Long-term conditions are highly prevalent in the general population affecting approximately 20% of people Reference Weich, Bebbington, Rai, Stranges, McBride and Spiers1 above the age of 16 and 58% of people over the age of 60. 2 Among the more prevalent long-term conditions in the general population are hypertension, chronic pain, gastrointestinal disorders, asthma, arthritis, diabetes, heart disease and chronic obstructive pulmonary disease (COPD). Reference Weich, Bebbington, Rai, Stranges, McBride and Spiers1,Reference Barnett, Mercer, Norbury, Watt, Wyke and Guthrie3 Multimorbidity is common Reference Barnett, Mercer, Norbury, Watt, Wyke and Guthrie3 and these long-term conditions carry a huge financial cost to health services, accounting for approximately 70% of total health and social care expenditure in public healthcare systems like the UK National Health Service. 2 This highlights the need to treat both long-term conditions and mental health problems concurrently. Although access to psychological care has been recommended as part of integrated care for people with long-term conditions, 4 it is possible that the effect of psychological interventions for mental health problems may be attenuated by long-term conditions. For example, Dickens and collaborators Reference Dickens, Cherrington, Adeyemi, Roughley, Bower and Garrett5 reviewed the efficacy of psychological interventions for depression in patients with coronary heart disease and concluded that clinical trials show mixed evidence with small effect sizes favouring cognitive–behavioural therapy (CBT) as the treatment of choice for this population. Similar conclusions were reached in a systematic review of psychological interventions for persistent pain, which indicates modest effects for CBT in depression and anxiety symptom reductions. Reference Williams, Eccleston and Morley6 Clinical trials reviewed in these meta-analyses often include participants with highly disabling long-term conditions treated in specialist services or hospital settings, and therefore it is unclear whether these findings are reflective of outcomes from routinely delivered therapy in primary care settings, where the majority of patients' long-term conditions are treated. The objective of this study was to investigate the clinical effectiveness of primary care psychological interventions for depression and anxiety in a large naturalistic sample, comparing outcomes between patients with and without long-term conditions.
Method
Setting and interventions
Retrospective clinical case records for a cohort of patients receiving psychological therapy were analysed. These data were gathered as part of routine clinical care at a psychological therapy service in the north of England that was linked to the national Improving Access to Psychological Therapies (IAPT) programme. Reference Clark, Layard, Smithies, Richards, Suckling and Wright7 The study was conducted as a service evaluation using fully de-identified data, and therefore did not require ethical approval. Patients provided informed consent for their anonymous data to be used for audit, evaluation and research purposes. Patients accessing the service presented with depression- and anxiety-related problems and received evidence-based interventions organised in a stepped-care model. 8 The majority accessed brief (less than eight sessions), low-intensity guided self-help interventions based on principles of CBT. Those with enduring symptoms after guided self-help and those with more severe conditions had access to high-intensity (up to 20 sessions) therapies including CBT, interpersonal psychotherapy, counselling for depression and eye movement desensitisation and reprocessing (EMDR) for post-traumatic stress.
Measures and data sources
Consistent with the national IAPT programme, patients in the sample were asked to self-complete three standard outcome measures to monitor progress on a weekly basis. The Patient Health Questionnaire (PHQ-9) is a nine-item measure of major depression symptoms. Reference Kroenke, Spitzer and Williams9 Each item is rated on four ordinal response options (0, not at all; 3, nearly every day), resulting in a depression severity score between 0 and 27. A cut-off ⩾10 is used to detect clinically significant depression symptoms. The Generalised Anxiety Disorder scale (GAD-7) is a seven-item measure of anxiety symptoms; it is also rated using four ordinal response options (0, not at all; 3, nearly every day), resulting in an anxiety severity score between 0 and 21. Reference Kroenke, Spitzer, Williams, Monahan and Löwe10 A cut-off score ⩾8 is recommended to identify the likely presence of a diagnosable anxiety disorder. The Work and Social Adjustment Scale (WSAS) is a measure of functioning across five domains: work, home management, social leisure activities, private leisure activities, and family and relationships. Reference Mundt, Marks, Shear and Greist11 Each item is rated between 0 (no impairment) and 8 (very severe impairment), with a total severity score between 0 and 40.
De-identified clinical assessment records were also collected for participants, including demographic (age, gender, ethnicity, employment, socioeconomic deprivation) and clinical information (primary diagnosis, baseline severity, use of psychotropic medication, number of treatment contacts, number of referrals into the service for each patient over a 5-year period, pre- and post-treatment outcome measures described above). Self-reported long-term conditions were gathered using a standardised checklist of chronic illnesses at the time of initial assessment. 12 This checklist prompts clinicians to gather information about 15 specific conditions including severe (psychotic) mental health problems and an option to note ‘other’ unspecified conditions. Socioeconomic deprivation was assessed by matching participants' home postcodes to the English Index of Multiple Deprivation (IMD) 13 and categorising participants into quintile levels of deprivation (categorical variable named IMD).
Sample characteristics
A total of 32 734 case records were gathered for patients who accessed psychological treatment (at least one session) over a period of 5 years between 2010 and 2015. Of these, 2676 (8.2%) were excluded because they had no recorded information about long-term conditions, and a further 1560 (4.8%) were excluded because no baseline and end scores were available for at least one of the outcome measures (PHQ-9 or GAD-7). This resulted in a sample of 28 498 case records that were available for analysis. The mean age in the sample was 38.27 (s.d. = 13.94, range 16–92); 64.6% were female; 85.5% were of a White British background; and 36.6% were unemployed. Primary presenting problems recorded in clinical assessments are presented in Table 1; the most common were recurrent depression (38.5%), mixed anxiety and depression (26.0%) and generalised anxiety disorder (12.1%). Self-reported long-term conditions are listed in order of prevalence in Table 2. Overall, 23.2% of patients reported having at least one long-term condition; the most common were asthma (6.8%), musculoskeletal problems (chronic pain, 1.8%) and hypertension (1.7%). Approximately 68.0% of patients in this sample only received low-intensity guided self-help interventions, and 32.0% accessed high-intensity therapies.
Table 1 Primary presenting problems recorded in clinical assessments (n = 28 498)

Description | n | % |
---|---|---|
Recorded presenting problem (n = 22 609) | ||
Recurrent depression | 8698 | 38.5 |
Mixed anxiety and depression | 5887 | 26.0 |
Generalised anxiety disorder | 2725 | 12.1 |
Depressive episode | 1160 | 5.1 |
Panic disorder | 970 | 4.3 |
Social phobia | 540 | 2.4 |
Obsessive–compulsive disorder | 643 | 2.8 |
Post-traumatic stress disorder | 565 | 2.5 |
Specific phobia | 290 | 1.3 |
Bereavement | 201 | 0.9 |
Eating disorder | 177 | 0.8 |
Agoraphobia | 163 | 0.7 |
Somatoform disorder | 149 | 0.7 |
Alcohol related mental or behavioural disorder | 40 | 0.2 |
Bipolar affective disorder | 22 | 0.1 |
Does not meet diagnostic criteria for a common mental disorder |
379 | 1.7 |
Presenting problem not specified in records | 5889 | 20.7 |
Table 2 Self-reported long-term medical conditions (n = 28 498)
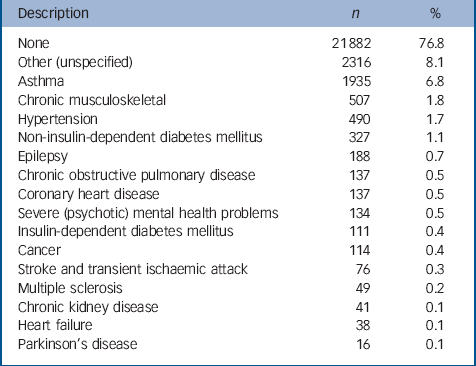
Description | n | % |
---|---|---|
None | 21 882 | 76.8 |
Other (unspecified) | 2316 | 8.1 |
Asthma | 1935 | 6.8 |
Chronic musculoskeletal | 507 | 1.8 |
Hypertension | 490 | 1.7 |
Non-insulin-dependent diabetes mellitus | 327 | 1.1 |
Epilepsy | 188 | 0.7 |
Chronic obstructive pulmonary disease | 137 | 0.5 |
Coronary heart disease | 137 | 0.5 |
Severe (psychotic) mental health problems | 134 | 0.5 |
Insulin-dependent diabetes mellitus | 111 | 0.4 |
Cancer | 114 | 0.4 |
Stroke and transient ischaemic attack | 76 | 0.3 |
Multiple sclerosis | 49 | 0.2 |
Chronic kidney disease | 41 | 0.1 |
Heart failure | 38 | 0.1 |
Parkinson's disease | 16 | 0.1 |
Statistical analysis
The goals of the analysis were to predict depression (PHQ-9) and anxiety (GAD-7) symptom severity at the end of treatment, controlling for the demographic and clinical characteristics described above, and to compare these outcomes between individuals with and without long-term conditions. Given the typically high correlations between the PHQ-9 and GAD-7 scales, Reference Böhnke, Lutz and Delgadillo14 we applied a seemingly unrelated regression (SUR) model. Reference Zellner15 SUR models estimate several equations simultaneously when the error terms of these equations are potentially correlated, which is a likely scenario for the prediction equations from PHQ-9 and GAD-7 scores. These models have been applied previously to model correlated dimensions of patient-reported outcomes and have been shown to increase the efficiency of estimates in such situations. Reference Keshavarzi, Ayatollahi, Zare and Farkhondeh16 As a sensitivity analysis, we repeated the SUR analysis using a dichotomous variable denoting the presence or absence of a long-term condition, instead of entering dummy variables for the different long-term condition categories. In order to compare outcomes between patients with and without long-term conditions, we estimated effect sizes (Cohen's d) based on comparing post-treatment outcomes between specific long-term condition groups v. the ‘no long-term conditions’ category and the root mean square error (RMSE) of the respective part (i.e. PHQ-9 or GAD-7) of the SUR model.
As a sensitivity analysis, we assessed differential item functioning (DIF) to verify whether responses to the PHQ-9 and GAD-7 measures are comparable across long-term condition groups, or whether differences in outcome scores could be biased in favour, or against, certain long-term conditions. Specifically, we aimed to assess whether responses to individual PHQ-9 or GAD-7 items corresponded to the same psychopathology severity levels across long-term condition groups. To achieve this, we applied logistic ordinal regressions standardising on latent variable estimates of depression and anxiety using a Generalised Partial Credit Model (uniform and non-uniform DIF), as described by Crane et al. Reference Crane, Gibbons, Ocepek-Welikson, Cook, Cella and Narasimhalu17 We evaluated differences in Pseudo-R 2 values between regression models to assess whether the long-term condition categories explained a relevant amount of variance in final PHQ-9 and GAD-7 scores.
Considering the stepped-care context in which psychological interventions were provided to this sample, we carried out secondary analyses to investigate whether patients with long-term conditions may differ in their probability of receiving and responding to high-intensity therapy, which would be plausible given their general higher level of distress and functional impairment. To test this hypothesis, we used the same predictors described above (in the SUR model) in a logistic regression model aiming to predict the likelihood of concluding a treatment episode at the higher step of care (high-intensity therapy v. low-intensity care as a reference category). In this analysis n = 29 (0.1% of total) case records were excluded because of inputting errors in the clinical database, which did not enable us to identify the assignment of individuals to low- or high-intensity steps of care. Furthermore, we repeated the SUR analysis separately analysing individuals that finished their treatment episode after accessing low- (n = 18 902) or high- (n = 8884) intensity interventions.
Since the amount of missing data was only minimal (n miss = 683, 2.4% of available cases), no additional imputation analyses were undertaken. As expected, most missing data points were for the outcome measures (PHQ-9 and GAD-7) and 390 individuals did not have valid postcodes documented in clinical records, so IMD could not be derived. Furthermore, IMD data could not be imputed, since it is a geographical rather than an individual characteristic.
Results
SUR equations modelling
As expected, the equations for PHQ-9 and GAD-7 outcomes were highly correlated. The correlation between the error terms of the two equations was large (r = 0.82) and statistically significant (Breusch–Pagan test, χ2 = 18 704.17, P<0.001). The SUR model explained a moderate amount of variance in post-treatment outcomes (PHQ-9 34%; GAD-7 29%). Table 3 presents the SUR model results in the full sample, which are interpreted below. In what follows, we refer to combined depression (PHQ-9) and anxiety (GAD-7) symptoms at the time of the last attended treatment session as ‘post-treatment distress levels’.
Table 3 Estimated coefficients for the seemingly unrelated regression (SUR) model jointly predicting post-treatment depression (PHQ-9) and anxiety (GAD-7) severity

B (s.e.) | ||
---|---|---|
Variable | PHQ-9 | GAD-7 |
PHQ-9 (baseline severity) | 0.31*** (0.00) | – |
GAD-7 (baseline severity) | – | 0.33*** (0.00) |
WSAS (baseline severity) | 0.17*** (0.00) | 0.13*** (0.00) |
Age (years) | −0.01** (0.00) | −0.01*** (0.00) |
Female | −0.07 (0.07) | 0.02 (0.07) |
Unemployed | 0.68*** (0.07) | 0.54*** (0.07) |
Ethnicity (reference category White British) |
||
Dual heritage | 0.49* (0.23) | 0.56** (0.20) |
South Asian | 1.29*** (0.18) | 1.13*** (0.16) |
Black British, African or Caribbean | −0.37 (0.25) | −0.38 (0.22) |
Other | 0.53*** (0.15) | 0.45*** (0.13) |
IMD (reference category 1st IMD quintile) |
||
2nd quintile | −0.77*** (0.11) | −0.63*** (0.10) |
3rd quintile | −1.11*** (0.11) | −0.85*** (0.09) |
4th quintile | −1.36*** (0.11) | −1.03*** (0.10) |
5th quintile | −1.75*** (0.12) | −1.33*** (0.10) |
Medication | −0.30 (0.18) | −0.20 (0.16) |
Number of referrals into service | 0.59*** (0.03) | 0.55*** (0.03) |
Treatment contacts attended | −0.25*** (0.01) | −0.22*** (0.01) |
Mental health (reference category depressive episode) |
||
Recurrent depression | 0.36 (0.19) | 0.29 (0.17) |
Mixed anxiety and depression | 0.16 (0.10) | 0.22* (0.09) |
Generalised anxiety disorder | −0.56*** (0.14) | −0.14 (0.12) |
Social phobia | −0.30 (0.27) | −0.24 (0.24) |
Panic disorder | −0.43* (0.20) | −0.12 (0.18) |
Agoraphobia | −0.09 (0.46) | 0.15 (0.41) |
Specific phobia | −0.23 (0.36) | 0.10 (0.31) |
OCD | −0.25 (0.25) | 0.69** (0.22) |
PTSD | 0.55* (0.26) | 1.01*** (0.23) |
Bereavement | 0.76 (0.42) | 0.69 (0.38) |
Eating disorder | 1.64*** (0.45) | 0.71 (0.40) |
Somatoform disorder | −1.18* (0.49) | −0.66 (0.43) |
Does not meet CMD criteria | 0.38 (0.31) | 0.18 (0.27) |
Other diagnosis | 0.06 (0.11) | 0.16 (0.10) |
LTC (reference category no self-reported LTC) |
||
Asthma | 0.17 (0.14) | 0.21 (0.12) |
Cancer | 0.48 (0.55) | 0.03 (0.48) |
Chronic musculoskeletal | 1.56*** (0.26) | 1.15*** (0.23) |
COPD | 1.92*** (0.51) | 1.35** (0.45) |
Cardiovascular | 0.10 (0.22) | −0.05 (0.20) |
Diabetes | 0.77** (0.29) | 0.24 (0.25) |
Epilepsy | −0.07 (0.43) | 0.04 (0.38) |
Severe mental health problems | 3.36*** (0.51) | 2.58*** (0.45) |
Other LTC | 0.67*** (0.13) | 0.52*** (0.11) |
Constant | 3.74*** (0.27) | 3.15*** (0.24) |
Observations | 27 815 | 27 815 |
R-squared | 0.34 | 0.29 |
PHQ-9, Patient Health Questionnaire (depression measure); GAD-7, Generalised Anxiety Disorder (anxiety measure); WSAS, Work and Social Adjustment Scale; IMD, index of multiple deprivation (quintile groups); OCD, obsessive-compulsive disorder; PTSD, post-traumatic stress disorder; CMD, common mental disorder; LTC, long-term condition; COPD, chronic obstructive pulmonary disease.
* P<0.05;
** P<0.01;
*** P<0.001.
Analysis of demographic variables
Age was significantly correlated with both outcomes, although the association was weak, equivalent to a reduction of 0.01 score points per year increase. Gender did not correlate with either measure. In contrast, unemployment was associated with higher average post-treatment distress; with an increase of 0.68 points for PHQ-9 and 0.54 points for GAD-7. Patients from South Asian backgrounds, dual heritage and other ethnicities also had higher average post-treatment distress in comparison with patients from White British backgrounds. The IMD quintiles also show significant associations in the expected direction: patients living in more socioeconomically deprived areas (as defined by their home postcode) tended to have greater symptom severity at the end of treatment, and there was an increasing trend in mean post-treatment scores for each quintile of deprivation.
Analysis of treatment-related variables
Differences attributable to taking medication were small and not statistically significant. The number of referrals for psychological treatment (over the past 5 years) was significantly associated with post-treatment outcomes. Each additional referral for care predicted an increase of 0.59 points for PHQ-9 and 0.55 for GAD-7. Since up to 11 referrals were observed in the data-set, this could account for substantially greater post-treatment symptom severity for patients with multiple treatment episodes. A greater number of treatment contacts (in the index treatment episode) was associated with lower average post-treatment distress; decreasing this by −0.25 PHQ-9 points per contact attended (−0.22 for GAD-7).
Some differences between diagnostic categories were observed. A patient with a diagnosis of depression (the reference category) will finish therapy with a predicted PHQ-9 score of 9.69 (s.e.= 0.08, marginal mean) and GAD-7 score of 8.45 (s.e. = 0.07). Some diagnoses were associated with higher post-treatment distress in comparison with the above reference scores; these were obsessive–compulsive disorder (GAD-7), post-traumatic stress disorder (PHQ-9 and GAD-7) and eating disorders (PHQ-9). Diagnoses associated with lower predicted distress levels compared with depression were generalised anxiety disorder (PHQ-9), panic disorder (PHQ-9) and somatoform disorders (PHQ-9). As expected, baseline severity (PHQ-9, GAD-7) and functional impairment (WSAS) measures were significantly correlated with post-treatment outcomes, such that individuals who were more severely impaired were expected to have higher post-treatment distress severity.
Analysis of long-term conditions data
After controlling for all of the above demographic and clinically relevant variables, five long-term condition categories were associated with higher post-treatment distress levels: chronic musculoskeletal problems, COPD, severe mental health problems, diabetes, and ‘other’ non-specified conditions. Figure 1 presents predicted marginal post-treatment outcome scores and Sidak-adjusted 95% confidence intervals; this shows that estimated post-treatment means differed substantially across the categories of long-term conditions. The figure also presents the estimated treatment effect sizes corresponding to each long-term condition category, by comparison with the reference group without any self-reported long-term conditions (and in relation to non-explained variance in the regression model; RMSE PHQ-9 = 5.82; RMSE GAD-7 = 5.14). The effect sizes for those five long-term condition categories, associated with poorer outcomes, range from small (around d = 0.20) to medium (around 0.50; according to Cohen Reference Cohen18 ).

Fig. 1 Predicted post-treatment anxiety (Generalised Anxiety Disorder scale (GAD-7)) and depression (Patient Health Questionnaire (PHQ-9)) scores across long-term condition (LTC) groups
Marginal post-treatment scores derived from seemingly unrelated regression model (Table 3) jointly predicting GAD-7 (a) and PHQ-9 (b) for a patient starting with mean age = 38.38, PHQ-9 = 15.17 and GAD-7 = 13.38 scores. The y-axes of both plots present the potential range in scores and the effect sizes (Cohen's d) are based on comparisons with the ‘no long-term condition’ category and the root mean square error of the respective part of the regression model.
a. Coefficient significant (see Table 3). COPD, chronic obstructive pulmonary disease; Other, other non-specified LTC.
Sensitivity and secondary analyses
Our sensitivity analyses found no evidence of DIF on PHQ-9 or GAD-7 across long-term condition groups. The maximal difference in Pseudo-R 2 values across regression models was 0.001, which is far smaller than the recommended cut-off of 0.035, Reference Crane, Gibbons, Ocepek-Welikson, Cook, Cella and Narasimhalu17 indicating that only very small amounts of variance in item responses were related to specific long-term conditions (online supplement DS1 and Tables DS1–DS8).
The SUR model including a dichotomous variable (long-term condition v. no long-term condition) resulted in an adjusted mean difference of 0.57 (s.e. = 0.09, P<0.001) for the PHQ-9 and 0.42 for GAD-7 (s.e. = 0.08, P<0.001), which speaks for a general disadvantage of this population with long-term conditions after controlling for potentially confounding variables. However, this effect is rather small (ES PHQ = 0.10 and ES GAD = 0.08; coefficients standardised on regression RMSE) and therefore modelling post-treatment outcomes for each specific long-term condition is more informative. When we repeated the SUR analysis in the separate samples of individuals that finished treatment after low- or high-intensity interventions, the results (online supplement DS2 and Table DS9) were largely consistent with the main SUR model shown in Table 3. The only differences were found for people with COPD and diabetes. COPD continued to be associated with higher post-treatment PHQ-9 (B = 3.81, P<0.001) and GAD-7 (B = 2.34, P<0.01) scores after high-intensity interventions, but not after low-intensity ones (PHQ-9: B = 0.90, P>0.05; GAD-7: B = 0.75, P>0.05). Diabetes continued to be associated with higher post-treatment PHQ-9 (B = 1.30, P<0.01) but not with GAD-7 (B = 0.50, P>0.05) scores after high-intensity interventions; no such associations were found for individuals that only accessed low-intensity interventions (PHQ-9: B = 0.43, P>0.05; GAD-7: B = 0.00, P>0.05).
Table 4 shows the results of the logistic regression model predicting the likelihood of receiving high-intensity therapy. As expected, patients with most long-term conditions had a significantly higher probability of receiving high-intensity interventions (odds ratios (OR) = 1.23–1.66; all P<0.05). The only exceptions to this were found for patients with cardiovascular conditions, epilepsy and severe (psychotic) mental disorders, who were no more likely to receive high-intensity therapy in comparison with those without any self-reported long-term conditions.
Table 4 Probability of accessing high-intensity therapy for patients with long-term conditions a

Long-term condition | Odds ratio (95% CI) |
---|---|
Asthma | 1.23** (1.09–1.39) |
Cancer | 1.66* (1.03–2.65) |
Chronic musculoskeletal | 1.47** (1.17–1.84) |
Chronic obstructive pulmonary disease | 1.60* (1.04–2.47) |
Cardiovascular | 1.15 (0.94–1.40) |
Diabetes | 1.39* (1.07–1.79) |
Epilepsy | 0.99 (0.68–1.44) |
Severe (psychotic) mental health problems | 1.00 (0.64–1.57) |
Other non-specified long-term condition | 1.30*** (1.17–1.46) |
a. All coefficients controlled for demographic and treatment-related variables as for the main analysis (see Table 3).
* P<0.05;
** P<0.01;
*** P<0.001.
Discussion
The overall goal of this study was to compare the effects of routinely delivered psychological care for patients with and without self-reported long-term conditions. We found that patients with certain long-term conditions tend to finish psychological treatment with greater depression and anxiety severity. In comparison with patients without long-term conditions, this trend was statistically significant for musculoskeletal problems (effect sizes d = 0.22–0.27), COPD (d = 0.26–0.33), diabetes (d = 0.05–0.13), severe mental health problems (d = 0.50–0.58) and ‘other’ non-specified conditions (d = 0.10–0.11). These findings converge with some observations of small to moderate effect sizes reported by meta-analyses of psychological interventions. Reference Williams, Eccleston and Morley6,Reference Coventry and Gellatly19–Reference van der Feltz-Cornelis, Nuyen, Stoop, Chan, Jacobson and Katon21 Furthermore, patients with most types of self-reported long-term conditions (except cardiovascular conditions, epilepsy and severe mental disorders) were significantly more likely to receive more intensive and costly psychological interventions, consistent with their higher level of impairment and symptom severity. Accessing high-intensity therapy continued to be associated with higher average post-treatment distress in secondary analyses, indicating that patients with long-term conditions are not necessarily better off after high-intensity (v. low-intensity) care in this primary care setting. We noted that, in particular, severe mental health problems (such as psychotic disorders) tended to be strongly associated with poorer outcomes, although the prevalence of such conditions was very small (0.5%) given the service's remit to offer treatment for common mental health problems. This evidence may indicate that the intensity and type of interventions offered in this primary care setting are clearly inadequate to improve psychological distress symptoms in people with a history of severe and enduring (i.e. psychotic) mental disorders. It is also plausible that those with severe mental disorders were identified as such during the early phases of low-intensity interventions and appropriately referred onwards to secondary care/psychiatric services; hence explaining why these individuals were not more likely to access high-intensity therapies in this primary care setting.
A range of other demographic and clinical factors were also associated with post-treatment outcomes. Baseline severity and impairment measures, employment status, socioeconomic deprivation and age have been shown to predict outcomes in comparable clinical samples and settings. Reference Delgadillo, Asaria, Ali and Gilbody22–Reference Firth, Barkham, Kellett and Saxon24 In addition, the present results also indicate that patients from certain ethnic backgrounds (South Asian, dual heritage) and diagnostic groups (obsessive–compulsive disorder, post-traumatic stress disorder and eating disorders) may be at increased risk of poor treatment outcomes.
The overall prevalence of self-reported long-term conditions in this cohort of patients receiving psychological treatment (23.2%) was comparable with general adult population estimates (20%) from England. Reference Weich, Bebbington, Rai, Stranges, McBride and Spiers1 Asthma was the most prevalent (identifiable) long-term condition (around 6% of patients); which may reflect the common coexistence of this condition with anxiety-related problems. However, a comparison of specific categories reveals a disproportionately small representation of certain conditions such as hypertension (1.7% in our sample v. 14.3% in the general population), musculoskeletal problems (1.8% v. 14.0%), diabetes (1.5% v. 3.8%), heart failure (0.1% v. 2.0%) and COPD (0.5% v. 1.8%). These discrepancies possibly indicate that patients with certain conditions are much less likely to access psychological care; for example, the large discrepancy for hypertension indicates a ratio of 1:12. This discrepancy might in some cases be explained by the existence of specialist teams for people with long-term conditions; for example, the local area for this cohort of patients had two specialist musculoskeletal treatment services. However, it is possible that some patients with long-term conditions commonly treated in primary care clinics may be inadequately screened or seldom referred for mental health treatment. Recent research indicates that this may be the case for some patients with coronary heart disease and diabetes. Reference Coventry, Hays, Dickens, Bundy, Garrett and Cherrington25
Strengths and limitations
This large (n = 28 498) naturalistic cohort was adequately powered to assess the predictive value of multiple demographic and clinical variables, which was optimally modelled using joint-prediction of correlated outcomes (PHQ-9, GAD-7). Our analyses additionally contained another layer of robustness tests. It is important to establish measurement invariance to have confidence that outcome questionnaires can be interpreted similarly across different patient groups. Reference Böhnke and Croudace26,Reference Teresi, Ocepek-Welikson, Kleinman, Eimicke, Crane and Jones27 Our sensitivity analyses found no evidence of DIF in PHQ-9 or GAD-7, which indicates that results of these outcome measures have the same meaning across long-term conditions and non-long-term condition groups in this sample. This finding supports the notion that post-treatment outcome differences between long-term condition groups are likely to represent actual differences in psychological distress, rather than measurement error or confounding of long-term condition symptoms with mental health symptoms.
Some limitations to note include the reliance on self-report of long-term conditions, as is common in large cohort and epidemiological studies. Reference Weich, Bebbington, Rai, Stranges, McBride and Spiers1,Reference Patten, Beck, Kassam, Williams, Barbui and Metz28 In particular, we did not have more specific information about the types of long-term condition of patients who endorsed the ‘other’ category in assessment records. Presenting problem categories for mental health issues were also likely to be error prone, since these were ascertained using brief screening measures 12 rather than structured diagnostic interviews. Future studies that gather long-term condition diagnoses recorded in medical records and structured diagnostic interviews may render more precise outcome prediction estimates. A further caveat is that the data from this study, albeit large, came from a single site in the north of England. Future replication studies using data from similar stepped-care services in other regions would enable us to assess the extent to which these findings are generalisable.
Implications for clinical practice
The impact of, and need for, integrated mental health services has often been discussed from a medical perspective, i.e. how psychological professionals could be brought into medical contexts. Reference Coventry, Lovell, Dickens, Bower, Chew-Graham and McElvenny29,Reference McDaniel and deGruy30 Specialist medical knowledge about long-term conditions is a key element of success and the integration of medical expertise within mental health services could also help to improve treatment outcomes in these settings. Our observations raise questions about the effectiveness of routinely delivered stepped-care psychological treatments for people with comorbid diabetes, COPD and chronic pain. These results also mirror findings from research into health-related quality of life (QOL), where it is also found that certain long-term conditions (such as chronic pain) and especially having multiple long-term conditions can considerably undermine QOL and exacerbate psychological distress. Reference Tyack, Frakes, Barnett, Cornwell, Kuys and McPhail31 Such studies highlight the importance of multidisciplinary care aiming to target multiple facets of well-being, adjustment and QOL. It may be particularly important to offer integrated multidisciplinary care for people with specific conditions described above. For example, collaborative care interventions can enhance self-management of depression symptoms for patients with diabetes and coronary heart disease. Reference Coventry, Lovell, Dickens, Bower, Chew-Graham and McElvenny29 Overall, we conclude that standard stepped-care interventions are insufficient to support patients with multimorbidity, especially if delivered in isolation from other healthcare specialists. Our observations concur with recent calls for closer integration of physical and mental healthcare. Reference Naylor, Das, Ross, Honeyman, Thompson and Gilburt32
Healthcare economies and policy-makers should systematically investigate the prevalence of long-term conditions in people using mental health services. This information could be crucial to design and deliver more integrated care for patients with long-term conditions, but can also serve to understand how the demographic and clinical profile of local populations could have an impact on service outcomes. This aspect is still underexplored, especially when thinking about new benchmarking models and quality indicators within primary care psychological services. Reference Delgadillo, McMillan, Leach, Lucock, Gilbody and Wood33
Funding
This study was funded by Leeds Community Healthcare NHS Trust and commissioned by the Long Term Conditions Project Team, Leeds, UK, January 2016.
Acknowledgements
We thank Peter A. Coventry for reviewing an earlier version of the study manuscript and for helpful advice.
eLetters
No eLetters have been published for this article.