Introduction
The year 2050 is a landmark date as perceived by government, industry, and the media. Around that time, the world’s human population is expected to peak at 9 billion, straining global capacity to provide sufficient energy, freshwater, and food (Figure 1A) (Alexandratos and Bruinsma Reference Alexandratos and Bruinsma2012). Current crop production levels are not adequate to feed the projected population, and meeting this anticipated demand is viewed as a major challenge for humanity. The burden of meeting these needs will be exacerbated by climate change, loss of water resources, and reductions in arable land due to multiple causes. Weed management is essential for agricultural production and management of landscapes and the environment and will play an important role in determining whether we meet future food production requirements.

Figure 1 Trends discussed in this article. (A) The growing world human population and the agricultural production needed to meet growing demand. Data and projections based on World Bank estimates. (B) Rise in herbicide-resistant weed biotypes and leveling off of new herbicide sites of action discovered. Historical data based on Heap (2017). Projections of herbicide resistance assume continued patterns of herbicide use, but eventual leveling off is expected due to reduced use of some herbicides and saturation of resistance in weed populations. Projection of new herbicide sites of action assumes breakthroughs leading to four new chemistries over the next 35 yr. (C) Broad trends in computational power, communication networking, and biological manipulation. Lines are representative of rising trajectories, but otherwise only intended to promote discussion within the context of the article. Gray vertical line indicates the present year in all graphs.
This article is the result of a symposium held at the 2016 WSSA conference in San Juan, Puerto Rico. The objective of the symposium was to consider the long-term future of weed control and the knowledge needed to frame a sustainable system for weed management. Symposium speakers were asked to extrapolate from current and emerging technologies to imagine what weed management must look like in 2050 if agriculture is to realize the yield increases required to sustain the world’s future population. Weeds are a persistent problem, and the continuing rise in numbers of herbicide-resistant biotypes reinforces the lesson that weed control technology must constantly advance to stay ahead of weed evolution and adaptation. Fortunately, the rapid pace of technological advancement and new breakthroughs in the life sciences offer the potential for new and improved methods of weed management.
The 32 yr until 2050 provide a relatively long time frame in which many things are possible, some of which we can imagine and many we cannot. This is both an opportunity and a challenge. Taking the optimistic viewpoint that innovation in crop genetics will be sufficient to meet the yield requirements of a world population of around 9 billion, the challenge of weed control will remain indefinitely. Weeds will continue to evolve and persist, and we should take this as a challenge to find truly sustainable solutions to weed management.
Recent reviews that look to the future of weed management provide excellent summaries of current strengths of, gaps in, and needs of weed science (e.g., Bajwa et al. Reference Bajwa, Mahajan and Chauhan2017; Shaner and Beckie Reference Shaner and Beckie2014). Various authors have advocated improved framing of research questions to reveal fundamental principles of weed ecology and evolution (Justine et al. Reference Justine, Erik, Paul, Santiago and Bruce2012; Ward et al. Reference Ward, Cousens, Bagavathiannan, Barney, Beckie, Busi, Davis, Dukes, Forcella, Freckleton, Gallandt, Hall, Jasieniuk, Lawton-Rauh, Lehnhoff, Liebman, Maxwell, Mesgaran, Murray, Neve, Nuñez, Pauchard, Queenborough and Webber2014), increasing the use of integrated weed management approaches (Mortensen et al. Reference Mortensen, Egan, Maxwell, Ryan and Smith2012), better integrating basic knowledge with applied weed science (Moss Reference Moss2008), and/or seizing on new research opportunities (Fernandez-Quintanilla et al. Reference Fernandez-Quintanilla, Quadranti, Kudsk and BÀRberi2008). We generally agree with these analyses and recommendations, but here we offer a different perspective to project how weed control will be conducted in the relatively distant future.
This exercise is intended to focus on weed management technologies that may change substantially over the next decades. Although we do not directly address weed biology, we expect the same advances in science and information processing that contribute to new weed management will also foster a revolution in understanding plant growth and development, responses to stress, and environmental interactions. Indeed, understanding of weed biology and ecology is integral to sustainable weed management, as weed populations adapt and evolve in response to new selective pressures. A priority on funding and training in these areas is reflected in our recommendations.
What Herbicides Will We Be Using in 2050?
Traditional Herbicide Chemistries
From the early 1950s until the early 1980s, a new herbicide mechanism of action (MOA) was commercialized every 2.5 to 3 yr (Figure 1B) (Duke Reference Duke2012). However, no new MOAs have been introduced since the 1980s. And although one major company has stated that they are developing a broad-spectrum herbicide with a new MOA (Bomgardner Reference Bomgardner2016), the relentless linear increase in weeds with evolved herbicide resistance since the mid-1970s (Heap Reference Heap2017) has created a great need for herbicides with new MOAs. Even new herbicides with old MOAs would be welcome (assuming they have no cross-resistance to cases of evolved resistance), but there has even been a decrease in the introduction of new herbicides with old MOAs (Jeschke Reference Jeschke2016). The lack of any new MOAs since the 1980s has been surprising, as normal diminishing returns would suggest discovery of at least a few new MOAs. The increase in evolved herbicide resistance, coupled with the lack of new MOAs threatens to make almost all existing herbicides unusable by 2050.
The lack of new MOAs and slowing of the herbicide discovery pipeline is probably due to several factors, including drastic consolidations of the pesticide industry, a substantial devaluation of the non-glyphosate herbicide market after glyphosate-resistant crops were introduced, more stringent regulatory requirements for new products (much greater cost to get a new product to market), and diminishing returns of discovery approaches. Use of combinatorial chemistry to inexpensively produce vast numbers of compounds for testing in high-throughput evaluations has not broken the bottleneck. In general, new herbicides with old MOAs and new transgenic crops that are resistant to old herbicides (e.g., 2,4-D and dicamba) are only short-term solutions to some existing weed problems, because resistance already exists to these herbicides.
Use of negative cross-resistance, the enhanced sensitivity to a herbicide from the herbicide class to which resistance has evolved, has the potential to prolong the use of some of our old herbicides. An example of negative cross-resistance is the mutation in a hydrilla [Hydrilla verticillata (L. f.) Royle] phytoene desaturase (PDS) gene (Arg-304 conversion to Thr) that provides 52-fold resistance to norflurazon but renders the mutant PDS 5-fold more sensitive to diflufenican (Arias et al. Reference Arias, Dayan, Michel, Howell and Scheffler2006). There are other examples of negative cross-resistance with herbicides with other MOAs such as photosystem II inhibitors (Fuerst et al. Reference Fuerst, Arntzen, Pfister and Penner1986). Thus, the herbicide to which there is negative cross-resistance can be either mixed or alternated with the herbicide to which there is resistance to prevent and/or remove mutant plants. This strategy has not yet been used, but it could be helpful in the future to prolong the use of older herbicides.
New Herbicide Targets and Biopesticides
The future of chemical control depends on the discovery of herbicides with new MOAs, but this raises the question of whether novel good herbicide target sites even exist. First, not every enzyme in a metabolic pathway is a good herbicide target. For example, even though 5-enolpyruvylshikimate-3-phosphate synthase and acetolactate synthase of the shikimate and branched-chain amino acid pathways, respectively, are excellent herbicide targets, good inhibitors of other enzymes of these pathways are too weakly herbicidal to be commercialized. A particular enzyme of a pathway may not be a good herbicide target if too much of it must be inhibited to cause plant death, if it is a relatively high abundance protein, or if there is more than one enzymatic path to the product. Thus, only a small fraction of potential herbicide targets are viable.
There are more than the 25 or so commercial herbicide target sites that are currently used (WSSA 2017), and research with natural phytotoxins has demonstrated several additional novel potential herbicide target sites (Dayan and Duke Reference Dayan and Duke2014; Evans-Roberts et al. Reference Evans-Roberts, Mitchenall, Wall, Leroux, Mylne and Maxwell2016; Venturelli et al. Reference Venturelli, Belz, Kamper, Berger, von Horn, Wegner, Bocker, Zabulon, Langenecker, Kohlbacher, Barneche, Weigel, Lauer, Bitzer and Becker2015). But, in most of these cases, the natural herbicide is too expensive, too toxic, or lacking appropriate physicochemical properties (e.g., uptake and translocation) to be a good herbicide. Nevertheless, these compounds can point the way to discovery of new target sites (i.e., new MOAs) that can be the focus of in vitro screening of less expensive and/or toxic compounds with better physicochemical properties.
One promising direction is the discovery and development of novel herbicides based on natural products that are by-products of microorganisms or extracts of plants. Only a small fraction of the world’s microbial and plant biodiversity has been screened for herbicidal activity. We know that interesting herbicidal compounds with novel mechanisms of action have been discovered, and some compounds have been very successful commercial herbicides (e.g., glufosinate based on phosphinothricin, a breakdown product of bialaphos discovered from Streptomyces viridochromogenes and S. hygroscopicus by researchers in Japan). One company, Marrone Bio Innovations, has screened water extracts from approximately 15,000 microorganisms (bacteria, fungi, and actinomycetes) and 350 plant extracts and found several novel herbicidal compounds with novel modes of action (P Marrone, personal communication). Some microbial strains and a plant extract are in development at Marrone Bio Innovations and show promise as cost-effective commercial herbicides, but based on success rates, it is more technically challenging to develop a cost-effective and broad-spectrum bioherbicide than a biofungicide, bioinsecticide, or bionematicide. In the 1980s and 1990s many companies had natural product-screening discovery efforts, but the advent of glyphosate-resistant crops led to the dismantling of these operations. Today’s molecular and other tools allow much more targeted and informed screening through genomics and metabolomics.
RNA Interference Herbicides and Genetic Engineering
A potential new technology is the use of RNA to silence key weed genes through the process of RNA interference (RNAi), leading to either enhanced weed susceptibility to herbicides or outright death of the weed. Applied as a spray, RNA has great potential for weed management, because sequences can be designed to selectively target a specific weed species or a group of related weed species. Presumably, targets of current herbicide chemistries could be inhibited, but there would be no cross-resistance with traditional herbicides, because RNAi works through a different mechanism. Potential new targets could also be identified.
Hurdles blocking the commercial implementation of RNAi herbicides include technical problems such as formulating RNA to achieve efficient uptake into the target plant as a sprayed product. Another challenge is the development of methods for economical large-scale production of RNAs, although the cost of this is being dramatically reduced. In addition, it is not known how fast weeds will develop resistance to RNAi herbicides. From a regulatory perspective, it is not clear how long it would take to register a new herbicide based on this technology, and the opposition from consumer groups is uncertain. Unfortunately, there are no peer-reviewed research articles on the topic of sprayable RNAi for weed management, so we only have conference reports from one major company indicating that substantial effort is being put into developing this technology (Sammons et al. Reference Sammons, Navarro, Croon, Schmuke, Wang, Rana, Griffith and Godara2015).
Other novel approaches to developing herbicides could be enabled by genetic engineering. For example, phosphite was proposed as an herbicide many years ago, but toxicity to crops was an issue (e.g., Rothbaum and Baillie Reference Rothbaum and Baillie1964). Engineering phosphite metabolism into crops so that they can transform it to phosphate can both eliminate the need to use phosphate fertilizers and kill weeds (López-Arredondo and Herrera-Estrella Reference López-Arredondo and Herrera-Estrella2012). Furthermore, phosphite is both toxic to some plant pathogens and induces plant defense systems against pathogens (Pinto et al. Reference Pinto, do Nascimento, Gomes, da Silva and Miranda2012; Saindrenan et al. Reference Saindrenan, Barchietto and Bompeix1988). The continuing decline of viable herbicides caused by increases in weed resistance will make such innovative approaches more attractive.
A revolutionary approach to suppressing pest populations is the use of “gene-drive” technology (Leftwich et al. Reference Leftwich, Bolton and Chapman2016). Although mechanisms vary, the concept involves a genetic element that is passed on to progeny at a rate greater than the 50% expected from Mendelian inheritance. While the phenomenon has been known for years, the emergence of the CRISPR/Cas9 (clustered regularly interspaced short palindromic repeats/CRISPR-associated protein 9) genetic editing system provides a simple, powerful tool for generating a gene drive (National Academies of Sciences, Engineering, and Medicine [NASEM] 2016). CRISPR/Cas9 is efficient in inserting targeted mutations in both alleles of an individual, resulting in a conversion from the heterozygous to the homozygous condition and transmission of a specific gene to nearly all progeny. This holds great promise, as the release of organisms containing a gene drive could—in just several generations—result in the replacement of a given gene with a version designed by humans. Perhaps the simplest and most compelling example of this is a proposal to eliminate malaria by releasing mosquitoes that carry a gene-drive version of a gene that compromises the mosquito’s ability to serve as a vector of the Plasmodium parasite (Hammond et al. Reference Hammond, Galizi, Kyrou, Simoni, Siniscalchi, Katsanos, Gribble, Baker, Marois, Russell, Burt, Windbichler, Crisanti and Nolan2016). Similar ideas could be used to suppress weed populations, for example, by rendering herbicide-resistant populations of Palmer amaranth (Amaranthus palmeri S. Wats.) to be once again susceptible to glyphosate, or even directly degrading the competitive advantage of an invasive species (NASEM 2016). Whether this will work or not depends on many factors, such as the reproductive system of the target weed, its longevity, seed persistence, and fitness of the introduced trait.
Gene-drive technology raises many issues related to ethics and potential unintended consequences, but it represents a bold new tool with potential for managing ecosystems. Concerns surrounding its deployment might prevent this approach from ever being used on plants in the field. The technology is young, and additional research and development of risk mitigation strategies could lead to public acceptance. In any case, it exemplifies the type of breakthrough that could fundamentally change weed management in coming decades.
Precision Agriculture and Robotics
The Need for Precision
Weed management in specialty crops will change significantly by 2050. In addition to the trends in herbicide discovery and resistance described earlier, weed management in high-value specialty crops like vegetables, flowers, and herbs faces specific problems, such as the reluctance of herbicide registrants to list specialty crops on herbicide labels due to financial liabilities, as well as farm labor shortages and other factors that have led to increases in the cost of hand weeding (Duke Reference Duke2012; Fennimore and Doohan Reference Fennimore and Doohan2008; Taylor et al. Reference Taylor, Charlton and Yunez-Naude2012). These trends will likely persist into the future, as it is unlikely that the cost of herbicide development will decline, that pesticide manufacturers will be more willing to accept potential liability from herbicide injury to specialty crops, or that farm labor costs will decrease. Another factor moving the market away from traditional herbicides is the increasing demand for organic food (USDA-ERS 2015). Conditions are optimal to realize dramatic improvements in robotics, machine vision, crop/weed detection (i.e., weed control automation), and solar power (efficiency, payload weight, battery life) that may form the leading edge of a technological revolution in broader weed management over the next 32 yr.
An example of how the combination of scarce labor availability and inadequate herbicide availability has led to innovation is the development of automated lettuce thinners and intelligent cultivators. The majority of lettuce in Arizona and California is direct seeded 4 to 7 cm apart in rows on raised beds 1- or 2-m wide with multiple rows per bed. Traditionally, laborers with hoes have thinned lettuce to 22- to 30-cm spacing between lettuce plants at a cost of approximately $444 ha−1 for both thinning and hand weeding (Fennimore et al. Reference Fennimore, Smith, Tourte, LeStrange and Rachuy2014). However, thinning requires timely operations, and when laborers are in short supply or unavailable, alternative methods are needed for lettuce thinning. Therefore, small engineering companies have stepped in to fill the void left by the labor shortage. The automated lettuce thinners and intelligent cultivators use a camera for machine vision, positional monitoring, and an actuator that consists of a cultivator knife or solenoid-activated spray nozzle. The machine-vision cameras feed information into a processor that uses an algorithm to detect the crop row (pattern recognition) and spacing between the crop plants in the row. Mosqueda et al. (Reference Mosqueda, Smith, Goorahoo and Shrestha2017) evaluated a lettuce thinner in five commercial fields. They found that both the automated thinner and hand thinning resulted in the same 26-cm spacing between lettuce plants, but the automated thinner had a standard deviation of 3.8 cm compared with 4.5 cm for hand thinning. The process of hand thinning and hand weeding took 29.3 hha−1, while machine thinning plus hand weeding reduced the time by about 36% (Smith Reference Smith2015).
Co-robotics, the Symbiosis among Man, Machine, and Crop Plants
To develop integrated approaches for commercial-scale weed control strategies, technological solutions including mechatronics (the combination of electronics and mechanical engineering), machine learning, and autonomous machines will play an important role. Technology is no stranger to weed control. Early research on the development of intelligent machines for the automation of on-farm cultural practices at a commercial scale began in the 1960s with work at the University of California–Davis and in the United Kingdom on automated machines for thinning of sugar beets (Beta vulgaris L.). Over the past six decades, engineers have been working on new and improved technologies that build upon these early visions. For example, in the 1990s, research at UC Davis successfully designed, developed, and demonstrated intelligent, precision pulsed–jet spray technology in which a robotic system can apply a micro-dose of herbicide at the individual leaf (1 cm) scale to weeds in a field from a mobile platform (Downey et al. Reference Downey, Giles and Slaughter2004; Giles et al. Reference Giles, Downey, Slaughter, Brevis-Acuna and Lanini2004; Lamm et al. Reference Lamm, Slaughter and Giles2002; Zhang et al. Reference Zhang, Staab, Slaughter, Giles and Downey2012b).
Advanced smart-machine technology for automatic weed control has been demonstrated in certain row crops when weed density is low; however, the proportion of weeds controlled automatically is still well below 100%, and commercial-scale applicability to all crops, weed species, and growing conditions has yet to be demonstrated (e.g., Fennimore et al. Reference Fennimore, Smith, Tourte, LeStrange and Rachuy2014). Currently, the most critical technological bottleneck preventing the realization of fully automatic weed control machines is the lack of a robust weed sensor that can detect and distinguish among closely related crop and weed species at rates well above 95% in the field environment. When isolated as images of individual leaves, advanced software algorithms for species identification have been developed (e.g., Leafsnap Reference Leafsnap2016). However, Hearn (Reference Hearn2009) demonstrated that even when advanced leaf shape recognition algorithms were employed, only 72% of 151 different species were successfully recognized by automated machine-vision methods. In a traditional machine-vision sensing approach using leaf- or plant shape–based feature recognition, high weed levels are problematic due to the visual occlusion caused by the intermingling of weed and crop foliage (Franz et al. Reference Franz, Gebhardt and Unklesbay1991). While some commercial success has been demonstrated at early growth stages when weed densities are low and crop plants are readily distinguished from weeds by plant size and planting pattern, new approaches are needed to identify weeds under moderate to heavy weed levels and when plant size is not a reliable means of weed detection. In the immediate term, the two most promising sensing technologies for automated weed control systems are hyperspectral imaging and a systems approach based upon a crop-mapping sensor used at planting.
Hyperspectral imaging methods for weed detection are more robust under high weed densities than shape-based methods, because the method measures the reflectance spectra at each point in the image regardless of the visibility of the entire plant or distinct leaf shape. The species identity is then determined for each point by spectral feature recognition rather than by shape analysis (e.g., Slaughter et al. Reference Slaughter, Lanini and Giles2004, Reference Slaughter, Giles, Fennimore and Smith2008; Zhang and Slaughter Reference Zhang and Slaughter2011b; Zhang et al. Reference Zhang, Staab, Slaughter, Giles and Downey2012b). In addition to the method’s robustness to partial leaf occlusion, Zhang and Slaughter (Reference Zhang and Slaughter2011a) observed that the technique could reliably distinguish between closely related species (e.g., domestic tomato [Solanum lycopersicum L.] and black nightshade [Solanum nigrum L.]), indicating a potential to overcome the challenge observed by Hearn (Reference Hearn2009) in distinguishing between species with similar leaf shapes. The hyperweeding method is subject to the genotype by environment interaction effect on the spectral reflectance of each species (Zhang et al. Reference Zhang, Slaughter and Staab2012a). To become a commercial success, equipment manufacturers will need to develop advanced machine-learning methods that characterize the spectral reflectance features of important crop and weed species over a wide range of growing environments. Zhang et al. (Reference Zhang, Slaughter and Staab2012a) demonstrated that a machine-learning approach using a two-stage expert system was robust to the genotype by environment interaction effect by first employing an “environment expert” that mined expert knowledge from historical data to identify the best environmental match in prior growing seasons to the current season. Then, as a second step, it employed a “species expert” that would classify unknown plants by species using historical data from the most similar growing environment in the database to accurately classify the crop and weed plants.
A systems approach is another promising technique that could be implemented commercially in the short term to develop smart machines for automated weed control. In the systems approach concept, knowledge of the crop-plant locations at planting is mapped and retained for future use in crop-plant care tasks such as weed control. A weeding robot can then access the crop-plant map in real time later in the season, once weeds emerge, to distinguish crop plants from weeds based upon location. Several researchers have demonstrated the feasibility of mapping the location of crop plants in real time during planting using centimeter-accuracy real-time kinematics global positioning system (GPS) (e.g., Ehsani et al. Reference Ehsani, Upadhyaya and Mattson2004; Griepentrog et al. Reference Griepentrog, Nørremark, Nielsen and Blackmore2005; Pérez-Ruiz et al. Reference Pérez-Ruiz, Slaughter, Gliever and Upadhyaya2012a; Sun et al. Reference Sun, Slaughter, Ruiz, Gliever, Upadhyaya and Smith2010). An automated GPS weeding system was developed by Pérez-Ruiz et al. (Reference Pérez-Ruiz, Slaughter, Gliever and Upadhyaya2012b) that demonstrated the feasibility of accessing the GPS crop-plant location map in real time and using that knowledge to control the path of a pair of miniature robotic hoes to automatically kill weeds growing between crop plants in the row. An advantage of this technique is that it does not require knowledge of any crop or weed genotype by environment interaction affects and is readily adapted across a wide range of crop species and growing conditions. Another advantage is that physically robust centimeter-accuracy GPS equipment is already in commercial use in agriculture, and the cost of this type of technology is likely to decrease while its accuracy increases with future development and adoption.
Beginning of the Robotic Weeding Era
Mechatronics and automation technologies are likely to become more effective and commercially viable as future weed control strategies and are already being used in industrialized countries in specific crops (King Reference King2017). Typically, vegetable crops like broccoli (Brassica oleracea L. var. botrytis L.) , cabbage (Brassica oleracea L.), field-grown flowers, herbs, lettuce (Lactuca sativa L.), onion (Allium cepa L.), and tomato among others are hand weeded to achieve intrarow weed control (Fennimore et al. Reference Fennimore, Smith, Tourte, LeStrange and Rachuy2014; Melander et al. Reference Melander, Lattanzi and Pannacci2015). Industry has responded to the need for automation of intrarow cultivators. As an example, the Robovator intrarow cultivator from Denmark is equipped with two flat-blade tines per crop row that undercut weeds at 1 to 2 cm below the soil surface. The tines are positioned in the intrarow area until they approach a crop plant, at which point the computer system opens the tines to safely pass by the crop, then closes them again on the following side (Melander et al. Reference Melander, Lattanzi and Pannacci2015). The Robovator system is designed to detect the difference between the crop plant and weed based on the recognition of the crop row (as described earlier) and the size difference between the crop and weed. In the Robovator, each row has a camera, and images from the cameras are processed to determine the position of the crop, and then the computer signals the actuator to open and close at the proper location (Lati et al. Reference Lati, Siemens, Rachuy and Fennimore2016; Melander et al. Reference Melander, Lattanzi and Pannacci2015). In broccoli and lettuce, the Robovator reduced hand-weeding time by 39% and 27%, respectively, compared with the standard cultivator (Lati et al. Reference Lati, Siemens, Rachuy and Fennimore2016). Another exciting approach is being developed by Blue River Technology (http://www.bluerivert.com), a robotics company spin out from Stanford University. They already market the LettuceBot, a machine for precision thinning of lettuce, and are now releasing a technology called “See & Spray” for precision application of herbicides for weed control in cotton. Their approach uses extensive collections of plant images that enable their tractor-mounted unit to differentiate crop plants and weeds. They state that this “does not rely on spacing or color to identify weeds” to detect differences between plants. An advantage of this approach is the potential reduction in volumes of herbicides applied, especially at low weed densities, where the differences in herbicide use between precision spraying and broadcast applications can be dramatic.
Automatic weed removal technology provides a path to alternative weed control tools that is much more promising, at least for specialty crops, than the traditional herbicide development model that has dominated for the past 60 yr. There are fewer than 10 companies in the world that have the capacity to discover, develop, and register herbicides (Jeschke Reference Jeschke2016). In contrast, there are many more companies with expertise in robotics that can build automatic weed removal equipment, and development of robotic weeders is much less expensive than development of herbicides. So far, automated lettuce thinners have relied upon control of automated control of spray nozzles to precisely apply herbicides, acids, or fertilizer solutions. Automated intrarow cultivators have relied upon modified hoe blades controlled by processors (Melander et al. Reference Melander, Lattanzi and Pannacci2015). However, there are many more weed control technologies to be explored for automation, such as lasers, sequential flaming, or spraying abrasives (Erazo-Barradas et al. Reference Erazo-Barradas, Friedrichsen, Forcella, Humburg and Clay2017; Forcella Reference Forcella2009, Reference Forcella2012, Reference Forcella2013; Nørremark et al. Reference Nørremark, Swain and Melander2009). The power of automated weed control is the merger of traditional weed control technology with robotics. When automation technology, weed detection, and actuation are combined with a weed control device, the result is creation of a different and more effective tool.
Harnessing Biology for Weed Management
Enhancing Crops for Improved Competitive Ability
Crop breeding for improved ability to compete with weeds has long been a goal for weed science. Early studies focused on identifying top-yielding cultivars when grown under intense weed pressure. Burnside (Reference Burnside1972) tested the competitive ability of 10 soybean [Glycine max (L.) Merr.] cultivars to season-long weed pressure. Three soybean cultivars, ‘Harosoy 63,’ ‘Amsoy,’ and ‘Corsoy,’ were identified as the most weed-competitive cultivars. No analyses, however, of why these cultivars were more productive was completed. Another study compared the weed competitiveness of three high-yielding, lodging-resistant rice (Oryza sativa L.) cultivars with populations of barnyardgrass [Echinochloa crus-galli (L.) Beauv.] ranging from 100 to 200 seed heads m−1 (Smith Reference Smith1974). Results suggested that late-maturing cultivars were more competitive, but again, there was no indication of any mechanism that may have accounted for this greater competitive ability. The competitive ability of tall wheat (Triticum aestivum L.) cultivars over wild oat (Avena sativa L.) was attributed to rapid dry matter accumulation per unit area during early seedling development (Balyan et al. Reference Balyan, Malik, Panwar and Singh1991). Recognizing that crop cultivar selection for weed-competitive ability was not proving successful, Korres and Froud-Williams (Reference Korres and Froud-Williams2002) concluded that crop density rather than cultivar selection was a better indicator of enhanced competitiveness with weeds. Finally, Watson et al. (Reference Watson, Derksen and Van Acker2006) summed up their research on the weed-competitive ability of 29 barley (Hordeum vulgare L.) cultivars by stating, “Correlation coefficients were not strong enough to attempt reliable co-selection within a breeding program.” In summary, the search for enhanced weed-competitive crops based on morphological traits has not resulted in the knowledge required by plant breeders to reliably enhance the competitive ability of crops with weeds.
An alternative strategy is to focus research on the molecular, physiological, and morphological mechanisms of both interspecific and intraspecific competition. To accomplish this, we must understand how crop plants detect neighbors via plant communication and how this knowledge is then transferred into action through molecular, physiological, and morphological changes within the crop plant (Ballare and Pierik Reference Ballare and Pierik2017; Choe et al. Reference Choe, Drnevich and Williams2016). Rajcan and Swanton (Reference Rajcan and Swanton2001) suggested that early detection of neighboring weeds through changes in light quality, specifically red/far-red light ratio, would be a novel approach to understanding the mechanisms of early plant competition. This was refined into a hypothesis that early-season crop–weed competition was not driven by resource limitation but rather by changes in light quality caused by the presence of neighboring weed seedlings (Rajcan et al. Reference Rajcan, Chandler and Swanton2004), and has been further tested and demonstrated since (Gal et al. Reference Gal, Afifi, Lee, Lukens and Swanton2015; Green-Tracewicz et al. Reference Green-Tracewicz, Page and Swanton2011; Page et al. Reference Page, Tollenaar, Lee, Lukens and Swanton2010). In the absence of direct competition for resources of light, water, and nutrients, neighboring weed seedlings can cause an accumulation of H2O2 (a stress indicator) in both corn (Zea mays L.) (Afifi and Swanton Reference Afifi and Swanton2012) and soybean tissue (McKenzie-Gopsill et al. Reference McKenzie-Gopsill, Lee, Lukens and Swanton2016). The molecular and physiological changes that occur as a result of the detection of neighboring weed seedlings may provide insights into the actual mechanism of crop yield loss.
There is also the opportunity to think of seed treatments not only for crop protection but as “gene triggers” that would enable crop plants to withstand physiological stress caused by abiotic and biotic variables. Seed treatments with neonicotinoid insecticides have been shown to enhance crop competitiveness in the presence of weeds. Thiamethoxam applied as a seed treatment was found to enhance maize seed germination and root growth (Afifi et al. Reference Afifi, Lee, Lukens and Swanton2015a) and to activate free radical–scavenging enzymes that reduced the accumulation of H2O2 in maize seedlings emerging in the presence of aboveground neighboring weed seedlings (Afifi et al. Reference Afifi, Lee, Lukens and Swanton2015b). Thiamethoxam also prevented the loss of nodules when soybean seedlings were grown in the presence of neighboring weeds (Kim et al. Reference Kim, Amirsadeghi, McKenzie-Gopsill, Afifi, Bozzo, Lee, Lukens and Swanton2016). The ability to place a small amount of chemical into a seed and trigger genes that enhance stress tolerance to weeds opens up a whole new area of research for manipulating crop–weed interactions.
Biological Control of Weeds
A prognosis of strategies that might be used for weed control in 2050 would be incomplete without including biological control of weeds and bioherbicides. Biological control is defined as the use of a natural enemy or a complex of natural enemies—biological control agents—to bring about weed suppression. The agents can be phytophagous arthropods (insects and mites), plant pathogens (fungi, bacteria, viruses, and nematodes), fish (e.g., grass carp [Ctenopharyngodon idella]), birds (e.g., geese (Anserini sp.), and other animals (e.g., sheep (Ovis aries)). The importation and use of nonnative agents from a different part of the world to control (i.e., suppress or manage) an exotic invasive weed in its new home is termed “classical biological control.” Use of agents indigenous to a region by augmenting their population densities above normal levels to bring about weed suppression is called an “augmentation biocontrol strategy.”
The term “bioherbicides” has two meanings. First, it has been applied to a subset of biological control achieved by mass-producing indigenous pathogens of weeds and applying them at higher than natural population densities to suppress susceptible weeds. In other words, it is an augmentation strategy, also known as inundative biocontrol. Second, the term is also used, broadly, according to the U.S. Environmental Protection Agency terminology to denote three types of biologically based herbicides: (1) biochemical herbicides (microbial metabolites, plant-derived compounds, and certain naturally occurring chemicals; discussed in “New Herbicide Targets and Biopesticides”); (2) microbial herbicides containing living or dead, plant-pathogenic or nonpathogenic microbes mixed in or not with their metabolites; and (3) genetically modified plants expressing pesticidal (herbicidal) substances (plant-incorporated protectants). It is anticipated that all of the above types of biologically based weed control methods will play a role by 2050, because they have the inherent advantages of relatively low cost of discovery and use, long-term benefits and sustainability, effectiveness, and environmental friendliness. Crops genetically modified to produce weed-fighting chemicals (allelochemicals) represent the least developed of these biocontrol technologies (Duke Reference Duke2003).
Classical biological control by arthropods and pathogens has had a long and illustrious history of practical contribution to weed control in non-crop situations. It has played an important role in different parts of the world, often with spectacular results, in bringing certain exotic invasive weeds to manageable levels, thereby providing vast savings in control costs while also mitigating environmental damage. Just a small list of examples of weeds successfully controlled by classical biological control include: control of alligatorweed [Alternanthera philoxeroides (Mart.) Griseb.] by arthropods; common St. Johnswort (Hypericum perforatum L.) by arthropods; creeping croftonweed [Ageratina riparia (Regel) R. M. King & H. Rob.] and pricklypears (Opuntia spp.) by arthropods and secondary pathogens; Port Jackson willow [Acacia saligna (Labill.) Wendl. f.] by a pathogen; purple loosestrife (Lythrum salicaria L.) by arthropods; and waterhyacinth [Eichhornia crassipes (Mart.) Solms] by arthropods and secondary pathogens. For more examples and details, see Winston et al. (Reference Winston, Schwarzländer, Hinz, Day, Cock and Julien2014).
While classical biocontrol is a proven method to manage invasive weeds in undisturbed sites such as natural areas, forests, rangelands, certain water bodies, and wastelands, it is not suitable for controlling weeds in agricultural lands that are disturbed by management practices and cropping cycles. Nonetheless, looking forward to 2050, there is value to investment in classical biocontrol of weeds. Although specific biological control agents take a decade or more in some cases to research and deploy, the return on investment from successful cases typically lasts a very long time, providing increasing returns on investment (McFadyen Reference McFadyen1998). To reap continued future benefits, certain challenges to our ability to practice biological control need to be addressed with policy leadership at governmental levels. For example, there should be a coordinated global framework to facilitate the collecting and sharing of agents for biological control and to introduce the agents following sound, scientifically based prerelease risk assessment, safety and efficacy determination, and cost–benefit analysis.
Mass-production of microbes applied as bioherbicides to suppress weeds is a promising method of weed control, and several agents have been registered or approved for use as herbicide products in Canada, China, Japan, the Netherlands, South Africa, and the United States (Bailey Reference Bailey2014; Stubbs and Kennedy Reference Stubbs and Kennedy2012). Examples include Lockdown® (previously registered as Collego®), containing Colletotrichum gloeosporioides f. sp. aeschynomene to control northern jointvetch [Aeschynomene virginica (L.) Britton, Sterns & Poggenb.]; Chontrol® (previously used as BioChon®), Chondrostereum purpureum for weedy broadleaved trees and shrubs; Hakatak®, Colletotrichum gloeosporioides f. sp. hakeae for silky hakea (Hakea sericea Schrad. & J. C. Wendl); Stumpout®, Cylindrobasidium laeve for black and golden wattles (Acacia mearnsii De Wild. and A. pycnantha Benth.); and SolviNix®, Tobacco mild green mosaic tobamovirus for tropical soda apple (Solanum viarum Dunal). Although these bioherbicides, which are in the market, and those that were registered but are currently out of the market have served specific clientele needs to an extent, their overall contribution to weed control in terms of total usage has been extremely small compared with a typical chemical herbicide. This is true with agricultural as well as environmental weeds, and the reasons include the living bioherbicides’ inconsistency in performance due to uncontrollable interactions of the biotic agent, climate, weed characteristics, and some technical aspects, such as product storage conditions and half-life, formulation issues, and sometimes the need for specialized application technology. In addition, user acceptance of this largely unfamiliar technology and competition from chemical herbicides are also major factors. The narrow spectrum of these products further destines them to be niche products. Certainly, with the ever-increasing weed resistance to chemical herbicides, an effective bioherbicide against herbicide-resistant A. palmeri could still be a valuable product.
To have wider acceptance and relevance in the coming decades, the future microbial herbicides must be capable of controlling multiple rather than single weed targets. Their efficacy should be based on certain stable gene functions or metabolites that can operate independent of minor fluctuations in ambient temperature and moisture conditions in the field. In addition, in the coming decades, understanding how certain pathogens kill plant cells, tissues, or entire plants should be a goal. To this end, work must continue on the conventional search-and-screen efforts to find suitable weed–pathogen pairs to serve as models for study. For example, host–pathogen systems wherein pathogenicity is controlled by a gene-for-gene interaction could be used to study how systemic necrosis resulting in plant death is triggered and cascaded. Exciting possibilities exist for using omics methods to discover weed and pathogen genes and gene products involved in the herbicidal end result. As more knowledge is gained on plant–pathogen interactions, the use of newer molecular methods based on RNAi, small interfering RNA, and CRISPR/Cas9 will play a significant role in the discovery of newer products and methods of weed control. These newer molecular genetic approaches to weed control will be more readily employed if they are considered to be biologically based controls and are regulated as such.
Information Technology for Extension
Communication and Computer Power
Advances in computational power and information technology will have value beyond weed control technology; they will also impact how information is transferred and used in making weed control decisions. The amount and availability of information are increasing at exponential rates, making it difficult to accurately predict how information will be transferred in the year 2050 (Figure 1C). Currently, university and industry professionals use all forms of communication resources available to convey research findings to clientele (Lubell et al. Reference Lubell, Niles and Hoffman2014). These include written publications and newsletters, telecommunicationss, office/field visits, and multiple electronic resources including email, Facebook, Twitter, Instagram, LinkedIn, Pinterest, blogs, NetMeeting, and many more. Web search engines have become so ubiquitous and powerful that almost any information can be accessed, although the accuracy of the information may be suspect. The future holds ample opportunity to make information delivery and application more efficient and effective.
One current trend is the transition from a literate to a postliterate society. At the dawn of the 21st century it was estimated that more than 80% of adults worldwide could read and write at a minimum level. However, we are entering a postliterate era, in which the ability to read or write may no longer be necessary or common (Lee Reference Lee2013). The postliterate society replaces the written word with recorded sounds—CDs, audiobooks, broadcast spoken word and music through radio, pictures (e.g., jpeg)—and moving images—television, film, MPG, streaming video, video games, and virtual reality. A postliterate society might still include people who are literate, who know how to read and write, but choose not to. Almost all people in this category would be media literate, multimedia literate, visually literate, and transliterate. Postliteracy occurs when the ability to comprehend the written word decays. Between 1982 and 2007, reading declined by nearly 20% for the overall U.S. population and 30% for young adults ages 18 to 24, and currently 40 million Americans read at the lowest literacy level (Tucker Reference Tucker2009). We spend less time reading, but the amount of pure information that we produce as a civilization continues to expand exponentially. The public appetite for electronic sounds and images seems insatiable.
Trends in Future Information Transfer
There are at least four trends that may affect future information transfer relevant to weed management: wearable technology, contextualized learning, big data, and augmented learning. Wearable technology could serve to link growers to the most relevant information sources while they are in the field. This technology is currently available in many forms and may include “awareables” (wearable technology that is aware of people and their environment). Wearables include smartwatches, activity trackers, smart jewelry, head-mounted optical displays, and earbuds. Three things are required for a wearable: contact, connections, and context. Contact is about physical contact with the body that allows devices to detect what the wearer is doing. Connections to other devices are required to create a form of intelligence. While wearables are currently tethered to smartphones today, in the future they will become independent devices that can connect to the cloud. Context means wearables have the intelligence to understand the context in which users are working with a device and to tailor the data sent as a result.
Contextual learning is when information is provided in a way that individuals are able to construct meaning based on their own experiences. Examples of contextualized learning in agriculture would be buying a bag of crop seed from your seed dealer, buying a six-pack of tomatoes from your neighborhood store, or buying a pesticide from your dealer. When a credit card is used in the transaction, the supplier of the product can download to the buyer’s electronic device personalized information such as planting dates or pesticide application advice based on that person’s location as determined through the device’s electronic tracking feature. Information about soil type, environmental requirements, fertility, moisture, maturity, and other specific facts can be modified for that person’s location.
“Big data” is a term for data sets so large or complex that traditional data-processing applications are inadequate. Data sets in agriculture are growing rapidly, in part because they are increasingly gathered by cheap and multiple information-sensing mobile devices, aerials (remote sensing), software logs, cameras, microphones, RFID readers, and wireless sensor networks. Most of these are already being used in agriculture, and their use can only be expected to grow. Accuracy in big data analysis may lead to more confident decision making, and better decisions can result in greater operational efficiency, cost reduction, and reduced risk. Challenges of big data include analysis, capture, data curation, searching, sharing, storage, transfer, visualization, querying, and information privacy.
Augmented learning is an on-demand learning technique in which the environment adapts to the learner. Learners can gain greater understanding of a topic from information provided on demand. For example, instead of focusing on memorization, the learner experiences an adaptive learning experience based on the current context. The augmented content can be dynamically tailored to the learner’s natural environment by displaying text, images, or video or transmitting audio. Most implementations of augmented learning are forms of e-learning. In desktop computing environments, the learner receives supplemental, contextual information through an on-screen pop-up window, toolbar, or sidebar. In mobile reality systems, annotations may appear on the learner’s individual “heads-up display” or through headphones for audio instruction. For example, apps for Google Glasses can provide video tutorials and interactive click-throughs. This technology could be used to provide timely education on aspects of weed biology and management practices, for example, scouting of weeds could integrate weed identification with relevant information on potential yield losses, management options, and alerts about herbicide-resistant populations.
Despite the future wide availability of information in novel formats, some traditional teaching venues will likely continue. These may include county, area, and state grower meetings, field and demonstration tours, and schools and workshops where hands-on training is conducted. State weed control guides in written or electronic formats may continue to help growers consider effective weed control practices. These educational formats have not been discontinued over many decades, even though the numbers attending have decreased. What evidence is there that these sources of education will continue? These group meetings allow on-site human interaction in the form of discussion, detailed explanation, impromptu divergence of topics, and questions and answers, all of which can be limited and cumbersome in some electronic formats. Some people may always prefer to supplement electronic resources with a hard copy of a state weed guide or pertinent publications in their pickup, tractor, or office.
Lack of changing human behavior in weed control and herbicide use is a central issue that has been extensively considered in academia and industry (Barrett et al. Reference Barrett, Soteres and Shaw2016; Ervin and Jussaume Reference Ervin and Jussaume2014; Jordan et al. Reference Jordan, Schut, Graham, Barney, Childs, Christensen, Cousens, Davis, Eizenberg, Ervin, Fernandez-Quintanilla, Harrison, Harsch, Heijting, Liebman, Loddo, Mirsky, Riemens, Neve, Peltzer, Renton, Williams, Recasens and Sonderskov2016; Riemens et al. Reference Riemens, Groeneveld, Kropff, Lotz, Renes, Sukkel and van der Weide2010; Simpson Reference Simpson2015). Accurate information on effective weed control strategies and herbicide use is available from many sources. The information has been delivered with such saturating penetration into mainstream agriculture that lack of knowledge is not a reason for nonacceptance. This pervasive and perennial trend of not adopting sustainable weed control practices has accelerated the evolution of herbicide-resistant weeds. All written journal articles, extension publications, print media, and educational venues use a myriad of tactics, including logic, reasoning, persuasion, objectivity, subjectivity, emotion, idealism, altruism, economics, efficiency, and fear to change human behavior. Despite the efforts and education spent on changing growers’ attitudes and behavior, evidence exists that adoption is slow. Rather than prognosticate how weed management information will be transferred in the year 2050, perhaps weed science should employ the expertise of sociologists and socioeconomists to make accurate information better adopted and used.
Training the Next Generation of Weed Scientists
The fundamental principles of weed science will be essential knowledge for weed science students for the foreseeable future. Herbicides will continue to be used, though perhaps in a more limited fashion, so training in herbicide chemistry, physiology, and technology must continue. Weed biology and ecology will continue to grow in importance through 2050, as insights into weed development and competitive interactions provide new options for managing weed populations. Keeping these facts and some of the other trends outlined earlier in mind, we must realize that weed science students will need more diverse training to be able to adapt to new employment opportunities. In Table 1 we suggest research and training areas that are needed if weed management is going to keep pace with weed evolution.
Table 1 Research and training needs for weed science.
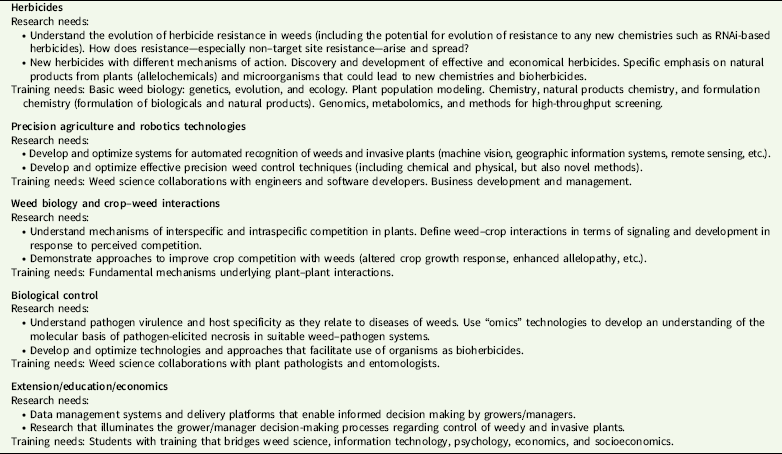
In terms of employment for weed science graduates, we foresee that opportunities in the agricultural chemical industry will likely decline further as the number of major chemical companies continues to shrink and herbicide sales shift to generic distributors that focus on sales with little emphasis on research. Instead, it is more likely that weed scientists in 2050 will work for small technology companies engaged in engineering, information technology/big data, or biotechnology that does not require large infrastructure. There is abundant opportunity for growth of agricultural implement engineering and manufacturing companies that integrate the use of robotic and weed/crop-sensing technologies with weed control actuators. These companies will need scientists who understand weed biology, ecology, and weed management, but at the same time are familiar with weed-sensing technology and advances in weed/crop detection and differentiation. Weed science students will also benefit from learning basic plant biology and plant pathology, with the interrelated fields of molecular biology, genomics, bioinformatics, and biotechnology providing fertile ground for developing new insights into plant competition, plant pathogen interactions, or gene silencing. Integration of the diverse and growing knowledge of weed biology and ecology, and factors that affect them, will be necessary for development of integrated weed and resistance management strategies that preserve new technologies as they become available. Above all, students will need to be technologically savvy. This is essential for manipulating and interpreting the big data that are the increasingly the common output from experiments. Students will also need to be ready to engage with growers who are embracing technology in their operations. The amount of data available to growers will only increase, and there will be many opportunities for the weed expert to use such data to understand and manage weed populations.
Conclusions
Meeting the world’s requirements for food and fiber crops in 2050 given current weed control methods is a daunting task. Prospects look bleak without new herbicide MOAs or a coordinated strategy to manage and prevent herbicide-resistant weeds. But trends in computing power, robotics, and life sciences suggest that multiple paths exist for improving weed control that can be integrated with existing methods to create more sustainable weed management systems (Figure 1). However, time is short and new technologies may take years to develop and implement, so a sense of urgency is needed. In this article, we have identified priority areas for research and education and hope that progress will follow. We would like to see more investment in research into novel and sustainable methodologies, ideally from a diverse range of sources, including industry and commodities as well as state and federal funders. Universities and government laboratories should create new positions devoted to novel approaches to weed management. Regulatory modifications and incentives that smooth the way for innovation in weed control would also be valuable in accelerating implementation of research advances over the next decade. Finally, today’s students are the ones who will deal with this challenge over the course of their professional careers, and they must be trained broadly and encouraged to think creatively to be ready to discover and implement transformational weed management strategies.
Acknowledgments
The authors acknowledge support for the symposium from WSSA. Additional support to J.H.W. was provided by National Institute of Food and Agriculture project no. 135997. No conflicts of interest have been declared.