, vitamin D receptors can be found in several brain areas associated with psychiatric disorders (e.g. hippocampus, prefrontal areas), such as depression and anxiety.Reference Eyles, Burne and McGrath8
In past decades, 25OHD was thought to represent an important factor in the development and prevention of diseases, especially cardiovascular disease, cancer and bone fractures.Reference Wang9 In recent years, however, a turning point has been observed in this area of research, as several lines of research have cast doubt on the extent of vitamin D's impact on disease development.Reference Pilz, Verheyen, Grübler, Tomaschitz and März10 One of these lines is the Mendelian randomisation method. In observational studies, the assessment of causality between an exposure and an outcome is limited because of the presence of confounding factors, information (e.g. misclassification) or selection bias (influence of the specific study sample). With the Mendelian randomisation approach, causality between exposures and outcome can be indirectly estimated using genetic variants as instrumental variables. If the genetic variants satisfy the assumptions of instrumental variables (see Method), causal effect between exposure and outcome can be estimated unbiased from any potential unobserved confounder.Reference Burgess, Foley and Zuber11
In observational studies, vitamin D deficiency is associated with an increased risk for psychiatric diseases such as cognitive impairment, schizophrenia, bipolar disorder, depression and anxiety.Reference Boerman, Cohen, Schulte and Nugter12,Reference Menon, Kar, Suthar and Nebhinani13 However, there also exist investigations that could not confirm this association.Reference Pan, Lu, Franco, Yu, Li and Lin14 So far, the majority of Mendelian randomisation studies investigating the relationship between 25OHD levels and psychiatric disorders have focused on depressionReference Libuda, Laabs, Ludwig, Bühlmeier, Antel and Hinney15 and schizophrenia,Reference Taylor, Burgess, Ware, Gage, Richard and Davey Smith16 mostly reporting no associations. However, some studies found a reverse relationship, especially in relation to depression.Reference Mulugeta, Lumsden and Hyppönen17,Reference Revez, Lin, Qiao, Xue, Holtz and Zhu18 One explanation for a reverse relationship is the consequences of symptoms of internalising disorders, i.e. it is plausible that withdrawal behaviour leads to reduced exposure to sunlight and thus to reduced vitamin D production. In addition, the loss of appetite that is often one of the symptoms of mental disorders impairs vitamin D absorption.
As a result of the aforementioned shared genetic basis between internalising disorders, combining disorders from the internalising dimension provides the opportunity to refine the selection of genetic variants across disorders and can thereby contribute to a better estimation of causality using Mendelian randomisation and could possibly reduce risk for pleiotropic effect (i.e. additional pathways from genetic variants because of the correlation of outcomes within the internalising spectrum). To the best of our knowledge, there is no Mendelian randomisation investigation integrating genome-wide association studies (GWASs) for different disorders from the internalising spectrum (e.g. depression, anxiety) in one common factor and assessing causal effects of 25OHD levels.
Aims
The current investigation aims at examining bidirectional causation between 25OHD levels and six disorders from the internalising spectrum, major depressive disorder (MDD), anxiety, post-traumatic stress disorder (PTSD), panic disorder, obsessive–compulsive disorder (OCD) and anorexia nervosa at the individual level and by combining shared genetic variants representing the internalising dimension.
Method
Mendelian randomisation analysis was conducted to evaluate causal effects using an instrumental variable framework for indirect estimation. Specifically, single-nucleotide polymorphisms (SNPs) from the largest GWAS of European descent on 25OHD levels served as instrumental variables.Reference Revez, Lin, Qiao, Xue, Holtz and Zhu18
There are three assumptions concerning the validity underlying this analytic approach:
(i) the instrumental variable should be associated with the exposure (relevance assumption);
(ii) the instrumental variable and outcome should not share common causes (exchangeability assumption);
(iii) the instrumental variable should influence the outcome only via the exposure (exclusion restriction).Reference Burgess, Foley and Zuber11,Reference Smith, Holmes, Davies and Ebrahim19
SNP-outcome associations were collected using genetic association data. Furthermore, several analyses were conducted to evaluate potential biasing effects of horizontal pleiotropy, selection bias and reverse causation.Reference Smith, Holmes, Davies and Ebrahim19–Reference Hemani, Bowden and Davey Smith22
Selection of instrumental variables for 25OHD
We selected 111 independent (not in linkage disequilibrium; r² < 0.001) SNPs associated with 25OHD levels from a GWAS in 417 580Reference Revez, Lin, Qiao, Xue, Holtz and Zhu18 participants (see Supplementary Table 1 available at https://doi.org/10.1192/bjp.2023.32) at a genome-wide significance level (P-value < 5 × 10−8) to assess the association between the instrumental variable and the exposure. Moreover, an F-statistic and the proportion of 25OHD-level variance explained by all 111 SNPs were calculated.
GWAS summary statistics for the six internalising phenotypes
For MDD, SNP-outcome associations stemmed from the largest GWAS meta-analysis with 45 591 patients with MDD and 97 674 controls (29 samples of European ancestry and 4 additional independent European-ancestry cohorts) without contributions of UK Biobank and 23andMe samples to avoid sample overlap with exposure GWAS.Reference Wray, Ripke, Mattheisen, Trzaskowski, Byrne and Abdellaoui23 The depression phenotype comprises a lifetime diagnosis of major depression following DSM-IV, ICD-9 or ICD-10 criteria assessed by a trained interviewer, clinician-administered checklists or medical record review. For anxiety, GWAS summary statistics stemmed from the Anxiety NeuroGenetics Study (ANGST) ConsortiumReference Otowa, Hek, Lee, Byrne, Mirza and Nivard24 with 5580 patients with diagnosed anxiety disorder and 11 730 controls. Summary statistic for PTSD was provided by the Psychiatric Genomics Consortium (PGC) with 12 080 patients with PTSD and 33 446 controls.Reference Nievergelt, Maihofer, Klengel, Atkinson, Chen and Choi25 Summary statistic for panic disorder included 2248 patients and 7992 controls of European ancestry from Denmark, Estonia, Sweden and Germany,Reference Forstner, Awasthi, Wolf, Maron, Erhardt and Czamara26 for OCD 2688 patients and 7037 controls from the International OCD Foundation Genetics Collaborative (IOCDF-GC) and the OCD Collaborative Genetics Association Study (OCGAS).Reference Arnold, Askland, Barlassina, Bellodi, Bienvenu and Black27 The summary statistic for anorexia nervosa contained 16 992 participants with anorexia nervosa and 55 525 controls from PGC, the Anorexia Nervosa Genetics Initiative (ANGI), the Wellcome Trust Case Control Consortium (WTCCC) as well as the UK BiobankReference Watson, Yilmaz, Thornton, Hübel, Coleman and Gaspar28 (Supplementary Table 1).
GWAS summary statistics for negative and positive control outcomes
We used the association between 25OHD and multiple sclerosis as a positive control outcome. This relationship is already well established by both observational and Mendelian randomisation studies.Reference Jacobs, Noyce, Giovannoni and Dobson29 If the causal relationship with multiple sclerosis cannot be replicated with the current exposure data-set,Reference Revez, Lin, Qiao, Xue, Holtz and Zhu18 validity of the used genetic variants should be questioned. GWAS summary statistics for multiple sclerosis were derived from the Wellcome Trust Case Control Consortium 2 (WTCCC2) and the Multiple Sclerosis Genetics Consortium (IMSGC).Reference Sawcer, Hellenthal, Pirinen, Spencer, Patsopoulos and Moutsianas30 The GWAS meta-analysis on ever-smoking served as negative control outcome stemming from the UK Biobank and the TAG Consortium (The Tobacco and Genetics Consortium).Reference Karlsson Linnér, Biroli, Kong, Meddens, Wedow and Fontana31 A potential association with a negative control outcome indicates violation of the instrumental variable assumptions.Reference Burgess, Foley and Zuber11
Statistical analyses
Shared genetic basis of the six internalising phenotypes: the internalising dimension
Following current transdiagnostic approaches to investigate mental disorders, we performed a common factor model without individual SNP effect by diagonally weighted least square (DWLS) estimation combining GWAS summary statistic data from the six internalising disorders, using GenomicSEM.Reference Grotzinger, Rhemtulla, de Vlaming, Ritchie, Mallard and Hill32 Model fit was evaluated with the comparative fit index (CFI), standardised root mean square residual (SRMR) and the square of standardised loading of the common internalising factor on the specific phenotypes. CFI scores of ≥0.90 are interpreted as adequate fit and values of ≥0.95 indicate a good model fit.Reference Hu and Bentler33 SRMR values below 0.10 point to an adequate model fit, values less than 0.05 can be interpreted as good fit and a value of 0 points as a perfect fitReference Kim, Turiano, Forbes, Kotov, Krueger and Eaton3Reference Ward, Tunbridge, Sandor, Lyall, Ferguson and Strawbridge4 (see also Supplementary Table 2 and Supplementary Material 1). Using the commonfactorGWAS function, we conducted a GWAS for the common internalising factor with DWLS estimation. This common factor GWAS included only SNPs exhibiting a non-significant heterogeneity test (P > 0.05). The effective sample size estimation was performed with a minor allele frequency between 0.4 and 0.1. This more holistic phenotype was termed ‘internalising factor’ in the Mendelian randomisation analyses.
Power analysis
Statistical power was calculated for a range of expected odds ratios (ORs, 0.60–0.90) for a given significance level of α = 0.05 according to the method described by Brion et al.Reference Brion, Shakhbazov and Visscher35 This method follows the asymptotic theory and performs power calculations via the non-centrality parameter from the respective asymptotic χ2-distribution. Further parameters for power calculations are the proportion of explained variance in the exposure related to the selected instrumental variables, the sample size of the outcome GWAS and its proportion of ‘cases’.
Primary analysis
For Mendelian randomisation analysis, we used Wald ratios by division of the log OR of the SNP-outcome association from the log OR of the SNP-exposure association, for each genetic variant. Standard errors (s.e.s) were computed by the delta method. Subsequently, these parameters were entered per SNP into the multiplicative random-effects inverse-variance weighted (IVW) model. Analyses were conducted at a false-discovery rate corrected threshold of 0.05 (q-value) to account for multiple testing.Reference Benjamini and Hochberg36
Sensitivity analysis: test for directional pleiotropy and pleiotropy-robust methods
Exclusion restriction assumption can be violated by horizontal pleiotropy (i.e. direct effects of genetic variants on the outcome independent of the exposure). To assess horizontal pleiotropic effects on the estimation of instrumental variables, we conducted Cochran's Q and the I² statistic investigating the heterogeneity of the estimates, which indicates the presence of pleiotropic effects. In the context of heterogeneity, leave-one out analysis was also performed to assess whether the IVW estimation strongly depended on a single SNP. Additionally, the Mendelian randomisation Egger intercept test was carried out to test for directional pleiotropy.
As pleiotropy-robust methods, we used weighted median, radial regression Mendelian randomisation and Mendelian randomisation pleiotropy residual sum and outlier (MR PRESSO).Reference Verbanck, Chen, Neale and Do37 In addition, causal analysis using summary effect estimates (CAUSE) were performed to compare the causal model (i.e. genetic variants affect the outcome through the exposure) and the sharing model (i.e. associations between variants and an unobserved heritable confounder that influences both exposure and outcome) for their goodness of fit.Reference Morrison, Knoblauch, Marcus, Stephens and He38 The CAUSE approach enables an enhanced control for false-positive causal results in the case of pleiotropy. Additionally, we searched the PhenoScanner database for previously reported associations of all SNPs related to our exposure with potential confounders causing pleiotropic effects, violating exchangeability assumption.Reference Kamat, Blackshaw, Young, Surendran, Burgess and Danesh39,Reference Staley, Blackshaw, Kamat, Ellis, Surendran and Sun40 As additional sensitivity analysis, we excluded SNPs associated with these potential confounders and compared Mendelian randomisation results with our original findings.
Test for reverse causation, and negative and positive control outcome
Reverse causation was examined by performing a Mendelian randomisation analysis using instrumental variables related to the outcomes (MDD, anxiety, PTSD, panic disorder, OCD, anorexia nervosa, the internalising factor) on the exposure with the CAUSE approach. Furthermore, we also conducted a multivariable Mendelian randomisation with additional pleiotropy-robust methods (IVW robust, Mendelian randomisation Egger, weighted median Mendelian randomisation methods) at a significant threshold of 5 × 10−06 estimating the causal effect of each internalising phenotype on vitamin D levels simultaneously in one model. For positive and negative control outcomes, corresponding Mendelian randomisation analyses were conducted with 25OHD levels as exposure variable on the outcomes multiple sclerosis and ever-smoking, respectively.
Replication analysis
To further assessed the validity and robustness of our analyses, we used genome-wide associated SNPs (P-value < 5 × 10−8) from a second 25OHD GWAS of 79 366 individuals of European descentReference Jiang, O'Reilly, Aschard, Hsu, Richards and Dupuis41 as exposure instrumental variables and carried out Mendelian randomisation analyses on the internalising disorders MDD, anxiety, PTSD, panic disorder, OCD, anorexia nervosa and the internalising factor.
All analyses were performed using the packages metaphor (2.4.0), MendelianRandomization (0.4.2), TwoSampleMR (0.5.5), MR PRESSO (1.0), CAUSE (1.2.0), and GenomicSEM (0.0.5c) in R, version 4.1. We report the methods and results following the STROBE-MR (Strengthening the Reporting of Observational studies in Epidemiology – Mendelian randomisation) statement.Reference Smith, Davies, Dimou, Egger, Gallo and Golub42
Results
Shared genetic basis of the six internalising phenotypes: the internalising dimension
The common factor model yielded a CFI of 0.923 and a SRMR of 0.108 (AIC = 56.048), indicating an adequate model fitReference Hu and Bentler33,Reference Hu, Bentler and RH34 (see Supplementary Table 2), although the SRMR value is located at the limits of the range. The model revealed strong positive loadings for MDD, anxiety, PTSD and moderate-sized loadings for panic disorder on the internalising factor. OCD and anorexia nervosa exhibit low loadings on the latent factor (see Fig. 1). The internalising factor explained 95.3% of the variance of MDD, 82.7% of anxiety, 47.6% of PTSD, 16.4% of panic disorder, 10.0% of OCD and 12.3% of anorexia nervosa. These findings support the assumption that there is a shared genetic basis of the six internalising phenotypes. The resulting summary statistic data consisted of 5 048244 SNPs associated with the internalising factor with an effective sample size of 102 837.45.

Fig. 1 Path diagram for the internalising factor (IF) with standardised loadings of major depressive disorder (MDD), anxiety (ANX), post-traumatic stress disorder (PTSD), panic disorder (PD), obsessive–compulsive disorder (OCD), and anorexia nervosa (AN). The rectangles represent the indicators, the latent common factor is presented as a circle. Single headed arrows indicate the direction of the regression effect with the standardised loadings. Double headed arrows reflect standardised residuals.
Primary analysis
The analysis had a power of ≥90% to detect a risk reduction in terms of ORs of at least 20% for four of six internalising disorders (MDD, anxiety, PTSD, anorexia nervosa). For panic disorder and OCD we had a power of ≥80% to detect a risk reduction of at least 35%. (Supplementary Table 3). Thus, the power of the current study is reasonably high, given the typically low power of Mendelian randomisation studies,Reference Burgess, Foley and Zuber11,Reference Larsson and Burgess43 however, it is comparable with other Mendelian randomisation studies on vitamin D and internalising disorders.Reference Libuda, Laabs, Ludwig, Bühlmeier, Antel and Hinney15,Reference Mulugeta, Lumsden and Hyppönen17
Analysis resulted in 111 SNPs that exhibited a minimum F-statistic of 30.8 each and together explained an overall variance of 3.4% of the exposure 25OHD levels. Standard IVW Mendelian randomisation analysis revealed no significant effects of 25OHD levels on MDD, anxiety, PTSD, panic disorder, OCD and anorexia nervosa as well as the internalising factor. For detailed statistics, we refer to Fig. 2 and Supplementary Table 4.
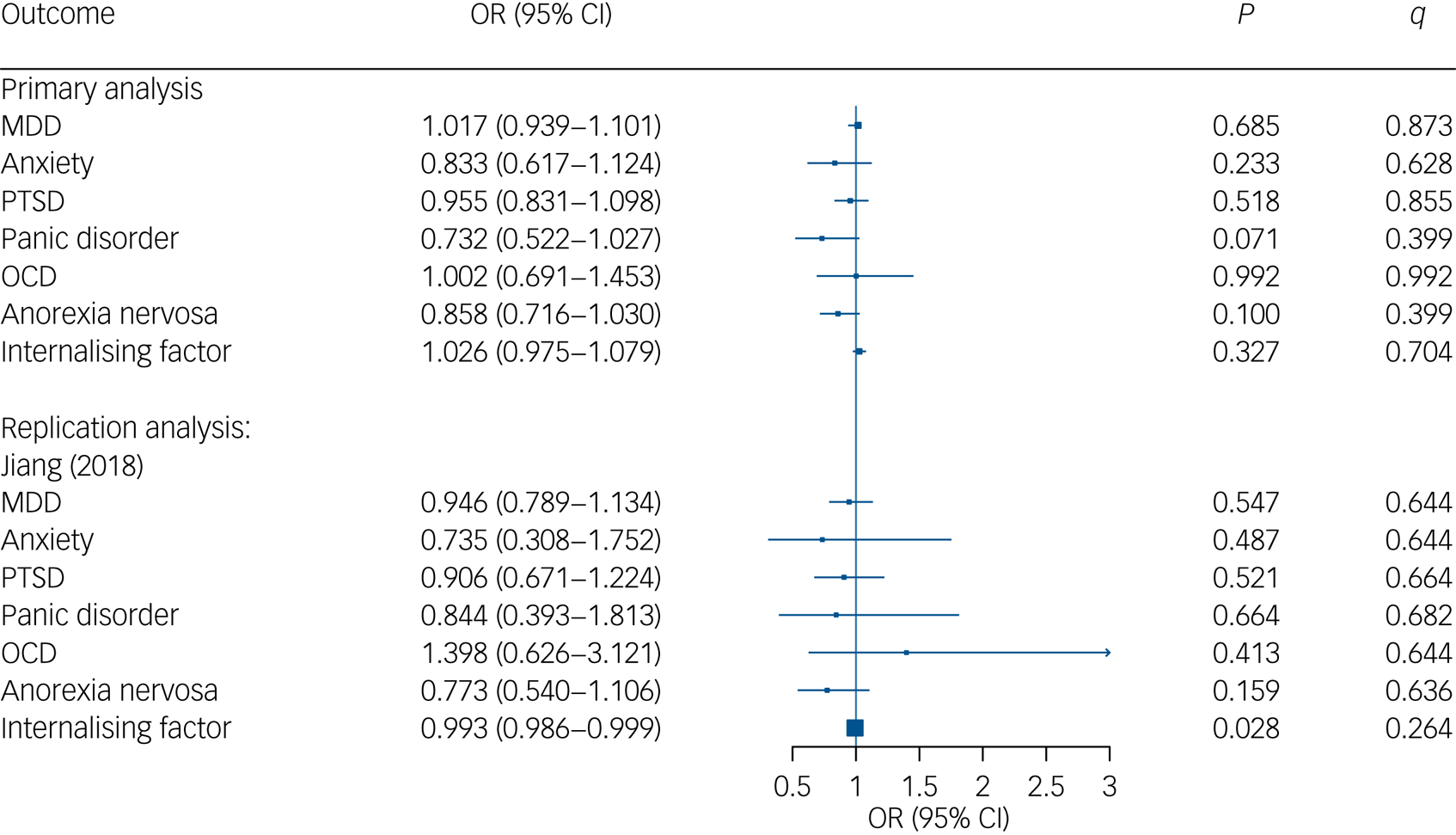
Fig. 2 Mendelian Randomisation estimates for association between genetically instrumented 25-hydroxyvitamin D (25OHD) and major depressive disorder (MDD), anxiety, post-traumatic stress disorder (PTSD), panic disorder, obsessive–compulsive disorder (OCD) as well as anorexia nervosa. CI, confidence interval; OR, odds ratio; q, adjusted P-values using a false-discovery rate approach.
Sensitivity analysis: test for directional pleiotropy and pleiotropy-robust methods
In anxiety, OCD, anorexia nervosa and the internalising factor, Cochran's Q surpassed the critical value of 122.11, 112.02, 104.14, and 100.75 (see Supplementary Table 5). For MDD, anxiety, PTSD, panic disorder, OCD and the internalising factor outcomes, tests for Mendelian randomisation Egger intercept did not reach statistical significance, thus indicating no directional pleiotropy. Only for anorexia nervosa (intercept −0.008, s.e. = 0.003, P = 0.019), the test for Mendelian randomisation Egger intercept revealed a significant result. Concerning pleiotropy-robust methods (weighted median, radial regression Mendelian randomisation and MR PRESSO), all tests revealed very similar results to the IVW analyses, supporting our null finding (see Supplementary Table 5). In the leave-one-out analysis, there was no significant result indicating that a single SNP strongly influenced model estimation (see Supplementary Table 6).
Regarding the CAUSE analyses, the goodness of fit did not significantly differ between the shared and causal model for MDD (OR = 0.970, 95% credible interval (CredIn) 0.869–1.094; P > 0.99), anxiety (OR = 0.887, 95% CredIn 0.651–1.234, P > 0.99), PTSD (OR = 1.010, 95% CredIn 0.970–1.041, P = 0.514), panic disorder (OR = 0.852, 95% CredIn 0.527–1.391, P > 0.99), OCD (OR = 0.779, 95% CredIn 0.477–1.259, P > 0.99), anorexia nervosa (OR = 1.094, 95% CredIn 0.896–1.297, P = 0.299) or the internalising factor (OR = 1.000, 95% CredIn 0.905–1.010, P > 0.99). This further supports no causal relationship between vitamin D and internalising disorders.
The PhenoScanner search identified 32 of 111 instrumental variables associated with traits such as serum lipid levels, blood, blood pressure and bone mineral density (see Supplementary Table 7). Excluding these SNPs, we found similar results compared with our primary analysis for MDD, anxiety, PTSD, panic disorder, OCD, anorexia nervosa and the internalising factor (see Supplementary Table 8).
Test for reverse causation, and negative and positive control outcome
Reverse causation analyses conducted with the CAUSE approach revealed no significant effect of MDD (OR = 1.000, 95% CredIn 0.980–1.030; P = 0.050), anxiety (OR = 1.000, 95% CredIn: 0.980–1.020, P > 0.99), PTSD (OR = 1.000, 95% CredIn 0.923–1.073, P > 0.99), panic disorder (OR = 1.010, 95% CredIn 0.990–1.030, P > 0.99), OCD (OR = 1.000, 95% CredIn 0.990–1.020, P > 0.99), anorexia nervosa (OR = 1.000, 95% CredIn 0.980–1.010, P > 0.99) and the internalising factor (OR = 0.980, 95% CredIn 0.961–1.000, P > 0.99) on 25 OHD levels.
In addition, multivariable Mendelian randomisation also showed no evidence for an effect of genetically predicted internalising disorders on 25OHD. Pleiotropy-robust methods (IVW robust, Mendelian randomisation Egger, weighted median Mendelian randomisation methods) revealed similar results (see Supplementary Table 9).
For the negative control outcome, we found no association between 25OHD levels and ever-smoking. However, we noted a significant relationship with the positive control outcome multiple sclerosis (see Supplementary Table 10).
Replication analysis
Analysis with six relevant genetic variants (all Fs > 25.00, total R² = 3.7%) from Jiang et al's (2018)Reference Jiang, O'Reilly, Aschard, Hsu, Richards and Dupuis41 GWAS also revealed no association between 25OHD levels and disorders from the internalising spectrum (MDD, anxiety, PTSD, panic disorder, OCD, anorexia nervosa) as well as the internalising factor, re-affirming the null association (see Supplementary Table 4 and Table 5).
Discussion
Main findings
To the best of our knowledge, this is the first study combining several disorders from the internalising spectrum in a transdiagnostic investigation of a bidirectional causal relationship between 25OHD levels and psychiatric symptoms. Using Mendelian randomisation with large sample sizes from the PGC and the UK Biobank, we found no evidence for a causal effect of 25OHD levels on the six internalising disorders, including MDD, anxiety, PTSD, panic disorder, OCD and anorexia nervosa. We also detected no effect of 25OHD levels on a latent trait representing the internalising dimension.
Interpretation of our findings and comparison with the literature
Although classic observational studies reported a significant inverse relationship with vitamin D, especially concerning depression,Reference Anglin, Samaan, Walter and McDonald44 our results are in line with most previous Mendelian randomisation studies assessing causation.Reference Libuda, Laabs, Ludwig, Bühlmeier, Antel and Hinney15 Additionally, most recent randomised clinical trials (RCTs) investigating vitamin D supplementation also reported null findings.Reference Jorde and Kubiak45 In particular, the recent RCT by Okereke et al (2020)Reference Okereke, Reynolds, Mischoulon, Chang, Vyas and Cook46 should be emphasised, which used a large-scale sample with 18 353 men and women and also reported no effect of vitamin D supplementation on depression symptoms in a 5-year follow-up study.
With regard to possible bidirectional causal effects, we found no evidence for a reverse relationship between 25OHD levels and the internalising phenotypes in the current study. Results of previous investigations are inconsistent, several studies reported null findings,Reference Libuda, Laabs, Ludwig, Bühlmeier, Antel and Hinney15 and a few found a reverse relationship.Reference Mulugeta, Lumsden and Hyppönen17,Reference Revez, Lin, Qiao, Xue, Holtz and Zhu18 These studies postulate that this reverse relationship is because of increased social isolation behaviour and thus reduced sunlight exposure and appetite in patients with internalising disorders, resulting in low 25OHD levels. Future research on the specific mechanisms underlying a possible reverse relationship is needed to clarify the conflicting results.
From a transdiagnostic perspective, the six subtypes of internalising disorders showed the same pattern of null findings at the individual disorder level. Going a step further, we combined a single GWAS to a common genetic internalising factor. Goodness of fit and standard loading on the single disorders confirmed existence of this shared genetic basis. There was no evidence for a causal relationship between 25OHD levels and the internalising dimension. Although Mendelian randomisation Egger analysis pointed to a significant non-zero pleiotropic effect, the robust Mendelian randomisation methods with partly different assumptions showed consistent results, underpinning the plausibility and robustness of the Mendelian randomisation analysis. These findings are in line with previous results showing shared genetic aetiology for closely related psychiatric traitsReference Ward, Tunbridge, Sandor, Lyall, Ferguson and Strawbridge4 and provide support for the dimensional view on psychiatric disorders and their biobehavioural substrates.
Looking at the results in the light of different models of psychopathologies, we refer to bifactorial models including an externalising and internalising dimension, which also postulate a general psychopathology factor.Reference Caspi, Houts, Belsky, Goldman-Mellor, Harrington and Israel47 This could also be a topic for future work with regard to the study of vitamin D as a broader transdiagnostic risk factor. From a hierarchical perspective, for example, the hierarchical taxonomy of psychopathology (HiTOP)Reference Kotov, Krueger, Watson, Achenbach, Althoff and Bagby48 assumes that the internalising dimension consists of a distress subfactor (unipolar mood, generalised anxiety and post-traumatic stress disorders), an anxiety subfactor (social anxiety disorder, phobias, OCD, agoraphobia and panic disorder), eating disorders and sexual problems. Looking more closely at the individual contribution of each indicator to the current latent internalising factor, the results may reflect the subdivisions of the HiTOP with strong loadings on the distress subfactor and lower loadings on the anxiety subfactor or anorexia nervosa. For the current study, we conceptualised our common factor model according to a priori considerations, as well as previous findings on the quality of risk prediction.Reference Kim, Turiano, Forbes, Kotov, Krueger and Eaton3 However, future research should focus strongly on the structure of psychopathology models in terms of a common genetic basis and underlying transdiagnostic risk factors.
Strengths and limitations
Notable strengths of our study are the large sample size of the outcome studies comprising a broad spectrum of the internalising dimension. A series of sensitivity analyses revealed that the Mendelian randomisation estimates were consistent and robust to various approaches assessing model violations. To minimise the possibility of population stratification bias, the 25OHD and internalising disorder SNP effect estimates were obtained from studies of European descent. Further, by exploiting a second independent 25OHD GWAS for exposure instrumental variables,Reference Jiang, O'Reilly, Aschard, Hsu, Richards and Dupuis41 we were able to replicate our initial findings and rule out weak instrument bias. Finally, the results of the positive and negative control outcome analyses provide additional confidence against pleiotropic pathway bias.
Our study has some limitations. We only included data-sets with European participants, which restricts the generalizability of our findings to other populations. For instance, sunlight exposure and 25OHD levels are known to be different in European and non-European ethnic minority samples.Reference Renzaho, Halliday and Nowson49 We were only able to detect an OR ≤ 0.90 (depending on the specific outcome) with a power of 90% and thus, smaller true effect sizes would have remained undetectable. The selected genetic instruments explained 3.4% of the total variance in 25OHD levels, which is common in Mendelian randomisation research. This shows that variations in vitamin D levels are mostly attributable to environmental factors (e.g. sunlight exposure, dietary behaviour), which could not be captured by Mendelian randomisation studies. However, the genetic instruments used in our study were all valid with F-statistics ≥ 30.8, and with the complementary CAUSE approach we incorporated all genetic variants, thereby increasing statistical power.
Implications
According to the transdiagnostic approach of phenotypes and biobehavioural substrates, we found the same pattern of results for the six types of internalising disorders (MDD, anxiety, PTSD, panic disorder, OCD, anorexia nervosa), at the individual disorder level and for the complex phenotype representing the internalising dimension. There was no evidence for causal relationships between 25OHD and phenotypes in either direction. These findings were confirmed by a series of complementary and pleiotropy-robust approaches.
Data availability
GWAS summary statistics for 25OHD levels from Revez et al. (2020)18 is available at https://cnsgenomics.com/data/revez_20/, for major depressive disorder from the Psychiatric Genomics Consortium at https://figshare.com/articles/dataset/mdd2018/14672085, for PTSD, panic disorder, OCD and anorexia nervosa at https://www.med.unc.edu/pgc/download-results/.
Acknowledgments
The authors assert that all procedures contributing to this work comply with the ethical standards of the relevant national and institutional committees on human experimentation and with the Helsinki Declaration of 1975, as revised in 2008. All procedures involving human participants were approved by the committee in the original studies. Written informed consent was obtained from all participants in the original studies.
Author contributions
H.B. and J.K. designed the study. J.K., H.B., T.W.W. and S.-E.B. performed the analysis. J.K. and H.B. drafted the manuscript. S.-E.B., T.W.W., M.F.L. and H.B. provided editorial revisions and suggestions.
Funding
This work was supported by the German Research Foundation (DFG) (grant number: BA 5459/2-1).
Declaration of interest
None.
eLetters
No eLetters have been published for this article.