Introduction
Mental disorders have consistently been associated with substance use – in particular cigarette smoking, alcohol drinking, cannabis use, and consumption of caffeinated drinks. Compared to the general population, individuals diagnosed with a mental disorder – or subclinical symptoms – are more likely to smoke (Garey et al., Reference Garey, Olofsson, Garza, Shepherd, Smit and Zvolensky2020), drink alcohol excessively (Stephen Rich & Martin, Reference Stephen Rich, Martin, Sullivan and Pfefferbaum2014), and use cannabis (Satre, Bahorik, Zaman, & Ramo, Reference Satre, Bahorik, Zaman and Ramo2018). For caffeine, there are conflicting findings with high(er) consumption being associated with a lower odds of depression (Grosso, Micek, Castellano, Pajak, & Galvano, Reference Grosso, Micek, Castellano, Pajak and Galvano2016) but a higher odds of schizophrenia (Williams & Gandhi, Reference Williams and Gandhi2008). A key factor in mental disorders is cognitive functioning, the majority of patients suffering from deficits in attention, learning and/or memory (Nieman et al., Reference Nieman, Chavez-Baldini, Vulink, Smit, Van Wingen, De Koning and Denys2020). In non-clinical populations, poor cognitive functioning has been associated with increased smoking (Campos, Serebrisky, & Castaldelli-Maia, Reference Campos, Serebrisky and Castaldelli-Maia2016), alcohol drinking (Topiwala & Ebmeier, Reference Topiwala and Ebmeier2018), and cannabis use (Curran et al., Reference Curran, Freeman, Mokrysz, Lewis, Morgan and Parsons2016), although for impaired response inhibition specifically there are contradicting findings (Liu et al., Reference Liu, van den Wildenberg, de Graaf, Ames, Baldacchino, Bø and Wiers2019b). Although caffeine is often thought to have acute beneficial effects on cognition (Irwin, Khalesi, Desbrow, & McCartney, Reference Irwin, Khalesi, Desbrow and McCartney2020), there is evidence that contests this (Galindo, Navarro, & Cavas, Reference Galindo, Navarro and Cavas2020; Weibel et al., Reference Weibel, Lin, Landolt, Garbazza, Kolodyazhniy, Kistler and Reichert2020) and its long(er) term effects remain unclear (Cornelis, Weintraub, & Morris, Reference Cornelis, Weintraub and Morris2020; Panza et al., Reference Panza, Solfrizzi, Barulli, Bonfiglio, Guerra, Osella and Logroscino2015).
To properly inform public health policy it is crucial to understand the mechanisms underlying associations between poor mental health and substance use. Typically, a distinction is made between three, not mutually exclusive, mechanisms: (1) shared risk factors, (2) causal effects where poor mental health increases substance use, and (3) causal effects where substance use negatively affects mental health. As for mechanism 1, important non-genetic shared risk factors are the death of a loved one (Keyes et al., Reference Keyes, Pratt, Galea, McLaughlin, Koenen and Shear2014) or (other) childhood trauma (Setién-Suero et al., Reference Setién-Suero, Suárez-Pinilla, Ferro, Tabarés-Seisdedos, Crespo-Facorro and Ayesa-Arriola2020). Although note that these seemingly environmental factors might have a heritable component (Sallis et al., Reference Sallis, Croft, Havdahl, Jones, Dunn, Davey Smith and Munafò2020). Poor mental health and substance use are substantially heritable and there is evidence for considerable genetic correlation (Abdellaoui, Smit, van den Brink, Denys, & Verweij, Reference Abdellaoui, Smit, van den Brink, Denys and Verweij2020; Vink & Schellekens, Reference Vink and Schellekens2018). However, genetic correlations can also reflect causal relationships. If trait 1 causally affects trait 2, then genetic variants predictive of trait 1 will, indirectly, also predict trait 2 (Kraft, Chen, & Lindström, Reference Kraft, Chen and Lindström2020).
We review evidence from studies that applied ‘Mendelian randomization’ (MR) (Davies, Holmes, & Davey Smith, Reference Davies, Holmes and Davey Smith2018b; Lawlor, Harbord, Sterne, Timpson, & Davey Smith, Reference Lawlor, Harbord, Sterne, Timpson and Davey Smith2008) to assess causal effects between poor mental health and substance use. When we talk about a true causal effect (e.g. A is causal for B), we imply that if A were to be altered this would lead B to change accordingly. To some extent, MR is analogous to a randomized controlled trial (RCT). Instead of participants being assigned to experimental conditions, MR compares subgroups in the population which are at differing levels of genetic risk for a proposed risk factor. We include MR studies that look at cigarette smoking, alcohol drinking, cannabis use, and/or caffeine consumption in relation to (symptoms of) a mental health disorder or cognitive functioning. Below, we briefly discuss epidemiological and (human) experimental evidence on these relationships and then introduce MR.
Epidemiological evidence
Causal inference can be attempted by looking at the temporal nature of relationships. For smoking, there is extensive longitudinal evidence that depression (Audrain-McGovern, Leventhal, & Strong, Reference Audrain-McGovern, Leventhal and Strong2015; Mathew, Hogarth, Leventhal, Cook, & Hitsman, Reference Mathew, Hogarth, Leventhal, Cook and Hitsman2017) and attention-deficit hyperactivity disorder (ADHD) (van Amsterdam, van der Velde, Schulte, & van den Brink, Reference van Amsterdam, van der Velde, Schulte and van den Brink2018) are associated with increased odds of smoking initiation and persistence. In the other direction – from smoking to mental health – a systematic review study including 26 studies with a follow-up of between seven weeks and nine years concluded that smoking cessation is followed by reduced depression, anxiety, and stress (Taylor et al., Reference Taylor, McNeill, Girling, Farley, Lindson-Hawley and Aveyard2014b). Smoking has also been associated with poorer cognitive performance, which improved after cessation (Vermeulen et al., Reference Vermeulen, Schirmbeck, Blankers, Van Tricht, Bruggeman, Van Den Brink and Van Winkel2018).
For alcohol, a review of 37 longitudinal studies found that (symptoms of) mental disorders in childhood predict an increased odds of alcohol dependence later on in life (Groenman, Janssen, & Oosterlaan, Reference Groenman, Janssen and Oosterlaan2017). In the other direction, alcohol dependence and heavy drinking predicted subsequent increases in depressive symptoms, but for heavy drinking, this association did not persist after adjustment for confounders (Li et al., Reference Li, Wang, Li, Shen, Li, Zhang and Peng2020). A systematic review of alcohol interventions reported that alcohol reduction led to a lower prevalence of psychiatric episodes, and improvement of anxiety and depressive symptoms, self-confidence, and mental quality of life (Charlet & Heinz, Reference Charlet and Heinz2017).
For cannabis use, the few available studies are smaller and the evidence is mixed. A 10-year prospective cohort study in 1395 adolescents found that symptoms of mental disorders (depression, bipolar, and anxiety disorder) increase the odds of cannabis initiation and cannabis use disorder (Wittchen et al., Reference Wittchen, Fröhlich, Behrendt, Günther, Rehm, Zimmermann and Perkonigg2007). There was no indication that cannabis causes elevated anxiety symptoms (Twomey, Reference Twomey2017), but substantial evidence to support that it increases the risk of manic symptoms (Gibbs et al., Reference Gibbs, Winsper, Marwaha, Gilbert, Broome and Singh2015) and psychosis (Gage, Hickman, & Zammit, Reference Gage, Hickman and Zammit2016a). Another study found evidence that cannabis can be beneficial for post-traumatic stress disorder but is associated with short-term cognitive deficits (Walsh et al., Reference Walsh, Gonzalez, Crosby, S. Thiessen, Carroll and Bonn-Miller2017).
For caffeine, research has focussed predominately on cognitive functioning or sleep. The largest available systematic review, including 28 studies, concluded that there is some evidence that caffeine is protective against cognitive decline (Panza et al., Reference Panza, Solfrizzi, Barulli, Bonfiglio, Guerra, Osella and Logroscino2015). Despite the fact that caffeine has stimulating properties which are thought to interfere with sleep acutely, a cohort study in 26 305 adolescents with a follow-up of 4 years found no association between average daily caffeine consumption and sleep duration (Patte, Qian, & Leatherdale, Reference Patte, Qian and Leatherdale2018).
Combined, the current epidemiological literature points topotential bi-directional effects between mental health and substance use. However, there are important methodological limitations to consider. First, there may be bias from confounders that were not included in the analysis or measured with considerable error (Gage, Munafò, & Davey Smith, Reference Gage, Munafò and Davey Smith2016b). Second, reverse causality, where the outcome variable or a precursor of the outcome variable has affected the exposure, can induce spurious associations (Gage et al., Reference Gage, Munafò and Davey Smith2016).
Family-based studies are better suited for causal inference. Most notable are twin methods. Because monozygotic and dizygotic twins share 100% of their family environment and 100% or 50% of their genetic make-up, respectively, causality can be inferred by looking at within-twin pair differences. For instance, differences in ADHD symptoms were associated with differential progression to daily smoking, cigarettes per day and nicotine dependence in female monozygotic twin pairs, indicating that ADHD causally impacts smoking (Elkins et al., Reference Elkins, Saunders, Malone, Keyes, Samek, McGue and Iacono2018). A study that identified monozygotic twin pairs who were discordant for smoking (one smoked, the other did not), found evidence suggesting that smoking can also causally increase ADHD symptoms (Treur et al., Reference Treur, Willemsen, Bartels, Geels, van Beek, Huppertz and Vink2015). However, twin methods also have important limitations – there may be bias from confounders that led twins to differ on the exposure as well as on the outcome of interest, and reverse causation cannot be ruled out (McGue, Osler, & Christensen, Reference McGue, Osler and Christensen2010).
Experimental evidence from human studies
Experimentally induced stress increased the perceived value of cigarettes in smokers with depressive symptoms (Dahne, Murphy, & MacPherson, Reference Dahne, Murphy and MacPherson2017). Similarly, when tested after overnight sleep deprivation smokers were more inclined to pick cigarettes over money than when they were tested after a normal night's sleep (Hamidovic & de Wit, Reference Hamidovic and de Wit2009). In the other direction, a meta-analysis of 35 clinical trials concluded that participants who were randomly assigned to use nicotine patches to quit smoking experienced more sleep problems than participants assigned not to use them (Greenland, Satterfield, & Lanes, Reference Greenland, Satterfield and Lanes1998). After randomly assigning 31 smokers to continue smoking and 33 smokers to quit, anxiety and depressive symptoms decreased (more) in the latter group during 3 months follow-up (Dawkins, Powell, Pickering, Powell, & West, Reference Dawkins, Powell, Pickering, Powell and West2009).
Among 540 participants randomly assigned to receive different types of treatment for depression there were significant treatment effects on depressive symptoms, but no changes in alcohol consumption (Strid, Hallgren, Forsell, Kraepelien, & Öjehagen, Reference Strid, Hallgren, Forsell, Kraepelien and Öjehagen2019). A considerable amount of work has focussed on cognitive behavioral therapy (CBT) to reduce alcohol consumption. A systematic review including eight RCTs concluded that CBT reduced alcohol use and depressive and/or anxiety symptoms, even when CBT targeted alcohol only (Baker, Thornton, Hiles, Hides, & Lubman, Reference Baker, Thornton, Hiles, Hides and Lubman2012). This could mean that decreases in alcohol use led to improvements in mental health, or that, though not targeted to it specifically, CBT affected depressive/anxiety symptoms.
As reflected in the work described here, only a limited number of causal questions can be answered with experimental designs. Moreover, these questions mostly relate to (relatively) short-term effects. Longer-term effects – for instance, potential effects of prolonged smoking on being diagnosed with a mental disorder, or the impact of lifetime alcohol use on the cognitive decline – cannot be investigated. There are also obvious ethical restrictions; it would not be acceptable to randomize people to initiate or increase their use of an addictive substance.
Mendelian randomization
MR has the potential to overcome (some of) the limitations of traditional epidemiological and experimental methods. We will explain MR's rationale by using one specific research question: does smoking (the ‘exposure’ of interest) causally impact depressive symptoms (the ‘outcome’ of interest)? As is the case for practically all human traits (Polderman et al., Reference Polderman, Benyamin, De Leeuw, Sullivan, Van Bochoven, Visscher and Posthuma2015), individual differences in smoking can partly be explained by genetic differences (Vink, Willemsen, & Boomsma, Reference Vink, Willemsen and Boomsma2005). Genetic variants robustly associated with smoking have been identified through genome-wide association studies (GWAS) – the most notable variants in nicotinic receptor genes (Liu et al., Reference Liu, Jiang, Wedow, Li, Brazel, Chen and Vrieze2019a). Because the transmission of genetic variants from parents to offspring occurs randomly (Mendel's second law – ‘The law of independent assortment’), there should be minimal bias from confounders and subgroups of differing genetic risk can be thought of as RCT treatment groups. To determine whether smoking causally affects depression, we take genetic variants robustly associated with smoking and test if these also predict higher levels of depressive symptoms. The genetic variants act as proxies for measured smoking behavior, or instrumental variables (Davies et al., Reference Davies, Holmes and Davey Smith2018b). The most commonly used genetic variants are Single Nucleotide polymorphisms (SNPs). MR provides unbiased results if three assumptions are met: (1) the SNPs used as instrumental variables – together referred to as the ‘genetic instrument’ – are robustly associated with the exposure, (2) the genetic instrument is not directly associated with confounders and (3) the genetic instrument is not directly associated with the outcome, apart from any causal effect running through the exposure variable (Fig. 1a).
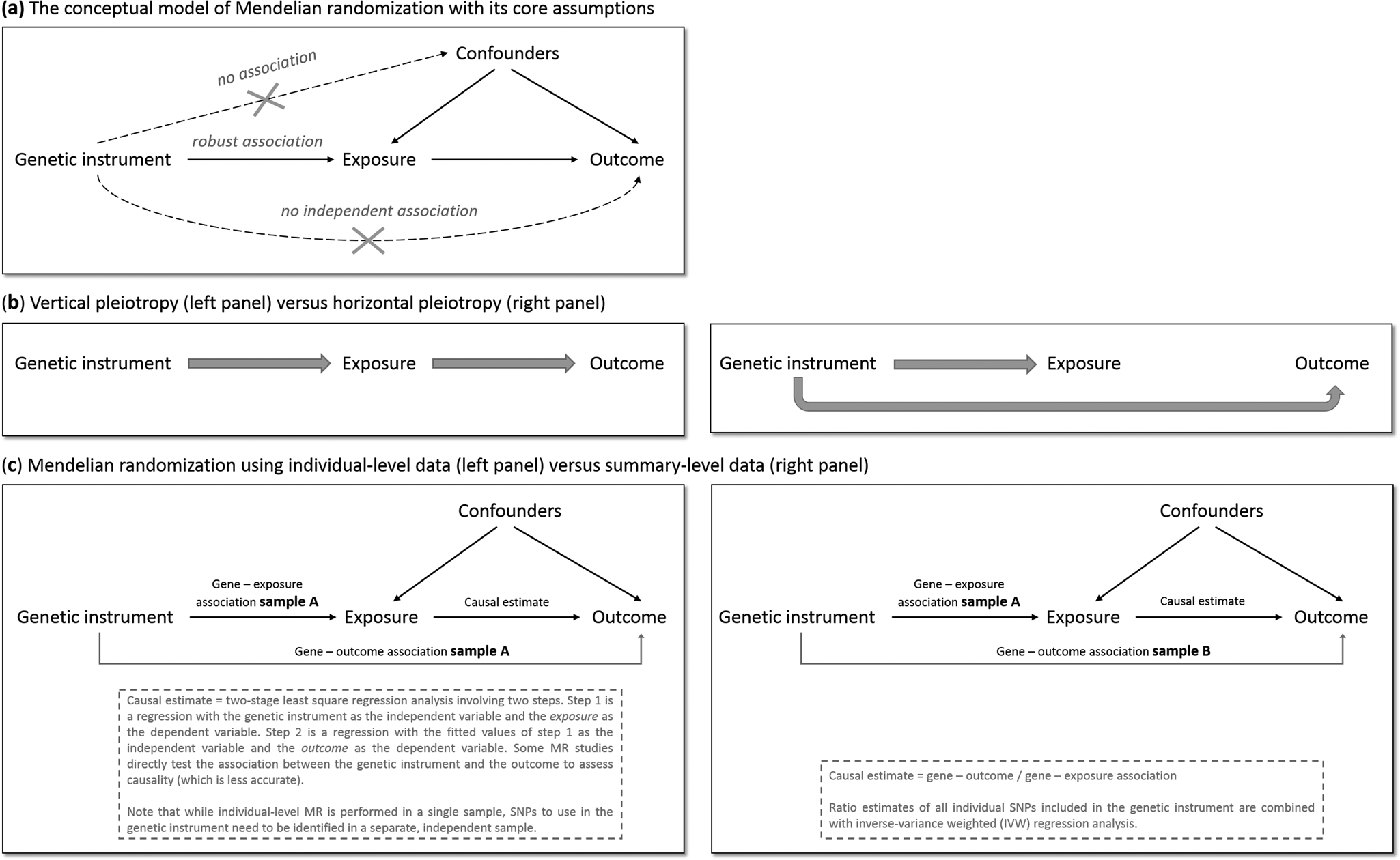
Fig. 1. The main principles of Mendelian randomization: (a) the conceptual model indicating the three core assumptions, (b) an illustration of vertical pleiotropy, that which causal inference is based on in a Mendelian randomization analysis, versus horizontal pleiotropy, which biases a Mendelian randomization analysis, and (c) an illustration of the framework and methods of Mendelian randomization using individual-level data versus summary-level data.
Since the second and third assumptions cannot be known or (exhaustively) tested, sensitivity analyses that assess the robustness of a causal finding are crucial. An important source of bias is pleiotropy, where a genetic variant affects multiple traits. Vertical pleiotropy (sometimes called mediated pleiotropy) occurs when a genetic variant affects the exposure and because of that indirectly also affects the outcome. This is not problematic and in fact is what an MR analysis aims to detect. Horizontal pleiotropy (sometimes referred to as biological pleiotropy) occurs when a genetic variant affects the outcome independently, not mediated through its effect on the exposure (Fig. 1b). This is problematic and could lead to bias.
There are two MR approaches: using individual-level data and using summary-level data from GWAS. Although MR using individual-level data requires a single data set of individuals with genotype data and information on both the exposure and outcome, MR using summary-level data takes summary estimates (i.e. the mean effect size for the genetic variants of interest) from separate GWAS for the exposure and the outcome. The two approaches use different methodology to estimate the causal effect (Burgess, Scott, Timpson, Davey Smith, & Thompson, Reference Burgess, Scott, Timpson, Davey Smith and Thompson2015; Burgess, Small, & Thompson, Reference Burgess, Small and Thompson2017) (Fig. 1c). MR using summary-level data has been the predominant method in recent years and currently has the most (powerful) sensitivity methods.
Methods
This study was pre-registered at PROSPERO (CRD42019133182; https://www.crd.york.ac.uk/prospero/display_record.php?RecordID=133182). We performed a literature search of Medline, EMBASE, PsycINFO, and Web of Science for published, peer-reviewed papers describing MR of one or more type(s) of substance use in combination with mental health (including diagnoses, subclinical symptoms, and cognitive functioning). We also performed a search of pre-print servers (bioRxiv, medRxiv, and arXiv). We restricted our search to English-language publications (search terms provided in Supplementary Methods). Similar to a recent, high-impact review (Firth et al., Reference Firth, Solmi, Wootton, Vancampfort, Schuch, Hoare and Stubbs2020), we designed our search to pick up studies that performed analyses referred to as ‘Mendelian randomization’ (or (very) closely related methods such as ‘genetic instrumental variable regression’ (DiPrete, Burik, & Koellinger, Reference DiPrete, Burik and Koellinger2018)). The final search was performed on 27 February 2020, and a final update of all papers (to incorporate transitions from pre-print to a newer pre-print version or published paper) on 12 April 2021.
We followed PRISMA guidelines in extracting and selecting the data and used a flowchart to document the stages of screening. After a deduplication step, two of the co-authors independently selected potentially eligible studies based on title and abstract, and if necessary in the following step, based on the full text. In case of disagreement between the two main reviewers, this was resolved through discussion with a third co-author.
Qualitative synthesis
The studies included in this review use a wide range of genetic instruments, phenotypes, and methods. This precluded us from formally combining effect estimates through meta-analysis. Instead, we extracted the most important information from each study, judged the quality based on an extensive set of predetermined criteria, and summarized our findings stratified on the addictive substance.
We developed a scoring system incorporating the factors most important to the validity of an MR study (Supplementary Table S1), based on our collective knowledge of MR and cross-checked with the most recent (still evolving) MR guidelines (Davey Smith et al., Reference Davey Smith, Davies, Dimou, Egger, Gallo, Golub and Yarmolinsky2019). Important indices of quality are phenotype measurement (sample size and quality of the exposure and outcome measurements) and instrument strength (p value threshold used to select genetic variants, number of genetic variants included, biological knowledge, F-statistic for instrument strength, % variance that the instrument explains). Taking all quality indices into consideration, each study was given a total score of ‘–’, ‘– +’or‘+’. We considered the total score based on a few key indicators that needed to be satisfied in order for the study to be considered sufficient (– + ), most notably: sufficient sample size and sufficient main analytical methods. When, on top of that, a study had used particularly extensive (sensitivity) methods, a total score of (+) was given. Two co-authors scored all studies independently and blind from each other, after which they compared their scores. In case of disagreement, a third co-author was consulted and together, all agreed on the final score.
Results
We identified 1464 potentially relevant records, of which 831 unique (Fig. 2). Of the final 63 studies included in qualitative synthesis, 40 investigated smoking, 24 investigated alcohol, 8 investigated cannabis, and 6 investigated caffeine (some investigated multiple substances; Table 1). The final quality rating was – for 16 studies, – + for 37 studies, and + for 10 studies (Supplementary Tables S2 and S3 for MR using individual-level and summary-level data, respectively). Note that some summary-level studies obtained genetic estimates from partly/largely the same data sets, either for the exposure alone or for both exposure and outcome. This is inherent to MR, as it requires robust, replicated estimates from the largest available GWAS. However, this means that the causal findings presented should not be regarded as (completely) independent. The importance of a particular study and its findings is determined not only on the basis of the data used, but also the quality of the analysis and, importantly, sensitivity methods. If two studies use (almost) exactly the same data sets for exposure and outcome, this is indicated in the text. For a more detailed comparison of data sets see Table 2.
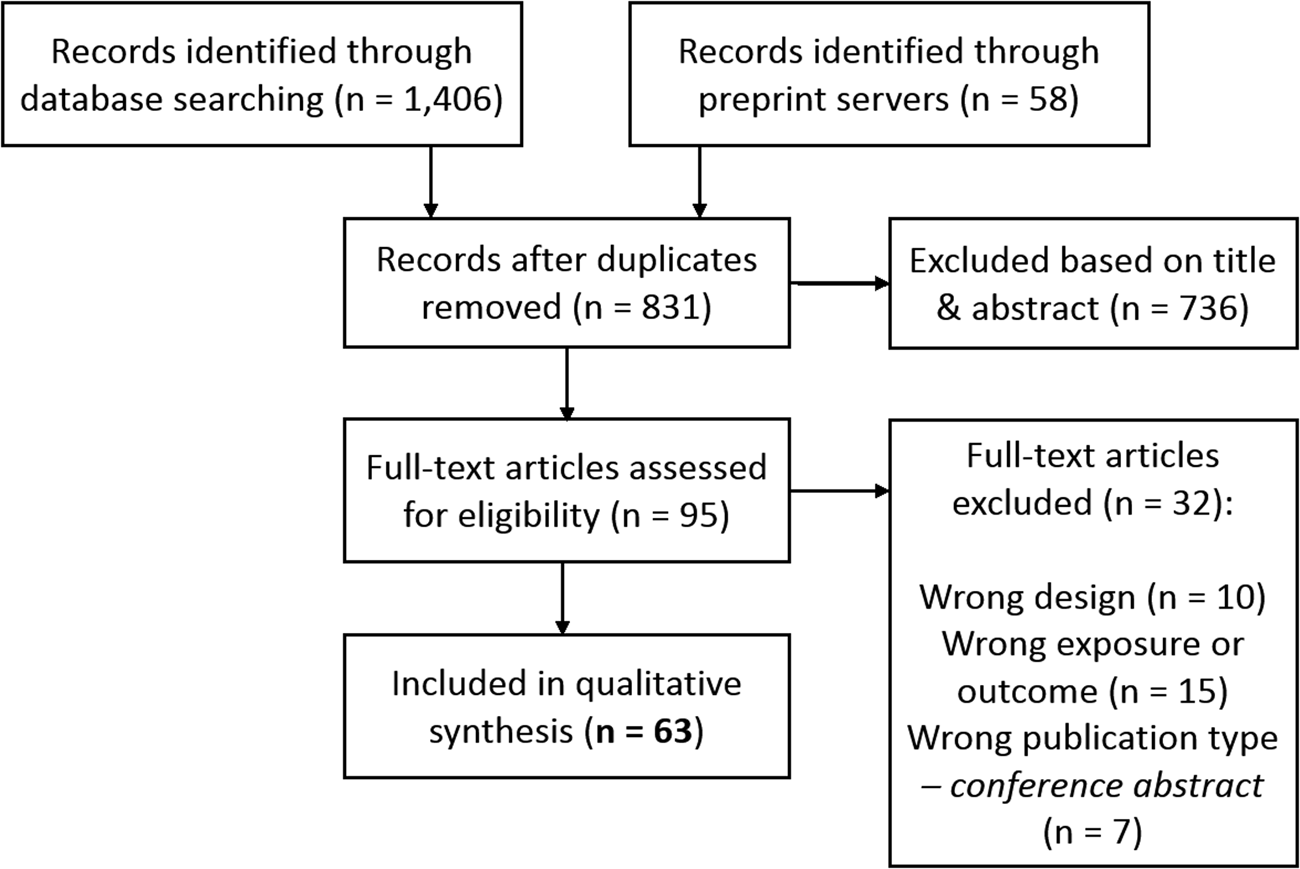
Fig. 2. PRISMA flow chart demonstrating the selection of articles to be included for qualitative synthesis.
Table 1. All Mendelian randomization (MR) studies included for qualitative synthesis, with their identifying information, description of the exposure and outcome variable(s), whether the study used individual-level and/or summary-level data, the total quality rating, and a brief summary of their findings

a This score pertains to the relationship that is of interest to the current systematic review, and not necessarily the whole study. For instance, it may be that in the study as a whole (more) extensive MR sensitivity methods were performed but for the causal estimate of interest no sensitivity methods were applied (e.g. when smoking is merely used as a mediator in a multivariable MR study).
b Pre-print publication (not peer-reviewed) obtained from bioRxiv.org, medRxiv.org or arXiv.org.
Note that the quality rating is based on a number of key indices, the most important being: phenotype measurement (sample size, quality of the exposure measurement, quality of the outcome measurement), instrument strength (p value threshold used to select genetic variants, number of genetic variants included, biological knowledge, F statistic for instrument strength, % variance that the instrument explains), and analytical factors (type of main analysis, whether or not basic sensitivity analyses were applied, whether or not additional sensitivity analyses were applied). Combined, these indices were weighted to come to a complete quality score (see Supplementary Table S1). A few important notes regarding this weighting of the evidence: (1) where absolute thresholds were used to judge the quality of a particular aspect of the study (e.g. sample size), it should be noted that these are somewhat arbitrary and were merely used to provide an indication of quality. (2) With regard to ‘phenotype measurement,’ a very well measured phenotype in a moderate sample size may be just as powerful as a more superficially measured phenotype in a very large sample. However, in case of very small sample sizes (e.g. n = 180 such as in the study by Irons et al., Reference Irons, McGue, Iacono and Oetting2007) even an extremely thoroughly measured phenotype will not lead to a high total score. (3) With regard to ‘instrument strength,’ when a study uses a single genetic variant that explains a relatively large amount of the variance and for which there is good biological knowledge, the fact that only one SNP was used is not necessarily problematic. For example, this is the case for SNP rs1051730 in the nicotinic acetylcholine receptor CHRNA5/A3/B4 gene cluster – each additional risk allele increases smoking heaviness with one additional cigarette smoked per day (Katikireddi, Green, Taylor, Davey Smith, and Munafò, Reference Katikireddi, Green, Taylor, Davey Smith and Munafò2018).
Table 2. All Mendelian randomization (MR) studies included for qualitative synthesis, with their identifying information, description of the data samples used for exposure and outcome variable(s), ancestry of those samples, the independence of the include SNPs, whether or not proxies were used, and whether or not a correction for multiple testing was applied

a Pre-print publication (not peer-reviewed) obtained from bioRxiv.org, medRxiv.org or arXiv.org.
Note that the complete references to the samples listed under ‘GWAS sample exposure variable(s)’ and ‘GWAS sample outcome variable(s)’ can be found in the original publications (1–63).
Cigarette smoking
Cognitive traits
There was consistent evidence that higher educational attainment decreases the odds of initiating smoking (Carter et al., Reference Carter, Gill, Davies, Taylor, Tillmann, Vaucher and Dehghan2019; Davies et al., Reference Davies, Dickson, Davey Smith, Windmeijer and van den Berg2018a; Davies et al., Reference Davies, Hill, Anderson, Sanderson, Deary and Smith2019; Ding, Barban, & Mills, Reference Ding, Barban and Mills2019; Gage, Bowden, Davey Smith, & Munafo, Reference Gage, Bowden, Davey Smith and Munafo2018; Sanderson, Davey Smith, Bowden, & Munafò, Reference Sanderson, Davey Smith, Bowden and Munafò2019; Tillmann et al., Reference Tillmann, Vaucher, Okbay, Pikhart, Peasey, Kubinova and Holmes2017; Zeng et al., Reference Zeng, Ntalla, Kessler, Kastrati, Erdmann, Danesh and Schunkert2019; Zhou et al., Reference Zhou, Zhang, Liu, Yang, Fang, Hong and Zhang2019a), increases the age at smoking initiation (Yuan, Xiong, Michaëlsson, Michaëlsson, & Larsson, Reference Yuan, Xiong, Michaëlsson, Michaëlsson and Larsson2020a; Zhou et al., Reference Zhou, Zhang, Liu, Yang, Fang, Hong and Zhang2019a), increases smoking heaviness, and decreases the odds of quitting (Gage et al., Reference Gage, Bowden, Davey Smith and Munafo2018; Sanderson et al., Reference Sanderson, Davey Smith, Bowden and Munafò2019; Zeng et al., Reference Zeng, Ntalla, Kessler, Kastrati, Erdmann, Danesh and Schunkert2019; Zhou et al., Reference Zhou, Zhang, Liu, Yang, Fang, Hong and Zhang2019a). One study triangulated self-report measures with cotinine (a metabolite of nicotine) in blood samples and found weak evidence that higher educational attainment causes lower cotinine levels (Gage et al., Reference Gage, Bowden, Davey Smith and Munafo2018). There was considerable overlap among the data sets used (Table 2). Two studies based their education-to-smoking estimate on the same data sets, one testing whether smoking mediated the effects of education on coronary heart disease, and the other whether smoking mediated the effects of education on lung cancer (Tillmann et al., Reference Tillmann, Vaucher, Okbay, Pikhart, Peasey, Kubinova and Holmes2017; Zhou et al., Reference Zhou, Zhang, Liu, Yang, Fang, Hong and Zhang2019a). There was strong evidence that higher general cognitive ability decreases lifetime smoking (Adams, Reference Adams2019), but no clear evidence for effects on smoking initiation or cessation (Davies et al., Reference Davies, Hill, Anderson, Sanderson, Deary and Smith2019). Two multivariable MR studies found that causal effects of education on smoking were not mediated by cognitive ability (Davies et al., Reference Davies, Hill, Anderson, Sanderson, Deary and Smith2019; Sanderson et al., Reference Sanderson, Davey Smith, Bowden and Munafò2019).
Substantially fewer studies looked at causal effects ofsmoking on cognitive functioning. There was consistent evidence that smoking initiation and lifetime smoking decrease educational attainment (Gage et al., Reference Gage, Sallis, Lassi, Wootton, Mokrysz, Davey Smith and Munafò2020; Harrison et al., Reference Harrison, Davies, Dickson, Tyrrell, Green, Katikireddi and Howe2020b), and weaker evidence that they decrease cognitive ability (Gage et al., Reference Gage, Sallis, Lassi, Wootton, Mokrysz, Davey Smith and Munafò2020). Two other studies found no clear evidence for causal effects of smoking initiation on cognitive functioning (Adams, Reference Adams2019; North et al., Reference North, Palmer, Lewis, Cooper, Power, Pattie and Day2015), but note that the analysis by Gage et al. (Reference Gage, Sallis, Lassi, Wootton, Mokrysz, Davey Smith and Munafò2020) was superior (+ v. – +). There was also no clear evidence that smoking affects working memory, response inhibition, or emotion recognition, but these analyses were likely underpowered (Mahedy et al., Reference Mahedy, Wootton, Suddell, Caroline, Heron, Hickman and Munafò2021). A single analysis (rated –) reported that current smoking increases the odds of cognitive impairment (Fu, Faul, Jin, Ware, & Bakulski, Reference Fu, Faul, Jin, Ware and Bakulski2019). Two studies found weak evidence that smoking decreases the odds of Alzheimer's disease (Larsson et al., Reference Larsson, Traylor, Malik, Dichgans, Burgess and Markus2017; Østergaard et al., Reference Østergaard, Mukherjee, Sharp, Proitsi, Lotta, Day and Wareham2015), but a more recent analysis, rated as superior (+ v. – +), found no effects of smoking on Alzheimer's disease (Andrews et al., Reference Andrews, Fulton-Howard, O'Reilly, Marcora, Goate, Farrer and Wang2021). The seemingly protective effect of smoking is likely survival bias – smokers who do not die from smoking-related diseases are less prone to diseases making them less likely to develop Alzheimer's disease. Of note, smoking initiation is not an ideal measure – there is accumulating evidence that genetic variants associated with this phenotype are horizontally pleiotropic (Khouja, Wootton, Taylor, Smith, & Munafò, Reference Khouja, Wootton, Taylor, Smith and Munafò2020). A conclusion on the causal effects of smoking can more reliably be made by testing the effects of smoking heaviness.
Sleep problems
There was weak evidence that insomnia increases smoking heaviness and decreases cessation from two studies (Gibson, Munafò, Taylor, & Treur, Reference Gibson, Munafò, Taylor and Treur2019; Jansen et al., Reference Jansen, Watanabe, Stringer, Skene, Bryois, Hammerschlag and Posthuma2019), but not from a third (Lane et al., Reference Lane, Jones, Dashti, Wood, Aragam, van Hees and Saxena2019). In contrast, there was no clear evidence that sleep duration impacts smoking (Gibson et al., Reference Gibson, Munafò, Taylor and Treur2019). There was particularly strong evidence that smoking heaviness impacts chronotype, decreasing the odds of being a morning person (Gibson et al., Reference Gibson, Munafò, Taylor and Treur2019; Millard, Munafò, Tilling, Wootton, & Davey Smith, Reference Millard, Munafò, Tilling, Wootton and Davey Smith2019), but no clear evidence that smoking influences insomnia risk or sleep duration (Gibson et al., Reference Gibson, Munafò, Taylor and Treur2019; Jansen et al., Reference Jansen, Watanabe, Stringer, Skene, Bryois, Hammerschlag and Posthuma2019).
Internalizing/mood disorders
There was some evidence for causal, increasing effects of depression (Wootton et al., Reference Wootton, Richmond, Stuijfzand, Lawn, Sallis, Taylor and Munafò2019), feelings of loneliness (Wootton et al., Reference Wootton, Greenstone, Abdellaoui, Denys, Verweij, Munafò and Treur2020), and neuroticism (Sallis, Smith, & Munafo, Reference Sallis, Smith and Munafo2019) on smoking behavior. Adams (Reference Adams2019), using a larger data set for the outcome than Sallis et al. (Reference Sallis, Smith and Munafo2019), did not find clear evidence that neuroticism affects smoking. There was no clear evidence for causal effects of depression or bipolar disorder on smoking (Barkhuizen, Dudbridge, & Ronald, Reference Barkhuizen, Dudbridge and Ronald2020; Vermeulen et al., Reference Vermeulen, Wootton, Treur, Sallis, Jones, Zammit and Munafò2019). Most studies also tested effects in the other direction. Earlier studies showed no clear evidence for causal effects (Bjorngaard et al., Reference Bjorngaard, Gunnell, Elvestad, Smith, Skorpen, Krokan and Romundstad2013; Skov-Ettrup, Nordestgaard, Petersen, & Tolstrup, Reference Skov-Ettrup, Nordestgaard, Petersen and Tolstrup2017; Taylor et al., Reference Taylor, Fluharty, Bjørngaard, Gabrielsen, Skorpen, Marioni and Munafò2014a; Wium-Andersen, Orsted, & Nordestgaard, Reference Wium-Andersen, Orsted and Nordestgaard2015a), with one exception: a small study (n = 6294) of low-rated quality reporting that smoking decreased depression during pregnancy (Lewis et al., Reference Lewis, Araya, Smith, Freathy, Gunnell, Palmer and Munafo2011). More recent studies, employing much larger samples, found strong evidence for causal, increasing effects of smoking initiation and lifetime smoking on depression and bipolar disorder risk (Barkhuizen et al., Reference Barkhuizen, Dudbridge and Ronald2020; Vermeulen et al., Reference Vermeulen, Wootton, Treur, Sallis, Jones, Zammit and Munafò2019; Wootton et al., Reference Wootton, Richmond, Stuijfzand, Lawn, Sallis, Taylor and Munafò2019). There was weak evidence that smoking initiation increases feelings of loneliness (a phenotype closely related to depression) from one study (Wootton et al., Reference Wootton, Greenstone, Abdellaoui, Denys, Verweij, Munafò and Treur2020), but no such evidence from another (Harrison et al., Reference Harrison, Davies, Dickson, Tyrrell, Green, Katikireddi and Howe2020b). One study reported weak evidence that smoking initiation decreases neuroticism (Sallis et al., Reference Sallis, Smith and Munafo2019), whereas another, better-powered study found that lifetime smoking increases neuroticism (Adams, Reference Adams2019). Finally, there was no clear evidence that smoking causally impacts suicidal ideation (Harrison et al., Reference Harrison, Munafò, Davey Smith and Wootton2020a).
Externalizing disorders
There was strong evidence that ADHD liability increases smoking initiation, smoking heaviness and lifetime smoking, and decreases cessation (Fluharty, Sallis, & Munafò, Reference Fluharty, Sallis and Munafò2018; Leppert et al., Reference Leppert, Riglin, Dardani, Thapar, Staley, Tilling and Stergiakouli2019; Sallis et al., Reference Sallis, Smith and Munafo2019; Treur et al., Reference Treur, Demontis, Sallis, Richardson, Wiers, Borglum and Munafo2019). There was no clear evidence that aggression causally affects smoking, but this analysis was likely underpowered (Fluharty et al., Reference Fluharty, Sallis and Munafò2018). One study also tested reverse effects, reporting weak evidence that smoking initiation increases ADHD risk, but with important cautionary notes about the pleiotropic nature of the initiation measure (Treur et al., Reference Treur, Demontis, Sallis, Richardson, Wiers, Borglum and Munafo2019).
Psychotic disorders
Multiple studies reported evidence (ranging from weak to strong) that smoking causally increases schizophrenia risk (Barkhuizen et al., Reference Barkhuizen, Dudbridge and Ronald2020; Byrne et al., Reference Byrne, Ferreira, Xue, Lindström, Jiang, Yang and Chenevix-Trench2019; Gage et al., Reference Gage, Jones, Taylor, Burgess, Zammit and Munafò2017b; Wium-Andersen et al., Reference Wium-Andersen, Orsted and Nordestgaard2015a, ; Wootton et al., Reference Wootton, Richmond, Stuijfzand, Lawn, Sallis, Taylor and Munafò2019). In the other direction there was no clear evidence for causal effects of liability to schizophrenia on smoking from one study (Gage et al., Reference Gage, Jones, Burgess, Bowden, Davey Smith, Zammit and Munafò2017a, Reference Gage, Jones, Taylor, Burgess, Zammit and Munafò2017b), and some evidence for such effects from two recent, better-powered studies with largely overlapping samples (Barkhuizen et al., Reference Barkhuizen, Dudbridge and Ronald2020; Wootton et al., Reference Wootton, Richmond, Stuijfzand, Lawn, Sallis, Taylor and Munafò2019).
Alcohol use
Cognitive traits
There was strong evidence that higher educational attainment increases alcohol use frequency (Davies et al., Reference Davies, Dickson, Davey Smith, Windmeijer and van den Berg2018a; Davies et al., Reference Davies, Hill, Anderson, Sanderson, Deary and Smith2019; Rosoff et al., Reference Rosoff, Clarke, Adams, McIntosh, Davey Smith, Jung and Lohoff2019; Zhou et al., Reference Zhou, Zhang, Liu, Yang, Fang, Hong and Zhang2019a, Reference Zhou, Sun, Li, Ma, Heianza and Qi2019b) and wine intake (Rosoff et al., Reference Rosoff, Clarke, Adams, McIntosh, Davey Smith, Jung and Lohoff2019; Zhou et al., Reference Zhou, Zhang, Liu, Yang, Fang, Hong and Zhang2019a, Reference Zhou, Sun, Li, Ma, Heianza and Qi2019b), whereas it decreases beer/cider intake (Zhou et al., Reference Zhou, Zhang, Liu, Yang, Fang, Hong and Zhang2019a, Reference Zhou, Sun, Li, Ma, Heianza and Qi2019b), and the risk of binge-drinking and alcohol use disorder (Rosoff et al., Reference Rosoff, Clarke, Adams, McIntosh, Davey Smith, Jung and Lohoff2019; Zhou et al., Reference Zhou, Sealock, Sanchez-Roige, Clarke, Levey, Cheng and Gelernter2020). Ding et al. (Reference Ding, Barban and Mills2019) did not find clear evidence for causality from education to alcohol use, but this analysis was likely underpowered. There was also evidence that general cognitive ability increases alcohol use frequency (Davies et al., Reference Davies, Hill, Anderson, Sanderson, Deary and Smith2019) and decreases the risk of alcohol use disorder (Zhou et al., Reference Zhou, Sealock, Sanchez-Roige, Clarke, Levey, Cheng and Gelernter2020). In the other direction, Rosoff et al. (Reference Rosoff, Clarke, Adams, McIntosh, Davey Smith, Jung and Lohoff2019) found weak evidence that higher alcohol use decreases educational attainment, whereas another study did not (Harrison et al., Reference Harrison, Davies, Dickson, Tyrrell, Green, Katikireddi and Howe2020b). A third, high-quality rated study found that liability to alcohol use disorder negatively impacts educational attainment (Zhou et al., Reference Zhou, Sealock, Sanchez-Roige, Clarke, Levey, Cheng and Gelernter2020). Note that GWAS of current alcohol use have largely been performed in adults, reflecting alcohol use after maximum educational attainment occurred for most. Although the genetic instrument may also reflect alcohol use at younger ages, this needs to be taken into account. There was no clear evidence that drinking more alcohol impacts cognition, but this was based on (very) small, low-quality rated studies (Almeida, Hankey, Yeap, Golledge, & Flicker, Reference Almeida, Hankey, Yeap, Golledge and Flicker2014a, Reference Almeida, Hankey, Yeap, Golledge and Flicker2014b; Au Yeung et al., Reference Au Yeung, Jiang, Cheng, Liu, Zhang, Lam and Schooling2012; Kumari et al., Reference Kumari, Holmes, Dale, Hubacek, Palmer, Pikhart and Bobak2014; Mahedy et al., Reference Mahedy, Suddell, Skirrow, Fernandes, Field, Heron and Munafò2020; Ritchie et al., Reference Ritchie, Bates, Corley, McNeill, Davies, Liewald and Deary2014). There were contradicting findings for Alzheimer's disease, with one study finding no causal effects of alcohol (Larsson et al., Reference Larsson, Traylor, Malik, Dichgans, Burgess and Markus2017) and another finding that while a higher number of drinks caused an earlier onset of Alzheimer's disease, alcohol use disorder caused a later onset (Andrews, Goate, & Anstey, Reference Andrews, Goate and Anstey2020). The latter likely reflects survival bias.
Sleep problems
There was some evidence that drinking more alcohol per week increases sleep duration, but this was based on only one, low-quality rated study (Nishiyama et al., Reference Nishiyama, Nakatochi, Goto, Iwasaki, Hachiya, Sutoh and Suzuki2019).
Internalizing/mood disorders
A recent, particularly large study reported strong evidence that major depressive disorder (MDD) liability increases alcohol use disorder risk (Polimanti et al., Reference Polimanti, Peterson, Ong, MacGregor, Edwards, Clarke and Derks2019). Similarly, there was evidence that worrying and neuroticism increase alcohol use disorder risk (Zhou et al., Reference Zhou, Sealock, Sanchez-Roige, Clarke, Levey, Cheng and Gelernter2020). There was no clear evidence that feelings of loneliness affect alcohol use (disorder) (Wootton et al., Reference Wootton, Greenstone, Abdellaoui, Denys, Verweij, Munafò and Treur2020). In the other direction, there was no clear evidence that alcohol use (disorder) causally impacts internalizing symptoms (Almeida et al., Reference Almeida, Hankey, Yeap, Golledge and Flicker2014a, Reference Almeida, Hankey, Yeap, Golledge and Flicker2014b; Chao, Li, & McGue, Reference Chao, Li and McGue2017; Lim et al., Reference Lim, Rijsdijk, Hagenaars, Socrates, Choi, Coleman and Pingault2020; Polimanti et al., Reference Polimanti, Peterson, Ong, MacGregor, Edwards, Clarke and Derks2019; Wium-Andersen, Orsted, Tolstrup, & Nordestgaard, Reference Wium-Andersen, Orsted, Tolstrup and Nordestgaard2015b; Wootton et al., Reference Wootton, Greenstone, Abdellaoui, Denys, Verweij, Munafò and Treur2020; Zhou et al., Reference Zhou, Sealock, Sanchez-Roige, Clarke, Levey, Cheng and Gelernter2020).
Externalizing disorders
There was weak evidence that ADHD liability increases alcohol use disorder risk (Treur et al., Reference Treur, Demontis, Sallis, Richardson, Wiers, Borglum and Munafo2019). In the other direction, there was some evidence that higher alcohol use frequency increases aggression and attention problems from one, small (n = 1608) low-rated analysis (Chao et al., Reference Chao, Li and McGue2017), and no evidence for causal effects on antisocial behavior from another very small (n = 180) low-rated analysis (Irons, McGue, Iacono, & Oetting, Reference Irons, McGue, Iacono and Oetting2007).
Psychotic disorders
There was no clear evidence for causal effects, in either direction, between alcohol use disorder and schizophrenia risk (Zhou et al., Reference Zhou, Sealock, Sanchez-Roige, Clarke, Levey, Cheng and Gelernter2020).
Cannabis use
Cognitive traits
There was no evidence for causal effects from cannabis initiation to cognitive functioning (Mahedy et al., Reference Mahedy, Wootton, Suddell, Caroline, Heron, Hickman and Munafò2021).
Internalizing disorders
There was neither clear evidence for causal effects in either direction between cannabis initiation and MDD (Hodgson et al., Reference Hodgson, Coleman, Hagenaars, Purves, Glanville, Choi and Lewis2020), nor was there evidence for causal effects from cannabis initiation to self-harm (Lim et al., Reference Lim, Rijsdijk, Hagenaars, Socrates, Choi, Coleman and Pingault2020).
Externalizing disorders
There was evidence that ADHD liability increases cannabis initiation without clear evidence for the reverse (Soler Artigas et al., Reference Soler Artigas, Sánchez-Mora, Rovira, Richarte, Garcia-Martínez, Pagerols and Ribasés2019; Treur et al., Reference Treur, Demontis, Sallis, Richardson, Wiers, Borglum and Munafo2019).
Psychotic disorders
Out of eight studies that included cannabis, three looked at schizophrenia. One tested causality from cannabis initiation to schizophrenia risk only, finding evidence for an increasing effect (Vaucher et al., Reference Vaucher, Keating, Lasserre, Gan, Lyall, Ward and Holmes2018). Two other studies tested causal effects in both directions and found weak evidence that cannabis initiation increases schizophrenia risk and strong evidence that schizophrenia liability increases the odds of cannabis initiation (Gage et al., Reference Gage, Jones, Burgess, Bowden, Davey Smith, Zammit and Munafò2017a, Reference Gage, Jones, Taylor, Burgess, Zammit and Munafò2017b; Pasman et al., Reference Pasman, Verweij, Gerring, Stringer, Sanchez-Roige, Treur and Vink2018).
Caffeine consumption
Cognitive traits
There was weak evidence that higher coffee consumption increases Alzheimer's risk from one study (Larsson et al., Reference Larsson, Traylor, Malik, Dichgans, Burgess and Markus2017), but no clear evidence from another (Kwok, Leung, & Schooling, Reference Kwok, Leung and Schooling2016). There was also no clear evidence for causal effects of coffee on general cognitive functioning (Zhou et al., Reference Zhou, Taylor, Karhunen, Zhan, Rovio, Lahti and Hypponen2018).
Sleep problems
There was weak evidence that higher plasma caffeine levels decrease the odds of being a morning person, but no clear evidence for causal effects between self-reported caffeine consumption and sleep duration, insomnia, or chronotype (Treur et al., Reference Treur, Gibson, Taylor, Rogers, Munafo and Munafò2018).
Internalizing disorders
There was no clear evidence for causal effects between caffeine consumption and ADHD, in either direction (Treur et al., Reference Treur, Demontis, Sallis, Richardson, Wiers, Borglum and Munafo2019),
Externalizing disorders
There was no evidence for causal effects of caffeine consumption on depression (Kwok et al., Reference Kwok, Leung and Schooling2016).
Discussion
We conducted the first systematic review of MR studies investigating causal relationships between mental health and substance use. From a total of 63 studies, we can draw important conclusions regarding if and how mental health and substance use are causally related.
Smoking was the most investigated, resulting in particularly strong evidence that higher educational attainment causally decreases smoking (lower risk of initiating, smoking fewer cigarettes, and more likely to quit). Although smoking prevalence has rapidly decreased in the past two decades, this decline has been most prominent among those with high educational attainment, leading to an increasing (health) gap (Agaku, Odani, Okuyemi, & Armour, Reference Agaku, Odani, Okuyemi and Armour2020). The causal role of education we report is important for policy-makers going forward. Interestingly, causal effects from education are neither mediated by cognitive ability (Sanderson et al., Reference Sanderson, Davey Smith, Bowden and Munafò2019) nor were there clear evidence that cognitive ability by itself affects smoking (Adams, Reference Adams2019; Davies et al., Reference Davies, Hill, Anderson, Sanderson, Deary and Smith2019). The studies included in this review cannot determine exactly why educational attainment affects smoking. Smoking initiation usually occurs during adolescence, at which time the home environment and peer influences are important. Adolescents in lower educational groups tend to experience lower levels of parental involvement, parental monitoring, and self-perceived social competence, factors associated with a higher odds of initiating smoking (Mahabee-Gittens, Xiao, Gordon, & Khoury, Reference Mahabee-Gittens, Xiao, Gordon and Khoury2013; Simons-Morton, Reference Simons-Morton2002). As for smoking heaviness and difficulty quitting, causal mechanisms may involve job opportunities that depend on educational attainment. A lower education often leads to jobs characterized by low skill discretion, high psychological demands and high physical exertion, potentially leading to stress and smoking to cope (Dobson, Gilbert-Ouimet, Mustard, & Smith, Reference Dobson, Gilbert-Ouimet, Mustard and Smith2018a).
Another striking pattern was that of bi-directional, increasing effects between smoking and mental disorders. There was more robust evidence that smoking causally increases the odds of mental disorders than vice versa – most notably for depression, bipolar disorder, and schizophrenia. This concurs with accumulating evidence from longitudinal cohort studies (Taylor et al., Reference Taylor, McNeill, Girling, Farley, Lindson-Hawley and Aveyard2014b) and animal research (Jobson et al., Reference Jobson, Renard, Szkudlarek, Rosen, Pereira, Wright and Laviolette2019) indicating neuropsychiatric effects of smoking. A causal mechanism may be that nicotine binds to nicotinic acetylcholine receptors in the brain, given that these are involved in regulating central nervous system pathways relevant to mental disorders (Berk et al., Reference Berk, Kapczinski, Andreazza, Dean, Giorlando, Maes and Malhi2011). There is some evidence that repeated nicotine exposure can lead to desensitization of these receptors (Mineur & Picciotto, Reference Mineur and Picciotto2009). Inflammation and oxidative stress induced by toxic compounds from inhaled cigarette smoke is another potential mechanism (Berk et al., Reference Berk, Kapczinski, Andreazza, Dean, Giorlando, Maes and Malhi2011). Our conclusion that smoking is detrimental to the brain warrants increased efforts to prevent (heavy) substance use. For individuals with a mental disorder, it implies that smoking cessation may be beneficial to alleviate symptoms. This is an important message given that smokers in this population are not always encouraged to quit (Taylor et al., Reference Taylor, Baker, Fox, Kessler, Aveyard and Munafò2020a). Although not an easy task, it should be communicated to health professionals that there are effective ways to help smokers with a co-morbid mental disorder quit.
A higher education increased alcohol use frequency but decreased the risk of problematic use. Those with higher education tend to drink alcohol more often but spread across multiple drinking occasions, and without developing a dependency. Those with lower education, on the other hand, are at increased odds of developing a problematic relationship with alcohol. This pattern of opposite effects was recently also highlighted in a study that computed genetic correlations and reported high alcohol use frequency to be genetically correlated with higher socio-economic status and lower risk of psychiatric disorders, whereas high alcohol consumption quantity was genetically correlated with lower socio-economic status and higher psychiatric disorder risk (Marees et al., Reference Marees, Smit, Ong, MacGregor, An, Denys and van den Brink2019). Similar to smoking, it could be that excessive alcohol use is a way to cope with job stress (Dobson, Ibrahim, Gilbert-Ouimet, Mustard, & Smith, Reference Dobson, Ibrahim, Gilbert-Ouimet, Mustard and Smith2018b). There was also consistent evidence that mental disorders increase (problematic) alcohol use, without strong effects in the other direction. The latter implies that observational findings indicating that alcohol use increases mental disorders were due to confounding and/or reverse causality. Indeed, associations between heavy drinking and subsequent increases in depressive symptoms disappeared after adjustment for confounders (Li et al., Reference Li, Wang, Li, Shen, Li, Zhang and Peng2020). It should be noted that this is in contrast to clinical observations where in the short-term, treating alcohol use disorder makes pre-existing depression symptoms disappear (Charlet & Heinz, Reference Charlet and Heinz2017). This discrepancy may be because MR assesses ‘lifetime’ (longer-term) effects of alcohol on mental health (Labrecque & Swanson, Reference Labrecque and Swanson2019), and the fact that only a small proportion of those with an alcohol use disorder will receive treatment [<9% (Mark, Kassed, Vandivort-Warren, Levit, & Kranzler, Reference Mark, Kassed, Vandivort-Warren, Levit and Kranzler2009)]. In sum, the current MR literature suggests that co-morbidity between poor mental health and alcohol use is primarily the result of alcohol being used as a type of ‘self-medication.’
There was stronger evidence that liability to schizophrenia increases the odds to initiate cannabis, than that cannabis initiation increases schizophrenia risk, as also indicated recently by others (Gillespie & Kendler, Reference Gillespie and Kendler2021). However, these results should be regarded as tentative, given that the genetic instrument for schizophrenia was more powerful than that for cannabis use, and more insightful analyses, with measures of cannabis use frequency, have not yet been performed. This is an important direction for future MR studies, now that such large-scale cannabis studies are becoming available (Hines, Treur, Jones, Sallis, & Munafò, Reference Hines, Treur, Jones, Sallis and Munafò2020).
For caffeine, the predominantly studied relationships were with cognitive functioning and sleep. Overall, there was no clear evidence that a high average intake of caffeine (negatively or positively) affects cognitive measures or sleep. This is consistent with recent evidence that average (high) caffeine intake does not necessarily result in changes in alertness or sleep patterns, due to the fact that adaptation occurs after repeated intake (Weibel et al., Reference Weibel, Lin, Landolt, Garbazza, Kolodyazhniy, Kistler and Reichert2020).
Limitations
Although our scoring system was carefully designed [using the collective experience of the authors and the tentative, developing STROBE-MR ("Strengthening the Reporting of Observational Studies in Epidemiology using Mendelian Randomization") guidelines (Davey Smith et al., Reference Davey Smith, Davies, Dimou, Egger, Gallo, Golub and Yarmolinsky2019)] it should be noted that it was not previously validated. As for the included MR studies, while the more recent were sufficiently powered and some included thorough sensitivity methods and triangulation, many earlier studies were low-quality. In the coming years, it will be important to extend and strengthen the current evidence through MR studies that combine better-powered data sets, preferably with more fine-grained phenotypes and extensive sensitivity methods. An important focal point for smoking and cannabis use as exposure variables is to not only investigate initiation, but also the heaviness of use. There is ample evidence that measurements of initiation can introduce bias due to horizontal pleiotropy and reverse causality (Khouja et al., Reference Khouja, Wootton, Taylor, Smith and Munafò2020; Li et al., Reference Li, Wang, Li, Shen, Li, Zhang and Peng2020; Treur et al., Reference Treur, Demontis, Sallis, Richardson, Wiers, Borglum and Munafo2019; Yuan, Yao, & Larsson, Reference Yuan, Yao and Larsson2020b). Another important addition to future work is multivariable MR, which allows the inclusion of multiple exposures to further decrease the risk of horizontal pleiotropy and provide more extensive testing of causal mechanisms. In addition, triangulating with high-quality observational analyses, or as was done by Davies et al. (Reference Davies, Dickson, Davey Smith, Windmeijer and van den Berg2018a) with results from policy reform, would be ideal. There are three important sources of potential bias that are not (sufficiently) accounted for in current MR studies; genetic nurturing (genetic variants that are not transmitted from parents to offspring still affecting offspring phenotype), assortative mating (spouses genetically resembling each other more than by chance because they selected each other based on a genetically influenced trait), and geographic genetic clustering (Brumpton et al., Reference Brumpton, Sanderson, Hartwig, Harrison, Vie, Cho and Bielak2020). These phenomena may re-introduce bias from potential confounders, shifting the MR estimate towards the observational association. This can be prevented by performing MR with genetic estimates from within-family GWAS, as these will be corrected for all factors shared within families. Finding large enough family samples will be an important challenge in coming years [the first of such efforts recently became available (Howe et al., Reference Howe, Nivard, Morris, Hansen, Rasheed, Cho and Davies2021). Finally, it is important to acknowledge that almost all MR studies were based on cohorts including participants of European descent. Because of the lack of diversity in the field of genetic research, genetic instruments needed to perform MR for other ethnic groups are rarely available. Increasing diversity in genetic research will be pivotal if we want to reach a comprehensive understanding of the genetic etiology of mental health and substance use, as well as the causal nature of their relationship (Abdellaoui & Verweij, Reference Abdellaoui and Verweij2021).
Conclusion
In this systematic review of MR studies, we found strong evidence that higher educational attainment decreases smoking and that there is a bi-directional, increasing relationship between smoking and (symptoms of) mental disorders (depression, bipolar disorder, and schizophrenia). Another robust finding was that higher educational attainment increases alcohol use frequency, whereas it decreases the risk of binge-drinking and alcohol use problems, and that (symptoms of) mental disorders causally lead to more alcohol drinking without evidence for the reverse. Future work should attempt to tackle important limitations that were highlighted in this review. An approach that is particularly noteworthy, and should be used more routinely, is multivariable MR. The etiology of mental health traits is complex and we have only a limited understanding of the biological pathways from SNP to phenotype. It is, therefore, important to test whether key variables act as confounders (inducing a false-positive causal finding) or mediate the causal relationship (i.e. are part of the causal chain from exposure to the outcome). This is especially relevant for MR studies investigating educational attainment as an exposure (McMartin & Conley, Reference McMartin and Conley2020). Multivariable MR allows the modeling of complex networks of genetic effects linking different mental health traits. Finally, triangulation of MR results with other research methods is crucial. This includes comparison to other genetically informative methods such as twin studies, latent causal variable analysis (O'Connor & Price, Reference O'Connor and Price2018), or genomic structural equation modeling (Grotzinger et al., Reference Grotzinger, Rhemtulla, de Vlaming, Ritchie, Mallard, Hill and Tucker-Drob2019), carefully conducted longitudinal analyses of cohort data, and/or instrumental variable methods that use environmental factors (e.g. policy changes) instead of genes as an instrument.
Taken together, the current body of MR studies is a valuable addition to the literature on mental health and substance use. It has provided more robust evidence that substance use (most notably smoking) can cause mental health problems, thereby (further) strengthening the incentive to decrease substance use, particularly among populations with poor mental health.
Supplementary material
The supplementary material for this article can be found at https://doi.org/10.1017/S003329172100180X.
Acknowledgements
MRM is a member of the MRC Integrative Epidemiology Unit at the University of Bristol (MC_UU_00011/7), and the National Institute for Health Research (NIHR) Biomedical Research Centre at the University Hospitals Bristol National Health Service (NHS) Foundation Trust, and the University of Bristol.
Financial support
JLT was supported by a Veni grant from the Netherlands Organization for Scientific Research (NWO; grant number 016.Veni.195.016). KJHV and JLT were supported by the Foundation Volksbond Rotterdam.
Conflict of interest
None of the authors have any conflicts of interest to declare, financial or otherwise.