Introduction
Clinical application of genome-scale sequencing could transform medicine, but its implementation presents formidable challenges. Genomic technology is highly dimensional, with many kinds of potential results, interpreting genomic findings requires specialized expertise, the genomic service is complex, coordination is required across multiple medical specialties, results have broader implications beyond the patient, and testing may have uncertain clinical utility. To address this, national agencies have funded transdisciplinary consortia to investigate the multidimensional public health challenges associated with using genome-scale sequencing technologies as part of clinical care. These consortia offer very large sample sizes and broad expertise to address key questions related to clinical utility. The consortia can identify common challenges and crowdsource for solutions. They also learn from one another to minimize duplicative efforts. Although research consortia provide the needed population sizes, they often span a diverse set of studies and institutions. As such, to realize their full promise, research consortia must address the challenge of developing approaches that are effective across transdisciplinary teams.
Team science is a promising approach to this issue. Team science refers to the approach of conducting research in teams within complex social, organizational, political, and technological contexts that heavily influence how scientific work occurs [Reference Hall1]. Research on “team science” has generated evidence regarding different factors that enable or constrain research collaborations [Reference Stokols2–Reference Hall4]. Funding agencies’ willingness to invest in building and cultivating an environment conducive to multi-institutional, multidisciplinary large-scale consortia is also essential to advancing team science [Reference Croyle5]. Despite promising evidence to date, more research is needed to understand the specific factors that enable or constrain collaboration across large-scale consortia. Varying levels of effective collaboration among research consortia point to the need for more research of the contextual determinants of collaborative success [Reference Stokols2]. Understanding the ecology of team science [Reference Stokols6], or the organizational, institutional, physical environmental, technologic, and other factors that influence the effectiveness of multidisciplinary collaboration in research, is critical and yet understudied.
To fill this gap in the literature, we describe our experience working in the Clinical Sequencing Evidence-Generating Research (CSER) consortium, a multi-institutional translational genomics consortium funded by the National Human Genome Research Institute (NHGRI) in collaboration with the National Cancer Institute and the National Institute on Minority Health and Health Disparities [Reference Amendola7]. This program was developed specifically to advance the science on clinical utility of genome-scale sequencing technology and required substantial collaboration throughout the consortium to achieve its objectives. Specifically, we used our experience engaging in team science to develop common, harmonized survey measures and outcomes to explore the tension between consortium goals and individual project goals. We also explore how this dynamic shaped the ultimate design of the harmonized measures. This account identifies the resources needed for a team science model that enables coordination and collaboration between individuals within institutions, institutions within projects, and projects within consortia.
Materials & Methods
CSER “Team” Description
The CSER consortium includes six extramural clinical projects, an NHGRI intramural project (ClinSeq), and a centralized coordinating center [Reference Amendola7] (Fig. 1) that all share two common themes. First, all projects are investigating the clinical utility of genome-scale sequencing, and thus share the challenges of implementing this technology. Second, all projects have a specific focus on enrolling diverse populations and thus share a commitment to understand and address the challenges that contribute to health disparities experienced by populations because of factors, such as race/ethnicity, socioeconomic status, literacy level, and primary spoken language.

Fig. 1. Structure of Clinical Sequencing Evidence-Generating Research (CSER) consortium. The CSER consortium includes six extramural projects (blue text), one intramural project (ClinSeq A2), and a coordinating center. Each project includes between one and seven implementation sites (bulleted lists).
Despite these shared interests, the CSER consortium projects are highly variable in terms of the application of genome-scale sequencing technology to different study populations and clinical contexts (Table 1). The CSER projects are geographically diverse, and all projects were designed to involve patient populations at more than one clinical setting or institution, including traditional academic medical centers, community hospitals, and federally qualified health centers. All sites partner with local stakeholders including patients, advocates, payers, clinicians, and health system leaders. The CSER projects are also implemented in various clinical settings from neonatal intensive care units to adult outpatient clinics. The CSER consortium developed the genomic medicine integrative research framework, which highlights the complexity involved in integrating genomic medicine and prevention into clinical practice and the need for diverse areas of expertise to address research gaps [Reference Horowitz8] and demonstrates domains that investigators can measure. Each project includes transdisciplinary teams of geneticists, clinicians, ethicists, and genetic counselors, among others (Table 2). In its entirety, the CSER consortium involves about 330 researchers and clinicians across 28 institutions.
Table 1. Description of Clinical Sequencing Evidence-Generating Research (CSER) projects

CHARM, Cancer Health Assessments Reaching Many; NCGENES 2, North Carolina Clinical Genomic Evaluation by Next-generation Exome Sequencing 2; P3EGS, Prenatal and Pediatric Genome Sequencing.
*CHCS, community health care system; AMC, academic medical center; FQHC, Federally Qualified Health Center; RI, research institute.
Table 2. Description of Clinical Sequencing Evidence-Generating Research (CSER) project teams

CSER Workgroup Structure and Processes
The CSER consortium established six working groups to facilitate collaboration across projects that have been described in detail [Reference Amendola7]. Briefly, these include Ethical, Legal, and Social Issues and Diversity; Stakeholder Engagement; Clinical Utility, Health Economics, and Policy; Education and Return of Results; Survey Measures and Outcomes (MOWG); and Sequence Analysis and Diagnostic Yield (SADY). Each work group includes at least one representative from each project with expertise appropriate to the work group and two co-chairs lead the activities of each working group. The CSER Coordinating Center facilitates monthly teleconferences, biannual in-person meetings, and a yearly online virtual meeting.
Relevant to this manuscript, all six CSER projects planned to administer surveys to participants or parents/guardians of pediatric participants. Most planned to administer surveys to participating clinicians. Four of the six working groups developed specific survey measures to be administered across all projects to collect harmonized data across the consortium. The SADY and the Stakeholder Engagement work groups did not develop harmonized survey measures because they focus on other types of data collection, including genomic sequence and interpretation and qualitative research approaches, respectively. In this manuscript, we share the perspectives and experiences from the MOWG. The MOWG focuses on measurement of patients’ and family members’ knowledge, attitudes, beliefs, behaviors, and psychological outcomes. The work group also studies provider knowledge, attitudes, beliefs, and behaviors, including diagnostic thinking and clinical management planning.
Initial Survey Development Plan
Although all CSER projects seek to generate data on the utility of genome-scale sequencing, each project independently established their research plans in the grant applications. Sites had widely varying aims, such that there was uneven alignment and limited overlap of planned measures. Projects selected different modes of survey administration and differed in their ability to capture utilization, referrals, and other downstream actions electronically through the medical record. Some projects planned to capture this information as patient-reported data through surveys, whereas others proposed to use the electronic medical record. These differences were largely driven by pragmatic considerations based on what was anticipated to be most successful within the context of the project design and study population.
The projects also varied in when they planned to administer surveys, as well as the amount of lead time they had prior to initiating recruitment. Some projects, for example, started almost immediately while others initiated recruitment up to 18 months later. Finally, the study design and survey population for each project were different. As the first step to harmonization, therefore, the MOWG organized measures around three groups (Decliners, Study Participants, and Providers) that were expected to participate at each site. Decliners were defined as people who declined participation in a study and were surveyed to understand the reasons why they did not join the study and to compare their characteristics with those who agreed to enroll (Study Participants). Decliners and Study Participants could include adult patients, pediatric patients, or parental proxies. Providers were clinicians recruited in order to capture how they understood and used the genomic information.
While each individual project was independently reviewed by an Institutional Review Board (IRB), the work described in this manuscript to harmonize survey measures and design and develop the survey instruments is primarily conducted as part of the development of study materials and occurred in preparation for submission of the materials for review by the IRB.
Results
Guiding Principles for Harmonization
A key aspect of the CSER Funding Opportunity Announcement was the juxtaposition of site-specific measures with the need to identify consensus measures related to clinical utility, resulting in the need for Working Group discussions to address the heterogeneity of study designs [9]. The goal of the harmonization process was to identify a core set of harmonized measures, although sites could still retain the flexibility to collect additional or more granular data. The MOWG was tasked with overcoming this variability between studies to develop and apply guiding principles for harmonizing measures across the consortium. One key goal was to balance the expectations of the consortium with the individual project’s plans and priorities. Many members of the MOWG have expertise in survey design, and individuals may also have recommended general principles of survey design as part of these considerations (Table 3).
Table 3. Elements of survey design*
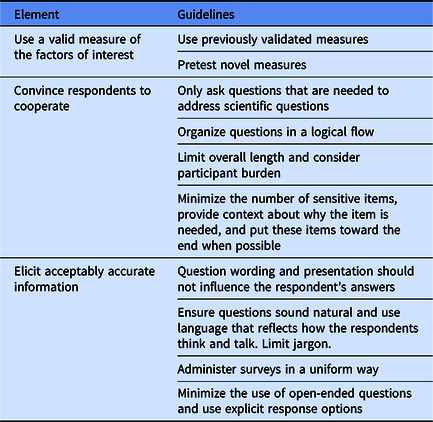
* Paraphrased from Ronald Czaja and Johnny Blair [Reference Czaja and Blair15].
Minimize Participant Burden
Projects sought to minimize questions unrelated to their primary research objectives that might increase participant burden and discourage survey completion. Thus, the principles emerged to limit the number of survey items proposed by the CSER consortium, to constrain the items to those that were needed to address specific hypotheses, and to ensure that the mode of administering consortium measures would be flexible.
Accommodate Project-Specific Choices in Timeline and Respondents
The ideal timing for deployment of harmonized measures from the CSER consortium perspective was for the timing of survey administration to be standardized across all projects. We found substantial variation, however, in each project’s existing plans. Although most projects planned to conduct a baseline survey immediately after consent, the timing of follow-up surveys ranged from immediately after the results disclosure visit to up to 4 weeks later. To measure longer-term outcomes, projects had to balance the pragmatic issues related to completing the project within 4 years with the challenge that some outcomes could take up to a year or longer to occur.
Once the projects were aware of each other’s plans, the project-specific plans partially converged in terms of the timing of surveys. Nevertheless, the unique objectives of each project naturally placed some constraints on the ability to harmonize the collection of outcome measures. It was particularly burdensome, for example, for projects to add a survey for a new type of respondent or time point compared with simply adding questions to an existing survey. Additionally, some projects started recruitment before the consortium had an opportunity to finalize the harmonized measures. These projects began data collection without the harmonized measures and then had to repeat some work (e.g., IRB submissions, programming online surveys or redesigning paper instruments) to accommodate the consortium’s harmonized measures. Thus, consortium-wide analyses will be unable to combine data for all study subjects across the consortium if either the survey measure was substantially altered from the harmonized version or were introduced mid-stream. This led to the guiding principles that projects could opt out of certain measures that were deemed too burdensome or they could choose other ways to minimize burden (e.g., to collect data for only a subset of respondents).
Use Validated Measures that Allow Data Sharing
To allow for the possibility of combining data with projects outside of CSER, the consortium preferred to use existing, validated measures whenever possible and to minimize changes to existing measures. Because projects were constrained by existing budgets and our principle to support broad data sharing, we avoided using proprietary measures that required payment in most instances. We also did not include survey measures that contained identifiable information (e.g., zip code or birthdate) that would complicate data sharing procedures.
Challenges with the Harmonization Process
Communication and Coordination
While monthly teleconferences were not held frequently enough to advance some harmonization goals, project teams did not have the capacity to hold teleconferences more frequently. Thus, a substantial amount of group work was conducted by email, which has known limitations. To address this issue, the consortium attempted to shift to another online communication platform to facilitate more organized communications. This strategy, however, had limited success and was adopted late into the harmonization process. Additional barriers to the adoption of the new online communication platform were project team members’ lack of familiarity and the fact that it was used exclusively for this project.
The consortium also lacks a common platform for sharing documents, which resulted in challenges with compiling measures across work groups that used different formats, version control, and managing iterations of the documents. Although several commercially available solutions exist, the institutions involved in CSER placed different constraints on access and use, with a primary concern being data security. Considering these limitations, the consortium used static text documents to share and compile measures. When the process started, there was no standardized format for formatting measures, so workgroups compiled their measures across several documents and in nonstandard formats that could not be easily combined. The CSER Coordinating Center reviewed these workgroup documents, sorted the individual measures according to when it would be administered to participants, and then recompiled the measures into static documents. To do so, the Coordinating Center had to standardize format for clarity and add meta-information to consider when programming the measures into research data capture systems. As these were static text documents, clearly indicating complex survey branches or skip logic was difficult and created ambiguity that took effort to clarify. To share these documents, the Coordinating Center posted finalized versions of these documents on a static webpage. Throughout this process, the Coordinating Center held version control of these documents. To facilitate version control, each document had a changelog dating all changes and reasons for the change. All documents were updated on a scheduled basis to allow for iterative revision. While these procedures do not address all barriers, they represent an important step forward for the consortium.
Project Variation in Defining Populations of Survey Respondents
Another challenge for the harmonization process was that different projects were defining types of respondents differently. The CSER consortium, for example, needed to harmonize the definition of what was meant by a “decliner” respondent. Some projects used a staged consent approach, where individuals could participate in some activities but not others. In the North Carolina Clinical Genomic Evaluation by Next-generation Exome Sequencing 2 (NCGENES2) project, for example, participants were randomized to two phases and could decline at either step. In the Cancer Health Assessments Reaching Many (CHARM) project, individuals could decline participation prior to full determination of their eligibility status. For the purposes of the survey, the consortium ultimately defined a decliner respondent as someone who was eligible for, and offered, genome-scale sequencing and declined to receive it either before or after providing consent.
The difference in study designs across the CSER consortium also made it difficult to define which providers were eligible for participation in surveys due to the inclusion of numerous types of providers and the fact that some projects involved providers who were not part of the study team. For the purposes of the surveys, the consortium decided to define a provider respondent as someone responsible for acting upon the results of the genome-scale sequencing, which may or may not be the same provider who disclosed the result to the participant. Thus, the provider respondents may include individuals with different areas of expertise on the project, which may or may not be part of the study team. These decisions necessitated the collection of additional data describing characteristics of the providers.
Challenges with Harmonizing the Measures
The CSER consortium harmonized a total of 31 survey domains with 128 items for study participants or parental proxies across 3 surveys, 31 items for provider respondents, and 24 items for decliners (Table 4). The consortium developed novel items for 13 of these domains, and we modified existing measures for 15 out of the remaining 18 domains. The types of modifications included altering wording in the introduction, stem, or item; changing response categories (adding, removing, or renaming); or deleting some items in a multi-item measure. We note that some measures presented in Table 4 were developed by other consortium work groups and may not have followed the same guiding principles of harmonization or experienced the same challenges, but we include the entire list for completeness.
Table 4. Clinical Sequencing Evidence-Generating Research (CSER) harmonized measures and survey domains
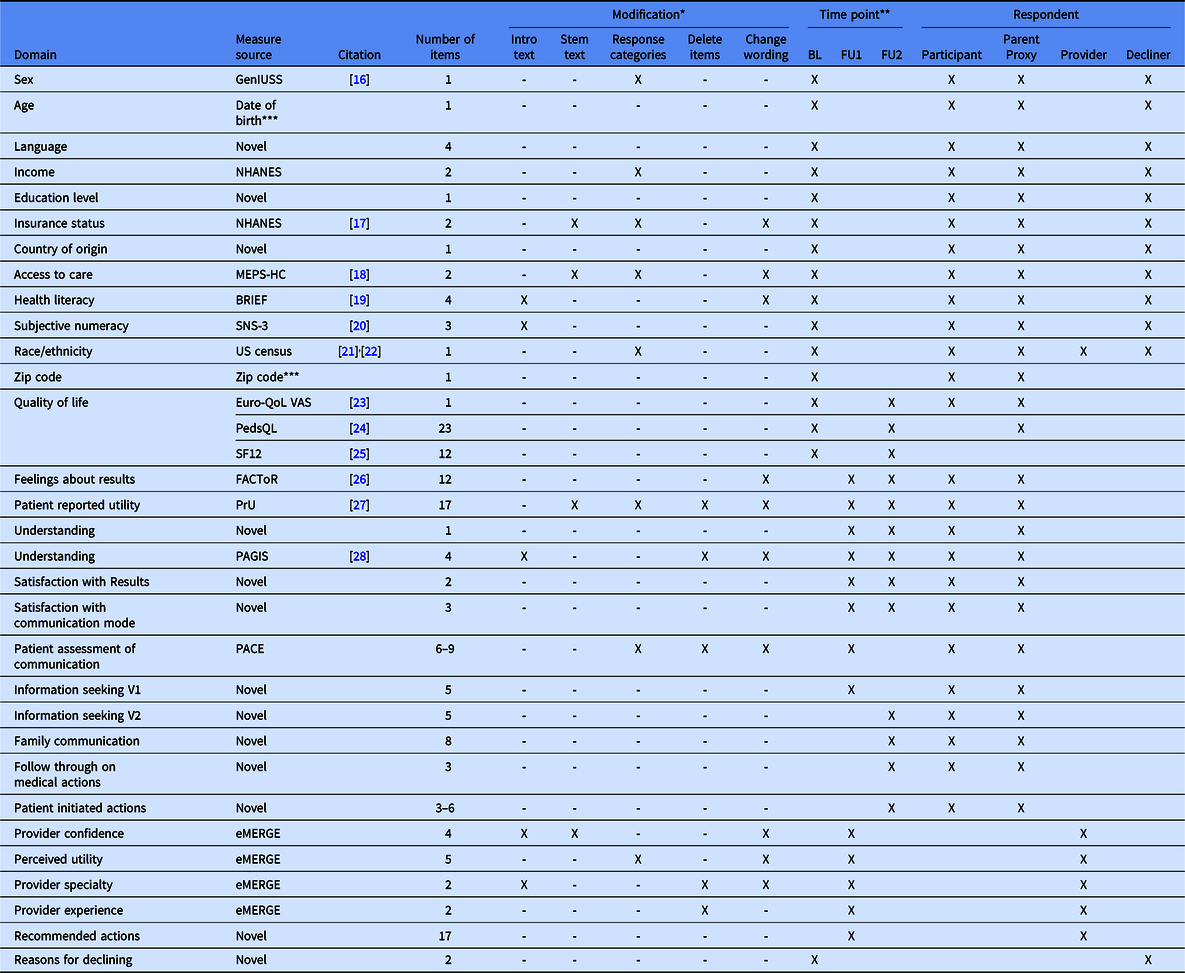
MEPS-HC, Medicare Expenditure Panel Survey-Household Component; NHANES, National Health and Nutrition Examination Survey; GenIUSS, Gender identity in US Surveillance; SNS, Subjective Numeracy Scale; QoL VAS, Quality of Life Visual Analogue Scale; FACToR, Feelings about genomic testing results; PrU, Patient-reported utility; PAGIS, Psychological adaptation to genetic information scale;PACE, Patient assessment of communication.
* Modification: Intro text, this means that introductory text was added, removed, or reworded; Stem text, this means that the stem text was added, removed, or reworded; Response categories, this means that response options were added, removed, or reworded; Delete items, this means that the original measure included multiple items, some of which were deleted; Change wording, this means that the wording of the item was revised.
** BL, baseline survey; FU1, follow-up survey at 0–4 weeks after results disclosure; FU2, follow-up survey at about 6 months after results disclosure.
*** These measures contain protected health information (PHI) that is converted by the project into another de-identified measure, such as age or age categories, prior to sharing across the consortium.
Adaptation Challenges
Because of our focus on diversity, many of the CSER consortium’s harmonized measures were developed and validated in prior studies that included populations very different from the populations in our studies. The CSER consortium teams made a considerable effort to ensure that study materials were appropriate for low-literacy participants and culturally acceptable. Thus, measures previously developed without this attention were noticeable when embedded within materials that included items at a lower literacy level. Although the consortium preferred to minimize changes to established and validated measures, it was necessary to adapt the materials to our settings and populations. In addition to adaptations for literacy and cultural sensitivity, validated measures were shortened to address concerns about participant burden.
Design Challenges
Design challenges refers to how the measures are presented and how they fit within the structure of the overall survey. One issue we encountered with the survey design was determining whether measures must be presented in the same order for all projects. From the consortium perspective, consistency was highly desired to minimize effects caused by differences across projects. However, projects that prioritized different measures and surveys also included project-specific measures. Researchers expressed concerns about the possibility of respondent fatigue, leading some to administer a particular measure early in a survey, whereas other studies might choose to administer it later. Researchers were also concerned that sensitive or confusing items might discourage survey completion and that local context contributed to different perspectives. For instance, items that asked about gender identity might be perceived differently by adult respondents compared to the parental proxies responding for their newborn child. Similar disagreements emerged among researchers over a question asking for country of birth and whether it was considered sensitive because of its implications for perceived immigration status. Ultimately, we did not harmonize the order in which items were asked on the surveys and sites were free to not include items due to context-specific sensitivities.
In addition, some projects preferred slight changes to the wording to fit the project-specific study design or population. For instance, one site changed the term “clinical team” to “genetic counselor” because the study activities would only involve interaction with a genetic counselor and thus referring to a team might be confusing. From the consortium perspective, wording changes were discouraged because of the potential impact on the ability to combine data across projects. From the individual project perspective, some changes were felt to be essential to not offend or confuse study participants and to ensure the integrity of data collected for that particular project. We documented project-specific deviations in the wording of the harmonized measures [10], which is infrequent.
A final design challenge was the overall number of measures. Individual projects were concerned that participant burden and survey length would discourage completion of study activities. The consortium identified measures as necessary, recommended, or optional to help sites prioritize measures of greatest scientific value to the MOWG. For the necessary measures, the project had to provide a justification to program officers for why the measure was not included and we documented whether projects opted out or changed wording of specific measures.
Work Group Challenges
The CSER consortium included multiple work groups who proposed survey items specific to their domain area, but that were developed independently on a parallel time frame. This process created challenges once all of the survey measures were assembled. First, it became clear that the work groups had proposed different words to represent the same concepts across items, which could be very confusing to survey respondents. One example is the work groups refer to the genetic test result as “gene change”, “test result”, and “study result”. The consortium did not have a mechanism for addressing these inconsistencies across workgroups, and we ultimately did not harmonize the terminology due to a lack of time and the need to get surveys into the field in a timely fashion.
Another issue experienced across work groups was the different intended uses for the same question, such as capturing self-reported race/ethnicity information. For the MOWG, these variables were used as covariates in analyses, and the preference was for only a small number of categories. For the SADY work group, on the other hand, these variables were included detailed analyses as part of variant interpretation in relation to genetic ancestry information, and a large number of categories with fine detail were preferred. In some instances, we were able to resolve the differences in priorities across work groups by capturing more detailed information, which could be collapsed into a smaller number of categories depending on the analysis.
Discussion
Our experience developing common measures to use across projects in a large research consortium highlights the tension between balancing the needs and priorities of the individual projects with those of the overall consortium. In contrast to multi-institutional studies that implement a common protocol at many sites, the CSER consortium is a set of loosely affiliated projects that share a common theme, but that have different project-specific objectives, clinical contexts, and study populations. While the CSER program was clearly established with a goal of addressing issues of clinical utility of genome-scale sequencing [9], the specific projects turned out to be highly variable. This variation across projects and competing priorities may represent one of the most challenging circumstances to find common ground in a research consortium, making this a particularly good example to explore this tension. For example, it was surprisingly difficult to come to agreement on measures, even for fundamental demographic information. The literature on team science has suggested that effective multidisciplinary collaboration relies on the development of shared conceptual frameworks that integrate and transcend the multiple disciplinary perspectives that are represented across teams [Reference Rosenfield11]. Our experience underscores this point and also suggests that researchers must account for differences in the local social contexts and the population’s needs that create challenges for harmonizing measures. Our experience also revealed several recommendations for future research consortia interested in engaging in team science.
Lessons Learned/Recommendations
Facilitate Communication across Projects and Work Groups
Effective communication and mechanisms to support and facilitate this communication are essential for collaboration. This often requires deliberation to determine the specific abilities and needs of participating teams. We recommend that consortia carefully consider the technology solutions and tools available to them at the beginning of their collaboration and select a communication platform that has useful features, including well-organized conversations, easy access to information, and limits to sideline conversations. We also recommend that consortia apportion sufficient time and funding for communication about project-specific and consortia goals, including anticipating the need for harmonization. This may require creating structures for easy communication between work groups to ensure that the work does not become too siloed.
Assure Management of Materials and Data
The need for consortia to ensure the appropriate management of written materials and data is a related issue. We recommend that the roles and responsibilities of managing and hosting documents and data are clearly assigned to individuals within the consortium who have access to appropriate resources, tools, and capacity to maintain this responsibility. A version control system should be used for documents to ensure that all interested parties are able to see the current version of documents, as well as historical versions, to conduct their work.
Information and Resources Needed in Building Consortia
Funding agencies that are planning to fund research consortia can promote successful collaboration through detailed planning and clear communication of expectations. Specific design elements that are key requirements for successful collaboration can be clarified as part of the request for applications (RFA). For instance, in the case of the CSER consortium, knowing the requirements for the use of surveys, their timing, and selected respondents would have allowed projects to build in this into their study design from the beginning. If the scope is not clear and changes emerge after the application stage, allowing for a budgeting step after the change in scope will facilitate greater success of the consortium in carrying out the proposed activities. Finally, collaboration across a consortium requires an iterative process, which takes time and effort. The consortium’s plans must be developed as a collaborative process that allows each project to integrate consortium goals into the individual project plans. Allowing more time for this iterative process to take place before launching the individual research programs will promote successful harmonization across the consortium. Providing sufficient funding for consortium activities is also critical to the success of any collaboration.
Challenges for Analysis and Future Use
We recognize that additional challenges will arise after data collection. The logistics of sharing data across institutions requires data sharing agreements to prevent misuse of data collected through the consortium. The large number of institutions involved in the consortium, however, provides an added layer of difficulty in navigating the processes to formalize these agreements. While the consortium did not include a data coordinating center when it was initially established, it became clear that a data coordinating center would be needed to establish efficient processes and procedures for transferring data across the consortium and to control versions of the data sets. We also recognized that the harmonized measures may be used for more than one purpose, which can lead to conflicts if different projects or workgroups propose overlapping ideas. To account for this, the consortium established a written document to outline the process to recognize and resolve such conflicts.
The work of the CSER consortium represents a substantial advancement over our prior work. The challenges associated with conducting consortium research without establishing common measures across projects were documented in several publications [Reference Robinson12,Reference Gray13]. Nevertheless, it is still unclear the extent to which combining data across the CSER consortium projects is advantageous given the different underlying populations, settings, and research questions for each project. The medley of programs that were ultimately selected to be part of the CSER consortium are loosely related through the themes of clinical utility and consensus measures of clinical utility, a common sequencing technology platform, and a focus on population diversity. These harmonization efforts will enable us to use common measures across a variety of clinical contexts to assess clinical utility, at least enabling cross-site comparisons of clinical utility on the same scale. However, there is not necessarily a strong rationale to combine data across fundamentally different populations or clinical contexts for all harmonized measures.
Conclusions
Many of the challenges identified in the team science literature are relevant to the CSER consortium. The high diversity of expertise across team members and the large size and geographic dispersion of projects and sites underscore the need for effective communication and organizational tools [14]. Furthermore, consortia that include variation in individual project goals create the potential for goal misalignment. In our experience, many of the challenges in harmonizing measures stemmed from conflicting perspectives between consortia and local projects, and the timing of consortia work. Furthermore, the lack of communication and interdependence between working groups led to obstacles to harmonization. Time and resources must be devoted to developing and implementing collaborative practices as preparatory work at the beginning of project timelines in order to improve the effectiveness of consortia. These experiences underscore results from team science studies that suggest the importance of prioritizing discussion of team processes to identify team goals and accessible tools for effective collaboration.
Acknowledgments
The Clinical Sequencing Evidence-Generating Research (CSER) consortium is funded by the National Human Genome Research Institute (NHGRI) with co-funding from the National Institute on Minority Health and Health Disparities (NIMHD) and the National Cancer Institute (NCI), supported by U01HG006487 (UNC), U01HG007292 (KPNW), U01HG009610 (Mt Sinai), U01HG006485 (Baylor), U01HG009599 (UCSF), U01HG007301 (HudsonAlpha), and U24HG007307 (Coordinating Center). The contents of this paper are solely the responsibility of the authors and do not necessarily represent the official views of the NIH. The CSER consortium thanks the staff and participants of all CSER studies for their important contributions. More information about CSER can be found at https://cser-consortium.org/. L.A.H., as an employee of the NIH, is responsible for scientific management of the CSER Consortium.
Disclosures
The authors have nothing to disclose.