Introduction
The identities of all organisms are embedded in their genes, which are often influenced by developmental and environmental cues. Sequential and temporal decoding of these genes confers physiological distinctiveness to each individual (Anderson Reference Anderson2008). In the last two decades, the term “omics” has been suffixed with several fields of study in biology (Brunetti et al. Reference Brunetti, Neto, Vera, Taboada, Pavarini, Bauermeister and Lopes2018). Recent advances in high-throughput functional omics technologies (Table 1) have facilitated an understanding of the various molecular–environmental interactions that regulate biological systems (Kitano Reference Kitano2002). The use of omics techniques to study various biological aspects would provide greater opportunities to dissect the molecular and physiological mechanisms in developing resilient phenotypes. Among the various omics platforms, functional genomics has seen rapid progress, resulting in a growing number of sequenced plant genomes. This has facilitated the development of plants selected for specific agronomic traits and biological processes (Kantar et al. Reference Kantar, Nashoba, Anderson, Blackman and Rieseberg2017; Nelson et al. Reference Nelson, Wiesner-Hanks, Wisser and Balint-Kurti2018). The traditional giants of omics platforms encompass genomics, transcriptomics, and proteomics (Palsson Reference Palsson2002; Rochfort Reference Rochfort2005). While genomics aims to understand how the genome functions, transcriptomics and proteomics perform systematic qualitative and quantitative analysis of the transcriptome and proteome content, respectively, in a tissue, cell, or subcellular compartment. Other recent omics techniques such as metabolomics, phenomics, and lipidomics complement the traditional techniques to depict a precise picture of the entire cellular process.
Table 1 Examples of applications of omics approaches in plant systems biology research.

Omics approaches in weeds science have been gaining momentum over the past decade. As with other domains, the number of studies using genomic approaches to investigate weed biology and physiology has increased over the years (Basu et al. Reference Basu, Halfhill, Mueller and Stewart2004; Chao et al. Reference Chao, Horvath, Anderson and Foley2005; Guo et al. Reference Guo, Qiu, Ye, Jin, Mao, Zhang, Yang, Peng, Wang, Jia and Lin2017; He et al. Reference He, Kim and Park2017; Kreiner et al. Reference Kreiner, Stinchcombe and Wright2018; Molin et al. Reference Molin, Wright, Lawton-Rauh and Saski2017; Olsen et al. 2007; Tranel and Horvath Reference Tranel and Horvath2009). DNA-based molecular studies using simple sequence repeats (SSRs), microsatellites, amplified fragment length polymorphisms (AFLPs), and inter simple sequence repeats (ISSRs) have provided tremendous opportunities to study weedy characteristics such as resilience, dormancy, and invasiveness, as well as weed genetic diversity and hybridization among related weed species (Corbett and Tardif Reference Corbett and Tardif2006; Horvath Reference Horvath2010). Excellent reviews on weed genomics and DNA-based herbicide-resistance techniques have been produced by Basu et al. (Reference Basu, Halfhill, Mueller and Stewart2004), Corbett and Tardif (Reference Corbett and Tardif2006), Stewart (Reference Stewart2009), and Tranel and Horvath (Reference Tranel and Horvath2009). Recently, the weed science community has initiated the International Weed Genomics Consortium to facilitate genomics for weed science (Ravet et al. Reference Ravet, Patterson, Krähmer, Hamouzová, Fan, Jasieniuk, Lawton-Rauh, Malone, McElroy, Merotto, Westra, Preston, Vila-Aiub, Busi, Tranel, Reinhardt, Saski, Beffa, Neve and Gaines2018). However, applications of other omics for studying agronomically important weeds are at a nascent stage, as seen by the limited number of published studies (Table 2).
Table 2 Examples of omics papers on phytotoxins, including herbicides.
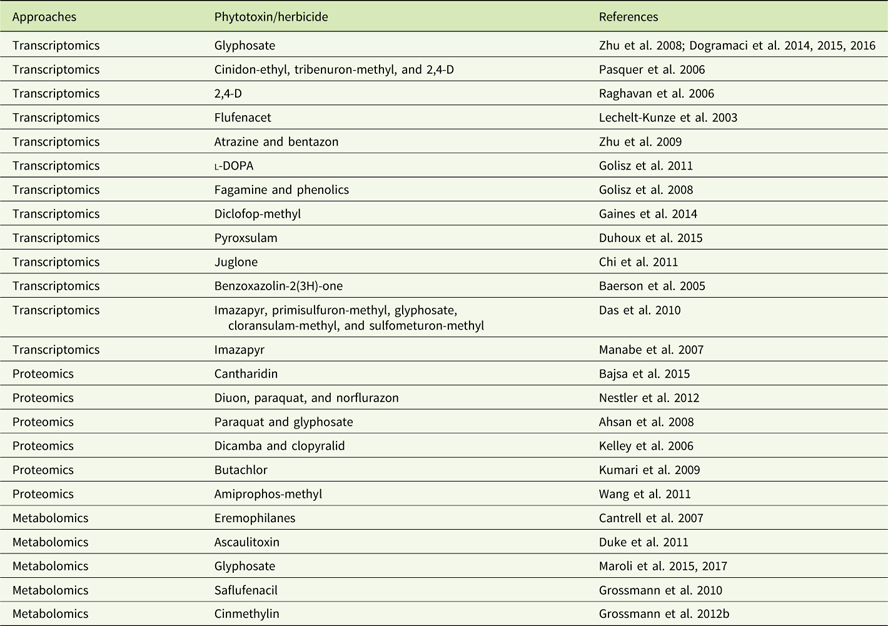
In addition to genomics, other omics techniques have also been used to investigate areas critical to weed science, including stress response, weediness/invasiveness, herbicide resistance, and genetic diversity (Délye Reference Délye2013; Grossmann et al Reference Grossmann, Niggeweg, Christiansen, Looser and Ehrhardt2010; Keith et al Reference Keith, Burns, Bothner, Carey, Mazurie, Hilmer, Biyiklioglu, Budak and Dyer2017; Stewart Reference Stewart2009; Stewart et al. Reference Stewart, Tranel, Horvath, Anderson, Rieseberg, Westwood, Mallory-Smith, Zapiola and Dlugosch2009, Reference Stewart, Yanhui, Abercrombie, Halfhill, Rao, Ranjan, Hu, Sammons, Heck, Tranel and Yuan2010; Zhang and Reichers Reference Zhang and Reichers2008). However, due to the complexity of the molecular and environmental interactions, no single omics analysis can independently explain the intricacies of fundamental physiology (Fukushima et al. Reference Fukushima, Kusano, Redestig, Arita and Saito2009; Hirai et al. Reference Hirai, Yano, Goodenowe, Kanaya, Kimura, Awazuhara, Arita, Fujiwara and Saito2004; Liberman et al. Reference Liberman, Sozzani and Benfey2012). Hence, an integrated systems biology approach is needed to provide precise information about the molecular, biochemical, and physiological status of the target organism (Figure 1). An integrated systems biology approach can help not only in annotating unknown genes, but also in identifying their regulatory networks and the metabolic pathways they would influence (Pérez-Alonso et al. Reference Pérez-Alonso, Carrasco-Loba, Medina, Vicente-Carbajosa and Pollmann2018). This would aid in understanding the genotype–phenotype relationship and consequently help to improve the existing weed management strategies in agricultural fields. Although there are several omics platforms, the present review will strive to highlight omics approaches used to study physiological aspects of agriculturally important weeds that have not been previously touched upon, such as elucidating physiology of bud dormancy, deciphering the mechanisms of herbicide resistance, and identifying potential herbicidal phytochemicals using omics approaches.
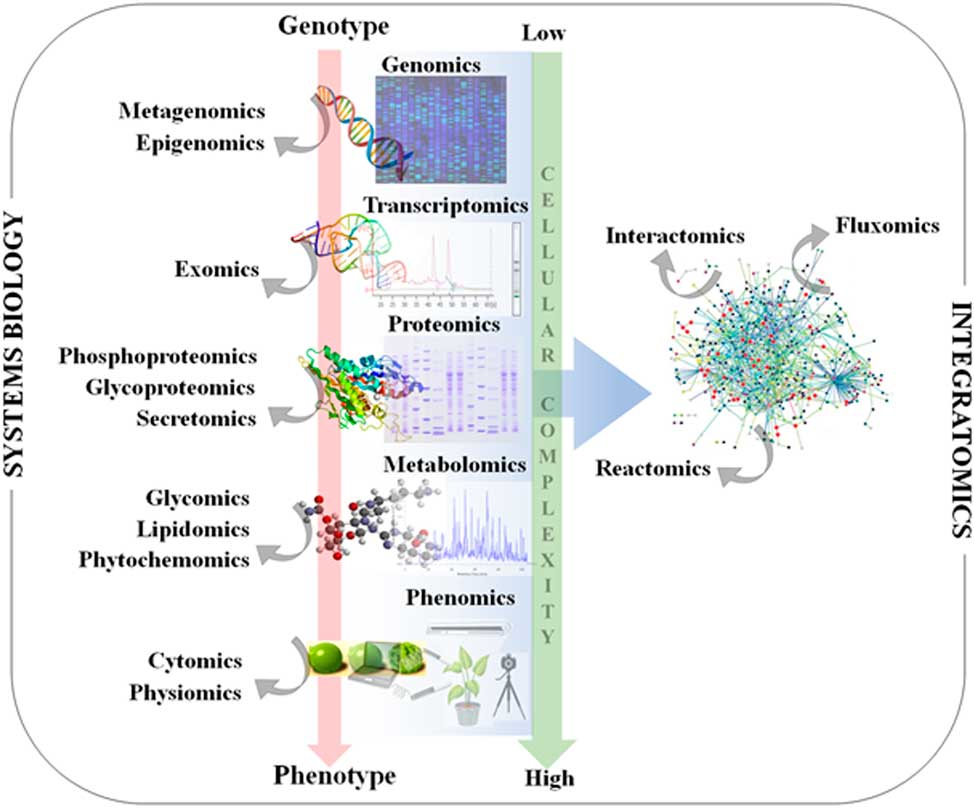
Figure 1 Classical systems biology concept and omics organization. The central dogma of molecular biology covers the progressive functionalization of the genotype to the phenotype. The omics techniques track and capture various molecular entities across the biological system.
Transcriptomics to Investigate Herbicide Resistance
Compared with the availability of genome sequence information and genetic resources for model plants such as mouse-ear cress [Arabidopsis thaliana (L.) Heynh.] (Arabidopsis Genome Initiative 2000), barrelclover (Medicago truncatula Gaertn.) (Bell et al. Reference Bell, Dixon, Farmer, Flores, Inman, Gonzales, Harrison, Paiva, Scott, Weller and May2001), and purple false brome [Brachypodium distachyon (L.) P. Beauv.] (Vogel et al. Reference Vogel, Garvin, Mockler, Schmutz, Rokhsar, Bevan, Barry, Lucas, Harmon-Smith, Lail and Tice2010) and the genome sequences of several other dicot and monocot crops that are either sequenced or soon will be, to date only four draft genome assemblies have been completed for agronomic weed species (Table 3). Next-generation sequencing (NGS) techniques such as RNA-Seq have enabled accurate and powerful transcriptome analysis approaches for non-model species such as weeds, without requiring a fully assembled genome. A review of 15 RNA-Seq studies conducted in weeds to find candidate genes for herbicide resistance and abiotic stress tolerance identified that increased replicate number and controlling genetic background were important factors to increase detection power and minimize the false-discovery rate (Giacomini et al. Reference Giacomini, Gaines, Beffa and Tranel2018). The first weed species transcriptomes released were horseweed (Erigeron canadensis L.) (Peng et al. Reference Peng, Abercrombie, Yuan, Riggins, Sammons, Tranel and Stewart2010) and waterhemp [Amaranthus tuberculatus (Moq.) J. D. Sauer] (Riggins et al. Reference Riggins, Peng, Stewart and Tranel2010), with at least 22 weed transcriptomes sequenced and assembled to date, including weeds of agronomic crops, turfgrass, and invasive weeds (Gaines et al. Reference Gaines, Tranel, Fleming, Patterson, Küpper, Ravet, Giacomini, Gonzalez and Beffa2017; McElroy Reference McElroy2018). Compared with transcriptomics methods such as microarray, which provide relative quantification, NGS-based transcriptome approaches produce absolute quantification of transcript expression as well as the sequence of all transcripts in a given sample. All expressed genes can be studied for changes in regulation (for example, upregulation of cytochrome P450s to increase herbicide metabolism), and several genes can be examined for candidate nonsynonymous mutations that could confer resistance. The identification of transcripts with differential regulation and/or mutations generates a hypothesis to be tested with subsequent validation. For discussion about transcriptomics in weeds before the introduction of NGS, the reader is referred to reviews by Lee and Tranel (Reference Lee and Tranel2008) and Horvath (Reference Horvath2010).
Table 3 Draft genome assemblies of agronomic weed species sequenced using next-generation sequencing technologies.

RNA-Seq measures the transcriptome abundance at a given time from a genome. The data can be used for various analyses, such as identification of differentially expressed transcripts between treatments, analysis of sequence variants, or characterization of alternative splicing. Due to its digital nature, RNA-Seq has a linear-detection dynamic range over five orders of magnitude, enabling quantification of even transcripts with very low expression. A typical RNA-Seq experiment consists of the steps outlined in Figure 2. Numerous downstream transcriptome data analyses can also be used to help interpret data, such as identification of enriched pathways with differentially expressed transcripts. RNA-Seq is also advantageous for studying complex gene families, such as those involved in enhanced metabolic resistance (for example, cytochrome P450s, glutathione-S-transferases, glucosyl transferases, ABC transporters). The results of the RNA-Seq experiments alone are not sufficient to prove causation for a candidate mechanism. RNA-Seq should be considered an experimental approach to generate robust hypotheses for candidate gene function. Subsequent forward genetics validation experiments are essential to prove function, such as testing cosegregation of a molecular marker (increased gene expression and/or a mutation) with resistance, testing for the presence of the molecular marker in unrelated populations of the same species, and preferably expression or knockout in a heterologous system such as Arabidopsis or yeast (for example, Cummins et al. Reference Cummins, Wortley, Sabbadin, He, Coxon, Straker, Sellars, Knight, Edwards, Hughes, Kaundun, Hutchings, Steel and Edwards2013; LeClere et al. Reference LeClere, Wu, Westra and Sammons2018). Differential expression can then be measured on validation samples using qRT-PCR on cDNA.

Figure 2 Workflow of transcript analyses by RNA-Seq and qRT-PCR.
In weed science, several transcriptomic studies have focused on herbicide-resistance traits, including target-site resistance mechanisms (Riggins et al. Reference Riggins, Peng, Stewart and Tranel2010; Wiersma et al. Reference Wiersma, Gaines, Preston, Hamilton, Giacomini, Buell, Leach and Westra2015; Yang et al. Reference Yang, Yu and Li2013) and non–target site resistance (NTSR) mechanisms (An et al. Reference An, Shen, Ma, Yang, Liu and Chen2014; Gaines et al. Reference Gaines, Lorentz, Figge, Herrmann, Maiwald, Ott, Han, Busi, Yu, Powles and Beffa2014; Gardin et al. Reference Gardin, Gouzy, Carrere and Delye2015; Leslie and Baucom Reference Leslie and Baucom2014; Peng et al. Reference Peng, Abercrombie, Yuan, Riggins, Sammons, Tranel and Stewart2010; Riggins et al. Reference Riggins, Peng, Stewart and Tranel2010; Yang et al. Reference Yang, Yu and Li2013). Studying the entire transcriptome is especially useful for NTSR mechanisms, because NTSR generally involves multiple genes and gene families (Délye Reference Délye2013). Examples of RNA-Seq studies on NTSR in grasses include acetyl-CoA carboxylase (ACCase)-inhibitor resistance in rigid ryegrass (Lolium rigidum Gaudin) (Gaines et al. Reference Gaines, Lorentz, Figge, Herrmann, Maiwald, Ott, Han, Busi, Yu, Powles and Beffa2014) and Brachypodium hybridum Catalán, Joch. Müll., L.A. Mur & T. Langdon (Matzrafi et al. Reference Matzrafi, Shaar-Moshe, Rubin and Peleg2017) and acetolactate synthase (ALS)-inhibitor resistance in L. rigidum (Duhoux et al. Reference Duhoux, Carrère, Gouzy, Bonin and Délye2015) and blackgrass (Alopecurus myosuroides Huds.) (Gardin et al. Reference Gardin, Gouzy, Carrere and Delye2015). Both NTSR and target-site mechanisms were evaluated in barnyardgrass [Echinochloa crus-galli (L.) P. Beauv.], using NGS to identify candidate genes involved in ALS-inhibitor and synthetic auxin (quinclorac) resistance (Yang et al. Reference Yang, Yu and Li2013). Responses to glyphosate and glyphosate resistance have also been studied using RNA-Seq in tall morningglory [Ipomoea purpurea (L.) Roth] (Leslie and Baucom Reference Leslie and Baucom2014) and kochia [Bassia scoparia (L.) A. J. Scott] (Wiersma et al. Reference Wiersma, Gaines, Preston, Hamilton, Giacomini, Buell, Leach and Westra2015). Employing differential expression (DE) analysis using RNA-Seq, the study by Leslie and Baucom (Reference Leslie and Baucom2014) found a range of candidate genes that may explain differences in glyphosate response between populations, including metabolism, signaling, and defense-related genes with differential expression. Similarly, in glyphosate-resistant B. scoparia, RNA-Seq was used to confirm overexpression of 5-enolpyruvylshikimate-3-phosphate synthase (EPSPS) due to gene amplification, to determine that no other genes in the shikimate pathway besides EPSPS were differentially expressed between resistant and susceptible populations, and to establish that no candidate resistance-conferring mutations were present in the EPSPS sequence from the resistant population (Wiersma et al. Reference Wiersma, Gaines, Preston, Hamilton, Giacomini, Buell, Leach and Westra2015). This mutation analysis is referred to as deep sequencing, and it is used to identify mutations that may be expressed at a low level and not detected by traditional sequencing approaches. Recently, a mutation for dicamba resistance in B. scoparia was identified using transcriptomics and subsequently functionally validated using forward genetics and expression in heterologous systems (LeClere et al. Reference LeClere, Wu, Westra and Sammons2018)
Transcriptomics to Investigate Bud Dormancy and Vegetative Growth
Early studies on bud dormancy employed traditional or accessible molecular approaches (Anderson et al. Reference Anderson, Gesch, Jia, Chao and Horvath2005; Horvath and Anderson Reference Horvath and Anderson2002; Horvath et al. Reference Horvath, Chao and Anderson2002). However, accessibility of the genome sequence for A. thaliana (Arabidopsis Genome Initiative 2000) and availability and adoption of cDNA microarray technology for plant genes (Schena et al. Reference Schena, Shalon, Davis and Brown1995) allowed researchers to examine transcriptome profiles for a variety of tissues and treatments and enabled the development of cross-species adoption of Arabidopsis platforms (Horvath et al. Reference Horvath, Schaffer, West and Wisman2003). Omics approaches were employed for elucidating signals, pathways, and mechanisms governing dormancy in underground adventitious buds (UABs) of leafy spurge (Euphorbia esula L.) (Anderson and Horvath Reference Anderson and Horvath2001; Anderson et al. Reference Anderson, Delseny, Fregene, Jorge, Mba, Lopez, Restrepo, Soto, Piegu, Verdier and Cooke2004, Reference Anderson, Horvath, Chao, Foley, Hernandez, Thimmapuram, Liu, Gong, Band, Kim and Mikel2007; Foley et al. Reference Foley, Chao, Horvath, Doğramacı and Anderson2013; Horvath et al. Reference Horvath, Anderson, Soto-Suárez and Chao2006, Reference Horvath, Chao, Suttle, Thimmapuram and Anderson2008). Euphorbia esula is a noxious and perennial rangeland weed that can reproduce and spread vegetatively from an abundance of UABs (Anderson et al. Reference Anderson, Gesch, Jia, Chao and Horvath2005). As dormancy in these buds often contributes to escape from control measures, it is essential to understand the seasonal dormancy cycles (paradormancy, endodormancy, and ecodormancy) for UABs. Horvath et al. (Reference Horvath, Anderson, Soto-Suárez and Chao2006, Reference Horvath, Chao, Suttle, Thimmapuram and Anderson2008) studied the transcriptome of E. esula UABs during transitions in these well-defined phases of dormancy under greenhouse and field conditions using high-density microarrays constructed from an E. esula expressed sequence tag database (Anderson et al. Reference Anderson, Horvath, Chao, Foley, Hernandez, Thimmapuram, Liu, Gong, Band, Kim and Mikel2007). This work helped to identify transcripts encoded by a gene with similarity to DORMANCY ASSOCIATED MADS-BOX, which has since been strongly implicated in dormancy processes of several perennial plant systems (Horvath Reference Horvath2015). Meta-analysis of microarray-based transcriptome data also identified transcripts similar to Arabidopsis COP1, HY5, MAF3-like, RD22, and RVE1 as potential molecular markers for endodormancy in E. esula UABs (Doğramacı et al. Reference Doğramacı, Horvath and Anderson2015).
Studies have also been done to determine the impact of growth regulators on dormancy by examining changes in transcriptome profiles of E. esula UABs in response to foliar glyphosate treatment (Doğramacı et al. Reference Doğramacı, Anderson, Chao and Foley2014, Reference Doğramacı, Horvath and Anderson2015, Reference Doğramacı, Gramig, Anderson, Chao and Foley2016). Although glyphosate is widely used as a broad-spectrum herbicide (Duke and Powles Reference Duke and Powles2008), it is also known to have hormetic activity (Belz and Duke Reference Belz and Duke2014; Velini et al. Reference Velini, Alves, Godoy, Meschede, Souza and Duke2008). When applied at sublethal concentrations, it can cause tillering in some plant species due to axillary and root-bud growth. Maxwell et al. (Reference Maxwell, Foley and Fay1987) reported that glyphosate application at higher rates (~2 to 6 kg ae ha−1) to E. esula under field conditions caused an increase in the number of stems per square meter as a result of shoot growth from UABs, a phenomenon referred to as “witches’ brooming.” Discovery or development of a growth regulator that could induce or inhibit shoot growth from UABs would be a significant step toward long-term control of other perennial weeds such as Canada thistle [Cirsium arvense (L.) Scop.], field bindweed (Convolvulus arvensis L.), and hedge bindweed [Calystegia sepium (L.) R. Br.]. Initial studies conducted using qRT-PCR, indicated that glyphosate had the most significant impact on abundance of ENT-COPALYL DIPHOSPHATE SYNTHETASE 1, which is involved in a committed step for gibberellic acid (GA) biosynthesis, and auxin transporters, including PINs, PIN-LIKES, and ABC TRANSPORTERS. Foliar glyphosate treatment also reduced the abundance of transcripts involved in cell cycle processes, which was consistent with altered growth patterns (Doğramacı et al. Reference Doğramacı, Anderson, Chao and Foley2014).
RNA-Seq identified nearly 13,000 differentially expressed transcripts in UABs in response to foliar glyphosate treatment (Doğramacı et al. Reference Doğramacı, Horvath and Anderson2015). Of these transcripts, 6,239 had significant changes ≥ 2-fold in either direction, which included transcripts associated with many processes involving shoot apical meristem maintenance and stem growth. The foliar glyphosate treatment increased shikimate abundance in UABs before decapitation of aboveground shoots, indicating that EPSPS, the target site of glyphosate, was inhibited. Interestingly, the abundance of shikimate in new aerial shoots (6 wk after growth-inducing decapitation) derived from UABs of foliar glyphosate-treated plants was similar to controls. The abundance of transcripts (i.e., EPSPS, EMB1144, SK1) involved in various stages of chorismate/shikimate biosynthesis had little change in amplitude, indicating glyphosate was not directly affecting transcription for components of the pathway in these tissues. Hormone analyses indicated that auxins, gibberellins (precursors and catabolites of bioactive gibberellins), and cytokinins (precursors and bioactive cytokinins) were more abundant in the aboveground shoots derived from UABs of glyphosate-treated plants versus the control. Based on the accumulation of transcriptome and metabolite data, it was proposed that the classic stunted and bushy phenotypes resulting from vegetative reproduction of E. esula UABs following foliar glyphosate treatment involve complex interactions, including shoot apical meristem maintenance, hormone biosynthesis and signaling (auxin, cytokinins, gibberellins, and strigolactones), cellular transport, and detoxification mechanisms (Doğramacı et al. Reference Doğramacı, Horvath and Anderson2015).
An expanded investigation into glyphosate-induced witches’ brooming under field conditions was accomplished (Doğramacı et al. Reference Doğramacı, Gramig, Anderson, Chao and Foley2016). Field plots treated with high rates (3.3 and 6.7 kg ae ha−1) of glyphosate had increased UAB-derived shoots displaying the stunted and bushy phenotype characteristics. qRT-PCR analysis to quantify the abundance of a selected set of transcripts in UABs of nontreated versus treated plants (0 vs. 6.7 kg ae ha−1) further supported the impact that glyphosate has on molecular processes involved in biosynthesis or signaling of tryptophan or auxin, GA, ethylene, and cytokinins, as well as cell cycle processes. Moreover, these glyphosate-induced effects on vegetative growth and transcript abundance persisted in the field for at least 2 yr. Transcriptome studies have now progressed to a point where testable hypothesis-driven studies could be initiated as a step toward next-generation approaches for weed management. Though foliar application of glyphosate to E. esula causes effects that impact molecular processes in UABs, this broad-spectrum herbicide would not be ideal for manipulation of bud growth in rangeland perennial weeds due to its effect on non–target plant species. Nevertheless, this proof of concept project sets the stage to screen commercially available libraries of compounds, growth regulators, natural products, and other bioactive molecules that could be applied to perturb bud growth and shoot development.
Although transcriptome and metabolite analysis can identify potentially important signals, pathways, and molecular mechanisms involved in dormancy and glyphosate-induced witches’ brooming, it is important to remember that these changes in transcript abundance do not reflect a direct association with activity occurring at the posttranscriptional levels (Beckwith and Yanovsky Reference Beckwith and Yanovsky2014). Moreover, as with many weedy species, the genome for E. esula has not been completely sequenced or annotated. Therefore, research employing molecular, genomics, and genetics approaches must rely on the annotated genomes of model species such as Arabidopsis.
Metabolomics and Fluxomics to Understand Weed Physiology
The realization that genes, transcripts, and proteins alone cannot completely explain several physiological responses has triggered a marked increase in employing approaches that can relate gene expression to the final phenotypic outcome. Metabolomics is one such approach that comprehensively identifies and quantitates low-molecular-weight metabolites (metabolome), thus offering a powerful approach for molecular phenotyping (Fiehn Reference Fiehn2002). A common workflow for metabolomics experiments involves metabolite extraction, chromatographic separation, detection, data processing, metabolite identification, and statistical validation (Figure 3). Most often in plant metabolomics, metabolite separation is carried out by either liquid chromatography or gas chromatography followed by mass spectrometer detection (De Vos et al. Reference De Vos, Moco, Lommen, Keurentjes, Bino and Hall2007; Haggarty and Burgess, Reference Haggarty and Burgess2017; Maroli et al. Reference Maroli, Nandula, Dayan, Duke, Gerard and Tharayil2015, Reference Maroli, Nandula, Duke, Gerard and Tharayil2017).

Figure 3 Designing a metabolomics study. (A) The various approaches for performing a metabolomics experimental study. GC-MS, gas chromatography–mass spectrometry; HILIC-LC-MS/MS, hydrophilic interaction chromatography for liquid chromatography–tandem mass spectrometry; LC-MS/MS, liquid chromatography–tandem mass spectrometry. (B) The general metabolomics workflow. It involves formulating a biological question, setting up an experimental design to test the hypothesis, sample treatment and harvest, metabolite extraction, clean-up, chromatographic separation, identification, statistical validation, and functional interpretation.
Metabolomics has been used in the past decade to study the mechanisms of action (MOAs) of synthetic and natural herbicidal compounds using several model plant species, such as maize (Zea mays L.) (Araníbar et al. Reference Araníbar, Singh, Stockton and Ott2001), sterile oat (Avena sterilis L.) (Aliferis and Chrysayi-Tokousbalides Reference Aliferis and Chrysayi-Tokousbalides2006), and Arabidopsis (Jaini et al. Reference Jaini, Wang, Dudareva, Chapple and Morgan2017; Sumner et al. Reference Sumner, Lei, Nikolau and Saito2015; Wu et al. Reference Wu, Tohge, Cuadros-Inostroza, Tong, Tenenboim, Kooke, Méret, Keurentjes, Nikoloski, Fernie and Willmitzer2018). However, limited studies have employed metabolomics to characterize weed physiology in response to herbicide applications (Aliferis and Chrysayi-Tokousbalides Reference Aliferis and Chrysayi-Tokousbalides2011; Miyagi et al. Reference Miyagi, Takahara, Takahashi, Kawai-Yamada and Uchimiya2010), herbicide-resistance mechanisms (Aliferis and Jabaji Reference Aliferis and Jabaji2011; Maroli et al. Reference Maroli, Nandula, Dayan, Duke, Gerard and Tharayil2015, Reference Maroli, Nandula, Duke, Gerard and Tharayil2017; Serra et al. Reference Serra, Couée, Renault, Gouesbet and Sulmon2015; Vivancos et al. Reference Vivancos, Driscoll, Bulman, Ying, Emami, Treumann, Mauve, Noctor and Foyer2011), and non–target site herbicide-resistance mechanisms such as detoxification and metabolism (Wang et al. Reference Wang, Lin, Chiang and Wang2017). As reviewed earlier, application of genomics and transcriptomics has helped to identify herbicide-resistance mechanisms in some weeds (Chen et al. Reference Chen, Huang, Wei, Huang, Wang and Zhang2017; Délye Reference Délye2013; Gaines et al. Reference Gaines, Zhang, Wang, Bukun, Chisholm, Shaner, Nissen, Patzoldt, Tranel, Culpepper, Grey, Webster, Vencill, Sammons, Jiang, Preston, Leach and Westra2010; Nandula et al. Reference Nandula, Reddy, Koger, Poston, Rimando, Duke, Bond and Ribeiro2012; Wright et al. Reference Wright, Rodriguez-Carres, Sasidharan, Koski, Peterson, Nandula, Ray, Bond and Shaw2018a, Reference Wright, Sasidharan, Koski, Rodriguez-Carres, Peterson, Nandula, Ray, Bond and Shaw2018b). Apart from this, metabolomics approaches have been recently adopted to understand effect of chemical stresses on perennial ryegrass (Lolium perenne L.) (Serra et al. Reference Serra, Couée, Renault, Gouesbet and Sulmon2015), to identify complementary glyphosate resistance mechanisms in Palmer amaranth (Amaranthus palmeri S. Watson) (Maroli et al. Reference Maroli, Nandula, Dayan, Duke, Gerard and Tharayil2015), to determine glyphosate-induced global physiological perturbations in glyphosate-resistant (Fernández-Escalada et al. Reference Fernández-Escalada, Gil-Monreal, Zabalza and Royuela2016, Reference Fernández-Escalada, Zulet-González, Gil-Monreal, Zabalza, Ravet, Gaines and Royuela2017) and glyphosate-tolerant (Maroli et al. Reference Maroli, Nandula, Duke, Gerard and Tharayil2017) weeds, and to examine herbicide metabolism in herbicide-resistant weeds (Wang et al. Reference Wang, Lin, Chiang and Wang2017). For determination of physiological perturbations, both Fernández-Escalada et al. (Reference Fernández-Escalada, Gil-Monreal, Zabalza and Royuela2016) and Maroli et al. (Reference Maroli, Nandula, Duke, Gerard and Tharayil2017) investigated the metabolic changes induced in the weeds following exposure to nonlethal doses of glyphosate.
Although MOAs of most herbicides have been well identified, in many cases the sequence of phytotoxic events that result in plant death is unclear, particularly for slow-acting herbicides, which exhibit a significant time lag between herbicide application and plant death. Using genetics and biochemical and metabolic analyses, Fernández-Escalada et al. (Reference Fernández-Escalada, Gil-Monreal, Zabalza and Royuela2016) studied the physiologies of a glyphosate-resistant and glyphosate-susceptible A. palmeri population and offered new insights into the physiological manifestations of the evolved glyphosate resistance. The authors indicated that aromatic amino acids do not have significant regulatory effects on EPSPS protein and suggested that a constant free amino acid pool including aromatic amino acids is a key parameter in complementing glyphosate resistance by EPSPS gene amplification. Similar observations were also reported earlier by Maroli et al. (Reference Maroli, Nandula, Dayan, Duke, Gerard and Tharayil2015). By means of metabolite profiling, Maroli et al. (Reference Maroli, Nandula, Dayan, Duke, Gerard and Tharayil2015) reported that in addition to EPSPS gene amplification, glyphosate resistance in a biotype of A. palmeri may also be complemented by elevated antioxidant capacity, with several metabolites having known antioxidant properties elevated in the resistant biotype compared with the susceptible biotype (Maroli et al. Reference Maroli, Nandula, Dayan, Duke, Gerard and Tharayil2015). Similarly, the study by Serra et al. (Reference Serra, Couée, Renault, Gouesbet and Sulmon2015) challenged L. perenne grass with a panel of different chemical stressors, including glyphosate and its degradation compound AMPA, at subtoxic levels. The authors concluded that all the subtoxic chemical stresses investigated induced discrete physiological perturbations and complex metabolic shifts via multilevel MOAs. Studies have thus reported that monitoring the perturbations induced in the metabolic-pool levels following herbicide exposure can therefore provide cues to the sequence of cellular phytotoxic events (Fernández-Escalada et al. Reference Fernández-Escalada, Gil-Monreal, Zabalza and Royuela2016; Maroli et al. Reference Maroli, Nandula, Dayan, Duke, Gerard and Tharayil2015, Reference Maroli, Nandula, Duke, Gerard and Tharayil2017; Serra et al. Reference Serra, Couée, Renault, Gouesbet and Sulmon2015; Vivancos et al. Reference Vivancos, Driscoll, Bulman, Ying, Emami, Treumann, Mauve, Noctor and Foyer2011).
Advances in nanotechnology have enabled the use of nanomaterials in agriculture (Fraceto et al Reference Fraceto, Grillo, de Medeiros, Scognamiglio, Rea and Bartolucci2016), with nanopesticides increasingly being looked at as alternates to chemical herbicides (Ali et al. Reference Ali, Nair, Kumar, Gopal, Srivastava and Siddiqi2017; Hayles et al. Reference Hayles, Johnson, Worthley and Losic2017; Tan et al. Reference Tan, Gao, Deng, Wang, Lee, Hernandez-Viezcas, Peralta-Videa and Gardea-Torresdey2018). Though they are reported to provide equal or better performance at lower doses compared with chemical herbicides (Parisi et al. Reference Parisi, Vigani and Rodríguez-Cerezo2015), their effects on crop plants are still poorly understood (Zhao et al. Reference Zhao, Hu, Huang, Fulton, Hannah-Bick, Adeleye and Keller2017a, Reference Zhao, Hu, Huang and Keller2017b, Reference Zhao, Huang, Adeleye and Keller2017c). A series of metabolomics and transcriptomics studies conducted to assess the metabolic response of crop plants such as cucumber (Cucumis sativus L.) (Zhao et al. Reference Zhao, Huang, Adeleye and Keller2017a), maize (Zhao et al. Reference Zhao, Hu, Huang and Keller2017b), and spinach (Spinacia oleracea L.) (Zhao et al. Reference Zhao, Hu, Huang, Fulton, Hannah-Bick, Adeleye and Keller2017c) to Cu(OH)2 nanopesticide exposure concluded that the nanopesticide induced significant alterations in the metabolite profiles of all the plants. In spinach, significant reductions in antioxidant- and defense-associated metabolites were reported, while in maize, Cu(OH)2 nanopesticide significantly decreased leaf chlorophyll content and biomass but induced an increase in the potassium and phosphorus levels and phenolic acid precursors. In contrast, foliar exposure of cucumber plants to a relatively lower dose of the nanopesticide induced activation and upregulation in mRNA levels of antioxidant and detoxification-related genes. Such studies bring into prominence the reliability of omics platforms to help us understand crop–environment interactions at a much finer level.
Metabolomics can robustly provide instantaneous information about metabolite concentrations by measuring the static metabolite-pool levels directly. However, as metabolic processes are interconnected and dynamic, with rapid turnover rates, characterization of metabolic networks requires quantitative knowledge of intracellular fluxes (Fernie and Morgan Reference Fernie and Morgan2013). Quantitation of metabolic fluxes through each reaction within a network can only be estimated indirectly with the help of isotopically labeled metabolic tracers (Gaudin et al. 2014; Gleixner et al Reference Gleixner, Scrimgeour, Schmidt and Viola1998; Sauer Reference Sauer2006). Fluxomics studies such as stable isotope–resolved metabolomics (SIRM) are emerging as powerful strategies used to measure fluxes in complex interconnected metabolic networks (Kikuchi et al. Reference Kikuchi, Shinozaki and Hirayama2004; Maroli et al. Reference Maroli, Nandula, Duke and Tharayil2016; Srivastava et al. Reference Srivastava, Kowalski, Callahan, Meikle and Creek2016). In weed science, only a couple of studies have used flux-based omics studies to examine competitive physiology (Maroli et al. Reference Maroli, Nandula, Duke and Tharayil2016; Miyagi et al. Reference Miyagi, Takahara, Kasajima, Takahashi, Kawai-Yamada and Uchimiya2011). SIRM experiments performed using stable isotope–labeled metabolic precursors (tracers) would be the most ideal approach to study metabolic fluxes in weeds. In these experiments, the growth media can be supplemented with labeled nutrients that can then be tracked throughout the metabolic network as part of endogenous metabolism (Gaudin et al. Reference Gaudin, Cerveau, Marnet, Bouchereau, Delavault, Simier and Pouvreau2014; Maroli et al. Reference Maroli, Nandula, Duke and Tharayil2016). Flux rates can then be indirectly estimated from metabolite changes and isotope distribution in a network. For example, accumulation of amino acids following glyphosate application is commonly observed in glyphosate-susceptible plants (Fernández-Escalada et al. Reference Fernández-Escalada, Gil-Monreal, Zabalza and Royuela2016; Maroli et al. Reference Maroli, Nandula, Dayan, Duke, Gerard and Tharayil2015; Vivancos et al. Reference Vivancos, Driscoll, Bulman, Ying, Emami, Treumann, Mauve, Noctor and Foyer2011). Independent studies conducted by Maroli et al. (Reference Maroli, Nandula, Dayan, Duke, Gerard and Tharayil2015) and Fernández-Escalada et al. (Reference Fernández-Escalada, Gil-Monreal, Zabalza and Royuela2016) have reported that glyphosate-susceptible A. palmeri biotypes accumulate higher concentrations of amino acids than resistant biotypes. It is generally accepted that the higher accumulation of amino acids following glyphosate treatment is due to proteolysis. However, using SIRM analysis, Maroli et al. (Reference Maroli, Nandula, Duke and Tharayil2016) essentially described the underlying cause of higher amino acid accumulation in the susceptible biotype. It was shown that glyphosate-induced amino acid accumulation in susceptible A. palmeri biotypes is a consequence of proteolysis (catabolism) coupled with de novo synthesis of certain amino acids. In contrast, amino acid concentrations in the glyphosate-resistant biotype were predominantly due to de novo synthesis (anabolism). Thus, it can be seen from this study that the use of modern omics platforms has helped to establish the connection between metabolome and metabolic pool dynamics to elucidate the link between the glyphosate MOA and de novo amino acid synthesis.
Integrated Omics Approaches to Understanding Phytotoxin MOA
Herbicides with new MOAs are desperately needed to combat evolved and evolving herbicide resistance (Duke and Heap Reference Duke and Heap2017), and no new commercial herbicides with a clearly new MOA have been commercialized since the 4-hydroxphenylpyruvate dioxygenase inhibitors in the 1980s (Duke Reference Duke2012). Thus, discovery of herbicides with new MOAs is of prime importance in herbicide discovery efforts. Evidence from the natural phytotoxin literature suggests that there are many more viable MOAs than the current 20 MOAs of commercial herbicides (Dayan and Duke Reference Dayan and Duke2014). However, determination of the MOA of phytotoxins is not a trivial pursuit, because what we observe after herbicide treatment of a plant is the manifestation of many secondary and tertiary effects resulting from an effect on the primary target site. The literature is full of papers confusing secondary and tertiary effects with primary effects. Many of the older herbicides were commercialized before their MOAs were known, partly because the target sites were not easy to determine, due to the difficulty in working back from physiological effects to a molecular target site.
With the advent of omics technologies, new strategies for MOA determination have been devised (Duke et al. Reference Duke, Bajsa and Pan2013; Grossmann et al. 2012a). Omics-based MOA discovery consists of building a database of any one of the different omics responses to herbicides with known MOAs and then comparing the response profile of a phytotoxin with an unknown MOA to profiles generated by phytotoxins with known MOAs. To our knowledge, this has been done in industry with only one omics method—metabolomics. This approach can be highly effective if the new compound happens to have an MOA that is in the database of omics responses to compounds with known MOAs. If not, the approach will indicate that the compound has a new MOA not represented in the database. Most companies involved in herbicide discovery have tried this approach, but only BASF has published a detailed description of how it has used omics methods to identify MOAs (Grossman et al. Reference Grossman, Chistiansen, Looser, Tresch, Hutzler and Ehrhardt2012a, Reference Grossmann, Hutzler, Tresch, Christiansen, Looser and Ehrhardt2012b). In that case, it combined both metabolomic and physionomic methods to build extensive databases of omics responses to phytotoxins with known MOAs against which to evaluate data from compounds of unknown MOAs. While a growing number of omics technologies are available to choose from, some of them being quite narrow (for example, lipidomics and glycomics), the scope of this segment will be limited to transcriptomics, proteomics, metabolomics, physionomics, and combined approaches.
Most MOA transcriptomics has been done with Arabidopsis. Transcription responses to several herbicides with known MOAs have been published (Table 2). However, a major problem with this method of determination is that at doses of the toxicant that have even a sublethal effect on the plant (for example, the dose that reduces growth by 50%), expression of many genes is affected within a short time after treatment. Many of the affected genes are those involved in stress responses and metabolic detoxification and other means of dealing with xenobiotics. For example, Baerson et al. (Reference Baerson, Sánchez-Moreiras, Pedrol-Bonjoch, Schulz, Kagan, Agarwal, Reigosa and Duke2005) found that the phytotoxic allelochemical benzoxazolin-2(3H)-one (BOA) upregulated many Arabidopsis genes involved in metabolism of xenobiotics and cell rescue and defense within 24 h after treatment. An extreme example is that of cantharidin, a potent natural phytotoxin that significantly affected gene expression of more than 6% of the genes of Arabidopsis within 2 h of treatment with a dose that reduced growth by 30% (Bajsa et al. Reference Bajsa, Pan and Duke2011a, Reference Bajsa, Pan and Duke2011b). Eventually, 10% of the genome was affected. This is not a surprise, as cantharidin and the herbicide chemical analogue endothall both inhibit all of the serine/threonine protein phosphatases (Arabidopsis has more than 20) in plants (Bajsa et al. Reference Bajsa, Pan and Duke2011a, Reference Bajsa, Pan, Dayan, Owens and Duke2012). These enzymes are heavily involved in signaling pathways and gene expression.
Proteomics has been used considerably less than transcriptomics to probe the MOAs or mechanisms of resistance to herbicides. Zhang and Reichers (Reference Zhang and Reichers2008) reviewed the use of proteomics in weed science research. The effects of paraquat, diuron, and norflurazon on Chlamydomonas reinhardtii were studied with proteomics (Nestler et al. Reference Nestler, Groh, Schönenberger, Eggen and Suter2012). Although the abundance of the target protein of norflurazon, phytoene desaturase, was unaffected, the amounts of other enzymes of the plastidic terpene pathway were affected. Diuron increased the amount of its target, the D1 protein of photosystem II, whereas some other proteins involved in photosynthetic electron transport decreased. The effects of the auxinic herbicides dicamba and clopyralid on the proteome of soybean [Glycine max (L.) Merr.] were examined by Kelley et al. (2006). They found four proteins to be strongly affected, and one of them was the product of the GH3 gene, a gene that they found to be strongly upregulated at the transcriptional level. Kumari et al. (Reference Kumari, Narayan and Rai2009) found that butachlor reduced levels of proteins involved in photosynthesis and respiration of the alga Aulosira fertilissima. Because the MOA of butachlor is inhibition of very long chain lipid synthesis, these effects are secondary or tertiary. Likewise, amiprophos-methyl, a herbicide that affects microtubule function, had effects on proteins associated with diverse physiological and biochemical processes but not directly associated with tubulin (Wang et al. Reference Wang, Li, Zhao and Peng2011). More recently, the natural phytotoxin α-terthienyl was found to affect 16 proteins associated with energy transduction, of which the transketolase protein was greatly reduced (Zhao et al. Reference Zhao, Huo, Liu, Zhang and Dong2018). A transketolase-altered mutant was less sensitive to the phytotoxin, and the enzyme from the mutant was less inhibited by the compound. But the weak effect of the toxin on the enzyme is not what one would expect for a primary target site.
Studies using natural phytotoxins with unknown target sites have revealed distinct metabolic effects but no clear indication of a molecular target (Cantrell et al. Reference Cantrell, Duke, Fronczek, Osbrink, Mamonov, Vassilyev, Wedge and Dayan2007; Duke et al. Reference Duke, Evidente, Fiore, Rimando, Dayan, Vurro, Christiansen, Looser, Hutzler and Grossmann2011). Other metabolomic studies of phytotoxin MOAs are discussed in Duke et al. (Reference Duke, Bajsa and Pan2013). One of the more complete studies of this type was that of Trenkamp et al. (Reference Trenkamp, Eckes, Busch and Fernie2009), who examined the effects of glufosinate, glyphosate, sulcotrione, foramsulfuron, benfuresate, and an experimental herbicide on the metabolome of Arabidopsis. Results matched the MOA for some but not all of the phytotoxins. More systematic approaches that rely on metabolic profiles of an array of phytotoxin MOAs have been more successful (Grossmann et al. Reference Grossmann, Niggeweg, Christiansen, Looser and Ehrhardt2010, 2012a, Reference Grossmann, Hutzler, Tresch, Christiansen, Looser and Ehrhardt2012b). Perhaps the only new phytotoxin MOAs discovered by omics methods are the determination that cinmethylin’s target site is tyrosine amino-transferase (Grossmann et al. Reference Grossmann, Hutzler, Tresch, Christiansen, Looser and Ehrhardt2012b) and that of a phenylalanine analogue (PHE1) is IAA synthesis (Grossmann et al. 2012a), although in the latter case the specific enzyme target to the IAA synthesis pathway was not determined. In both cases, physionomic and metabolomic databases were used to narrow the search for the target sites. Verification of the omics indications were followed by physiological and biochemical studies.
Limitations, Conclusions, and Future Directions
Relying on orthologous genomes to annotate related genomes of weedy species has pitfalls associated with proposing biological interactions and processes based on spurious assumptions that homologous genes have conserved functionality across species (Doğramacı et al. Reference Doğramacı, Horvath and Anderson2015). Similarly, assembling a quality de novo reference transcriptome for weeds can be computationally difficult, due to complex gene families and high levels of heterozygosity that often occur in weeds. Polyploidy further complicates reference transcriptome assembly, although the assembly can be completed and yield insight into evolution of polyploidy in weeds (Chen et al. Reference Chen, McElroy, Dane and Goertzen2016). This becomes more critical when studying herbicide resistance, because RNA-Seq will detect all differences in gene expression and sequence; therefore, using highly unrelated resistant and susceptible populations will result in a large number of false positives (genes with significant DE that are completely unrelated to herbicide resistance). Hence, not all resistance mechanisms can be detected using RNA-Seq. Therefore, for a successful RNA-Seq experiment in non-model species such as weeds, ideally, a high-quality reference transcriptome is desired for identifying and quantifying DE genes or sequence variations. Additionally, the quality of the de novo reference transcriptome is also important, as genes will only be identified for DE and/or sequence variation if they are present in the reference assembly. In contrast, the major challenge for developing an effective high-throughput metabolomics platform lies in the chemical complexity, heterogeneity, and dynamic range of the metabolites and the challenges in developing a single extraction procedure for all metabolites. Plant extracts have a complicated biochemical composition and require extensive extraction and separation procedures to achieve reproducible results. Furthermore, very few of these metabolites can act as distinct biomarkers for a particular herbicide or phytotoxin MOA. Exceptions are EPSPS, PPO, and ceramide synthase, which cause dramatic increases in the pools of shikimic acid (Duke et al. Reference Duke, Baerson and Rimando2003), protoporphyrin IX (Dayan and Duke Reference Dayan and Duke2003), and sphingoid bases (Abbas et al. Reference Abbas, Duke, Sheir and Duke2002), respectively. Unfortunately, most other metabolites or phytotoxins do not have such dramatic effects.
As no single omics method is likely to reveal the MOA of a herbicide or natural phytotoxin, omics approaches to probe MOAs, though powerful, have to be used with caution. Several factors can influence the outcome of an omics experiment. First, physiological effects and responses are dose dependent, such that at high doses, results might be confounded by secondary targets, while at low doses, a plant might compensate too rapidly to observe anything meaningful. Second, the results are highly dependent on exposure time, wherein responses can be rapid, gradual, or delayed or sometimes may even reverse over time. Third, omics responses can vary between plant tissues and cell types, such that important effects in some cells could be masked when the entire tissue or organ is extracted. Finally, metabolic-pool sizes can be deceiving, as the pool size is determined by both input and output of the pool. In many cases, changes in pool fluxes would be much more informative about the effect of a herbicide than the pool size. Moreover, even when omics methods suggest a molecular target site, it must be verified by physiological and biochemical methods. For example, histone deacetylase was found to be the target site of a phytotoxic metabolic product of BOA, and its MOA was further probed by transcriptome analysis (Venturelli et al. Reference Venturelli, Belz, Kämper, Berger, von Horn, Wegner, Böcker, Zabulon, Lagenecker, Kohlbacher, Bameche, Weigel, Lauer, Bitzer and Becker2015). It is evident that the transcriptome data would have been very unlikely to reveal the molecular target site.
Another caveat involving omics studies is that all herbicides and phytotoxins cause stress, including oxidative stress, so omics methods can be misleading to the naïve researcher. For example, Ahsan et al. (Reference Ahsan, Lee, Lee, Alam, Lee, Bahk and Lee2008) found both paraquat and glyphosate to enhance the amount of proteins involved in defense against oxidative stress in leaves of glyphosate-susceptible rice (Oryza sativa L.). They concluded that this was an “alternative” effect, rather than a secondary or tertiary effect of herbicide-induced stress. Clearly, the approximately 50-fold level of resistance of crops made resistant to glyphosate by means of a glyphosate-resistant EPSPS (Nandula et al. Reference Nandula, Reddy, Rimando, Duke and Poston2007) is proof that there is no alternate primary effect of glyphosate. Comparing results with different omics approaches is rare, but quite different effects have been reported with different omics approaches. For example, in the same experiment in which cantharidin’s effects on the transcriptome were determined (Bajsa et al. Reference Bajsa, Pan and Duke2011a, Reference Bajsa, Pan and Duke2011b), samples were taken for proteome studies (Bajsa et al. Reference Bajsa, Pan and Duke2015). A remarkable lack of correlation between transcriptome and proteome results was observed, although the lack of correspondence between transcriptome and proteome data could be due to multiple factors (Duke et al. Reference Duke, Bajsa and Pan2013; Narayanan and Van de Ven Reference Narayanan and Van de Ven2014; Payne Reference Payne2015). Similarly, Zhao et al. (Reference Zhao, Huo, Liu, Zhang and Dong2018) found decreases in the transketolase protein of Arabidopsis treated with α-terthienyl, but the gene for this enzyme was upregulated by the same treatment. They hypothesized that the decrease in protein was due to direct interaction with α-terthienyl, which resulted in upregulation of the gene to compensate.
The disconnect between comparing individual omics platforms to understand weed genetics, diversity, heterozygosity, and importantly, evolution of herbicide resistance in weeds, especially non–target site resistance, highlights the need to develop an integrated omics platform. As an example of developing blueprints for constructing low-cost genomic assemblies in weed species, Horvath et al. (Reference Horvath, Patel, Doğramacı, Chao, Anderson, Foley, Scheffler, Lazo, Dorn, Yan and Childers2018) have sequenced gene space and transcriptome assemblies of E. esula that were used to identify promoter sequences, high-quality markers, and repetitive elements. Based on this framework, a reliable sequence for >90% of the expressed E. esula protein-coding genes was made available. Compared with conventional screening techniques, developing herbicides with new MOAs and chemistries or evaluating natural products for use as bioherbicides can be achieved at a much faster rate using next-generation omics. Despite requiring a cautionary approach, integrated systems biology can revolutionize weed management practices by providing hitherto unknown biological information (Han et al. Reference Han, Vila-Aiub, Jalaludin, Yu and Powles2017; Kraehmer Reference Kraehmer2012). A holistic line of action with multidisciplinary integrated approaches and collaboration between weed scientist, extension specialist, and farmers is required to allow for the development of long-term, weed management strategies. Though information on candidate genes is lacking for most weed species, global gene expression profiling techniques, such as microarrays, can serve as effective tools for understanding NTSR mechanisms (Peng et al. Reference Peng, Abercrombie, Yuan, Riggins, Sammons, Tranel and Stewart2010), while RNA-Seq and whole-metabolome profiling can identify genes and metabolites involved in regulating biochemical processes in a weed. An outcome of a systems biology approach is the ambitious RNAi technology (BioDirectTM) developed by Monsanto to exploit precise RNA segments coding for EPSPS protein in reversing glyphosate resistance in weeds (Hollomon Reference Hollomon2012; Shaner and Beckie Reference Shaner and Beckie2014). In conclusion, it can be said that genomics, transcriptomics, and other methods for high-throughput screening can yield promising results for elucidating basic weed biology concepts as well as insights into the response of weeds to biotic and abiotic stresses and crop–weed competition. Thus, with the aid of these omics platforms, improved knowledge of weed biology, genetics, and physiology can be gained quickly, paving the way for the development of long-term, sustainable weed management practices.
Acknowledgements
The authors would like to thank the WSSA for providing the funding and platform to organize this symposium at the 55th Annual Meeting of the Weed Science Society of America, Lexington, KY. The authors would also like to acknowledge Amy Lawton-Rauh and Patrick Tranel for their contributions to the symposium. No conflicts of interest have been declared.