Neonatal birth weight (BW) remains critical for the study of adverse pregnancy outcomes and the prediction of neonatal mortality and morbidity(Reference Dobbins, Sullivan and Roberts1,Reference Yao, Miao and Li2) . Low birth weight (LBW) and small for gestational age (SGA) are the two primary adverse birth outcomes determined by BW. LBW infant is the one born weighing <2500 g according to the WHO(Reference Goldenberg and Culhane3), and SGA is commonly defined as BW below the 10th percentile for gestational age and sex. Both LBW and SGA are the critical causes of perinatal and neonatal mortality and are related to many short-term morbidities (including respiratory diseases and necrotising enterocolitis) and long-term health issues (including cognitive deficiencies, mental retardation, cerebral palsy, diabetes and CVD)(Reference Goldenberg and Culhane3,Reference Shorer, Wainstock and Sheiner4) . Therefore, poor fetal development in terms of BW could influence life-long health and take great economic costs and burdens for the society and the families involved in.
Maternal nutrition during pregnancy significantly contributes to fetal development. Dietary intakes of essential macro- and micronutrients are closely linked with pregnancy outcomes including BW, LBW and SGA, but the relationships are inconclusive(Reference Mousa, Naqash and Lim5). For the macronutrients, Godfrey et al. observed a negative association between carbohydrate (CHO) intake in early pregnancy and BW(Reference Godfrey, Robinson and Barker6), but a positive association between the percentage of total energy from CHO and BW was found in Cohen et al.’s study(Reference Cohen, Curet and Levine7). Discrepant findings in the relationship between maternal protein intake and BW were also presented in previous studies(Reference Godfrey, Robinson and Barker6,Reference Cucó, Arija and Iranzo8–Reference Switkowski, Jacques and Must11) . Watson et al. found a quadratic relationship between CHO, protein and fat intakes and BW(Reference Watson and McDonald12). In addition, recent evidence showed that the effect of protein on BW is non-linear and that a balanced diet fulfilling the minimum requirement for all macronutrients was ideal for avoiding fetal growth restriction(Reference Morisaki, Nagata and Yasuo13). These indicated the joint effect of macronutrients on fetal growth and could be an explanation for the inconsistent results in the previous studies. For the micronutrient, increased BW was associated with increasing pantothenic, biotin, Mg, vitamin D and vitamin B12 intakes(Reference Watson and McDonald12). Total Fe intake from food and supplements, prenatal Fe use and the dietary haem Fe intake were also in relation to improved BW and related outcomes such as LBW and SGA(Reference Yang, Cheng and Pei14–Reference Alwan, Greenwood and Simpson16).
As stated above, evidence on the association between maternal nutrition and fetal growth usually focused on the effect of a single nutrient or a specific food component. Maternal dietary patterns (DP) could explicate the relationship more comprehensively due to the synergistic and antagonistic effects between the foods consumed are considered(Reference Hoffmann, Schulze and Schienkiewitz17). The principal component analyses (PCA) are a common method to obtain the DP. Evidence showed that DP derived from PCA are related to the susceptibility for developing LBW and SGA(Reference Coelho Nde, Cunha and Esteves18–Reference Martínez-Galiano, Olmedo-Requena and Barrios-Rodríguez20). However, the PCA could not capture the patterns that were most strongly related to the outcome(Reference Sartorelli, Zuccolotto and Crivellenti21). Instead, the reduced-rank regression (RRR)(Reference Hoffmann, Schulze and Schienkiewitz17) is suitable for extracting the DP that were associated with the outcome. RRR is a statistical method used to derive disease-related DP, which determine a combination of foods (patterns) that better explain the variation of intermediate response variables (usually nutrients or biochemical markers) that are known to be predictive for disease(Reference Sartorelli, Zuccolotto and Crivellenti21). It allows us to evaluate the combined effect of intermediate variables on the outcomes. To our knowledge, investigations on the association between maternal DP during pregnancy derived from the RRR and BW were rare. Accordingly, this study aims to identify maternal DP during pregnancy, derived by the RRR method, associated with dietary macronutrients and specific micronutrient intake and to examine their relationship with BW and related birth outcomes.
Method
Data source and participants
The study employed data from a large-scale cross-sectional survey conducted between August and November 2013 in Shaanxi Province of Northwest China. This population-based survey was aimed to investigate the risk factors of adverse pregnancy and birth outcomes. Detailed design and methods have been described elsewhere(Reference Yang, Dang and Cheng22). A brief sampling method was showed here. Women aged from 16 to 49 years, pregnant during 2010–2013 and had pregnant outcomes (including live birth, stillbirth and abortion) before the survey were enrolled using a stratified multistage random sampling method. According to the proportion of rural to urban residents, population size and fertility rate in Shaanxi, twenty counties and ten districts, were randomly sampled. In each sampled county, six villages each from six townships were randomly selected; in each sampled district, six communities each from three streets were randomly selected(Reference Yang, Dang and Cheng22). A total of 30 027 women were selected and interviewed by trained investigators to complete a structured questionnaire for collecting general information and characteristics during pregnancy. A sample of 7750 women who were pregnant during 2012–2013, had live births and delivered in less than 12 months were further interviewed to report their diets during pregnancy.
Ethics
This study was conducted according to the guidelines laid down in the Declaration of Helsinki, and all procedures involving human subjects were approved by the Ethic Review Committee and Academic Committee of Xi’an Jiaotong University Health Science Center (approval no. 2012008). Written informed consent was obtained from all participants.
Dietary assessment
Maternal DP and the overall nutrient intakes from foods did not change appreciably from early to late pregnancy(Reference Rifas-Shiman, Rich-Edwards and Willett23–Reference Crozier, Robinson and Godfrey25). And for large-scale epidemiological surveys with multiple dietary exposures and outcomes, like our present study, diet assessment during the pregnancy at one time was reasonable, convenient and economical for both participants and investigators(Reference Rifas-Shiman, Rich-Edwards and Willett23). Evidence showed that the dietary information during pregnancy could be recalled with relatively good accuracy and reproducibility by mothers using FFQ even after years(Reference Bosco, Tseng and Spector26–Reference Willett, Sampson and Browne29). In this study, to ensure the accuracy of diet recall, the women who delivered in <12 months (median 3; 10th–90th percentiles 0–7) were asked to report their diet during pregnancy and the dietary information was collected by a 107-item semi-quantitative FFQ(Reference Yang, Dang and Cheng22). The FFQ was established according to the previously validated FFQ used for pregnant women in Shaanxi(Reference Cheng, Yan and Dibley30,Reference Cheng, Dibley and Zhang31) . Pearson correlation coefficients between the validated FFQ and the average of 24-h recalls for all investigated nutrients, including the macronutrients and most of the micronutrients according to the Chinese Food Composition Tables, were ranged from 0·53 to 0·70. Pearson correlation coefficient was 0·61 for protein, 0·68 for CHO, 0·55 for fat and 0·65 for Fe. Among all the investigated food, five food items (animal oils, vegetable oils, salt, sugar and sauce) were recorded using open-ended frequency scales that marked as kg of use per month and the number of people regularly consuming them. The consumption frequencies of other 102 food items were reported by women through choosing one of the eight predefined categories which ranging from never to ≥2 times/d, along with identifying the portion sizes (large, medium or small) consumed per time based on food portion images(Reference Yang, Dang and Cheng22). Chinese Food Composition Tables(32) were referenced to transform daily food consumptions into total energy, macronutrient and micronutrient intakes, which were the sum of energy and nutrients in each food items. Total energy intake <2092 kJ or >20 920 kJ was regarded as implausible energy intake(Reference Cheng, Dibley and Zhang31).
In this study, DP were obtained using RRR, which uses response variables as an intermediate between the dietary intake (predictor variables) and the outcome(Reference Hoffmann, Schulze and Schienkiewitz17). Compared with the PCA method used in many previous studies, the RRR reduces the dimension of predictor variables to the dimension of response variables and it could give better interpretations of the extracted DP by the explained variation in intermediate response variables that should be related to disease. With reference to the previous research, protein density (g/4184 kJ), CHO density (g/4184 kJ), the ratio of PUFA and MUFA density:SFA density ((PUFA+MUFA):SFA) and haem Fe density (mg/4184 kJ) were chosen as the response variables according to their associations with BW. Protein and CHO intake during pregnancy were linked with BW(Reference Watson and McDonald12). Intake of MUFA and PUFA may be related to the increased BW and reduced risk of LBW(Reference Mousa, Naqash and Lim5,Reference Watson and McDonald12) . Increased maternal haem Fe intake may protect against LBW and SGA(Reference Yang, Cheng and Pei14). The 107 food items were consolidated into twenty-four food groups (wheat, rice, potatoes, legumes, soyabean products, green vegetables, other vegetables, fungi, fruits, nuts, red meat, poultry, processed meat, dairy products, eggs, fish, snacks, fast food, soft drinks, alcohol drinks, sweets, oils, solid fats and condiments) based on China Food Composition Tables and the logic of intake(32). The twenty-four food groups (g/d) were considered as the predictor variables in the RRR model.
Outcome assessment
Neonatal information including birth date, sex, BW and gestational age was obtained by reviewing birth certificates. BW was measured with a baby scale with precision to the nearest 10 g. Gestational age at delivery was calculated according to the last menstrual period and was confirmed by ultrasound scans. The primary outcome of this study was BW, while the BW z-score, LBW and SGA were the secondary outcomes. The sex- and gestational age-adjusted BW z-score were calculated according to international standards developed by the International Fetal and Newborn Growth Consortium for the 21st Century(Reference Villar, Cheikh Ismail and Victora33). LBW infant was defined as one born weighing <2500 g according to the WHO(Reference Goldenberg and Culhane3). SGA was defined as BW below the 10th percentile for gestational age and sex according to the international standards(Reference Villar, Cheikh Ismail and Victora33).
Covariate assessment
We used face-to-face interviews to collect data on maternal characteristics using a structured questionnaire at the same time as diet information collection. We asked the participants to report maternal information during their pregnancy. Covariates mainly include two parts: (1) socio-demographic characteristics, including maternal age at delivery (<25, 25–34 and ≥35 years), education level (junior high school or below, senior high school and college or above), residence (urban and rural), occupation (farmers and others), per capita annual household income (low <8000, medium 8000–14 000 and high ≥14 000 Yuan, where 1 Yuan = 0·145 $US on 3 July 2019) and county (district) (twenty counties and ten districts); (2) health-related characteristics, including parity (primipara and multipara), smoking (yes and no), passive smoking (yes and no), alcohol consumption (yes and no), the times of antenatal visits (<5 and ≥5), pregnancy consultation (yes and no) and micronutrient supplementation (yes and no) including folic acid, Fe and multiple-micronutrient. Passive smoking was defined as being exposed to other people’s cigarettes for more than 15 min/d. Pregnancy consultation was referred to the participation of health counselling that was related to maternal health care and fetal development before or during pregnancy. Women who consumed certain supplement more than 1 week during pregnancy were considered as users of such supplement, otherwise, were regarded as non-users.
Statistical analysis
Data set was established using Epidata 3.1 (The Epidata Association) with double entry. The RRR procedure was used to identify maternal DP during pregnancy, using the intake of twenty-four food groups (g/d) as predictor variables and protein density (g/4184 kJ), CHO density (g/4184 kJ), (PUFA+MUFA):SFA and haem Fe density (mg/4184 kJ) as response variables. Factor loadings derived from RRR were the measures of the association between food groups and the DP. Food groups with the absolute value of factor loading ≥0·15 were main contributors to the pattern. The association between each DP and the response variables was evaluated via Spearman’s correlation coefficients. Factor scores were the evaluation of the adherence for each DP. Women were classified into three groups according to the tertiles of factor scores for each DP. T1, T2 and T3 were the low, medium and high adherence group, respectively. BW was divided into three groups by tertiles for comparing maternal characteristics. We used χ 2 tests for categorical variables and ANOVA tests for continuous variables to compare the maternal characteristics according to BW and the adherence to the DP.
Because of the hierarchical structure of the data derived from the stratified multistage random sampling design, generalised estimating equation models(Reference Hubbard, Ahern and Fleischer34) with random effect at the county (district) level were applied to evaluate the associations between DP with the outcomes. Three models were established to estimate the change in BW and BW z-score (normal distribution and identity link function), OR for LBW and SGA (binomial distribution and logit link function), as well as their accompanying 95 % CI. Model 1 was the unadjusted model. Model 2 was adjusted for total energy intake and socio-demographic characteristics (including maternal age, education, residence and per capita annual household income). Model 3 was adjusted for all variables in model 2 and health-related factors in pregnancy (including parity, smoking, passive smoking, alcohol consumption, pregnancy consultation, number of antenatal visits, folic acid/Fe/multiple-micronutrient supplementation). Adjusted models for BW and LBW were additionally adjusted for sex and gestational age. To test for a linear trend, we used the tertiles of adherence to DP as a continuous variable. After examining the overall association between DP and BW, we further conducted the subgroup analysis to evaluate the relationship within different BW groups by tertiles.
All analyses were performed using SAS version 9.4 (SAS Institute). All statistical tests were two-tailed, and statistical significance was set as P < 0·05.
Results
Totally, 7194 eligible women were included in the final analysis, after excluding women who had multiple gestation (n 87), had no clear information on BW or gestational age (n 57), had gestational age of <33 or ≥43 weeks (n 64), had implausible BW (<1000 g or >5000 g) (n 8) and had an implausible total energy intake (>20 920 or <2092 kJ/d) (n 340) from women who completed the FFQ. Among the participants, 56·7 % of women were aged between 25 and 34 years and 63·2 % of women had junior high school or below educational level. Most of the participants were rural residents (76·6 %) and famers (73·5 %). 60·4 % of women were primiparous. The mean value of BW of the newborns was 3253·9 (sd 448·3) g, and the prevalence of LBW was 3·6 %. The gestational age ranged from 33+0 to 42+6 weeks, and 53·8 % newborn were boys. The mean value of BW z-score was –0·07 (sd 1·15), and the overall prevalence of SGA was 13·2 % (data were not shown in table).
Maternal characteristics of participants by birth weight
Maternal characteristics of participants by BW are displayed in Table 1. Women with lower BW were more likely to be aged below 25 or above 35 years, have junior high school or below educational level, be rural residents and farmers, have no pregnancy consultation and have less than five antenatal visits.
Table 1. Maternal characteristics by birth weight (BW) tertiles among pregnant women in Shaanxi Province, Northwest China, 2010–2013
(Numbers and percentages; mean values and standard deviations)

T1–T3, tertiles 1–3; RMB, Ren Min Bi; FA, folic acid; MMN, multiple-micronutrient.
* P values for the differences among groups were derived from χ 2 tests for categorical variables and ANOVA tests for continuous variables.
† Pregnancy consultation was referred to the participation of health counselling that related to maternal health care and fetal development before or during pregnancy.
Dietary patterns
Table 2 shows the factor loadings of the food groups in the DP during the pregnancy derived by RRR, using protein density (g/4184 kJ), CHO density (g/4184 kJ), (PUFA+MUFA):SFA and haem Fe density (mg/4184 kJ) as response variables. Four DP were identified: DP1: high legumes, soyabean products, vegetables, meat, dairy products, eggs and fish, with relative low wheat and oils; DP2: high wheat, rice, potatoes, vegetables and fruits, with low nuts, red meat and oils; DP3: high wheat, potatoes, legumes, soyabean products, vegetables, nuts and fish, with low solid fat; DP4: high fruits, nuts, red meat, dairy products and soft drinks, with low legumes, eggs and solid fat. The four DP explained 25·5 % of the total variation in the food group intake and 72·4 % of the total variation in the response variables. DP1 and DP2 were the primary DP explaining 63·1 % (DP1 40·1 %; DP2 23·0 %) of variation of the response variables. DP1 score was correlated positively with protein and haem Fe density and negatively with CHO density and (PUFA+MUFA):SFA. The percentage of variation in the response variables explained by DP1 was 54·2 % for protein, 13·9 % for CHO, 48·1 % for (PUFA+MUFA):SFA and 45·2 % for haem Fe. DP2 score was correlated positively with protein and CHO and negatively with (PUFA+MUFA):SFA and haem Fe. It mainly explained the variation of CHO (65·6 %) and (PUFA+MUFA):SFA (22·1 %).
Table 2. Factor loadings of food groups in the four dietary patterns (DP) derived by reduced-rank regression (RRR) among pregnant women in Shaanxi Province, Northwest China, 2010–2013†
(Factor loadings; Spearman’s correlation coefficients; percentages of variation)
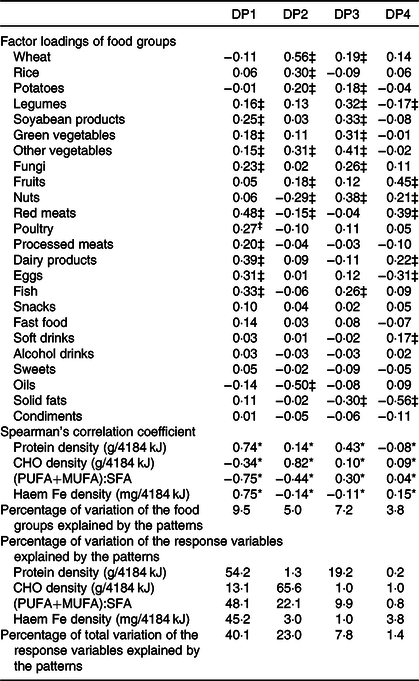
CHO, carbohydrate.
* P < 0·05 for the correlations between factor score of DP and the response variables.
† Patterns were derived by RRR using dietary protein, CHO and haem Fe density, and PUFA and MUFA:SFA as the response variables and twenty-four food groups as the predictor variables. The DP obtained from RRR explained 25·5 % of the total variation in the food group intake and 72·4 % of the total variation in the response variables.
‡ Food groups with factor loadings’ absolute value ≥0·15.
Maternal characteristics of participants according to adherence to the two main dietary patterns
Women with higher adherence to DP1 were more likely to be aged between 25 and 34 years, be better educated, be employed, live in the urban area, have high income level, be primiparous, have better antenatal care, have no passive smoking and have more micronutrient supplementation. Opposite characteristics were observed among women had higher adherence to DP2, including lived in rural areas, had lower income levels, were multiparous, had no pregnancy consultation and had less than five antenatal visits (Table 3).
Table 3. Maternal characteristics of participants according to adherence to the two main dietary patterns (DP) among pregnant women in Shaanxi Province, Northwest China, 2010–2013
(Numbers and percentages; mean values and standard deviations)

RMB, Ren Min Bi; FA, folic acid; MMN, multiple-micronutrient.
* P values for the differences among groups were derived from χ 2 tests for categorical variables and ANOVA tests for continuous variables.
Association between dietary patterns and birth weight and related outcomes
Compared with women with the low adherence to DP1, after adjusting for the covariates, those with the medium and high adherence had significant increases in BW (changes: medium 28·6 (95 % CI 7·1, 50·1); high 25·2 (95 % CI 2·7, 47·6); P trend = 0·023) and BW z-score (changes: medium 0·07 (95 % CI 0·02, 0·12); high 0·06 (95 % CI 0·01, 0·11); P trend = 0·028) and had significant reduction in risk of SGA (OR : medium 0·86 (95 % CI 0·77, 0·97); high 0·81 (95 % CI 0·70, 0·94); P trend = 0·004). In addition, the high adherence to DP1 had 35 % reduced risk of LBW (OR 0·65 (95 % CI 0·48, 0·87); P trend = 0·005). There is no association between DP2/DP3/DP4 and the outcomes (Table 4). Subgroup analysis showed that the association between adherence to DP1 and BW was observed in women with babies <3100 g and between 3100 and 3400 g, and as the rising of the adherence, the increases of BW were greater in women with babies <3100 g. There is no association between DP1 and BW among women with newborn ≥3400 g (online Supplementary Table S1).
Table 4. Associations between dietary patterns (DP) and birth weight and related outcomes among pregnant women in Shaanxi Province, Northwest China, 2010–2013†
(Values are changes in grams, scores and odds ratios and 95 % confidence intervals)

Ref., reference; LBW, low birth weight; SGA, small for gestational age.
* P < 0·05.
† Generalised estimating equation models with random effect at the county (district) level were used to estimate the change (95 % CI) for birth weight/birth weight z-score and OR and 95 % CI for LBW/SGA according to the adherence to the DP.
‡ Model 1 was an unadjusted model.
§ Model 2 was adjusted for total energy intake and socio-demographic characteristics (including maternal age, education, residence and per capita annual household income). Model 2 for birth weight and LBW was also adjusted for sex and gestational age.
|| Model 3 was adjusted for all variables in model 2 and health-related factors in pregnancy (including parity, smoking, passive smoking, alcohol consumption, pregnancy consultation, number of antenatal visits, folic acid/Fe/multiple-micronutrient supplementation).
¶ P trend was estimated using the tertiles of adherence to DP as a continuous independent variable in the models.
Discussion
In the present population-based cross-sectional study conducted with 7194 pregnant women in Northwest China, four DP during pregnancy were identified using the RRR method. DP1 and DP2 were the two main DP that explained most of the variation in the response variables. Women with medium and/or high adherence to DP1 presented significantly higher BW and BW z-score and lower risk of LBW and SGA. The associations between DP1 and BW were observed in women with babies <3100 g and between 3100 and 3400 g, and it seemed to be stronger in the group of the newborn <3100 g. DP2, DP3 and DP4 were not associated with the outcomes.
DP in this study were derived from the RRR method with the response variables of dietary protein, CHO, haem Fe density and the ratio of (PUFA+MUFA):SFA based on their association with BW and related birth outcomes. The DP1 identified in this study presented a pattern high in dietary protein and haem Fe and low in CHO and the ratio of (PUFA+MUFA):SFA. This pattern comprehensively reflected the combined importance of dietary macronutrients and haem Fe intake on BW. Our finding of the positive association between the adherence to DP1 and BW was consistent with the relationship between dietary protein as well as haem Fe intake and BW shown in previous studies. Higher dietary protein intake or higher percentage of energy from protein during specific pregnancy phase was positively associated with BW(Reference Cucó, Arija and Iranzo8,Reference Haste, Brooke and Anderson9,Reference Moore, Davies and Willson35) . Higher haem Fe significantly contributed to improved maternal Fe status(Reference Yang, Cheng and Pei14), which was linked with reduced adverse birth outcomes such as LBW, preterm birth and SGA(Reference Alwan, Cade and McArdle36,Reference Rahman, Abe and Rahman37) . DP1 was negatively correlated with CHO density and the ratio of (PUFA+MUFA):SFA, which indicate that lower dietary CHO and the ratio of (PUFA+MUFA):SFA were associated with increased BW. The relationship between CHO and BW was in accordance with the finding of Godfrey et al.’s study(Reference Godfrey, Robinson and Barker6). DP that was low in SFA and high in MUFA and n-3 PUFA was associated with decreased risk of delivering a fetal growth-restricted infant for weight(Reference Chatzi, Mendez and Garcia38).Our finding about the ratio of (PUFA+MUFA):SFA and BW was partially inconsistent with the previous study. This difference may be attributed to the joint effect of macro- and micronutrients on BW in the present study, rather than the impact from a single nutrient.
DP1 obtained in our study was characterised by high intakes of legumes, soyabean products, vegetables, meat, dairy products, eggs and fish, with relatively low intakes of wheat and oils. This was partially different from the recognised healthy pattern, for example, the pattern recommended by the USDA, with higher intakes of fruits, vegetables, grains (with at least half of the intake from whole grains), moderate intakes of protein (such as low-fat meat and poultry, beans and legumes, nuts and seeds, or fish) and low-fat dairy products, and a limited intake of oils (with a preference for MUFA and PUFA), refined grains, and added sugars(Reference Starling, Sauder and Kaar39). However, the dominant food groups of DP1 have benefits on BW, as were previously reported. Higher meat and fish intakes were associated with increased BW(Reference Watson and McDonald12). Higher dairy intake during pregnancy was also related to the increased BW and reduced risk of LBW(Reference Moore, Davies and Willson35,Reference Clark40) . Legumes and vegetables are an important source of polyphenols, which acts as antioxidants to protect against oxidative stress and inflammation(Reference Hussain, Tan and Yin41). Elevated level of oxidative stress as well as inflammatory response could lead to pregnancy complications and result in adverse outcomes, including intra-uterine growth restriction, preterm birth and LBW(Reference Žák and Souček42). Therefore, it is plausible that, for women with higher adherence to DP1, higher intake of plant foods rich in polyphenols may reduce the risk of adverse birth outcomes through its antioxidative and anti-inflammatory effect. We did not observe significant association between other DP and the outcomes. The factors (DP in this study) obtained by RRR usually are sorted by decreasing eigenvalues, and thus the first factor (DP1) of RRR explains more variation in response than any other linear function of predictors(Reference Hoffmann, Schulze and Schienkiewitz17). In this study, the DP1 explained the highest percentage (40·1 %) of the variation of response variables, which implied that the DP1 is more relevant to the intermediate variables that were related to the outcomes, and this may explain why the significant results were found restricted to DP1.
To the best of our knowledge, a few previous studies has investigated the association between DP during pregnancy derived by RRR and BW(Reference Starling, Sauder and Kaar39,Reference Papadopoulou, Kogevinas and Botsivali43) . Because of the different intermediate variables, the DP extracted by RRR in existing studies were usually distinct from each other, so were the associations between DP and BW. Unlike our study, Starling et al. used the gestational weight gain and the fasting glucose as the response variables in the RRR method and reported that a pattern characterised by starchy vegetables, eggs, non-whole grains, and a low intake of dairy products, dark-green vegetables, whole grains and soya was associated with greater newborn BW and adiposity(Reference Starling, Sauder and Kaar39). Our results were also different from the findings of several previous studies that used the PCA method to extract DP. Knudsen et al.’s research showed that a DP characterised by high intake of fruits, vegetables, fish and poultry was associated with decreased risk of SGA(Reference Knudsen, Orozova-Bekkevold and Mikkelsen44), while Thompson et al. exhibited that a DP composed of meat, potatoes, fruits (particularly citrus fruits), green vegetables, carrots, dairy products and water was protective for SGA(Reference Thompson, Wall and Becroft45). Compared with studies that employed the PCA approach, the RRR method applied in our study, which used the macro- and micronutrients that are closely related to the outcomes as intermediate variables, could utilise and combine the finding of previous studies more efficiently and consider the relationship between diet and outcomes more comprehensively(Reference Hoffmann, Schulze and Schienkiewitz17).
In our population, women with disadvantageous socio-demographic conditions and less antenatal care were more likely to have lower newborn BW. The associations between DP1 and BW were only observed in women with newborn <3100 g and between 3100 and 3400 g, and it seemed to be stronger in the group of the newborn <3100 g. This indicated that the DP1 mainly contributed to the betterment of BW among socio-demographically disadvantaged pregnant women. Women with disadvantageous socio-demographic conditions among our participants were more likely to have higher adherence to DP2, which was low in animal-source foods, rather than DP1. Considering the evidence that women with high adherence to a vegetarian DP had lower nutrient intakes compared with those who had high adherence to a balanced pattern(Reference Yang, Dang and Cheng22), the inadequate nutrient intakes could be a major cause of the lower newborn BW among the socio-demographically disadvantaged group. DP1 in our study was high in both plant- and animal-source foods, and thus, an appropriate increase of the adherence to DP1 among Chinese pregnant women with disadvantageous socio-demographic conditions could efficiently improve the fetal development in terms of BW and reduce the risk of LBW and SGA through increasing their overall nutrient intakes.
The primary strength of the present study is that, for the first time, the associations between maternal DP during pregnancy derived by the RRR and BW in the Chinese population were examined, using dietary protein, CHO, haem Fe density and the ratio of (PUFA+MUFA):SFA as the response variables. The RRR method could extract the DP that were strongly related to the outcome by combining prior information and dietary information from the study. In addition, this is a representative and relatively large-scale study that investigated the effect of maternal DP during pregnancy on the adverse birth outcomes, from the background of the dietary culture in Northwest China. The cross-sectional study used a stratified multistage random sampling method to guarantee its representativeness in Shaanxi Province. The findings could be generalised to other regions in Northwest China according to the similarities in terms of economy, culture, lifestyle and diet habits in these regions and could partly reflect the dietary habits among pregnant women in China. Nonetheless, several limitations of the present study should be addressed. Firstly, the dietary and other maternal information during pregnancy was retrospectively self-reported by the mothers after delivery. Although the dietary information during pregnancy could be recalled rather well by mothers even after years(Reference Bosco, Tseng and Spector26–Reference Willett, Sampson and Browne29), the possible influence of current diet on the accuracy of recall of past diet was inevitable, especially when the recall interval was long(Reference Friedenreich, Slimani and Riboli46), and also we could not rule out the possible exposure misclassification due to recall bias. To minimise the recall bias, our study was limited to the women who were pregnant during 2012–2013 and delivered in <12 months to report their diet during pregnancy. Most of the mothers recalled their dietary intake during pregnancy at <7 months after delivery and half of them recalled at <3 months after delivery. In addition, we made efforts to help them recall accurately through face-to-face interviews by well-trained investigators and using detailed supporting materials such as food portion images and calendars to collect information. We also conducted a pilot study to test the survey materials and interviewers based on the detailed guides before the formal survey. All measures we adopted could ensure data quality to a certain extent. In addition, we believe that the possible exposure misclassification could be non-differential as the association between DP and BW was not known during the investigation, and it may cause the attenuation of the association. Secondly, we used FFQ to assess the dietary intakes, which may be not precise in estimating absolute intake of nutrients of individuals. However, at the population level, the FFQ method is good for measuring most of the common nutrients in pregnant women and can be used to rank dietary intakes(Reference Hjartåker, Andersen and Lund47,Reference Ortiz-Andrellucchi, Doreste-Alonso and Henríquez-Sánchez48) . The FFQ used in this study was established based on the previously validated FFQ used for pregnant women in the same region, which could guarantee its accuracy and reliability. Therefore, our FFQ could reflect the overall situation of dietary intakes and DP among our population. Moreover, compared with other dietary assessment methods such as food records and 24-h recalls, FFQ can reflect the dietary intakes rather well over a longer period and is more practical for the dietary assessment in large-scale population-based epidemiological investigations(Reference Cheng, Yan and Dibley30). Thirdly, although we did the analysis by controlling for some potential confounders, there were still some unobserved or unknown confounders that may influence the association. For example, maternal pre-pregnancy BMI and gestational weight gain are associated with risks of adverse maternal and infant adverse outcomes(Reference Voerman, Santos and Inskip49). Reduced risks of LBW and SGA were related to higher maternal pre-pregnancy BMI and excessive maternal gestational weight gain(Reference Li, Liu and Guo50). Also, the prenatal physical activity level of mothers was reported to be associated with reduced BW(Reference McCullough, Mendez and Miller51). Unfortunately, the information about the factors mentioned above was not available in the present study, and thus we could not control for their impact on the relationship between maternal DP and birth outcomes. Therefore, our results were interpreted cautiously and require further assessment by prospective study. Finally, the cross-sectional design limited the interpretation on a real causal association. Further well-designed studies are suggested to explore the effect of DP during pregnancy on birth outcomes.
Conclusion
Findings in this study suggested the combined importance of macronutrients and haem Fe intake of DP on BW. During pregnancy, higher adherence to the DP that presented high intakes of legumes, soyabean products, vegetables and animal-source foods was associated with increased BW and BW z-score as well as reduced risk of LBW and SGA in the Chinese population, particularly among socio-demographically disadvantaged pregnant women. Targeted health education and intervention should be implemented to appropriately increase the adherence to this DP during pregnancy for the improvement of BW and its related birth outcomes.
Acknowledgements
The authors are grateful to all women who took part in this research, all health staff who coordinated fieldwork and all investigators who contributed to data collection.
This research was funded by the National Natural Science Foundation of China (grant nos. 81230016 and 81202218), the Shaanxi Health and Family Planning Commission (grant no. Sxwsjswzfcght2016-013) and the National Key Research and Development Program of China (grant nos. 2017YF0907200 and 2017YF0907201).
D. L., Y. C., S. D. and H. Y. conceived and designed the study; D. L., B. M., P. Q., S. L., R. Z., Q. Q., C. W., X. G. and Y. L. collected and cleared the data; D. L., S. D., B. M., L. Z. and P. Q. analysed and interpreted the data; D. L., Y. C., B. M., L. Z., S. L., R. Z., S. D. and H. Y. drafted and revised the manuscript. All authors reviewed and approved the final version of the manuscript.
The authors declare that there are no conflicts of interest.
Supplementary material
For supplementary material referred to in this article, please visit https://doi.org/10.1017/S0007114520000392