Introduction
In recent years, machine learning (ML) has increasingly been used to enhance the capabilities of products. As a branch of Artificial Intelligence (AI), ML is defined as a set of algorithms that can learn patterns from data and improve performance (Mitchell, Reference Mitchell1999). ML-enhanced products, which include hardware products such as smart speakers and software products such as photo-recognition applications, have the potential to respond appropriately to various situations and handle complex tasks that could only be solved by human intelligence in the past (Sun et al., Reference Sun, Zhou, Wu, Zhang, Zhang and Xiang2020).
In light of the growing universality of ML-enhanced products, the design research community has been actively exploring how to support the conceptual design of ML-enhanced products. Prior literature has revealed the challenges in using ML as a design material (Dove et al., Reference Dove, Halskov, Forlizzi and Zimmerman2017) and the corresponding design strategies (Luciani et al., Reference Luciani, Lindvall and Löwgren2018). Based on traditional design process models such as the Design Thinking Process (DTP) (Foster, Reference Foster2021) model, the process models proposed by IBM (Zarattini Chebabi and von Atzingen Amaral, Reference Zarattini Chebabi, von Atzingen Amaral, Marcus and Rosenzweig2020), Gonçalves and da Rocha (Reference Gonçalves and da Rocha2019), and Subramonyam et al. (Reference Subramonyam, Seifert and Adar2021) have made initial attempts to help designers work with ML. More practically, tools such as Human-Centered AI Canvas (Maillet, Reference Maillet2019), the Value Card toolkit (Shen et al., Reference Shen, Deng, Chattopadhyay, Wu, Wang and Zhu2021), and Delft AI Toolkit (van Allen, Reference van Allen2018) made efforts to assist designers at different stages of designing ML-enhanced products.
Although the above work made a solid first step, a further question raises about how to create user experience (UX) value for ML-enhanced products. UX value refers to the emotions, perceptions, physical and psychological responses, as well as behaviors that are brought about by the product (Mirnig et al., Reference Mirnig, Meschtscherjakov, Wurhofer, Meneweger and Tscheligi2015). Beyond the basic capabilities, UX value offers uniqueness and more opportunities for ML-enhanced products. However, it is a complex cognitive skill for designers to purposefully use ML to enhance the UX of products (Clay et al., Reference Clay, Li, Rahman, Zabelina, Xie and Sha2021). To put it more specifically, the UX value creation of ML-enhanced products requires the use of multidisciplinary design knowledge (Nie et al., Reference Nie, Tong, Li, Zhang, Zheng and Fan2022), and designers need to consider not only the unique characteristics of ML but also numerous complex factors such as the properties of stakeholders and context, which brings cognitive load to designers.
On the one hand, ML's typical characteristics can be summarized as growability and opacity from the perspective of conceptual design, which contributes to the UX value of ML-enhanced products and meanwhile present challenges. Growability comes from ML's lifecycle, in which ML continuously accumulates data and optimizes algorithms, enabling products to provide personalized service. Opacity means that ML's mechanism is not transparent to humans, and incomprehensible information is hidden in a “black box” (McQuillan, Reference McQuillan2018). However, growability makes it difficult to anticipate products’ performance. Additionally, the opaque inference process of ML might confuse stakeholders, thereby hindering their interaction with products.
On the other hand, many other factors could impact the UX values of ML-enhanced products. As suggested by prior work, the UX of ML-enhanced products is affected by stakeholders-related factors (Forlizzi, Reference Forlizzi2018) such as users’ needs and humanistic considerations (Yang, Reference Yang2017). Human-centered AI (HAI) emphasizes the collective needs and benefits of humanity and society (Auernhammer, Reference Auernhammer2020). However, balancing the factors between ML and human brings a heavy cognitive load onto designers (Dove et al., Reference Dove, Halskov, Forlizzi and Zimmerman2017), let alone taking into account the context-related factors. In addition, UX value creation is not a transient process (Kliman-Silver et al., Reference Kliman-Silver, Siy, Awadalla, Lentz, Convertino and Churchill2020), and it requires close coordination among co-creators throughout the entire conceptual design process. Nevertheless, existing design frameworks and processes do not provide designers with the guidance to leverage ML, stakeholders, and context, and they fail to clarify the mechanism for creating the UX value of ML-enhanced products. Meanwhile, current design tools are commonly separated from the ongoing design process, which does not conform to the contiguity principle that can help reduce cognitive load (Moreno and Mayer, Reference Moreno and Mayer2000).
Considering the above issues, this paper aims to facilitate UX value creation for ML-enhanced products in conceptual design by developing the UX value framework and the CoMLUX (Co-creating ML-enhanced products’ UX) design process. Based on the bibliometric analysis, integrative literature review, and concept matrix, concepts related to the UX value of ML-enhanced products were synthesized into an initial framework. To avoid cognitive overload for designers, we invited design experts to provide feedback and rank all concepts into three levels according to the requirement of mastery. The key stages of the design process were distilled based on Material Lifecycle Thinking (MLT) (Zhou et al., Reference Zhou, Sun, Zhang, Liu and Gong2020), and the key activities at each stage were identified by integrating human-centered methods. We then iterated the design process in an expert interview and incorporated design tools to bridge the gap between the process and tools. The framework and process were then applied to an actual design project. The completed project provided preliminary evidence that our work can assist designers in creating UX values for ML-enhanced products. To further improve our work, future efforts could be made to incorporate more practical design experience, extend our framework and process to other design phases, and tailor the UX value framework to designers’ cognitive styles.
This paper makes three major contributions. First, the proposed framework outlines how ML, stakeholders, and context co-create the UX value of ML-enhanced products. This framework identifies the growability and opacity of ML and helps designers systematically comprehend the co-creators, their dimensions, and corresponding properties while avoiding cognitive overload. Second, the CoMLUX design process offers practical guidance for designing ML-enhanced products that are growable and transparent. With the design tools tailored to ML's characteristics, the process helps designers leverage the co-creators by following the five key stages including Capture, Co-create, Conceptualize, Construct, and Cultivate in a cognitively-friendly manner. Finally, the application of the framework and process in an actual project demonstrates the usage methods, advantages, and limitations of our work. Our research could hopefully inspire future research in the conceptual design of ML-enhanced products.
Related work
Recent research has become increasingly interested in the conceptual design of ML-enhanced products. Numerous research institutes and commercial organizations have investigated the conceptual design process and developed conceptual design tools for ML-enhanced products.
Design research about ML and UX
The increasing ubiquity of ML encourages the research community to explore how to integrate ML into products. The idea of ML as design material assumed that ML is a tough design material to work with (Holmquist, Reference Holmquist2017), and designers are supposed to understand the characteristics of ML for design innovations (Rozendaal et al., Reference Rozendaal, Ghajargar, Pasman, Wiberg, Filimowicz and Tzankova2018). Furthermore, Benjamin et al. (Reference Benjamin, Berger, Merrill and Pierce2021) advocated utilizing the uncertainty of ML as a design material that constitutes the UX of ML-enhanced products. These claims agree that ML is the creator of ML-enhanced products’ UX instead of an add-on technical solution. As a design material, the ability of ML to process large amounts of data is remarkably higher than that of human and traditional technology (Holmquist, Reference Holmquist2017). However, ML struggles to accomplish cognitive tasks due to its lack of common sense about the real world (Zimmerman et al., Reference Zimmerman, Tomasic, Simmons, Hargraves, Mohnkern, Cornwell and McGuire2007). Prior work believed that the capability uncertainty and output complexity of ML lead to unique design challenges (Yang et al., Reference Yang, Steinfeld, Rosé and Zimmerman2020). On the one hand, the capability of ML can evolve as algorithm updates and data accumulates. As a result, ML sometimes works against human expectations or even makes bizarre errors (Zimmerman et al., Reference Zimmerman, Tomasic, Simmons, Hargraves, Mohnkern, Cornwell and McGuire2007). On the other hand, the prediction outcome of ML contains several possibilities (Holbrook, Reference Holbrook2018) and is less interpretable (Burrell, Reference Burrell2016; Kliman-Silver et al., Reference Kliman-Silver, Siy, Awadalla, Lentz, Convertino and Churchill2020). Any potential bias or abuse of data may result in unfair results (Yang et al., Reference Yang, Scuito, Zimmerman, Forlizzi and Steinfeld2018b). These characteristics of ML might not only lead to negative UX but also be harmful to humans.
To mitigate the potential harm caused by AI, HAI aims to serve the collective needs and benefits of humanity (Li, Reference Li2018). Researches such as humanistic AI and responsible AI (Auernhammer, Reference Auernhammer2020) emphasize that the relationship between humans and AI should be considered at the beginning of design (Riedl, Reference Riedl2019). In recent years, the European Union, Stanford University, and many other organizations have established research institutes focused on HAI, exploring the design, development, and application of AI (Xu, Reference Xu2019). For example, several HAI frameworks have been proposed (see Table 1). Besides, by combining cross-disciplinary knowledge such as cognitive science (Zhou et al., Reference Zhou, Yu, Chen, Zhou and Chen2018) and psychology (Lombrozo, Reference Lombrozo2009), prior work proposed design principles and strategies to improve algorithm experience (Alvarado and Waern, Reference Alvarado and Waern2018) and avoid information overload (Poursabzi-Sangdeh et al., Reference Poursabzi-Sangdeh, Goldstein, Hofman, Wortman Vaughan and Wallach2021).
Table 1. Typical HAI frameworks

Based on the theory of value co-creation, UX researchers become interested in creating values for ML-enhanced products. Value co-creation theory encourages stakeholders to interact with each other for creating personalized value (Galvagno and Dalli, Reference Galvagno and Dalli2014). With the engagement of AI, the created value becomes more diverse. For example, AI provides new forms of interaction (Zhu et al., Reference Zhu, Wang and Duan2022), enables products to recognize human feelings (Leone et al., Reference Leone, Schiavone, Appio and Chiao2021), and assists humans’ behaviors and decision-making (Abbad et al., Reference Abbad, Jaber, AlQeisi, Eletter, Hamdan, Hassanien, Razzaque and Alareeni2021). Kliman-Silver et al. (Reference Kliman-Silver, Siy, Awadalla, Lentz, Convertino and Churchill2020) further summarized three dimensions of AI-driven UX. Besides, prior work suggested that the UX of ML-enhanced products is co-created by several stakeholders-related factors (Forlizzi, Reference Forlizzi2018) such as users’ needs and humanistic considerations (Yang, Reference Yang2017).
The above researches provide valuable contributions to the UX design of ML-enhanced products, but meanwhile expose challenges. During the design process of ML-enhanced products, leveraging the complex factors of ML, stakeholders, and context can bring a heavy cognitive load onto designers, especially for those who lack systematical knowledge about ML. Furthermore, there is no decent framework currently available to assist designers in organizing related factors and clarifying the mechanism that creates UX value for ML-enhanced products.
Design process models
Early on, the design of ML-enhanced products mostly adopted the traditional design process models. These process models could be divided into activity-based ones and stage-based ones according to their different purposes (Clarkson and Eckert, Reference Clarkson and Eckert2005).
Activity-based design process models focus on problem-solving, providing holistic design strategies or specific instructions for design activities. Clarkson and Eckert (Reference Clarkson and Eckert2005) proposed the process of problem-solving, which includes activities of analysis, synthesis, and evaluation. Focused on a certain design stage, C-Sketch (Shah et al., Reference Shah, Vargas-Hernandez, Summers and Kulkarni2001) and the work of Inie and Dalsgaard (Reference Inie and Dalsgaard2020) supported creative activities in ideation, and the morphology matrix instructed designers to explore combinations of design solutions (Pahl et al., Reference Pahl, Beitz, Feldhusen and Grote2007). The analytic hierarchy process (Saaty, Reference Saaty1987) and controlled convergence (O'Connor, Reference O'Connor1991) guided designers to make design decisions, while TRIZ supported the innovation by identifying and resolving conflicts in design (Al'tshuller, Reference Al'tshuller1999).
Most stage-based design process models follow a certain sequence, and some of them encompass iterative activities. Among them, the DTP is one of the most commonly used process models, including stages of Empathize, Define, Ideate, Prototype, and Test (Foster, Reference Foster2021). To compare commonalities and differences between numerous stage-based process models, these models are mapped into DTP (as shown in Fig. 1). Although some of the models refine or weaken certain stages, they mainly follow the stages of DTP. For example, the Munich Procedural Model split the Ideate stage into “structure task” and “generate solutions” (Lindemann, Reference Lindemann2006), and the Design Cycle Model skipped the Define Stage (Hugentobler et al., Reference Hugentobler, Jonas and Rahe2004).

Fig. 1. Comparison among different stage-based process models.
Despite that traditional design process models provide some guidance, designers need processes that focus more on ML's characteristics and other related factors including context and stakeholders (Yang et al., Reference Yang, Banovic and Zimmerman2018a, Reference Yang, Scuito, Zimmerman, Forlizzi and Steinfeld2018b, Reference Yang, Suh, Chen and Ramos2018c; Yang et al., Reference Yang, Steinfeld, Rosé and Zimmerman2020). To this end, some researchers attempt to modify traditional design processes concerning ML. For example, researchers from IBM extended the Empathize stage and proposed Blue Journey for AI (Zarattini Chebabi and von Atzingen Amaral, Reference Zarattini Chebabi, von Atzingen Amaral, Marcus and Rosenzweig2020). It requires designers to empathize with users of ML-enhanced products, explore the possibilities to use AI, and identify available data and resources. Similarly, following the process of designing user interfaces, the process presented by Gonçalves and da Rocha (Reference Gonçalves and da Rocha2019) offered recommendations regarding methods and technologies for intelligent user interfaces. Besides, Subramonyam et al. (Reference Subramonyam, Seifert and Adar2021) proposed an activity-based process model to promote design ideation with AI. Considering the advocacy that design processes of ML-enhanced products should focus more on stakeholders (Forlizzi, Reference Forlizzi2018) and collaborations (Girardin and Lathia, Reference Girardin and Lathia2017), some researchers have investigated how to promote collaborations among different groups (Cerejo, Reference Cerejo2021).
Although current design process models make efforts to support designers to work with ML, it is not sufficient for the design of ML-enhanced products. According to prior work (Sun et al., Reference Sun, Zhang, Li, Zhou and Zhou2022), the design process of ML-enhanced products is distinct from that of traditional technologies. To optimize the UX of ML-enhanced products, an ideal process should echo the typical characteristics and unique lifecycle of ML. Besides, Green et al. (Reference Green, Southee and Boult2014) claimed that a design process has to clarify possible impact factors about UX, which indicates that the influence of ML, stakeholders, and context should be assessed throughout the conceptual design.
Conceptual design tools
Conceptual design tools are essential for designers to perceive information, inquire about solutions, and communicate with stakeholders. Table 2 summarizes available traditional tools at each stage, and designers could take advantage of these tools in the process of designing ML-enhanced products. For example, Yang et al. (Reference Yang, Zimmerman, Steinfeld, Carey and Antaki2016a) applied Journey Map to identify design opportunities for ML-enhanced products in medical treatment. However, these traditional tools are not adapted to ML's characteristics and lifecycle, which means that designers have to learn about ML on their own, and they cannot assess the effectiveness of ML-enhanced products. To this end, several design tools tailored to ML have been developed to support designers at different design stages.
Table 2. Available traditional design tools in different stages of DTP

For the stages of Empathize and Define, several design tools guide designers to explore UX issues brought by ML. Maillet (Reference Maillet2019) developed the Human-Centered AI Canvas, which is composed of 10 factors including jobs-to-be-done, benefits for humans, etc. By filling this canvas, designers can clarify the uncertainty and risk of each factor. The data landscape canvas proposed by Wirth and Szugat (Reference Wirth and Szugat2020) focused on data-related issues such as data privacy and quality, promoting designers’ empathy with individuals, governments, and enterprises.
For the Ideate stage, design tools help to uncover design opportunities concerning ML. Google (2022a) and Microsoft (Amershi et al., Reference Amershi, Weld, Vorvoreanu, Fourney, Nushi, Collisson, Suh, Iqbal, Bennett, Inkpen, Teevan, Kikin-Gil and Horvitz2019) provide a set of principles and examples to inspire designers’ ideation about ML-enhanced products, involving issues about data collection, mental model, explainability, controllability, etc. To help designers generate AI-powered ideas from existing examples, Jin et al. (Reference Jin, Evans, Dong and Yao2021) concluded four design heuristics including decision-making, personalization, productivity, and security. For the same purpose, the AI table provides a tridimensional view for envisioning AI solutions, which includes the impact area, AI technology, and use-case (Zarattini Chebabi and von Atzingen Amaral, Reference Zarattini Chebabi, von Atzingen Amaral, Marcus and Rosenzweig2020). More recently, the Value Cards toolkit helps design practitioners to leverage factors about models, stakeholders, and social consideration, and optimize existing ideas (Shen et al., Reference Shen, Deng, Chattopadhyay, Wu, Wang and Zhu2021). Apart from that, the Fairlearn toolkit (Agarwal et al., Reference Agarwal, Beygelzimer, Dudik, Langford and Wallach2018) and the AI fairness 360 toolkit (Bellamy et al., Reference Bellamy, Dey, Hind, Hoffman, Houde, Kannan, Lohia, Martino, Mehta, Mojsilović, Nagar, Ramamurthy, Richards, Saha, Sattigeri, Singh, Varshney and Zhang2019) guide design practitioners to assess and mitigate potential unfairness caused by AI during ideation.
To facilitate the Prototype and Test stages of ML-enhanced products, design tools are developed to support people with different expertise. Open-source platforms like TensorFlow (Abadi et al., Reference Abadi, Barham, Chen, Chen, Davis, Dean, Devin, Ghemawat, Irving, Isard, Kudlur, Levenberg, Monga, Moore, Murray, Steiner, Tucker, Vasudevan, Warden, Wicke, Yu and Zheng2016) and ML as a service (MLaaS) platforms (Ribeiro et al., Reference Ribeiro, Grolinger and Capretz2015) like IBM Watson provide diverse functions for users to participate in ML lifecycle and create complex ML algorithms, but they require skilled programing ability. As for non-programing toolkits like Delft AI Toolkit (van Allen, Reference van Allen2018), Wekinator (Fiebrink and Cook, Reference Fiebrink and Cook2010), and Yale (Mierswa et al., Reference Mierswa, Wurst, Klinkenberg, Scholz and Euler2006), they significantly lower the barrier of prototyping ML-enhanced products, but designers can hardly know ML's characteristics or iterate products according to ML's lifecycle. Besides, low-code toolkits for creators like Google AIY (Google, 2022b) and ML-Rapid toolkit (Sun et al., Reference Sun, Zhou, Wu, Zhang, Zhang and Xiang2020) are more flexible and only require rudimentary programing skills.
Existing design tools address several challenges in designing ML-enhanced products, but there is still room for improvement. Most of the tools are oriented towards certain design stages but do not cover the entire design process. Furthermore, according to the contiguity principle, displaying relevant content simultaneously rather than successively could reduce cognitive load (Berbar, Reference Berbar2019). It indicates that design tools and design processes are supposed to facilitate the conceptual design of ML-enhanced products as a whole, but currently, the use of tools is separated from the process.
Methodology
To cope with challenges in designing ML-enhanced products, we followed the methodology below to construct the design framework and design process.
Construct the design framework
The construction of the UX value framework for ML-enhanced products included three phases as shown in Figure 2.

Fig. 2. The construction process of the UX value framework.
Extract concepts
To extract concepts from multidisciplinary literature and design cases, we adopted bibliometric analysis and integrative literature review (Torraco, Reference Torraco2005) in the field of ML-enhanced products.
Referring to the work of MacDonald (Reference MacDonald2019), we first applied bibliometric analysis to extract concepts quantitatively. We searched the ACM Digital Library and Web of Science for relevant literature in the field of HCI using search terms including “AI”, “ML”, “UX”, “interface”, “interaction”, “human-centered”, “design framework”, and “design process”. We manually excluded irrelevant literature, and finally 970 papers including 32 key papers were chosen. The titles, keywords, and abstracts of the 970 papers were collected as corpus for analysis. The corpus was analyzed following the below steps: (1) remove stop words that do not convey actual meanings and break down the remained words into their roots; (2) generate high-frequency terms through the term frequency-inverse document frequency (TF-IDF) (Wu et al., Reference Wu, Luk, Wong and Kwok2008); (3) analyze and visualize the co-occurrence of high-frequency terms to show the relationship among the terms.
Because the algorithmically identified terms are not sufficiently informative, the integrative literature review was applied to further extract concepts. We conducted a second round of literature search through the snowballing technique (Boell and Cecez-Kecmanovic, Reference Boell and Cecez-Kecmanovic2014) using high-frequency terms, and 55 additional key papers and 18 design cases were collected. Based on the high-frequency terms and co-occurrence graph, we read all 87 key papers following a hermeneutic approach, defined concepts, and finally obtained 38 preliminary concepts. Several domain-specific concepts were extended into generic settings. For example, the automation level of vehicles was extended to the automation level of general ML-enhanced products.
Construct the initial framework
The concept matrix, which is usually used to organize concepts through a set of existing theories (Webster and Watson, Reference Webster and Watson2002), was applied to guide the construction of the initial framework. According to the theories of ML as a design material, HAI, and value co-creation (introduced in Section “Design Research about ML and UX”), we distill ML, stakeholders, context, and UX value as components of the concept matrix.
We iteratively reorganized existing concepts using the above-mentioned concept matrix, and finally defined the dimensions and properties of each component. For example, stakeholders include dimensions such as basic information, mental model, etc., while uncertainty and explainability pertain to cognition in UX value. After the above steps, the initial framework consisting of 30 concepts in 4 categories was constructed. Besides, we summarized how to create UX value in response to the growability and opacity of ML, providing inspiration for the design process.
Iterate the framework
To refine the framework, we invited eight design experts (with at least 5 years of experience designing ML-enhanced products) to evaluate the initial framework and rank all the concepts. Experts first read the description of the framework independently, and they were required to think aloud (van den Haak et al., Reference van den Haak, De Jong and Jan Schellens2003) while reading. After that, experts evaluated the clarity, coherence, and completeness of this framework following a set of laddering questions (Grüninger and Fox, Reference Grüninger and Fox1995). Additionally, to avoid cognitive overload for designers, experts ranked the concepts into three levels, Know, Understand, and Apply, based on the requirements of mastery.
The evaluation results revealed that all participants held a positive attitude towards this framework, stating that it was concise and could effectively direct designers to envision ML-enhanced products. Furthermore, we iterated the initial framework to the final version (see Section “The UX value framework”) according to feedback. The main modifications include: (1) Separating critical properties into independent dimensions. For example, separating “social environment” as an independent dimension from the “task type” dimension in context. (2) Expanding some dimensions with more properties. For example, expanding stakeholders’ “familiarity of technology” to “mental model”, so as to incorporate properties related to the usage experience of ML-enhanced products. (3) Enriching concepts with examples. We introduce representative types of stakeholders and context and use examples to help designers understand different dimensions.
Construct the design process
Identify the key stages
Based on MLT, we determined the key stages of the design process. MLT regards ML as a growable design material with its own lifecycle, during which the properties of ML change as ML interacts with co-creators, thus affecting the performance of products (Zhou et al., Reference Zhou, Sun, Zhang, Liu and Gong2020). The gray cycle in Figure 3 illustrates the main steps of MLT using an example of how a potted plant is cultivated. As shown in Figure 3, each stage of the process corresponds to a step of MLT. For example, at the third step of MLT, designers are prompted to illustrate the lifecycle of the material, and outline how co-creators change and interact in the lifecycle. Similarly, the third stage Conceptualize requires designers to envision the whole ML lifecycle and explore design solutions.

Fig. 3. The inner gray cycle illustrates the main steps of MLT. The outer red cycle shows the identified key stages based on MLT.
Identify the key activities
Referring to human-centered design methods such as participatory design, we specified design activities at each design stage. The identification of activities obeyed the following principles: (1) Refer to existing design process models and design practice. For example, by combining the insights in guidelines for AI (Amershi et al., Reference Amershi, Weld, Vorvoreanu, Fourney, Nushi, Collisson, Suh, Iqbal, Bennett, Inkpen, Teevan, Kikin-Gil and Horvitz2019) and People + AI Handbook (People+AI Research, 2022), design activities such as user research were added to the corresponding stage. (2) Implement the core ideas of MLT. For example, at the stage of Co-create, designers identify touchpoints and relationships between co-creators. (3) Encourage the participation of stakeholders. For example, the Construct stage adopted the evaluation matrix and functional prototype, driving stakeholders to select desired design solutions. (4) Guide designers to integrate the dynamically changing co-creators into the ML lifecycle. For example, the activities at Cultivate stage require designers to monitor the delicate changes of stakeholders and context and develop strategies to cope with these changes.
Iterate the process
To integrate practical design experience and iterate the design process, the eight design experts involved in the framework iteration were also invited to evaluate the design process. Experts were first required to introduce the design process of their prior design projects. Then they evaluated the CoMLUX design process, putting forward suggestions for improvement as well as supplementing design activities.
According to the suggestions from experts, we modified the CoMLUX process as follows: (1) Adjust the sequence of some design activities. For example, “select tested solutions” was moved to the Conceptualize stage. (2) Supplement specific design strategies at some stages. For example, introducing brainstorming in “explore design opportunities”, and regulating data collection in “research co-creators”. (3) Explain how designers collaborate with other stakeholders (e.g., developers and maintenance personnel) at each stage.
Incorporate design tools
Based on the iterated design process, we incorporated two design tools proposed in our prior work. Transparent ML Blueprint (Zhou et al., Reference Zhou, Li, Zhang and Sun2022) aims to address the opacity of ML, and ML Lifecycle Canvas (Zhou et al., Reference Zhou, Sun, Zhang, Liu and Gong2020) illustrates the growability of ML. The specific introduction to each tool can be found in Section “Overview of conceptual design tools”. To incorporate the tools into the CoMLUX design process, for each tool, we interviewed six designers who had used it, and asked for their opinions about which stages should the tool be applied to. By integrating design tools with the CoMLUX design process, designers can use different tools seamlessly throughout the design process (see Section “The CoMLUX design process”), which is consistent with the contiguity principle.
The UX value framework
This section introduces the UX value framework, which defines ML, stakeholders, and context as co-creators, and identifies representative UX values (see Fig. 4). Concentrating on the growability and opacity of ML, this framework helps designers to understand how co-creators contribute to UX values.

Fig. 4. The UX value framework.
Combining the knowledge level of AI (Newell, Reference Newell1982) and the hierarchical model of design knowledge (Kolarić et al., Reference Kolarić, Beck and Stolterman2020), we adopted a differentiation approach to describe the requirements for mastery of concepts in the framework, including levels of Know, Understand, and Apply. The description of the three levels is as follows:
• Know: designers only need to know the basic meaning of concepts, so as to collaborate with engineers or other stakeholders.
• Understand: designers have to specify the details or rationales of concepts.
• Apply: designers should be capable of implementing the concept when designing ML-enhanced products.
The framework of ML
The framework of ML applied a hierarchical approach to elaborate the dimensions, properties, as well as the typical characteristics of ML (see Fig. 5).
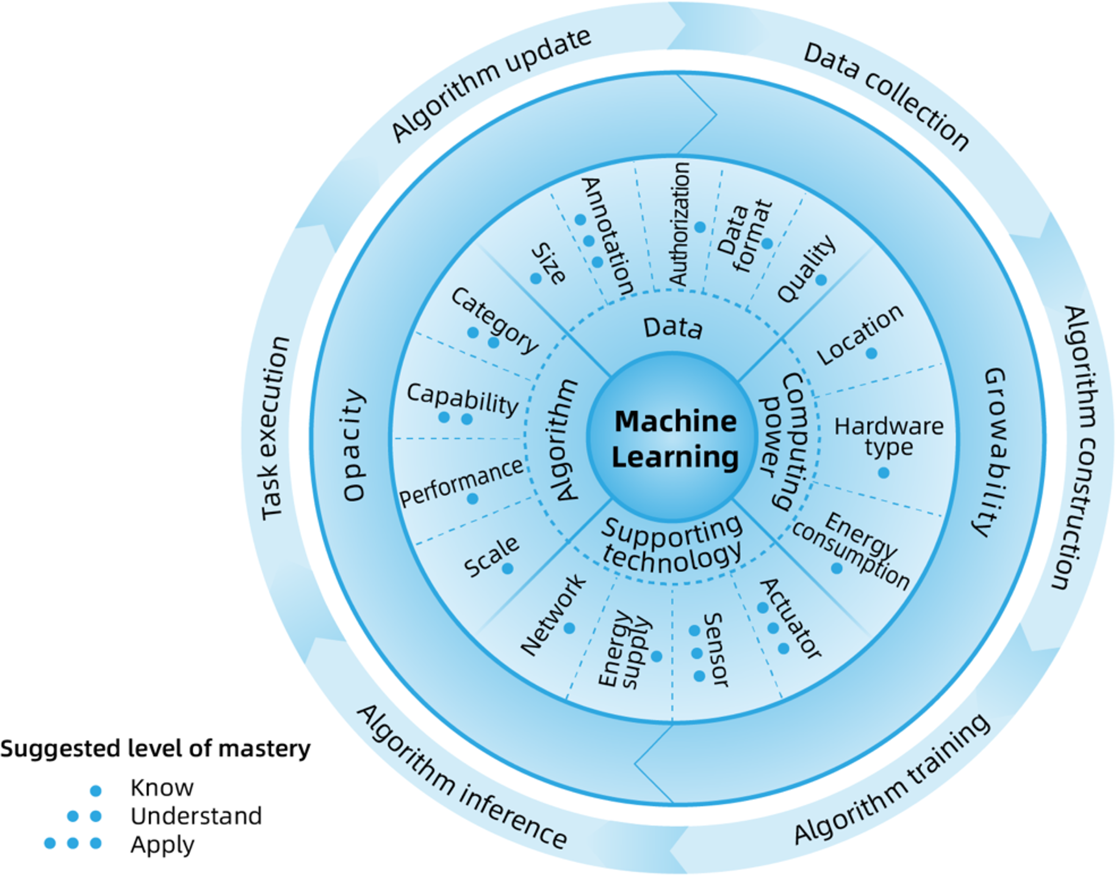
Fig. 5. The framework of ML and the suggested level of mastery for the properties.
Dimensions and properties of ML
Data, algorithms, computing power, and supporting technology work together to support the functioning of ML, and they constitute the four dimensions of ML: (1) data: collected data for algorithm training, inference, updating, and task execution; (2) algorithm: specific methods that enable ML to gain intelligence from the given data and solve problems, for example, neural network; (3) computing power: the resource for algorithm training and inference; (4) supporting technology: other technologies to support the functioning of products. Each dimension contains several properties. Figure 5 introduces the properties of different dimensions and the corresponding level of mastery. For example, properties of data include size, annotation, authorization, data format, and quality.
The four dimensions are not isolated but collaborate to support the ML lifecycle, which includes the following steps: (1) data collection: to collect and annotate data; (2) algorithm construction: to construct algorithms to learn patterns from data; (3) algorithm training: to train algorithms with data based on computing power; (4) algorithm inference: to make inference based on input data; (5) task execution: to execute tasks with the help of algorithms and supporting technologies; (6) algorithm update: to collect new data and feedback to improve algorithm performance. Within the lifecycle, ML empowers products to learn and make decisions autonomously.
Typical characteristics of ML
From the perspective of designing ML-enhanced products, the typical characteristics of ML could be summarized as growability and opacity. The two characteristics are closely related to the creation of ML-enhanced products’ UX value.
Growability
ML's growability, primarily due to its learning mechanism, brings benefits and challenges to ML-enhanced products (Yang et al., Reference Yang, Steinfeld, Rosé and Zimmerman2020). Growability allows ML-enhanced products to constantly evolve, which could be analogized to the cultivation of a tree. With the gardener's nurture, the tree is able to adapt to changing weather as water and nutrients accumulate. Similarly, during the ML lifecycle, the performance of ML improves through learning data from stakeholders and context, thus creating UX value. However, it is difficult to cultivate a growable “tree” into a pre-determined shape. In other words, the continuously changing performance of ML prevents the creation of the desired UX value.
Specifically, the challenges brought by the growability of ML are twofold. On the one hand, the performance of ML can be easily influenced by the size and quality of data. Meanwhile, more data accumulates during the usage process of ML-enhanced products. Such uncertainty makes it difficult to anticipate the behavior of ML-enhanced products and their UX value. On the other hand, ML-enhanced products can outperform humans in certain tasks but sometimes they cannot complete tasks independently. Therefore, it is crucial to flexibly adjust the collaboration between humans and ML-enhanced products according to different tasks.
Opacity
The opacity of ML-enhanced products mainly comes from the hidden decision-making process and the unexplainable inference results. Traditional products usually follow human-understandable rules, and their working mechanism is transparent to humans. However, through synthesizing information such as the physical environment and user intention, ML-enhanced products could make inferences without human intervention (Schaefer et al., Reference Schaefer, Chen, Szalma and Hancock2016). The decision-making process involves complex parameters, which are hidden in a black box and opaque to humans.
The opacity of ML brings several challenges to the conceptual design of ML-enhanced products. First, it may hinder humans from understanding the performance of products and subsequently impact their trust, especially in contexts such as autonomous driving. Second, opacity challenges the collaboration between stakeholders and products. For example, when a product encounters a problem that requires human assistance, stakeholders could not take over in time if they are not informed of the product's status. Third, the opaque algorithm training process may impede stakeholders from providing needed data or necessary assistance, thus affecting the training progress.
The framework of stakeholders
Stakeholders refer to those who can either affect or be affected by ML-enhanced products. In conceptual design, designers should clarify the involved stakeholders and take their needs and rights into consideration. This framework illustrates the dimensions and properties of stakeholders as well as typical stakeholders (see Fig. 6).

Fig. 6. The framework of stakeholders and the suggested level of mastery for the properties.
Dimensions and properties of stakeholders
When interacting with ML-enhanced products, different stakeholders may exhibit various characteristics. For example, stakeholders differ in their capabilities to utilize information and understand products’ behavior (Tam et al., Reference Tam, Huy, Thoa, Long, Trang, Hirayama and Karbwang2015). Therefore, the design of ML-enhanced products should consider stakeholders’ dimensions. The dimensions of stakeholders include (1) basic information: mainly includes stakeholders’ age, gender, occupations, etc. (2) function need: the function of ML-enhanced products needed by stakeholders (Shen et al., Reference Shen, Deng, Chattopadhyay, Wu, Wang and Zhu2021); (3) mental model: experience of using ML-enhanced products and familiarity of related technologies (Browne, Reference Browne2019); (4) ethical consideration: consideration about ethical issues such as privacy and fairness; (5) esthetic preference: stakeholders’ consideration about the appearance of products (Li and Yeh, Reference Li and Yeh2010); (6) lifecycle: properties’ changes within the lifecycle. For example, during the usage of products, end-users’ mental models may change and new function needs may emerge. The corresponding properties of each dimension and their levels of mastery are shown in Figure 6.
Types of stakeholders
This section summarizes the most typical stakeholders, including end-users, bystanders, developers, maintenance personnel, and regulators (Weller, Reference Weller2019). It should be noted that different types of stakeholders are not mutually exclusive, which means that a single individual may play multiple roles. In addition, stakeholders do not necessarily have a preference for all dimensions.
End-users
End-users refer to those who use ML-enhanced products in their daily life. The basic information of end-users often affects the product definition. As for function needs, end-users usually need an intuitive interface to interact with the product more effectively. For the mental model, most end-users possess limited expertise about the mechanisms and supporting technology of ML, but some of them may have used ML-enhanced products in the past. Additionally, they are concerned about data privacy and want to know how their data are being used. In some cases, end-users should also include elderly and disabled users (Kuner et al., Reference Kuner, Svantesson, Cate, Lynskey and Millard2017), whose function need and mental model are somewhat different from others. End-users are encouraged to actively acquire relevant knowledge, so as to interact with ML-enhanced products legally and efficiently.
Bystanders
Bystanders do not proactively interact with ML-enhanced products (Tang et al., Reference Tang, Finke, Blackstock, Leung, Deutscher and Lea2008), but they may perceive end-users’ interaction with products and be affected by it (Montero et al., Reference Montero, Alexander, Marshall and Subramanian2010). In terms of function needs, bystanders might want to know the product's behavior and intention to avoid being affected. For example, pedestrians as bystanders need to know the intention of autonomous vehicles (Merat et al., Reference Merat, Louw, Madigan, Wilbrink and Schieben2018) in case of traffic accidents. As for ethical considerations, bystanders are particularly concerned about privacy issues. For example, smart speakers sometimes inadvertently record conversations from bystanders, which can negatively impact the product's social acceptance. When confronted with these situations, bystanders could take the initiative to protect their legitimate rights.
Developers
Developers refer to those who take part in the development of products, including ML experts, data scientists, etc. Typically, they need to comprehensively know the purpose and behavioral details of algorithms, compare different algorithms (Mohseni et al., Reference Mohseni, Zarei and Ragan2018), or even develop new algorithms for products. In terms of the mental model, developers are familiar with ML and able to understand abstract explanations of algorithms. As for ethical considerations, developers desire unbiased data and a clear mechanism of accountability, and they should strictly follow relevant regulations. For example, at the steps of data collection and task execution, they are responsible for ensuring the completeness and accuracy of data and avoiding bias.
Maintenance personnel
Maintenance personnel includes engineers and designers who maintain the service of ML-enhanced products. Their function needs include accurate data and diverse user feedback for identifying errors and improving UX. Meanwhile, maintenance personnel need information about products’ performance and working status (Kim et al., Reference Kim, Wattenberg, Gilmer, Cai, Wexler, Viegas and Sayres2018), so that they can collaborate with developers to refine algorithms. The mental model of maintenance personnel is similar to that of developers, with a more in-depth understanding of explainable algorithms. As for ethical considerations, they would continuously pay attention to the social impact caused by algorithms and timely identify ethical issues such as algorithm bias. Maintenance personnel is supposed to ensure the growability of ML and monitor the status of products to avoid risk and breakdowns.
Regulators
Regulators (e.g., legislators, government) audit the behavior and legal liability of ML-enhanced products (Weller, Reference Weller2019), thus making products ethically and socially responsible (Blass, Reference Blass2018). Regulators need information about products’ working environment, working process, performance, etc. As for the mental model, regulators have to comprehensively understand the capabilities and potential risks of ML. Regulators should pay particular attention to the ethical issues and social impact brought by ML-enhanced products, and they are obligated to determine whether a product could be used in public society (Mikhail et al., Reference Mikhail, Aleksei and Ekaterina2018).
The framework of context
The design of ML-enhanced products is highly related to the context (Springer and Whittaker, Reference Springer and Whittaker2020), which describes the environment of products and the activities that happened during a period of time. Figure 8 hierarchically illustrates the dimensions of contexts and corresponding properties as well as the most typical contexts.
Dimensions and properties of the context
The design and performance of ML-enhanced products can be influenced by dimensions of context, which include: (1) physical environment: factors in the physical environment that might directly impact the performance of products. For example, inappropriate temperature and humidity may reduce the lifetime of hardware; (2) social environment: factors in the social environment that might impact products (Cuevas et al., Reference Cuevas, Fiore, Caldwell and Laura2007). For example, the regulations and culture of different countries and regions; (3) task type: characteristics of tasks to be accomplished by ML-enhanced products; (4) lifecycle: the changing properties of the context within the lifecycle, such as the frequent change in light and sound. Figure 7 shows the corresponding properties of each dimension.

Fig. 7. The framework of context and the suggested level of mastery for the properties.
Types of contexts
The context of ML-enhanced products can be categorized into four quadrants according to risk and timeliness (see Fig. 8). The four types of contexts are not strictly separated, so designers should determine the type of context according to their design projects. Additionally, in the same type of context, ML-enhanced products can also interact with stakeholders using varying levels of automation.

Fig. 8. Typical types of context.
Context #1: low risk and low timeliness
In context #1, the consequences of failing a task are not severe, and real-time feedback is not necessary. Taking the shopping recommender system as an example, there is no severe consequence even if the recommended content is irrelevant. In addition, the system only needs to recommend the content of interest when users browse next time instead of adjusting the content in real time. In this context, the speed of algorithms can be sacrificed in pursuit of higher accuracy, thus improving the UX value of ML-enhanced products.
Context #2: high risk and low timeliness
For risky but low timely tasks, failure of tasks will probably lead to injury, death, or other serious consequences. In context #2, designers should pay special attention to the transparency of the decision-making process and the explainability of prediction results, so as to reduce potential risks and build trust. According to the research of Pu and Chen (Reference Pu and Chen2006), the risk perceived by users will influence their interaction. Therefore, ML-enhanced products need to help humans assess the risk of current tasks and allow humans to question decisions made by ML (Edwards and Veale, Reference Edwards and Veale2018). As an example, the clinic diagnosis system should inform stakeholders about uncertainties and risks associated with its decisions, allowing patients and doctors to adjust the decision based on the actual situation (Sendak et al., Reference Sendak, Elish, Gao, Futoma, Ratliff, Nichols, Bedoya, Balu and O'Brien2020).
Context #3: low risk and high timeliness
In a less risky but timely context, ML-enhanced products have to respond to stakeholders promptly, and potential errors or misunderstandings will not bring risks. ML-enhanced products could adjust the response time according to the current task and context (Amershi et al., Reference Amershi, Weld, Vorvoreanu, Fourney, Nushi, Collisson, Suh, Iqbal, Bennett, Inkpen, Teevan, Kikin-Gil and Horvitz2019). For example, the accuracy of a chatbot can be reduced to provide immediate response for end-users. Since the interaction between products and end-users is real-time, information overload should also be avoided. Additionally, in context #3, products should allow stakeholders to provide feedback on faults, so as to continuously improve products’ performance (Ashktorab et al., Reference Ashktorab, Jain, Liao and Weisz2019).
Context #4: high risk and high timeliness
Context #4 requires high timeliness, and failure of tasks could lead to serious consequences. Designers should take measures to balance the accuracy and timeliness of ML-enhanced products. Specifically, products have to report unexpected errors immediately (Theodorou et al., Reference Theodorou, Wortham and Bryson2017), helping stakeholders to adjust their expectations of products’ capabilities. For example, in an autonomous driving task, prediction results and alerts (e.g., engine failure) are necessary for end-users to take over in time. Furthermore, the provided information should be concise, because end-users usually need to make decisions immediately when faced with an emergency.
Except for the levels of risk and timeliness, products of different automation levels could cooperate with humans in distinct ways, thus affecting the design of ML-enhanced products (Mercado et al., Reference Mercado, Rupp, Chen, Barnes, Barber and Procci2016; Bhaskara et al., Reference Bhaskara, Skinner and Loft2020). For instance, a sweeping robot is supposed to complete the task fully automatically, whereas a chatbot generally only provides information according to human instructions. Based on the Society of Automotive Engineers (SAE) taxonomy of driving automation (Wright et al., Reference Wright, Chen, Barnes and Boyce2015; Selkowitz et al., Reference Selkowitz, Lakhmani, Larios and Chen2016), we produced a universal taxonomy for general ML-enhanced products, which could be found in Appendix 1.
UX values of ML-enhanced products
As shown in Figure 4, the UX value of ML-enhanced products is co-created by ML, stakeholders, and context. This section introduces six representative UX values and discusses how co-creators bring UX values around ML's characteristics.
Typical UX values
The UX values brought about by ML reveal design opportunities for ML-enhanced products while posing several challenges (see Table 3). The challenges designers faced in creating UX values are mainly related to the growability and opacity of ML. For example, the autonomy of products relies on the evolving ability of algorithms and users’ understanding of algorithm decisions. As a result, products’ autonomy could be influenced by both growability and opacity.
Table 3. Typical UX values of ML-enhanced products

How to create UX values
This section introduces general ways to overcome design challenges and create UX values for stakeholders during the conceptual design of ML-enhanced products with regard to ML's growability and transparency.
Create UX values around growability
The growability of ML is directly related to most UX values. Through learning patterns from massive data, ML-enhanced products could have the autonomy to make decisions independently or assist humans in completing certain tasks. ML-enhanced products could also provide affective support. Growability also enables products to adapt to stakeholders’ habits and meet personalized needs. During the usage process of products, ML will continuously learn from new data, and UX values will also evolve as the lifecycle updates.
To create UX values around growability, designers have to participate in the ML lifecycle and coordinate co-creators. For example, to create learnability value, designers should get involved in steps including data collection, algorithm training, and algorithm update. Ideally, ML could continuously obtain data about the social environment from the context, and stakeholders (especially end-users) could regularly provide feedback data. Using these data, designers are able to improve the performance of ML-enhanced products and refine the created UX values. At the algorithm construction step, for example, the function needs of stakeholders and the task type of context provide a reference for algorithm selection. At the step of data collection, designers should consider regulations in the context, encourage stakeholders to contribute data, and incorporate data into the algorithm training (Yang et al., Reference Yang, Suh, Chen and Ramos2018c).
Create UX values around transparency
To address the challenges brought by opacity (discussed in Section “Typical characteristics of ML”) and create UX values, the transparency of ML-enhanced products is necessary. As the opposite of transparency, opacity hinders the creation of UX values including cognition, interactivity, auxiliary, and learnability. For example, if humans are unable to know the status of a product, they cannot interact with it appropriately, which affects its interactivity and auxiliary. Although improving transparency is effective to build trust (Miglani et al., Reference Miglani, Diels and Terken2016), an inappropriate increase in transparency is dispensable (Braun et al., Reference Braun, Li, Weber, Pfleging, Butz and Alt2020) and might lead to information overload (Chuang et al., Reference Chuang, Manstetten, Boll and Baumann2017). To this end, designers should determine the transparency of products with systematic consideration of co-creators.
ML plays an influential role in determining the transparency of products. To moderately increase transparency, designers can: (1) select explainable algorithms. Generally, the explainability of algorithms decreases as their complexity increases. For example, the linear regression algorithm is easy to explain but it could not handle complex data. (2) apply methods such as explainable AI (XAI) to provide explanations (Du et al., Reference Du, Liu and Hu2019). For instance, by analyzing various inputs and outputs of algorithms, XAI can report how different inputs influence the inference results. (3) disclose information about ML's inference process (Oxborough et al., Reference Oxborough, Cameron, Rao, Birchall, Townsend and Westermann2018), including general demonstrations of how ML processes data (Lyons, Reference Lyons2013) and how ML is performing the analysis. (4) provide background information, which includes the purpose of the current algorithms (Bhaskara et al., Reference Bhaskara, Skinner and Loft2020) and reasons behind breakdowns (Theodorou et al., Reference Theodorou, Wortham and Bryson2017), to help stakeholders understand ML.
Apart from that, stakeholders and context would also impact the transparency of ML-enhanced products. Due to stakeholders’ distinct ability to perceive information, they have different requirements for transparency. For example, in the clinical diagnosis task, well-trained doctors are able to perceive mass information, but patients would struggle to handle complex information. As for context, the task type can also affect the desired transparency of products, and this point has been introduced in Section “Types of contexts” in detail. Meanwhile, certain regulations, such as the EU's General Data Protection Regulation, have forced products to implement transparency at a legal level.
The COMLUX design process
Within the CoMLUX design process, designers coordinate three co-creators (ML, stakeholders, and context) to create UX values for ML-enhanced products. This process provides specific strategies for designers to consider the growability and opacity of ML, and to apply the UX value framework systematically.
Overview of the conceptual design process
The CoMLUX design process comprises five design stages: Capture, Co-create, Conceptualize, Construct, and Cultivate. Each stage includes specific design activities, such as making trade-offs and collecting related information (see Fig. 9).

Fig. 9. The CoMLUX design process.
In terms of growability, this process instructs designers to participate in the ML lifecycle. Through activities such as “map the lifecycle” and “monitor the growing process”, designers could focus on the changes in stakeholders and contexts, and update the conceptual design solutions accordingly.
In terms of opacity, this process guides designers to appropriately improve the transparency of ML-enhanced products. By determining the dimensions of ML based on the properties of stakeholders and contexts, designers are able to cope with the opacity of ML and design appropriate interfaces.
Ideally, this process will result in a mutually supportive ecosystem composed of the three co-creators. The ecosystem will be dynamically updated according to the ML lifecycle and be able to respond to the changes in stakeholders and context agilely.
Overview of conceptual design tools
The two design tools incorporated in this process could support designers in implementing the UX value framework while participating in the CoMLUX process. The Transparent ML Blueprint (Blueprint) copes with the opacity of ML, while the ML Lifecycle Canvas (Canvas) addresses the growability of ML. Traditional tools such as brainstorming and storyboards, as well as prototyping tools such as Delft AI Toolkit and ML-Rapid Kit can be used as a complement to these tools.
Transparent ML Blueprint for transparency
Blueprint contains a visualized ontology framework of design concepts and a supportive handbook. Blueprint helps designers learn about the key concepts of ML, particularly those related to its opacity, and assists designers in defining design goals. Blueprint is composed of seven modules, each representing a key concept about the transparency of ML-enhanced products (see Fig. 10). For each key concept, it contains several sub-concepts. Designers can refer to the handbook for a detailed introduction to each concept. These concepts might interact with each other and are not mutually exclusive. The specific priorities of concepts are case-dependent for any particular design project and agent. In addition to the information related to the opacity of ML, designers are free to complement other relevant information about ML-enhanced products.

Fig. 10. Overview of Blueprint.
The ML lifecycle Canvas for growability
Canvas treats ML as a growable co-creator and guides designers to represent the ML lifecycle and the relationships between co-creators visually. With the help of Canvas, designers can clarify the collaboration and constraints between co-creators, and ideate growable conceptual design solutions.
Canvas creates a visualized diagram containing ML, stakeholders, and context. It consists of the canvas itself, Question List, Persona, and Issue Card (see Fig. 11). The canvas contains six sectors corresponding to the six steps of the ML lifecycle. Designers collect information about the three co-creators at different steps of the lifecycle under the reminder of Question List, which contains questions related to steps of the ML lifecycle and different co-creators. Designers could collect and record user feedback on specific issues in Persona. Details of Canvas could refer to Appendix 2.

Fig. 11. Overview of Canvas.
The core stages of conceptual design
Stage #1: capture
Stage #1 guides designers to gain an initial understanding of the co-creators, capture potential design opportunities, and clarify target specifications such as the UX value. The main activities and usage of tools in stage #1 are shown in Figure 12.

Fig. 12. Main activities and usage of tools in Stage #1.
Understand co-creators
A general understanding of co-creators is the basis for identifying design opportunities. Designers could first read the handbook of Blueprint to understand relevant concepts and their inter-relationships. For each concept, designers could gather information through literature review, user research, etc., and then record the information on sticky notes and paste them into Blueprint. Designers can flexibly arrange the order of information collection according to their actual project. The “Other” option in each module is for additional related information.
Designers need to identify the stakeholders involved in the design project and understand their different dimensions. In particular, they should clarify the functional need and mental models of end-users during conceptual design in order to identify unsolved problems. Designers can collate the collected information and fill it in the “Stakeholder” module.
In addition, designers need to understand the algorithm and data dimensions of ML to support UX value creation. Designers can collect design cases as a reference to explore design opportunities. The collected information can be filled in the “Algorithm” module.
Designers should also conduct research on the product's context, including the physical environment, type of task, etc. Designers can fill most of the collected information in the “Context” module, collate information such as laws and regulations in the “Ethical Consideration” module, and record information related to design goals in the “Design Goal” module.
Explore design opportunities
Designers can explore possible UX values, general constraints, and broad design goals around stakeholders and context. For example, designers can consider that: (1) is the autonomy/auxiliary of products useful in the given context? (2) how to apply ML to a particular context, or (3) which stakeholders could gain better experiences? After that, designers can broadly envision multiple opportunities through brainstorming.
Screen design opportunities
Through comprehensively considering co-creators, designers can preliminarily determine whether a design opportunity deserves in-depth investigation and eliminate less valuable opportunities. The screening criteria could refer to the “Design Goal” module. Designers need to pay particular attention to (1) feasibility: whether ML is feasible to solve the problem, whether there exists available datasets or algorithms, etc. (2) uniqueness: whether ML could solve the problem in a unique way and provide unique UX value.
Define target specifications
Considering the design opportunities, designers should define clear and concise target specifications of a product, which represent the expectations that need to be achieved. At this time, designers only need to generally describe the product's core functions, main stakeholders, contexts, and UX values instead of specific algorithms and data collection methods.
The description of specifications should cover the needs of different stakeholders (Yang et al., Reference Yang, Zimmerman, Steinfeld and Tomasic2016b). For example, in a news-feed application, there is often a trade-off between the needs of end-users (e.g., rapid access to information) and maintenance personnel (e.g., end-user dwell time). Specifically, designers can use the “Design Goal” module to define target specifications, and ultimately keep only the most crucial information in Blueprint and prioritize them.
Stage #2: co-create
This stage requires designers to conduct in-depth research into ML, stakeholders, and contexts, so as to initially clarify the static relationships between co-creators and lay the foundation for building ML-enhanced products with growability and transparency (see Fig. 13).

Fig. 13. Main activities and usage of tools in Stage #2.
Research co-creators
Based on the target specifications, designers conduct further research with the help of Question list and Persona, gaining insight into the co-creators of UX value. Question list can be used as a checklist, and designers are free to alter the list if necessary.
The first step is to learn more about the relevant dimensions of ML based on the target specifications, and complement modules of “Transparent Algorithm”, “Transparent Interface” and “Transparent Content”. For example, in the “Transparent Algorithm” module, designers can preliminarily describe the data and computing power needed by different algorithms.
Secondly, designers could further understand the stakeholders through methods such as interviews and focus groups. Designers should especially clarify different stakeholders’ functional needs and ethical preferences related to opacity and growability. Designers can also identify the overall features of stakeholders through the collected data. The obtained information could be filled into Persona, and designers can supplement missing stakeholders in the “Stakeholder” module of Blueprint.
As for context, designers should thoroughly consider the dimensions of the physical environment, social environment, and task type with the help of the Question List (see Appendix 2). Designers can summarize different types of contexts and document specific content in the “Context” module of Blueprint.
Finally, designers should review the research results to find the missing co-creators and try to transform the documented information into a machine-readable and analyzable format. For example, designers could digitize information about the physical environment and quantify stakeholders’ experiences/feelings.
Locate touchpoints
Touchpoints are the temporal or spatial loci where connections such as data interchange and physical interaction occur between co-creators. By locating the touchpoints, designers can clarify the mechanisms of resource exchange between co-creators at different steps within the ML lifecycle.
To build ML-enhanced products with transparency, designers should: (1) check whether essential information could be exchanged through existing touchpoints; (2) exchange only the necessary information and details to avoid cognitive overload; (3) remove touchpoints that might collect sensitive data or disclose privacy, and retain only the necessary private information.
To build ML-enhanced products with growability, designers can: (1) check whether growability can be achieved by information obtained from existing touchpoints; (2) remove biased touchpoints (e.g., annotators with bias) to avoid data ambiguity, inconsistency, and other unfairness at the data collection step; (3) construct datasets without missing critical data.
Identify co-creation relationships
Designers need to identify the constraints and collaboration between co-creators and map out the static relationships between them. For example, ML provides prediction results to end-users at the step of task execution, and end-users “teach” their preference to ML through interaction. Designers could record these details in the relevant sector of Canvas.
To initially build growable relationships, designers could: (1) build a data-sharing loop, which consists of contributing data, getting feedback, and contributing more data, to realize reciprocity between stakeholders and context; (2) guide stakeholders to share data through incentive strategies such as gratuities and gamification strategies.
To initially build transparent relationships, designers could: (1) provide stakeholders with information about ethical considerations. For example, when a product tracks stakeholders’ behaviors, stakeholders need to be informed; (2) provide access for stakeholders to understand the working mechanisms of products.
Stage #3: conceptualize
With a full understanding of co-creators, the static relationships between them could be developed into dynamic ones within the ML lifecycle. At stage #3, designers are able to explore conceptual design solutions with growability and transparency (Fig. 14).

Fig. 14. Main activities and usage of tools in Stage #3.
Illustrate the lifecycle
Designers need to pay close attention to how co-creators interact through touchpoints and evolve dynamically as the ML lifecycle progresses. For example, continuously monitoring stakeholders’ behaviors in different contexts.
To collect and organize all the changing information regarding co-creators, designers can: (1) map the known properties of different co-creators and their relationships to different sectors of Canvas; (2) explore the unknown areas of Canvas and consider how to fill the blanks. For example, observing end-users’ behavior, interviewing developers and maintenance personnel, etc. (3) integrate the collected insights into the algorithm, allowing the algorithm to grow by itself.
Explore design solutions
Based on the resulting Canvas, designers are required to transform insights into conceptual design solutions and continuously adapt them to the changing properties of stakeholders and context (Barmer et al., Reference Barmer, Dzombak, Gaston, Palat, Redner, Smith and Smith2021). In this process, ML needs to meet the requirements of context and stakeholders, but designers should make fewer restrictions on the solutions or algorithms chosen. Designers need to anticipate the potential problems caused by ML's characteristics, come up with possible design solutions, and record them on the Issue Card.
To ensure the growability of design solutions, designers can: (1) describe long-term changes of different co-creators and envisage how the changes in context will affect data, algorithms, etc. (2) envision how to coordinate stakeholders and context in response to potential changes; (3) predict the most favorable and most undesirable performance of ML-enhanced products, and ensure that the conceptual design solutions still meet the target specifications in these situations.
To ensure the transparency of design solutions, designers can: (1) adopt more explainable algorithms; (2) explore the ways of human-ML collaboration according to dimensions of context; (3) adopt reasonable interaction methods (e.g., sound and text) to explain the product's behavior; (4) apply appropriate methods to present information to avoid cognitive overload.
For the creation of UX values, designers need to balance the potential conflicting properties of co-creators. For example, the social environment may hinder the data collection process. Designers can identify and resolve conflicting factors through design methods such as the co-creation matrix and hierarchical analysis (Saaty, Reference Saaty1987). Designers can fill out scattered conceptual design solutions in the Issue Card, and then use Canvas to trade off and integrate different design solutions.
Express design solutions
Designers can express conceptual design solutions through texts or sketches for communication and evaluation. Specifically, designers should describe the design solution broadly in relation to the target specifications, including the form of interaction and the working mechanism of the product.
Designers can express the dynamic change of co-creators within the ML lifecycle with the help of Canvas: (1) Clarify the working status of the ML-enhanced product at each step of the ML lifecycle to minimize the ambiguity between humans and ML. For example, enumerating all actions the ML-enhanced product can take and their automation level; (2) Describe the changes of co-creators and their relationship, envisioning possible changes of touchpoints and coping strategies. For example, when the automation level of a product changes from high autonomation to no automation, end-users need to be informed in time.
Select tested solutions
Based on the specifications listed in Blueprint, designers may use methods such as majority voting to rank design solutions and select the most promising solution for testing. A team consensus should be established while selecting solutions. In some cases, the team may choose a relatively low-rated but more practical solution rather than the highest-rated but least practical one.
Stage #4: construct
To test and iterate the selected solutions, designers need to construct functional prototypes and build an evaluation matrix. After the evaluation of prototypes, one or more promising solutions can be selected for further iteration (see Fig. 15).

Fig. 15. Main activities and usage of tools in Stage #4.
Build functional prototypes
Convert one or more conceptual solutions into functional prototypes for testing and iteration. To detect unforeseen issues, the functional prototype should represent the majority of a product's functions. Designers could quickly construct prototypes based on real-world data with the help of prototyping tools in Table 4.
Table 4. Different kinds of prototyping tools and their suitable products

Prepare the evaluation matrix
Prepare an evaluation matrix and define evaluation criteria to assess the strengths and weaknesses of solutions. To construct the matrix and comprehensively evaluate the solutions, designers can: (1) combine the information in the “Design Goal” and “Ethical Consideration” modules of Blueprint; (2) provide a matrix with different weights for different stakeholders; (3) examine the design solution's positive/negative impact on stakeholders; (4) expand the target specifications and UX values into evaluation criteria; (5) optimize the matrix dynamically with regard to ML's growability.
Evaluate functional prototypes
Designers should verify whether the current solution conforms to the target specifications, and gain insights for iteration. Both subjective measures (e.g., the expert group, A/B test, usability test), as well as biometric measures (e.g., electroencephalogram, eye-tracking, heart rate variability) that are widely adopted by cognitive science research, could be used (Borgianni and Maccioni, Reference Borgianni and Maccioni2020). Designers should evaluate the prototypes based on the evaluation matrix, and they may discover missed touchpoints or emerging issues in the functional prototype.
Iterate design solutions
Designers could select a better solution and iterate it based on the evaluation's feedback. Specifically, designers should further refine the relationship between co-creators in the ML lifecycle. The activities in this step focus on inspiring creative reflections and improvements. Designers can improve a particular solution, integrate different solutions, or ideate new solutions based on the insights obtained from the evaluation. During the iteration, co-creators are constantly changing and could be influenced by UX values (Wynn and Eckert, Reference Wynn and Eckert2017). Models such as the Define-Stimulate-Analyze-Improve-Control (DSAC) cycle can be used to support complex and ambiguous iterative processes within the ML lifecycle.
Stage #5: cultivate
At stage #5, designers clarify product specifications for the design solution and make a plan to monitor the performance of the ML-enhanced products (see Fig. 16). In this way, designers are able to cultivate a growable and transparent product.

Fig. 16. Main activities and usage of tools in Stage #5.
Clarify product specifications
The final product specifications provide a scheme for the detailed design and guide subsequent development. In the product specifications, designers should detail issues and strategies regarding growability and transparency. Designers should consider the mental model of developers and maintenance personnel in order to describe the product specifications in accordance with their perception. At the same time, a preliminary plan is necessary for the subsequent development. Designers can use Blueprint to document the specifications or use a functional prototype to show the expected UX values provided by the product.
Monitor the product
With the help of Canvas, designers can continuously track the interaction between co-creators, and ensure that the product does not deviate from the initial product specifications as it continues to grow. For example, in the “Algorithm Update” sector, maintenance personnel monitors the performance of the product regularly, and they will be prompted to take contingency measures when the product does not perform as expected.
To ensure the growability of the product, designers could: (1) ensure long-term data collection and utilization; (2) regularly monitor co-creators’ properties that will influence growability and the key operating metrics of products, and actively intervene when the product is not performing as expected.
To ensure the transparency of the product, designers can: (1) collect stakeholders’ preference on transparency regularly; (2) timely adjust design solutions related to transparency, such as rearranging the way to present information and explain algorithms.
Case study: Beelive intelligent beehive
The proposed design framework and process were applied to the design of Beelive, an intelligent beehive for sightseeing, to validate the effectiveness of our work.
The design of Beelive is inspired by the survival crisis of bees and the agricultural transformation in China. The declining population of bees has impacted the food supply and biodiversity. Meanwhile, scientific education-based tourism agriculture has been advocated in many places, which can also be applied to the bee industry to increase the income of bee farmers and promote tourism. However, at present, bees live in opaque and dark hives that cannot be observed up close.
In this background, Beelive's designers began to envision how to enhance the bee industry. We will introduce the design of Beelive based on the UX value framework and the CoMLUX design process.
Stage #1: capture
To begin with, designers preliminarily identified co-creators. Among the stakeholders are bee lovers and beekeepers as end-users, visitors as bystanders, developers, maintenance personnel, and regulators. In the “Transparent Algorithm” module of Blueprint, designers learned about various capabilities of algorithms that can be used for beehives, including bee recognition, pollen recognition, etc. As for the context, beehives are normally located outdoors, so the functions of beehives can be easily affected by noise, temperature, and humidity. According to the description of timeliness and risk, the task performed by Beelive is less risky, but the timeliness level is relatively high due to the bees’ rapid movement. Additionally, bioethics should be considered since the beehive cannot threaten the normal existence of bees.
Based on the collected information, the intelligent beehive is supposed to be: (1) observable: to allow tourists and bee lovers to observe bees up close; (2) scientific: to help bee lovers know more about bees; (3) professional: to ensure the survival of bees and honey production; and (4) safe: to prevent issues like bee stings. Designers wrote down the above information in the “Design Goal” module.
Designers then ideated and screened possible design opportunities through brainstorming according to the design goal. Specifically, design opportunities include the domestic hive for bee lovers and the outdoor sightseeing hive for beekeepers. For the safety of bystanders, the domestic hive was excluded because it could interfere with the neighbors. The idea of the outdoor sightseeing hive was further refined in the following activities.
Then, designers initially defined the target specifications of Beelive. The outdoor sightseeing hive should provide end-users with: (1) affectivity: bee lovers can obtain long-term affective sustenance from observing the activities of bees and honey production. (2) interactivity: end-users can interact with the recognition results and receive real-time feedback. (3) auxiliary: end-users could gain professional knowledge about bees with the assistance of the recognition results. (4) learnability: bee lovers and beekeepers can help improve the performance of the beehive by annotating different bee images, correcting wrong annotations, and so forth. To achieve the above UX values, it is necessary to use a variety of sensors and actuators. For example, capturing bee images via a camera, and uploading them to the cloud via network or recognizing them through a local computing device. As for software, the beehive should present the recognition results in a graphical interface for bee lovers.
Stage #2: co-create
In order to refine the design opportunity, designers conducted in-depth research on co-creators. In terms of ML, since there are no publicly available high-quality datasets, designers collected images by themselves. In terms of computing power, the cloud-based server that required less local hardware was selected. As for the supporting technology, designers chose Raspberry Pi as a miniature host. To gain a deeper understanding of stakeholders, designers conducted a field study on a bee farm in the suburbs. They observed the behavior of tourists and then interviewed beekeepers. Designers found that some tourists (especially children) have an intense interest in bees. Therefore, tourists can also be considered as end-users. Moreover, children prefer an interesting way of conveying information but they know little about ML, so the information presented in the interface should be simple but vivid. In terms of context, the activity of bees and the functioning of hardware would mutually interfere with each other. In this regard, it is preferable to separate the hardware components from the living area of bees. Based on the in-depth research, designers located touchpoints and identified static co-creation relationships (see Fig. 17).

Fig. 17. The static co-creation relationships between co-creators.
Stage #3: conceptualize
According to Question List, designers outlined relationships between co-creators during the ML lifecycle in Canvas. (1) Data collection: developers or end-users collect and annotate bee images for training. (2) Algorithm construction: developers determine whether the capability and performance of algorithms can meet the needs of end-users, and then construct algorithms. (3) Algorithm training: developers train algorithms with collected data and exclude unsuitable algorithms. (4) Algorithm inference: developers run the algorithm to make inferences based on real-time images from the beehive, and the algorithm displays the recognition results to developers. (5) Task execution: ML presents recognition results for end-users in an interesting way and encourages end-users (especially bee lovers and beekeepers) to provide feedback on the accuracy of the recognition. The algorithm and supporting technology should adapt to different climates and environments. (6) Algorithm update: developers retrain the algorithm based on feedback from bee lovers and tourists. Maintenance personnel should continuously monitor the algorithm's performance and decide whether to update it. Considering the above information, designers explored design solutions regarding the shape of the beehive, ways of observation, and ways of presenting results (see Table 5).
Table 5. Scattered design solutions of Beelive

The selected solution is shown in Figure 18a. Because bees are afraid of light and noise, designers excluded the transparent appearance and the loudspeaker in order to preserve the normal existence of bees.

Fig. 18. (a) Sketch of the selected solution. (b) A part of Beelive's functional prototype.
Stage #4: construct
As the next stage of the design process, designers constructed a functional prototype in accordance with the design solution (see Fig. 18b). Designers collected 200 bee images, annotated them, and trained the algorithm on an MLaaS platform called Easy DL. Designers redesigned a traditional beehive with rails, a Raspberry Pi, a camera, and other supporting technology. Additionally, designers developed a mobile application, allowing end-users to see the images inside the beehive.
Next, designers prepare an evaluation matrix to iterate the beehive. The main evaluation metrics and results are shown in Table 6. According to the feedback, designers made the following iterations: (1) Added a transparent tiny hive and updated the algorithm using color bee images (images from the infrared camera are monochrome). In this way, end-users can observe bees directly, and the mobile application is able to recognize photos of bees taken by end-users’ phones. (2) Added a gravity sensor and utilized the regression algorithm to predict the progress of honey production based on the weight of the beehive. By visualizing the honey-making progress, the beehive became more interesting for kids. (3) Applied local computing power to overcome poor network connectivity in the field. (4) Adopted solar cells to improve endurance and applied Zigbee to reduce energy consumption.
Table 6. The evaluation matrix and feedback from stakeholders

Stage #5: cultivate
Designers finally clarified the product specifications in Blueprint (see Fig. 19). In particular, designers clarified the specifications about the growability and opacity of ML. To ensure growability, the beehive should: (1) continuously collect bee images in various environmental conditions to improve accuracy. (2) allow end-users to upload new data by taking photos. In order to promote transparency, the beehive should: (1) provide a user guide for tourists (especially children) to explain the main functions and use methods of the beehive. (2) document the source and usage of data in detail, allowing regulators to check whether the intelligent beehive complies with ethical norms.

Fig. 19. The completed Blueprint.
In order to monitor the changes in the intelligent beehive, designers completed Canvas to ensure growability (see Fig. 20). Designers additionally proposed the following strategies: (1) pay particular attention to breakdowns in recognition. For example, if the beehive fails to recognize bees because of network failures or extreme weather conditions, it could inform end-users in an interesting way and encourage them to notify maintenance personnel. (2) create an interface for feedback, allowing end-users to report errors timely and increase the trust of end-users. (3) require the maintenance personnel to check the beehive on a regular basis and intervene when it not performing as expected.

Fig. 20. The completed Canvas.
Discussion
Guidelines for practically using the framework and process
To facilitate the use of the framework and process in practical design projects, we further clarify the roles of our work in the design of ML-enhanced products and summarize our suggestions for using our work.
The UX value framework works like an index and a dictionary, in which designers could search for design concepts concerning the design of ML-enhanced products. However, since the framework only encompasses generally applicable concepts, it is highly recommended to combine this framework with other field-specific technologies, stakeholders, and contexts. As an example, for the design of medical products, designers are required to possess knowledge of medical ethics, bioimaging technology, etc. The case study (see Section “Case study: Beelive intelligent beehive”) shows an example, in which designers complemented the framework with further research about the bee industry.
The CoMLUX design process provides a step-by-step guidebook, with which designers could go through the entire conceptual design process. It is necessary for designers to flexibly adapt the CoMLUX process according to their projects and extend/delete some activities when needed. For instance, in the case study (see Section “Case study: Beelive intelligent beehive”), designers conducted an additional field study to gain information about beekeepers, tourists, and the bee industry. Apart from that, Blueprint and Canvas echo the characteristics of ML well, but they are not the only available tools. Designers could supplement other tools such as the Business Model Canvas. Meanwhile, designers could refer to the Munich Procedural model (Lindemann, Reference Lindemann2006) to further improve the flexibility of the CoMLUX process, so as to transform between different stages.
Last but not least, the UX value framework and the CoMLUX process mutually support the conceptual design of ML-enhanced products. If designers get stuck at a certain stage of the process, they could refer to the framework and think about whether there are any ignored co-creators or any misunderstood properties for the co-creators.
Inspiration from our work
Through methods including bibliometric analysis and integrative literature review, our work clarifies several ill-defined problems during the conceptual design of ML-enhanced products: (1) We identify ML's characteristics from the perspective of conceptual design. Existing research has investigated ML's characteristics reflected in the process of algorithm development (Hancox-Li, Reference Hancox-Li2020), prototyping (Yang et al., Reference Yang, Steinfeld, Rosé and Zimmerman2020), etc. Based on their findings, we clarify the growability and opacity of ML to help designers cope with the concomitant challenges. (2) We conclude typical UX values of ML-enhanced products. Prior work has summarized different types of UX in general settings (Berni and Borgianni, Reference Berni and Borgianni2021) or based on a certain technology (Jenson, Reference Jenson2017). However, the unique UX values brought by ML have not been systematically clarified. The UX values proposed in this paper could help designers to envision design opportunities in relation to ML's characteristics. (3) We identify the co-creators (i.e., ML, stakeholders, and context) of ML-enhanced UX values and distill the methods to create UX values around ML's growability and transparency, guiding designers to manage factors that might influence UX values and overcome design challenges.
Our research brings the following values to the conceptual design of ML-enhanced products: (1) The UX value framework helps designers to systematically understand concepts related to the conceptual design of ML-enhanced products. According to the findings of cognitive science research, humans could not easily grasp systematic field knowledge without external support (Jonassen, Reference Jonassen2003; Richmond, Reference Richmond1993). To this end, our framework functions as an external support that assists designers in comprehending the dimensions and properties of co-creators, as well as different UX values. (2) The CoMLUX process bridges the gap between theory and practice. It enables designers to take part in the ML lifecycle, coordinate the complex relationships among co-creators, and explore conceptual design solutions with growability and transparency.
Differences between our work and the existing ones
Though prior work proposed frameworks or processes to help designers work with AI or ML, there are several crucial differences between our work and the existing ones.
The UX value framework not only describes the holistic relationship between co-creators as prior work did but also introduces the dimensions and properties of each co-creator. Existing frameworks such as XU's HAI framework (Xu, Reference Xu2019) and two-dimensional HAI framework (Shneiderman, Reference Shneiderman2020) mostly illustrated the macro relationship between AI, human, and ethical aspects, but did not detail the inner factors of each aspect. Grounded on prior work, the UX value framework elaborates on the properties of all the concepts and how these properties influence the UX value of ML-enhanced products. In this way, designers can have an in-depth understanding of the co-creators and their intertwined relationships.
As for the CoMLUX design process, it is customized for the conceptual design of ML-enhanced products instead of following the traditional design process. Existing design processes about ML or AI (e.g., Blue Journey for AI) are mostly similar to the traditional design process. However, prior work pointed out that an ideal design process should be adjusted to the unique characteristics and lifecycle of ML (Sun et al., Reference Sun, Zhang, Li, Zhou and Zhou2022). To this end, CoMLUX process is tailored to the growability and opacity of ML, covering all essential stages of conceptual design and providing comprehensive support for the design of ML-enhanced products.
Apart from that, our framework and process adopt cognitive theories to avoid the cognitive overload of designers. First, by applying schema acquisition to the UX value framework, we represent knowledge as several concepts and link them into hierarchical structures, so as to reduce the difficulty of acquiring new knowledge (Kalyuga, Reference Kalyuga, Plass, Brünken and Moreno2010). Second, the framework ranks the concepts in terms of Know, Understand, and Apply, instead of simply listing all concepts or guidelines as prior work did (Amershi et al., Reference Amershi, Weld, Vorvoreanu, Fourney, Nushi, Collisson, Suh, Iqbal, Bennett, Inkpen, Teevan, Kikin-Gil and Horvitz2019). Taking advantage of the knowledge level (Kolarić et al., Reference Kolarić, Beck and Stolterman2020), the ranked framework helps designers focus on critical concepts and reduce cognitive loading difficulties. Third, following the contiguity principle, we integrate the usage of tools into the design process. Previously, different design tools stood alone to support the conceptual design, which somewhat brought the cognitive burden onto designers. Our process promotes the combination of multiple forms of design research, guiding designers to apply different tools systematically and ultimately create UX values.
In addition to proposing relatively universal frameworks and processes for ML-enhanced products like we did, some related work focused on a specific type of ML-enhanced products. For example, Morrow et al. (Reference Morrow, Lane and Rogers2021) proposed a framework for the design of conversational agents to support the elders. These two kinds of work bare their own advantages and disadvantages. The UX value framework and the CoMLUX design process could be suitable for various ML-enhanced products, and designers could follow the same framework and process in different projects. However, the general work does not contain domain-specific information, and designers have to collect domain information through additional surveys. By contrast, domain-specific frameworks and processes could help designers efficiently gain knowledge about an unfamiliar domain, but it is challenging to extend them to other projects.
Limitations and future work
Our research provides support for the conceptual design of ML-enhanced products, but may still have several limitations. In this section, we discuss the potential limitations and highlight possible future work.
First, the inherent complexity of ML-enhanced products makes it difficult to enumerate all the relevant concepts in the UX value framework. Although we distilled concepts through both quantitative and qualitative methods, a few concepts might be omitted. It is beyond the scope of one paper to list all the concepts related to ML-enhanced products, but we believe the current framework has included the majority of the relevant concepts. Besides, we only introduced the most valuable and representative types of stakeholders and contexts given the length of the paper. The less critical stakeholders (e.g., data annotators) and contexts (e.g., different climates) were excluded. Furthermore, the fast-growing researches in ML-enhanced products make it necessary to continuously pay attention to the latest progress in this field, so as to enrich the current framework.
Second, practical design experience was not fully integrated into the early construction of the UX value framework. The applied bibliometric analysis provides us with an overview of academic research about ML-enhanced products, but the bibliometric method is not suitable for analyzing design cases. Although some design cases and laws/regulations were collected in the second round of search, they did not constitute the main body of material analysis. In the future, this framework could be enriched by introducing more practical insights from design cases.
Third, the proposed framework and process were validated through a case study rather than a quantitative evaluation. It is because we are concerned more about whether our work can support designers throughout the conceptual design process, which is difficult to quantify. Besides, the framework and process have been iteratively evaluated and refined according to design experts’ opinions (see Section “Methodology”). Therefore, referring to prior work (Bryan-Kinns et al., Reference Bryan-Kinns, Wang and Ji2022), we applied the framework and process to an actual design project and elaborated on how they worked through a case study. In future work, we may conduct a more rigorous evaluation to provide more comprehensive feedback on the framework and process.
Besides, the current framework and process primarily focus on the conceptual design phase but do not cover activities related to the phases of production, commerce, etc. During conceptual design, designers establish initial ideas, preliminarily create UX values, and describe how the final ML-enhanced product would be. However, in actual design practice, factors such as production and commerce could also impact the design of products. To this end, future work could incorporate factors such as production and commerce to extend the design framework and process to other phases, or focus on a particular field and propose targeted design processes and frameworks.
Lastly, the effectiveness of our design framework and process still depends on designers’ individual differences in cognitive styles and ML knowledge background. Design is actually a cognitive activity (Cross, Reference Cross, Eastman, McCracken and Newstetter2001), which involves lots of personal and subjective factors (Hubka et al., Reference Hubka, Eder and Scientific2015). Although we ranked the concepts within the framework to different knowledge levels, it is still challenging for designers who are not familiar with ML-enhanced products to comprehend and apply them. Therefore, how to popularize ML-related design knowledge is an issue that should be addressed. Future research could refer to literature about design cognition (Lu, Reference Lu2015) and investigate designers’ cognitive process of learning emerging technologies. This would enable us to tailor the framework and design process to a more comprehensible format. Besides, researchers may also apply electroencephalography (EEG)-based evaluation methods to the design of ML-enhanced products (Frey et al., Reference Frey, Hachet, Lotte, Ayaz and Dehais2018), providing objective, instant, and non-disruptive metrics that facilitate data-driven design outcomes through quantitative analysis.
Conclusion
In this paper, we propose the UX value framework and the CoMLUX design process for the conceptual design of ML-enhanced products. We first applied bibliometric analysis and integrative literature review to extract concepts and integrated them into the UX value framework. The framework identifies ML, stakeholders, and context as co-creators of UX values, and clarifies their dimensions and properties respectively. To construct the design process, we identified the key stages and activities based on MLT, human-centered design methods, and practical design experience. Consisting of stages including Capture, Co-create, Conceptualize, Construct, and Cultivate, the design process assists designers to participate in the ML lifecycle, coming up with growable and transparent design solutions. By organizing concepts hierarchically and taking advantage of the contiguity principle, our work attempted to avoid designers’ cognitive overload during conceptual design. The framework and process were validated to be effective in a case study. Although future work is required to integrate more design practice and cross-disciplinary insights, we believe that our work provides a promising foothold for the conceptual design of ML-enhanced products. We encourage researchers to apply, critique, and refine our work in their projects and research.
Funding
This paper is funded by the National Key R&D Program of China (2018AAA0100703), and the National Natural Science Foundation of China (No. 62006208 and No. 62107035).
Lingyun Sun is a professor and vice dean at the College of Computer Science and Technology at Zhejiang University. He is also the director of the International Design Institute, chair professor and the director of ZJU-SUTD Innovation, Design and Entrepreneurship Alliance, and the director of Zhejiang-Singapore Joint Lab on AI, Innovation and Design. His research interests include big data and AI, digital twins, human-computer interaction, and information visualization.
Zhuoshu Li is a master's candidate at the International Design Institute of Zhejiang University. Stemming from her background in user experience design and interests in AI, she is dedicated to addressing the interaction design challenges brought by AI as well as exploring novel tools and methods for designing human-AI interaction. Her prior work includes prototyping tools for designers and researchers about the transparency issues of AI-empowered products.
Zhibin Zhou is a research assistant professor at the School of Design, The Hong Kong Polytechnic University. He focuses on capturing the interaction between humans and AI and gaining an increased understanding of AI as a design material. With a background in user experience design and AI, his prior research aims to bring together knowledge in the fields of design and AI as well as help the next generation of designers to foster AI literacy to understand its nature and thus creatively ideate and practically prototype ML-enhanced products.
Shanghua Lou is a bachelor's student at Southeast University. Based on his prior experience in UX design and computer science, he is interested in exploring innovative methods of human-machine interaction in both virtual and real ways. His previous work includes a set of hand-ware equipment for easy-way VR interaction and research about healing tools for Alzheimer's disease.
Wenan Li is a UX researcher with a background in industrial design. She graduated from Zhejiang Sci-tech University with a bachelor's degree in Industrial Design and is currently a master's candidate at Zhejiang University. She is interested in exploring how to use design as a tool to improve the user experience of various people.
Yuyang Zhang is a mater's candidate at the International Design Institute of Zhejiang University. She graduated from Zhejiang University with a bachelor's degree in Industrial Design and is currently an HCI researcher. She is interested in AI and Design, exploring how to address the issues of designing AI-empowered products. Her prior work includes the design challenges of AI-empowered products as well as design tools for AI-empowered products.