In many countries, healthcare policy has been concerned to minimise hospital admissions and reduce length of stay (LOS) for people with long-term conditions, often using case management (Hokenstad and Johansson Reference Hokenstad and Johansson1996; Landi et al., Reference Landi, Lattanzio, Gambassi, Zuccala, Sgadari, Panfilo, Ruffilli and Bernabei1999; Ashton, Reference Ashton2000; Johri et al., Reference Johri, Beland and Bergman2003; Dixon et al., Reference Dixon, Lewis, Rosen, Finlayson and Gray2004; Department of Health, 2005a; National Health Priority Action Council, 2006; Hofmarcher et al., Reference Hofmarcher, Oxley and Rusticelli2007). In England, case management has been characterised as ‘the active management of high-risk people with complex needs, with case managers (usually nurses) taking responsibility for caseloads working in an integrated care system’ (Department of Health, 2004a: 3). Case management for long-term conditions (CMLTC) therefore has the broad aim of identifying ‘very high-intensity users’ of unplanned secondary care and actively managing their care to enable them to remain at home longer and require less unplanned reactive care from specialist services. The role of community matrons (case managers with clinical nursing skills) has been specifically developed to undertake the case management function. It was estimated that there were around 250 000 ‘very high-intensity users’ eligible for case management, and that 3000 community matrons would be required to manage them (Department of Health, 2004b; 2005b). This approach was expected to contribute significantly to delivering the Public Service Agreement target of reducing bed days by 5% by 2008 (Department of Health, 2004b). Subsequently, it has been suggested that this target has been achieved, although driven by reductions in mental health and circulatory bed days, attributable to disease-specific management rather than CMLTC (Department of Health, 2007).
Although patient and carer satisfaction may improve, the evidence that case management for patients with complex long-term conditions contributes to outcomes such as reducing hospital admission, LOS and improving patient's well-being is equivocal (Hutt et al., Reference Hutt, Rosen and McCauley2004; Singh, Reference Singh2005). Some studies of case management interventions have reported significant reductions in emergency admissions, other hospital admissions or bed days (Bernabei et al., Reference Bernabei, Landi, Gambassi, Sgadari, Zuccala, Mor, Rubenstein and Carbonin1998; Landi et al., Reference Landi, Lattanzio, Gambassi, Zuccala, Sgadari, Panfilo, Ruffilli and Bernabei1999; Leung et al., Reference Leung, Liu, Chow and Chi2004; Lyon et al., Reference Lyon, Miller and Pine2006; Patrick et al., Reference Patrick, Roberts, Hutt, Hewitt, Connelly and Oliver2006; Bird et al., Reference Bird, Kurowski, Dickman and Kronberg2007; Huws et al., Reference Huws, Cashmore, Newcombe, Roberts, Vincent and Elwyn2008). However, other studies including the evaluation of the Evercare demonstration in England (Gravelle et al., Reference Gravelle, Dusheiko, Sheaff, Sargent, Boaden, Pickard, Parker and Roland2006) and a large randomised control trial (RCT) of an integrated system that included case management did not show significant reductions in inpatient care or hospital days (Béland et al., Reference Béland, Bergman, Lebel and Clarfield2006).
This paper reports on a Department of Health-funded multi-method study that examines what factors may be associated with changes in emergency admissions and associated LOS for people with long-term conditions in receipt of case management.
Methods
Both organisational and patient level data were collected for this study (REC ref 07/H1006/51). Observable features of case management arrangements were obtained through a cross-sectional postal survey of managers with lead responsibility for CMLTC in 10 primary care trusts (PCTs). The 49-item questionnaire, developed by the research team (with a long history of process and outcome research in the field), described the current provision of nurse case management services in all 10 of the PCTs in Greater Manchester. The questionnaire was distributed in July 2007 and non-respondents were contacted up to 10 weeks later to maximise the response rate. A 100% response was achieved from 10 managers who all had particular responsibilities for the development of the CMLTC service in their locality. In-depth interviews were undertaken in eight areas and these are reported elsewhere (Challis et al., Reference Challis, Abell, Reilly, Hughes, Berzins and Brand2008; Abell et al., Reference Abell, Hughes, Reilly, Berzins and Challis2010).
The impact of these services was measured through a retrospective cohort analysis of patients enrolled to CMLTC with complete longitudinal admission data for the nine months before and nine months after entry (n = 867). Patients were excluded from the analysis if they had died within the nine months after entry period (n = 99), but included if they died after that (n = 50). The dates these patients had been added to CMLTC caseloads ranged from 1 July 2005 to 1 October 2006. Patients registered after this period were ineligible, as they did not have nine months follow-up data at the time of data extraction. Resource utilisation outcome data for patients with long-term conditions in receipt of case management were tracked through data held by the Tactical Information Service (TIS)Footnote 1. Data were extracted from it at the end of September 2007. Individual patient level data were transferred into a pseudonymised format by researchers for subsequent data preparation and analysis. To check the consistency and validity of the data the team looked for conflicts in data on individual cases and arranged for review of these by the National Health Service (NHS) staff. Where the conflicts could not be resolved, cases were excluded from the analysis. Questionnaire data and patient-related data were analysed in SPSS (version 14).
The results are presented in four sections. The first section summarises the organisation and process of CMLTC services using a range of indicators developed from the survey questionnaire. The second section describes the cohort sample in receipt of case management in terms of demographic and diagnostic characteristics and the third section shows service use over the time periods measured. In the final section, the overall contribution of CMLTC service arrangements upon service utilisation is explored and we attempt to explain changes in patient level health service utilisation outcomes on the basis of measured factors. Particular focus is given to changes in one of the health service utilisation outcome measures: LOS for emergency admissions. Its distributional properties rendered it the most suitable for regression analysis. Potential predictors were identified by means of a model exploring the simultaneous effect of diagnosis and features of case management on changes in LOS associated with emergency admissions. The two most common longitudinal analysis designs were used: direct analysis of change (or change-score analysis) and analysis of covariance (ANCOVA), although only the results of the latter are presented in this paper. It is acknowledged that great care must be taken when attempting to draw causal inferences from observational data in general and an ANCOVA design in particular. However, it is also noted that recent statistical research has cast doubts on the traditionally cited reservations about applying ANCOVA to observational data (Senn, Reference Senn2006).
Regression analyses were carried out in a series of stages (Stata version 9.2), initially to select the most appropriate variables to be entered into the model. Variables considered were those related to the organisation and process of CMLTC services and demographic and diagnostic characteristics of the patients. As a rule, P-values ⩽0.05 were considered to be statistically significant (Altman, Reference Altman1991). First, all relevant variables (demographic, diagnoses/hospitalisation related, PCTs, CMLTC characteristics, etc.) were tested individually and short-listed for regression models if statistically significant effects were found. Second, groups of variables were entered and the least significant ones were removed in a stepwise elimination process until only significant ones remained. Finally, further adjustments were made after regression diagnostics, such as the removal of outliers, alternative analyses with transformed variables or robust standard errors, in order to arrive at final models that are both sufficiently robust and interpretable. It was assumed, and there was reason to believe, that the available cases with before and after nine months information represent a simple random sample of the broader target population. Calculating the so-called intra-class correlationFootnote 2 revealed no clustering effects within PCTs, that is, the most logical source of such effects, suggesting the suitability of the sample for standard statistical analysis methods that assume independent observations.
Findings
Case management services
Table 1 describes the CMLTC services using a range of indicators drawn from the survey questionnaire. They are described in detail with reference to individual PCTs elsewhere (Abell et al., Reference Abell, Hughes, Reilly, Berzins and Challis2010). The date the first patient was accepted into each CMLTC service ranged from the first six months of 2005 to the first six months of 2006 for the 10 PCTs. Case managers were managed by health service staff in all PCTs and nearly all were part of a nursing team. Those respondents who reported staff based in more than one location had pilots running alongside standard service arrangements. One service had an integrated healthcare and social-care team pilot, in addition to case managers located in general practitioner (GP) practices and the other had some staff based in GP practices, alongside those in nurse teams. Formal links with social care, such as social workers acting as case managers, were relatively uncommon. Similarly, the extent to which the CMLTC service was linked to self-care arrangements, one of the means of ensuring effective step down from case management, was not wide ranging.
Table 1 The case management services

PARR = patient at risk of re-hospitalisation; PCT = primary care trust.
Staff groups most likely to act as case managers were community matrons, district nurses and other qualified community nurses such as disease specialist nurses. However, this varied by complexity of patient need, with a higher proportion of very high intensity users having community matrons, nurse consultants and advanced practitioners in training as case managers. A broad range of tasks were usually carried out by case managers in all PCTs and some role conflict for certain staff groups was highlighted. For example, district nurses undertook a disproportionate amount of ‘hands on’ or direct care. Size of caseload was an issue of contention and varied from 30 to 80 (mean 47). Some felt that the target caseload of 80 was unrealistic.
With regard to the process of case management, all PCTs used referrals from other professionals to identify patients for the CMLTC service and the majority also utilised the Castlefields tool (Lyon et al., Reference Lyon, Miller and Pine2006) and Patient at Risk of Re-hospitalisation II (PARR II; Billings et al., Reference Billings, Dixon, Mijanovich and Wennberg2006). A locally approved Single Assessment Process tool to assess CMLTC patients was used in all services. Case managers did not undertake financial assessments or manage budgets for their patients in the CMLTC service in any of the PCTs. Only half of the CMLTC services had written policies to allocate cases of different levels of need or complexity to different levels of case management and only four targeted their service at specific diseases such as chronic obstructive pulmonary disease and coronary heart disease. Several interviewees described cases being stepped down to a ‘maintenance level’ rather than discharged, a low tier approach to monitoring shared with other primary care colleagues.
Demographic and diagnostic characteristics of cohort sample
The proportion of the total eligible patients (n = 867) added to the CMLTC caseloads in the 10 PCTs varied widely ranging from 4.1% to 44.3%. Analysis is therefore based on the combined PCT results. The majority of patients were white (88%), female (63%) and over 75 years of age (65%; Table 2). Around half of the sample resided in the most deprived area of the locality (49%), measured by the Index of Multiple Deprivation (Department of Environment, Transport and the Regions, 2000). The most prevalent primary diagnoses were: (1) ‘symptoms, signs and abnormal clinical and laboratory findings, not elsewhere classified’ (37%); (2) ‘diseases of the circulatory system’ (28%), (3) ‘diseases of the respiratory system’ (26%); (4) ‘diseases of the digestive system’ (19%); and (5) ‘injury, poising and certain other consequences of external causes’ (18%).
Table 2 Patient characteristics: demographics and diagnosis (n = 867)
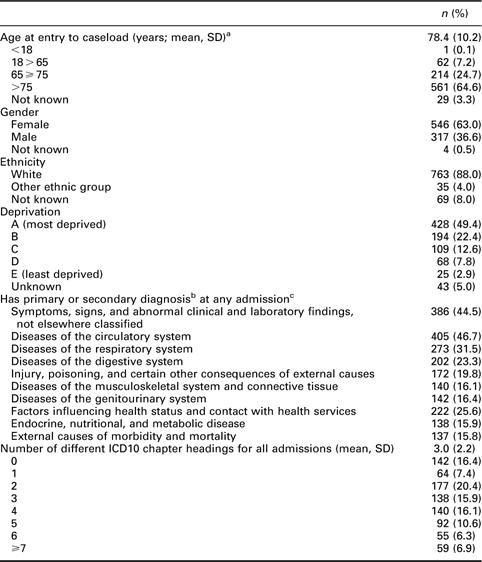
an = 838 (age not known; n = 29).
bICD10 chapter headings (WHO, 2007; only categories >10% are reported).
cDiagnosis refers to those recorded for all admissions.
Service utilisation
The use of hospital services in the nine months before the CMLTC intervention and nine months after entry was compared in the paired sample of 867 cases. Table 3 indicates that the mean number of hospital admissions and the mean LOS for all admissions reduced significantly at 1%. A similar pattern of results was detected in the number of emergency admissions and associated LOS (mean reduction of 0.3 for emergency hospital admissions and 2.9 days in LOS for emergency admissions). This was also reflected in significant reductions in tariffs. Table 4 shows that this pattern is also reflected in the recorded methods of admissions, highlighted in particular by a reduced proportion of accident and emergency department admissions. Table 5 maps the actual transitions between the before and after nine months categories in numbers of admissions and associated LOS (note the row percentages). It reveals a complex pattern of both increases and decreases of admissions after addition to a CMLTC caseload. Naturally, the majority of those without any prior hospital spells also did not experience any after being added to a CMLTC caseload. However, it is clearly noticeable that the overall pattern is that of a gradual shift towards fewer and shorter hospital spells with typically between 50% and 70% of patients changing to a lower category. This downward shift is more pronounced for emergency admissions.
Table 3 Hospital admissions and LOS in the nine months before and after addition to a CMLTC caseload (n = 867)

LOS = length of stay; CMLTC = case management for long-term conditions.
aWilcoxon signed-ranks test on nine months before and after figures (paired design).
bCalculated using the Payment by Results tariff for 2007–08.
cThe number of emergency admissions was calculated using the information about method of admission which was recorded for each hospital admission. Emergency admissions include those made through an accident and emergency (A&E) department; requested by a general practitioner; those which may have been requested by a bed bureau or a consultant clinic; or transferred through the A&E department of another provider.
Table 4 Method of admission (⩾1 range, n = 867)

A&E; accident and emergency department; GP = general practitioner.
aThese figures indicate the proportions of patients with at least one admission per category and cannot be summed vertically.
Table 5 Transitions in hospital admissions and associated LOS between nine months before and after addition to a CMLTC caseload

LOS = length of stay; CMLTC = case management for long-term conditions.
The relationship between service utilisation and case management
Of the two outcome variables of interest, the one that was most suited for regression analysis was LOS. Table 6 summarises the best ANCOVA model that could be fitted for LOS change in LOS for emergency admissions. Nine cases with unusually large positive changes were omitted from this analysis due to their strong biasing influence on other coefficients and model fit. Although we do not consider these cases to be incorrect, we acknowledge that this model cannot predict extremely large positive changes on the basis of the available information.
Table 6 ANOVA: change in LOS for emergency admissions

ANOVA = analysis of covariance; LOS = length of stay; CMLTC = case management for long-term conditions.
a95% CI for regression coefficients are calculated as ±1.96 times the SE.
bInterpretation of P-values: the probability of observing an effect of this magnitude if the null-hypothesis is correct (ie, there is no effect on LOS).
cA negative coefficient is associated with a reduction in LOS for emergency admissions.
The model predicts approximately 74% of the outcome variation in our sample (adjusted R 2). However, it must be stressed that the contribution of each individual predictor towards the overall model fit was very unequal, as highlighted by the additional column showing the partial sum of squares statistic (ie, each predictor's independent contribution to the model). The baseline measure (pre-CMLTC service entry), representing one half of the dependent variable (t2–t1), naturally accounts for the lion's share of the predictive quality of this model. Its strong negative coefficient signifies, and controls for, the inevitable effect of regression towards the mean. For each day spent in hospital before CMLTC service entry, patients are predicted to experience a reduction of nearly one day after. A large proportion of patients with pre-CMLTC emergency admissions had no further ones after service entry. It is expected that over time patients with many admissions show marked improvement. This imposes a negative relationship between baseline and the outcome measure, in addition to any potential effects of CMLTC. Conversely, a nearly equal share of the sample shows an increase in LOS for emergency admissions. Apart from low scores before service entry, which is another potential example of regression towards the mean, the number of primary and secondary diagnoses is the main contributor towards explaining increases in LOS for emergency admissions. Each added diagnosis is associated with a 2.4 day increase in LOS, everything else being equal.
A number of dummy-coded specific diagnoses have further negative or positive impacts on LOS. Detection of malignant neoplasms, for instance, is associated with a marked reduction in LOS. It should be noted that these indicators are related to relatively basic summary categories of types of diagnoses. A more detailed analysis might reveal better interpretable causal pathways from diagnoses to associated reductions or increases in the outcome measure.
The only CMLTC variable included is one of several which could produce comparable negative impacts (and corresponding positive impacts, depending on how a quality characteristic is operationalised and coded). These included ‘whether the CMLTC service is aimed at improving the extent and scope of services’ and ‘whether the PCT operates a computerised client-record system’. However, these very selective impacts of PCT characteristics as identified in questionnaire responses also appear to be a reflection of three geographical groups within the Greater Manchester area (ie, there are groups of neighbouring PCTs that, if pooled together, show either small negative, positive or neutral effects on the outcome variable while controlling for all other predictors). The design of this study does not permit us to go beyond speculation at this point. It may well be that these effects hint at unknown structural factors that are in some way associated with the local areas and/or their populations. In any case, these speculative influences, or the observable CMLTC influences for that matter, contribute only little to our ability to predict changes in service utilisation.
Several technical comments must accompany the model presented in Table 6. Although it proved fairly robust to a number of changes in its operationalisation, the actual size of coefficients should not be overrated, as they do to some extent depend on the aforementioned decisions of model fitting (in particular the exclusion of ‘outliers’), and are subject to sampling variation. Instead, one should merely acknowledge that there are fairly robust positive and negative influences on the outcome measure (eg, when ANCOVA and change-score analysis are compared for subsets of the sample). Furthermore, the inclusion of specific diagnoses and other potential predictors is ultimately dependent on the sample size and the chosen significance level. In other words, it is likely that a bigger sample would have produced more statistically significant predictors, which in this case were rejected on the basis that their P-values were just above 5%. On the other hand, the comparison of conventional and robust standard errors does raise the possibility that two predictors (infectious or parasitic diseases and mental and behavioural disorder) are somewhat dependent on outliers. However, most robust standard errors do not differ from their conventional equivalents. This indicates that the model's non-normal error distribution – stemming from the fact that negative changes are better predicted than positive ones – does not undermine its statistical validity.
Discussion
Methodological issues
This study utilised a unique mix of primary and secondary care data and presented a number of methodological challenges, three of which are particularly relevant to the findings. First, along with comparable studies (Roland et al., Reference Roland, Dusjeiko, Gravelle and Parker2005; Gravelle et al., Reference Gravelle, Dusheiko, Sheaff, Sargent, Boaden, Pickard, Parker and Roland2006; Patrick et al., Reference Patrick, Roberts, Hutt, Hewitt, Connelly and Oliver2006) it has relied on processes of data extraction by Acute and Foundation Trusts in relation to hospital admissions. Although organisations involved in these data flows seek to encourage and facilitate the submission of complete and valid data and to reduce the possibility of any inaccuracies, some shortcomings will inevitably remain. It is also worth noting that our more robust findings related to number of emergency bed days rather than the number of emergency admissions. This is consistent with the former being used as an indicator of unplanned use of acute care resources by the Health Care Commission when compiling their national targets.
Second, the lack of a control group, simultaneously experiencing the same level of health status as patients in the intervention, is a potential source of bias, although an inevitable consequence of its design as an observational study in which each individual patient acted as their own control through time. Consequently, direct attribution of the reductions in mean number of emergency hospital admissions and associated mean LOS to the effect of the CMLTC intervention is not possible without a number of caveats. The study risks the effect of regression to the mean on indicators such as hospital admission and LOS. Since patients were identified precisely because of their high hospital utilisation before CMLTC service entry it could be argued that any reduction in hospital utilisation is attributable to a reduction in their health problems from that peak. This has previously been found to be the case in an analysis of Hospital Episode Statistics data relating to frail older people (Roland et al., Reference Roland, Dusjeiko, Gravelle and Parker2005). This becomes a methodological problem in the context of an ANCOVA model if groups of interest are associated with different levels of baseline values (which is likely in the absence of randomisation), thus causing them to regress towards different means and making it more difficult to isolate the actual group effect in the model (Wright, Reference Wright2006).
Third, contrary to our original intention, we were unable to include in our analysis individual patient level data relating to the amount and intensity of the CMLTC intervention. Although hospital and case management data were available on a linked basis, community health data systems were not sufficiently developed in the majority of PCTs to permit indicators of the amount and intensity of CMLTC input to be used. Another potential source of bias was the decision to include only those patients with a full nine-month follow-up data in the analysis and thus exclude those who had died during the nine months following CMLTC service entry. However, other analyses of the data suggested that exclusion of this group made no difference to the overall hospital utilisation rate. Indeed, another study has indicated that including patients who had died is likely to increase the difference in admissions or LOS between time periods (Huws et al., Reference Huws, Cashmore, Newcombe, Roberts, Vincent and Elwyn2008), and thus our results are likely to be more conservative.
Case management arrangements
Our results suggest that the organisation of case management in the 10 PCTs showed both similarities and differences. In terms of both organisational and process measures links between all the CMLTC services and their local authority adult social care services were poorly developed. As noted elsewhere it is important that CMLTC services become embedded in the network of partner organisations (Abell et al., Reference Abell, Hughes, Reilly, Berzins and Challis2010). Caseload size, however, proved to be a marker of variation with only one PCT having caseloads at 80, the level recommended by policy (Department of Health, 2005b). Moreover, this concealed considerable variations in definition over what constituted an active or an inactive or maintained case. Some PCTs addressed this by having a clear two-tier system of active and maintenance within caseloads. Clearly, effective case management of highly vulnerable people is only possible with manageable caseloads (Challis, Reference Challis2003) and a variety of approaches can contribute to this. One strategy is to have clearly designed systems for step down of patients to less-intensive modes of support. Von Korff and Tiemens (Reference Von Korff and Tiemens2000) have described three major assumptions of stepped-care models that can be applied here: different people require different levels of care; finding the best level of care depends on monitoring outcomes; and moving from lower to higher levels of care based on observed outcomes can increase effectiveness while lowering overall costs. Furthermore, although stepped-care principles are guided by outcomes, they can be tailored to patient preferences and initial clinical complexity and severity, so the first line of treatment is not always the least expensive and least intensive.
Service utilisation
Four findings merit further discussion. First, comparing the pattern of hospital utilisation between the nine-month period before and after CMLTC service entry, the mean number of all and emergency hospital admissions and mean LOS were reduced. Unsurprisingly, it was found that case complexity measured by the number of diagnostic categories present was associated with a greater number of emergency admissions and greater LOS. Indeed, this definition of clinical complexity, which is akin to the concept of frailty as identified by Rockwood et al. (Reference Rockwood, Andrew and Mitnitski2007), is indicated by a quarter of the sample having five or more primary or secondary diagnoses. Clearly, targeting this group with multiple problems should be considered a priority. However, services also need to target patients who are neither too low a risk of hospitalisation to have positive service outcomes within a desired time period, nor so seriously ill that it is too late for such interventions to prevent hospital admissions (Peikes et al., Reference Peikes, Chen, Schore and Brown2009). Thus, efficient methods are required to determine which patients are likely to benefit from which levels of case management support and at what times (Bayliss et al., Reference Bayliss, Edwards, Steiner and Main2008). Despite the investment of time and resources in a variety of case-finding methodologies, it appeared that a considerable proportion (38%) of people identified for case management did not have an episode of hospital care in the nine-month period before CMLTC service entry. It is possible that further work on the process of caseload management, that is, improved targeting and case finding and regular review of cases and caseloads, would ensure that those selected for case management are those who are most likely to benefit (Challis and Davies, Reference Challis and Davies1986; Challis et al., Reference Challis, Darton, Johnson, Stone and Traske1995).
Second, the most powerful predictor of emergency hospital admissions within nine months from CMLTC service entry was prior admissions. This is consistent with the attempts to reduce readmissions in patients by focusing on those with prior recent admissions. However, it does not constitute definitive evidence that the reduction is attributable to CMLTC. This was the premise upon which much of the long-term conditions policy in England (Department of Health, 2005b) was founded. As we discuss below, any measure of impact of this kind in a non-randomised trial risks the effect of regression towards the mean being the major cause of changes in both hospital admissions and LOS and confounding the expectedly smaller effects of CMLTC configurations. Indeed, defying the policy objective, most cases without any or with few admissions experienced an increase in admissions and corresponding LOS, while a certain proportion of frequent service users with particular diagnoses also experienced an increase or remained at a high level. This is consistent with a systematic review and meta-regression assessing the use of intensive case management to reduce time in hospital for people with severe mental illness (Burns et al., Reference Burns, Catty, Dash, Roberts, Lockwood and Marshall2007). Nonetheless, and related to this observation, the number of patients who had no recorded LOS (as they were admitted and discharged on the same day of an admission) rose in the post-nine-month cohort. This could be inferred to suggest that the process of preventing admissions was having some effect under case management with efforts being made by hospitals to discharge case-managed patients on the same day if possible.
Third, a very modest effect was shown with regard to CMLTC features suggesting possibly the benefit of good communication between CMLTC and hospital services. We discuss two possible methodological reasons why this may have been so. Although this multi-site study benefited from a fairly large patient sample size, comparable with other studies of the same initiative (Patrick et al., Reference Patrick, Roberts, Hutt, Hewitt, Connelly and Oliver2006), variation in the form and type of case management provided by the 10 PCTs in Greater Manchester was less than that which would have been found in a sample of non-interconnected trusts. This was a deliberate policy arising from the commitment of The Association of Greater Manchester PCTs to work together and develop common approaches to implementation. This perspective clearly enabled more coherent regional development and permitted concerted approaches to roll out from a group of PCTs in relation to such matters as workforce and case management. In addition, the variation in the sample size of the patient from different PCTs could in part explain the lack of variation. For example, the majority of cases included in the final cohort sample were from two PCTs (40% and 22%). This was in part, due to the different timescales of the commencement of each CMLTC service (which varied from January 2005 to June 2006) and our need to obtain nine-month follow-up data before our data extraction cut-off date. Thus, CMLTC services that started later were less well represented in the final analysis. It is possible that this may have attenuated any effect attributable to different forms of case management arrangements.
Fourth, after pooling PCTs a slight clustering of effects was observed whereby geographically adjacent PCTs appeared to share both CMLTC characteristics and changes in the LOS related to emergency admissions. However, further investigation of wider phenomena that could be connected to this may be useful, as the local context could well be confounding the suspected effects of case management. Clearly, whichever model is implemented, it does not stand alone, but it rests within the existing network of local healthcare and social-care providers and in part its ‘success or failure’ is related to this local environment (Abell et al., Reference Abell, Hughes, Reilly, Berzins and Challis2010).
Conclusion
In conclusion, it must be remembered that the design of the study deliberately replicates the policy model whereby patients are identified through high hospital utilisation using tools such asPARR (Billings et al., Reference Billings, Dixon, Mijanovich and Wennberg2006). Nonetheless, only with a properly designed RCT would it be possible to identify the size of the effect of CMLTC on the use of hospital resources. The methodology used in this study does, however, offer some benefits in relation to other sources of evidence. The follow-up period used to asses the impact of case management was nine months, rather than shorter periods used in other studies (eg, Patrick et al., Reference Patrick, Roberts, Hutt, Hewitt, Connelly and Oliver2006) and the data were also available at the individual patient level thereby permitting further exploration of patient-level characteristics upon outcome rather than relying upon aggregates (Gravelle et al., Reference Gravelle, Dusheiko, Sheaff, Sargent, Boaden, Pickard, Parker and Roland2006).
Clearly, the findings of this study are exploratory and cannot be definitive. There is a clear need for adequately funded and methodologically robust evaluations to look at the effects of CMLTC, including the relative cost effectiveness of different approaches and involving the perspectives of patients, carers and healthcare staff and management. Any case management intervention needs to be part of a wider system such as a collaborative care approach (Von Korff et al., Reference Von Korff, Gruman, Schaefer, Curry and Wagner1997) or a model such as the Chronic Illness Model which originated in the United States but provides a back drop for the recent policy in England (Department of Health, 2005a). The latter identifies changes in the healthcare systems that help practices in primary care settings improve outcomes among patients with chronic illnesses. It has six components: community resources; patient self-management; decision support; delivery system redesign (including case management); the use of clinical information systems; and the healthcare system (Bodenheimer et al., Reference Bodenheimer, Wagner and Grumbach2002; Shortell et al., Reference Shortell, Marsteller, Lin, Pearson, Wu, Mendel, Cretin and Rosen2004). Case management also needs an internal logical coherence between models, resources and outcome (Challis, Reference Challis2003). These other elements add further layers of complexity to any evaluation. Ideally, an appropriate design would be an RCT that would permit the evaluation to address effects such as that of variations in targeting. Such a strategy in England would seem unlikely given the national roll out of the policy. Hence, in the absence of such a robust RCT, there remains a need to explore further the costs, process and outcomes of CMLTC programmes with wider, more diverse PCT representation, adopting a longitudinal approach with more extensive and detailed outcome and process data collected through local healthcare and social-care systems. Coulter (Reference Coulter1991) proposed six categories of disease and treatment outcomes: death, disease, physical well-being, psychological well-being, social well-being and quality of life. Furthermore, ideally, health outcomes should include both the patient and carer experience and well-being (Hunt et al., Reference Hunt, McEwan and McKenna1985; Goldberg, Reference Goldberg1992; Picker Institute, 2008); quality of care (Challis et al., Reference Challis, Darton, Johnson, Stone and Traske1995) service content (changes in nature and content of care plans; Sutcliffe et al., Reference Sutcliffe, Hughes, Abendstern, Clarkson and Challis2008); as well as resource use such as service receipt. This would permit greater exploration of the implications of differences in CMLTC systems and processes as well as making closer linkages between the activities undertaken by case managers and the service-level outcomes experienced by patients.
This study integrates primary data sources such as the style and type of CMLTC with routinely collected NHS data at the patient level for people in receipt of CMLTC. As yet most other studies in this area of research have not been able to examine significant amounts of data at the individual patient level. The capacity to link these different sources of NHS data in a pseudonymised fashion suitable for research purposes was only possible due to the recent development of the TIS by the NHS Northwest. The future opportunities that such data sets present to examine the relationship between variations in health service utilisation and outcomes are immense.
Acknowledgements
This study was undertaken by the Personal Social Services Research Unit, which receives funding from the Department of Health who supported the study. The views expressed in the publication are those of the authors and not necessarily those of the Department of Health. The authors also appreciate the support of the Tactical Information Service and generous assistance of John McGovern who facilitated the transfer of NHS pseudonymised data. They are also very grateful to staff within the Greater Manchester PCTs for their participation in the study.