Introduction
The COVID-19 pandemic has had governments across the world scrambling to devise effective strategies against the spread of the virus and reduce death rates. Almost all governments have proclaimed increased spending to tackle the problem, which is arguably the primary policy instrument for governments. Global financial institutions, such as the International Monetary Fund (IMF), have pledged over 1 trillion United States (US)$ for financing COVID-related spending.Footnote 1 Does public spending on health matters for dealing with crises of this nature? Evidence on the effects of government spending on health outcomes has generally been inconclusive. While some studies show that increased spending on health does not necessarily translate into better health outcomes (Filmer and Pritchett Reference Filmer and Pritchett1999; Ray and Linden Reference Ray and Linden2020), others show that higher spending does contribute to better health (Chirowa et al. Reference Chirowa, Atwood and Van der Putten2013; Owusu et al. Reference Owusu, Sarkodie and Pedersen2021). Fighting viruses, however, might require more than just finances. How increased budgets for health might prevent the next pandemic, thus, is a critical question that might be addressed by assessing how spending in the past relates to the outcomes of the present pandemic.
We contribute to the literature by investigating the effects of government healthcare expenditure on the recent COVID-19 pandemic. The magnitude of this crisis led governments to respond by committing US$10 trillion in the first two months alone, which was three times that of the response to the 2008–2009 financial crisis (Cassim et al. Reference Cassim, Handjiski, Schubert and Zouaoui2020). According to Cassim et al. (Reference Cassim, Handjiski, Schubert and Zouaoui2020), countries in Western Europe alone devoted approximately US$ 4 trillion to the pandemic, while some countries committed as much as 40% of their GDP to deal with the crisis. Studies also suggest that disparities in healthcare access affect pandemic outcomes (Garoon and Duggan Reference Garoon and Duggan2008; Tricco et al. Reference Tricco, Lillie, Soobiah, Perrier and Straus2012; Quinn and Kumar Reference Quinn and Kumar2014; Mamelund et al. Reference Mamelund, Shelley-Egan and Rogeberg2019). The distribution of vaccinations has also been unequal. The rich countries hogged the majority of vaccines, leaving little for the poorer countries. Access to vaccines is related directly to the death count. In a study of Europe and Israel, Jabloriska et al. (Reference Jabloriska, Aballea and Toumi2021) find that vaccination efficacy in terms of protection against deaths was around 72%, with a fall in number of deaths. Similar results are documented for the US. Schneider et al. (Reference Schneider, Shah, Sah, Moghadas, Vilches and Galvani2021) observe that if not for the vaccination programme, there would have been approximately 1.1 million more deaths as a result of COVID-19 and more than 10.3 million additional COVID-19 hospitalisations in the US by November 2021. On 21 June 2021, only 10.04% of the world population had been fully vaccinated, with just 0.9% of people in low-income countries receiving one dose (Gleeson Reference Gleeson2021). Clearly, global and local inequalities affect pandemic outcomes, and the size of budgets is potentially a major part of the story.
The unequal access to resources has clearly played a part in how the COVID pandemic has progressed across the world. Clearly, access to financial resources is in only one tool, albeit an important one, when fighting a highly contagious disease. How money and budgets translate into effective action, however, is critical. As many suggest, larger health budgets do not always correlate with good health outcomes. How money is deployed must matter. We examine whether government health budgets on COVID deaths have been conditional on having more effective governance, such as more equitable healthcare systems and lower political corruption. Given that such large volumes of spending could easily be misappropriated, we examine whether health spending conditioned by good governance has influenced the number of COVID deaths. While politicians and the public expect government spending to solve the problem, experts on public sector finance often see public spending as either wasteful or benefiting only the rich, through what is known as “tax churning” (Tanzi Reference Tanzi2011). We argue that the degree of effectiveness of health spending on fighting the COVID pandemic might very well be conditional on the degree to which a robust health system in terms of capacity to reach people exists and the true purpose and intent of public policy, which might be assessed best by gauging the degree of political corruption.Footnote 2
Using a cross-section of data for 213 countries which faced the COVID-19 pandemic up until 15 June 2021, we find evidence to suggest that spending levels in the past correlate with higher numbers of COVID deaths, suggesting that health spending is a poor predictor of pandemic outcomes. Consistent with our expectations, however, government spending on health care reduces the number of COVID-19 deaths conditional on an equitable healthcare system and lower levels of corruption. Our results demonstrate that the capacity to reach and treat captured by equitable access matters more than the size of a country’s health budget. Indirectly, therefore, our study also suggests that higher health spending alone need not necessarily lead to the existence of effective and resilient health systems if indeed spending does not build equitable health infrastructure. Thus, it is not the size of budgets alone but the quality of governance that helps tackle the worst of a pandemic. The results are robust to alternative testing procedures, including the application of instrumental variable (IV) technique for addressing endogeneity concerns.
Previous research
Arguably, the main tool in the hands of governments for solving public health crises is to increase spending on healthcare. Scholars have long debated, however, the efficacy of the public sector spending money to resolve problems (Tanzi Reference Tanzi2011). Studies suggest that an increase in government spending alone may not necessarily lead to improvements in healthcare and disease outcomes, while the question of whether private spending does better than public is still debated (Filmer and Pritchett Reference Filmer and Pritchett1999; Deaton Reference Deaton2013; Gallet and Doucouliagos Reference Gallet and Doucouliagos2017; Linden and Ray Reference Linden and Ray2017).
While some studies show a positive relationship between government health spending and health outcomes, others show a negative or no relationship at all. Owusu et al. (Reference Owusu, Sarkodie and Pedersen2021) examine the effect of health expenditure on infant and maternal mortality rates and find that higher health expenditure reduces both infant and maternal mortality rates in low- and middle-income countries. Chirowa et al. (Reference Chirowa, Atwood and Van der Putten2013) similarly observe that countries which spend more on health care experience lower maternal deaths compared to those which spent less. Ray and Linden (Reference Ray and Linden2020) using sophisticated econometric analysis find that the efficiency of transforming spending into better outcomes varies greatly among countries across the world. Filmer and Pritchett (Reference Filmer and Pritchett1999) in a study of the effects of public health spending on child and infant mortality find no strong link between the two variables, however. They argue that the efficacy of public health spending depends on the degree to which it can generate effective public services. Similar findings are documented by Bhalotra (Reference Bhalotra2007) for infant mortality rates in India. However, when controlling for lagged effects and other variables, a significant effect emerges.
While finance is undoubtedly necessary for building up a resilient health system that can handle epidemics and pandemics, it is uncertain that governments really target monies efficiently for such purposes. An increase in spending on healthcare, therefore, is only a sufficient but not necessary condition for improved health outcomes. If the majority of the population in a country do not have access to healthcare despite high expenditures, this might result in a high number of deaths when a pandemic hits. Regardless, theoretically at least, a government that spends well on health is likely to be building resilience and capacity of a health system to cope with a citizenry’s health needs and future contingencies, such as epidemics and pandemics.
The recent outbreak of the COVID pandemic has highlighted once again, that high healthcare expenditure need not necessarily reflect better healthcare outcomes. For example, the US spent twice as much as other high-income economies on healthcare in 2016, but had lower health outcomes in terms of life expectancy and infant mortality rates, compared with Organization for Economic Cooperation and Development (OECD) counterparts (Papanicolas et al. Reference Papanicolas, Woskie and Jha2018). Indeed, the US is the only country among the industrialised countries that has recently seen its life expectancy decline, mostly due to what some term “deaths of despair,” despite having one of the world’s most expensive healthcare systems, much of that expense reflecting corporate corruption rather than public goods (Deaton and Case Reference Deaton and Case2020). Likewise, although health expenditure per person in the US is US$ 9,237, the highest in the world, the US experienced the largest number of COVID deaths, 205,666 as of 3 October 2020 (Brink Reference Brink2017).Footnote 3 Spending statistics might hide much that is relevant in such a case, however, because disparities in healthcare access can influence pandemic outcomes. Chen and Krieger (Reference Chen and Krieger2020), in a study of the US, show that the highest COVID-19 death rates were observed for those living in the most disadvantaged counties in relation to poverty – 19.3 per 100,000, compared to 9.9 per 100,000 in the most economically advantaged counties (Brink Reference Brink2017; Chen and Krieger Reference Chen and Krieger2020). If a health system reflects inequality in access, then one might assume that a government’s ability to reach and treat people, when crises occur, will remain limited. Similar findings have been documented for other countries. For example, spending on healthcare per person in the UK, is US$ 3749, but the UK also experienced a large number of COVID deaths – 42,202 per 100,000 as of 3 October 2020.Footnote 4 The Intensive Care National Audit and Research Centre in the UK observed that 35% of approximately 2,000 patients were from nonwhite backgrounds with lower access to healthcare, although they comprised only 13% of the total population of the UK (Booth Reference Booth2020).
Evidence suggests that countries with equal access to healthcare have been more successful in containing the virus and have experienced fewer deaths (Vadlamannati et al. Reference Vadlamannati, Cooray and de Soysa2020). For instance, Thailand, which has moved towards an universal healthcare system, has experienced only 59 deaths as of 3 October 2020, despite spending only US$ 400 per person on healthcare (Brink Reference Brink2017). Australia, which has a well-developed and equitable public health system, has handled the COVID crisis much better than many countries in Europe and the US. Thus, rather than spending levels alone, the infrastructure for accessing people might be critical for reducing the spread of disease. Bosancianu et al. (Reference Bosancianu, Hanno Hilbig, Humphreys, Sampada, Lieber and Scacco2020), however, challenge the use of political solutions to social problems. They examine the influence of state capacity, political institutions, political priorities, and social structures to investigate variation in the ability of countries to deal with the COVID-19 pandemic. They observe that interpersonal and institutional trusts are consistently positively associated with pandemic mortality. In contrast to other studies, they do not find a robust relation between pandemic mortality and press focused on populist governments, women-led governments, pandemic preparedness, state capacity, democracy, and inequality.
Indeed, high health spending could simply be “pork” or wasteful spending designed to buy political support rather than generate effective public policy. High healthcare spending could also be spending that reflects high levels of corruption or a political economy where governance is unresponsive to the needs of ordinary people – corruption kills, particularly the poor and vulnerable (Hanf et al. Reference Hanf, Van-Melle, Fraisse, Roger, Carme and Nacher2011) – and contingencies for dealing with a crisis is likely to be weak under these circumstances. It is documented that approximately US$ 455 billion of the US$ 7.35 trillion spent on health care worldwide is lost each year to some form of corruption.Footnote 5 According to the OECD, a third of their citizens and 45% of citizens globally consider the health sector to be corrupt (OECD 2017). This is because corruption in the health sector can transpire in various areas including health facility construction, the purchase of technology and equipment, the purchase of pharmaceuticals, procurement of pharmaceuticals etcetera (Vian Reference Vian2008). One study reports that 1.6 per cent of annual deaths of children under 5 globally, which is more than 140,000 deaths, might be explained in part by corruption (Hanf et al. Reference Hanf, Van-Melle, Fraisse, Roger, Carme and Nacher2011). The IMF estimates that infant and child mortality in countries with high levels of corruption are almost double that of countries with low levels of corruption (Gupta et al. Reference Gupta, Davoodi and Tiongson2000). Concerns of a comparable nature have been highlighted by a Berlin-based anti-fraud consulting firm, Nemexis, regarding the recent COVID outbreak. Nemexis finds that fraud and corruption in healthcare services have contributed to COVID-19-related deaths in every third country of the 58 countries surveyed (Medcity News 2020).
Governments typically use spending as a primary tool for addressing health crises, and spending might indeed have resulted in necessary infrastructures for addressing epidemics and pandemics. We argue that health spending is likely to be effective only if healthcare is distributed equitably, or to be specific, more pro-poor. What matters is not specifically equity per se, but by following pro-poor strategies, states are likely to have built up health provision capacity by being able to reach and treat people, or the capacity to access people that are most vulnerable to catching and spreading disease. We argue, thus, that health spending is only likely to reduce the impact of COVID when conditioned by an effective health system measured as equitable access. Similarly, health spending should have the most effective impact when accompanied by low political corruption. We contribute to the literature by showing that (1) high healthcare expenditure by the government need not necessarily lead to better outcomes in terms of COVID deaths unless accompanied by greater equity in healthcare access, which essentially proxies for health system capacity and reach; (2) high healthcare expenditures could suggest high levels of corruption leading to a higher number of COVID deaths. The findings of our study will have important implications for policy as limited access to health services and corruption in politics affects all processes and systems in place to fight disease, not least the trust and confidence in government required of a citizenry.
Data and methods
Model specifications
To examine our theoretical propositions, we utilise a cross-sectional dataset containing 213 countries for which COVID-19 death rates are reported as of 15 June 2021 when we began this study. We estimate:

wherein ln (COVID) c captures COVID-19 deaths per million (log) in country c as of 15 June 2021. The data are sourced from the Worldometer COVID-19 dataset, which is an ongoing data collection project, manually sourcing real-time information on the COVID-19 pandemic from various countries across the world.Footnote 6 A global COVID-19 live statistic is generated by analysing, validating, and aggregating the data collected from various sources.Footnote 7 The mean value of COVID-19 deaths in our sample is 608 per million, while the standard deviation is 810 per million, which shows the wide variation in the way in which this disease has impacted death rates across countries. The maximum value of deaths is roughly 5649 per million and a minimum value of 0 per million. Figure 1 captures the COVID-19 deaths per million across the world on 15 June 2021. Figure 1 suggests significant variation in the number of COVID deaths across countries. This figure also reflects the uneven spread of COVID-19 deaths. Appendix 2 provides a full list of countries with the number of COVID-19 deaths and deaths per million.

Figure 1. COVID-19 deaths per million (log) across the world (15 June 2021).
The hypothesis variable denoted GHS/GDP c measures the level of government spending on healthcare as a share of GDP in country c as the five-year average before 2019(2014–2018) sourced from the World Bank’s 2020 World Development Indicators (WDI) online database.Footnote 8 Average government spending on health to GDP in our sample is roughly 3.4% with a minimum value of 0.18% (Venezuela) and a maximum of 9.22% (Sweden).
The vector of control variables ( Z c ) includes other potential determinants of COVID-19 outcomes, based on the literature emerging on the subject (Cepaluni et al. Reference Cepaluni, Dorsch and Branyiczki2020; Chen and Krieger Reference Chen and Krieger2020; Vadlamannati et al. Reference Vadlamannati, Cooray and de Soysa2020). The list of potential control variables is long, and we are aware of the trap of “garbage-can models” in which numerous variables are lumped onto the right-hand side of the equation, making meaningful interpretation of results difficult (Achen Reference Achen2005). Therefore, we adopt a conservative strategy of accounting only for key factors that affect COVID-19 outcomes and government spending. In robustness checks, we add several more control variables. Moreover, given the uneven spread of COVID-19 deaths across countries it is likely that there are many other factors which may be potentially important but are omitted from our baseline models (e.g. degree of geographic isolation, ruggedness of the terrain, interconnectivity of populations). It is, therefore, important that we control for all other potential factors explaining the variation in COVID deaths in robustness tests.
Accordingly, we include the level of development measured by per capita income in US$ 2010 constant prices obtained from the WDI. The income level has a bearing on COVID-19 deaths through its impact on health spending as richer countries will usually spend more on healthcare. Next, we also include a measure of urbanisation (percentage share of urban population) as studies show that the transmission of COVID cases is high in urban centres, and the degree of urbanicity could influence the degree of health spending. We also control for democracy using the Freedom House civil rights and political liberties indices as previous studies on COVID-19 find that democratic countries experienced more COVID deaths compared to nondemocratic countries. Democracies generally also have higher health spending. We take the average value of the civil rights and political liberties indices to measure democracy and re-scale them on a 1–7 scale in which a lower value of 1 denotes complete absence of civil rights and political liberties and vice-versa.Footnote 9 We also include a measure of life expectancy at age 60, which reflects the mortality level of a population in country c sourced from the World Health Organization’s (WHO) data platform.Footnote 10 This variable captures the vulnerability of the population to COVID-19, as elderly populations with comorbidities are less likely to survive the virus (Jordan et al. Reference Jordan, Adab and Cheng2020). Finally, we also include COVID-19 cases per million (log) in country c as of 15 June 2021 sourced from the Worldometer COVID-19 dataset. It is noteworthy that COVID mortality data depend on the quality of systems of vital registration. AbouZahr et al. (Reference AbouZahr, Bratschi, Cercone, Mangharam, Dincu, Forsingdal, Joos, Kamal, Fat, Mathenge, Marinho, Mitra, Montgomery, Muhwava, Mwamba, Mwanza, Onaka, Sejersen, Tuoane-Nkhasi, Sferrazza and Setel2021) find significant intercountry variation on COVID deaths. They highlight the difficulties faced by countries in maintaining data systems, which require detailed and continuously available mortality statistics. In order to take this variation into account, we control for COVID-19 cases per million (log). We also undertake a robustness check where we replace our dependent variable COVID deaths per million (log) with the fatality rate.
It should be noted that we use the previous five-year averages (2014–2018) of each of these variables (with the exception of COVID cases variable). The descriptive statistics are provided in Appendix 3, and data definitions and sources are provided in Appendix 4. We use an OLS estimation specification with Huber-White corrected robust standard errors, a method which is robust to heteroskedasticity (Wiggins Reference Wiggins1999). We also include geographic regional dummies (
${\lambda _r})$
to account for the difference in the susceptibility to viruses based on geographic factors.
Endogeneity
It is quite plausible that our measure of government health expenditure is an outcome rather than the cause of COVID-19. Even though we use the previous five-year average, COVID-19 might be more deadly where other viruses also have been more deadly in the past, potentially biasing the result between spending and deaths. This issue is not trivial because those who argue that government health spending impacts the response of the system to health pandemics also make causal claims that health spending by governments is an outcome of health pandemics (Lafortune Reference Lafortune2020). Furthermore, government health spending could reflect other factors that explain COVID-19 outcomes, such as budgetary constraints, state capacity in terms of administrative efficiency and reach of the healthcare system. To address the problem of endogeneity, we utilise a two-stage least squares instrumental variable (2SLS-IV hereafter) estimator including the control variables discussed above, along with geographic regional dummies. We use two IVs, namely average public sector health spending to GDP of geographic neighbours sharing borders with country c
and average public sector health spending to GDP of countries in the geographic region
Footnote
11
of country c
. The validity of the instruments depends on two conditions. First, they must pass the instrument relevance test, which is that the selected instruments must be correlated with the explanatory variable. It is customary to accept instrument relevance if the joint F-statistic in the first stage regression is above 10 (Bound et al. Reference Bound, Jaeger and Baker1995). Furthermore, we apply a more powerful test, namely the Kleibergen-Paap F-statistic, which offers reliable statistical inferences in a weak instrument setting (Kleibergen and Paap Reference Kleibergen and Paap2006). Once again, the null of weak instruments can be rejected if the F-statistic is above the critical value of 10. Second, the selected instruments should not differ systematically with the error term in the second stage of the equation, i.e.
$\left[ {{\omega _{it}}\;\left| {\,I{V_{it}}} \right.} \right]\; = \;0$
. In other words, the selected instruments should not be correlated with the outcome variable of interest – COVID-19 deaths – except through health spending.
We believe that our instruments satisfy the exclusion restriction based on the following logic: previous studies find a strong correlation between health spending of a country and its geographic neighbours (Benos et al. Reference Benos, Karkalakos and Zotou2019). A rationale for this is that if neighbouring countries have better health outcomes because of higher spending on healthcare services, it influences the discourse of the healthcare system in the country in question and presents an opportunity to learn, if not imitate. Research suggests that governments of neighbouring countries often coordinate their health policies to achieve similar goals for their citizens, such as the prevention of contagious diseases (Baltagi et al. Reference Baltagi, Moscone and Tosetti2012; Benos et al. Reference Benos, Karkalakos and Zotou2019). It should be noted that these instruments have been used in previous studies on government spending on health (Filmer and Pritchett Reference Filmer and Pritchett1999; Wagstaff and Claeson Reference Wagstaff and Claeson2004; Benos et al. Reference Benos, Karkalakos and Zotou2019). If a neighbourhood spillover occurs in health spending, deaths from COVID should not necessarily be similar due to the spillover across borders, given the restrictions on travel and other lockdown measures. We find the joint F-statistic from the first stage rejects the null hypothesis that our selected instruments are not relevant. In fact, we obtain a higher joint F-statistic and Kleibergen-Paap F-statistic on both estimation models as reported in Table 1, which remain significantly different from zero at the 1% level.
Table 1. Government spending on health and COVID-19 deaths per million (log)

Note: Standard errors in parenthesis. Statistical significance:
*** p < 0.01,
** p < 0.05,
* p < 0.1.
Interaction effects
Next, we introduce interaction terms to examine whether the effect of government health spending on COVID-19 deaths is conditional upon (i) an equitable health system and (ii) the degree of political corruption. We estimate the following conditional equations:


wherein
$\left( {GHS/GDP}\; \times \;equity\ \right)_c; $
is the interaction term and
${equit{y_c}}$
measures the extent of equity in healthcare in country
c
. The VDEM projectFootnote
12
measures the degree to which any given country at any given time provides access to adequate healthcare for the poor that is comparable with the healthcare accessed by the rich. The VDEM data take into account several aspects of equity to measure the equal distribution of political power in any given society in terms of gaining access to the government and to resources that empower people politically and enable people to participate meaningfully in economic activity (Coppedge et al. Reference Coppedge, Gerring, Lindberg, Skaaning, Teorell, Altman, Andersson, Bernhard, Fish, Glynn, Hicken, Knutsen, Marquandt, McMann, Mechkova, Paxton, Pemstein, Saxer, Seim, Sigman and Staton2017). The VDEM data on equity are generated by asking several country experts to score countries on the following question, according to the scale below:
To what extent is high-quality basic health guaranteed to all, sufficient to enable them to exercise their basic rights as adult citizens?
0: Extreme. Provision of high-quality basic health is extremely unequal, and at least 75 per cent (%) of citizens receive such low-quality health that undermines their ability to exercise their basic rights as adult citizens.
1: Unequal. Provision of high-quality basic health is extremely unequal, and at least 25 per cent (%) of citizens receive such low-quality health that undermines their ability to exercise their basic rights as adult citizens.
2: Somewhat equal. Basic health is relatively equal in quality but ten to 25 per cent (%) of citizens receive such low-quality health that undermines their ability to exercise their basic rights as adult citizens.
3: Relatively equal. Basic health is overall equal in quality but five to ten per cent (%) of citizens receive such low-quality health that probably undermines their ability to exercise their basic rights as adult citizens.
4: Equal. Basic health is equal in quality and less than five per cent (%) of citizens receive such low-quality health that probably undermines their ability to exercise their basic rights as adult citizens.
The VDEM codes health equality by consulting numerous country and regional experts who make subjective judgements about the level of access of the poorest segments of society to healthcare compared with the richest segments. These expert codings are then subject to rigorous scrutiny and testing, using item response theory that reduces uncertainty in the coding and assigns a single value to each country for each year (Pemstein et al. Reference Pemstein, Marquardt, Tzelgov, Wang, Krusell and Miri2018). The data are coded as health equality ranging from −3.99 to +3.16, where higher values capture greater equity. Once again, we use the five-year average (2014–2018).
In the second interaction model in equation (3), we examine the role of political corruption (
$corr{p_c}$
) on COVID-19 deaths per million (log). Accordingly, we use the
corruption index
developed by the VDEM project that measures the degree of political corruption within a regime, assessed as the prevalence of neopatrimonial and clientelist tendencies within the regime taken as a whole (executive embezzlements, executive bribes, legislature and judiciary) and corruption specific to the public sector (McMann et al. Reference McMann, Pemstein, Seim, Teorell and Lindberg2016). The corruption indicators are based on country experts who answer a specific question on corruption, which is:
To what extent do political actors use political office for private or political gain?
The expert coding is then subjected to rigorous Bayesian factor analysis, namely item response theory, for minimising intercoder bias and increasing reliability. According to VDEM, regime corruption is defined in the following manner.
In systems of neopatrimonial rule, politicians use their offices for private and/or political gain. This index relates closely to V-Dem’s political corruption index (v2x_corr) but focuses on a more specific set of actors – those who occupy political offices – and a more specific set of corrupt acts that relate more closely to the conceptualisation of corruption in literature on neopatrimonial rule (VDEM 2019, 274).
The corruption index is coded on a 0–1 scale where a higher score denotes more regime corruption and vice-versa. As before, we use the five-year average of this index (2014–2018). Again, we employ the OLS estimator robust to heteroskedasticity including the geographic regional fixed effects to estimate equation (2) and (3). We generate marginal plots to assess the interaction effects and their significance (Brambor et al. Reference Brambor, Clark and Golder2006).Footnote 13
Empirical results
Table 1 reports the impact of government spending on health on COVID-19 deaths. While columns 1–2 show the results estimated with OLS with basic control variables and controlling for geographic regional dummies, columns 3–4 present findings using the 2SLS-IV estimator to address endogeneity concerns. Finally, Table 2 presents the results of the interaction effects. As seen in column 1, Table 1, a higher share of government spending on health per GDP has a positive effect on COVID-19 deaths, which is significantly different from zero at the 1% level.
Table 2. Conditional effects of health spending on COVID-19 deaths per million (log)

Note: Standard errors in parenthesis. Statistical significance:
*** p < 0.01,
** p < 0.05,
* p < 0.1.
Substantively, a standard deviation increase in the spending share in health increases COVID deaths per million (log) by roughly 22% of a standard deviation of deaths per million. This effect is not inconsequential because it suggests that an additional 40.4 individuals per million die for each percentage increase in health spending. In column 2, when we enter the controls, the positive and statistically significant effect of government spending holds, albeit at the 5% level of significance. The substantive effects are reduced only slightly. These results suggest that government spending on healthcare has had the opposite effect of cushioning the pandemic. With respect to the control variables, only urban population share shows a positive and significant effect on COVID-19 deaths, consistent with the findings of others (Chen and Krieger Reference Chen and Krieger2020). The results are robust across the columns in Table 1. Interestingly, while income per capita and the level of democracy remain statistically not significant, urbanisation and life expectancy over the age of 60 remain significantly different from zero at the conventional levels of statistical significance. As expected, a higher life expectancy is not associated with an increase in COVID deaths. Urbanisation on the other hand increases deaths from COVID-19.
In columns 3–4, we present the IV estimations. We correct for endogeneity of health spending and deaths using two IVs. While column 3 reports the results of COVID-19 deaths without any controls, column 4 includes the other control variables. There are three observations that can be drawn from these results. First, the IV estimation results for government spending on healthcare to GDP on COVID-19 deaths per million in columns 3–4 are similar to those reported in our baseline estimates in columns 1–2. The positive and statistically significant effect of health spending to GDP on COVID-19 deaths remains after controlling for endogeneity concerns. Second, the substantive impact after instrumenting for spending remains similar to the results in columns 1–2 estimated using OLS. Holding the controls constant, a standard deviation increase in government spending on health to GDP is associated with an increase in COVID deaths per million (log) by 23% of a standard deviation of COVID deaths (log). The instrumented effects are roughly similar as the impact of the standard linear regression effects (column 2). This suggests that our basic results may not reflect endogeneity bias, i.e. from omitted variables or reverse causality. Thirdly, note that the additional statistics provided below in columns 3–4 suggest that the selected instruments are valid. The joint F-statistic from the first stage rejects the null that the instruments selected are not relevant. In fact, we obtained a higher joint F-statistic and Kleibergen-Paap F-statistic on both estimation models reported in columns 3–4, which remain significantly different from zero at the 1% level. Moreover, we employ the Hansen J-test to assess whether the selected instruments satisfy the exclusion restriction criteria (Hansen Reference Hansen1982). The Hansen J-statistic shows that the null of exogeneity cannot be rejected at the conventional level of significance in the 2SLS-IV models, suggesting that our instruments pass the instrument exclusion criteria. Taken together, our results on the impact of government spending on health to GDP remain robust to alternative estimation techniques and potential endogeneity. The results for the control variables are roughly the same as those reported in columns 1–2.
In Table 2, we introduce the interaction terms between government spending on health to GDP and health equity and the level of corruption.
In columns 1–2, we show the conditional effects of healthcare spending share in GDP and equitable access to healthcare index, while columns 3–4 report the interaction effects for healthcare spending and regime corruption on COVID-19 deaths per million. As seen in column 1, without any controls, the interaction term is negative and statistically significant at the 5% level. The interaction term continues to be statistically significant at the 5% level in column 2 when we include other control variables. Interestingly, healthcare spending to GDP on its own, i.e. when the health equity index is 0, has a positive and statistically significant effect on COVID-19 deaths per million as estimated in Table 1. It is also interesting to note that the access to healthcare equity index on its own, when health spending/GDP is 0, has a negative effect on COVID-19 deaths per million. This is not surprising and is in line with previous studies showing the importance of equitable access to health care for reducing COVID death (Vadlamannati et al. Reference Vadlamannati, Cooray and de Soysa2020). It seems that the capacity to reach and treat captured by equitable access matters more than just the financing power of health spending.
The interpretation of the interaction terms even in linear models is not straightforward. Consequently, a simple t-test on the coefficient of the interaction term is not sufficient to examine whether the interaction term is statistically significant (Ai and Norton Reference Ai and Norton2003). We rely on margins plots which depict the magnitude of the interaction effect and their significance levels along the scale of the conditioning variable, given increases in the x variable of interest.
To calculate the marginal effect of government health spending to GDP on COVID-19 deaths, we consider both the conditioning variable (healthcare equity index) and the interaction term and display graphically the total marginal effect conditional on healthcare equity coded on a scale of −3.99 and 3.16. The y-axis of Figure 2 displays the marginal effect of government spending on health/GDP. The marginal effect is evaluated on the healthcare equity index on the x-axis. Note that we include the 90% confidence interval.

Figure 2. Government health spending, health care equality & marginal effect on COVID-19 deaths (per Mn).
As seen in Figure 2, and in line with our theoretical expectations, government spending on health to GDP actually reduces the COVID-19 deaths per million when the healthcare equity index increases from −3.99 to 3.16. For instance, the marginal effects suggest that government spending on health to GDP is associated with an increase in COVID-19 death per million (log) by only 16% when the healthcare equity index is at a score of 2, i.e. compared to 53% in a country where access to health in completely unequal. This result is significantly different from zero at the 5% level. These results suggest that countries with a robust health system and equal access to health care have a higher capacity to deal with a pandemic. In other words, government spending on healthcare could generate positive outcomes if such expenditure goes towards improving equity and greater access to healthcare facilities for its citizens, or in other words, increase capacity to reach and treat people.
We turn next to the conditional effect of healthcare spending and corruption presented in column 3–4. The interaction term is positive and significant, suggesting that higher healthcare spending conditional on increasing corruption results in increased death from COVID-19. Once again, we examine the margins plot for a graphical interpretation of the magnitude of the interaction effect. On the y-axis of Figure 3, we show the marginal effect of an additional increase in a unit of the health spending to GDP, whereas the x-axis shows the regime corruption index at which the marginal effect is evaluated. As before, we include the 90% confidence interval in Figure 3.

Figure 3. Government health spending, corruption & marginal effect on COVID-19 deaths (per Mn).
The conditional plot in Figure 3 reveals that an additional unit of healthcare spending to GDP increases COVID-19 deaths per million (log) when the corruption index is above 0.2 (on the scale of 0-1). For instance, health spending increases COVID-19 deaths per million (log) by 30% when corruption is very high (i.e. an index score of 1), which is statistically significant at the 5% level. Once again, the gains realised in the fight against COVID-19, as shown in Figures 2 and 3, occur not when government spending on healthcare is high, but when accompanied by greater access to healthcare and low regime corruption. Overall, our results from the interaction effects suggest that the negative effect on COVID-19 deaths largely accruing from the “structural effect” of having a robust health system, i.e. the question of capacity and reach rather than mere spending on healthcare by governments. In a way, our results are also in line with those who argue that the unequal burden of the COVID-19 pandemic in countries might be traced to dilapidating public health infrastructure and inequitable access (Quinn and Kumar Reference Quinn and Kumar2014; Chen and Krieger Reference Chen and Krieger2020; Van Dorn et al. Reference Van Dorn, Cooney and Sabin2020). Also, fighting disease might need all of government effort, such as reduced corruption, and not simply increased spending.
Robustness checks
We examine the robustness of our findings in several ways. We report the regression result Tables and Figures on all robustness tests in an online appendix. First, to provide more credence to our analysis, we assess if our results hold up if we examine COVID-19 deaths over time. However, given the cross-sectional nature of our data, we intend to examine if the relationship between our main variable of interest and COVID-19 deaths hold by considering one other data point in time. We use COVID-19 deaths per million (log) from July 2020, which is roughly a year prior to the current observation we use in our main analysis in Tables 1 and 2. We re-estimate our baseline estimates with the earlier death count including in the IV estimations and the interaction models reported in Tables 1 and 2. We report these results in Table A in the online appendix (see supplementary file). Our main results remain robust to using COVID-19 deaths from the previous year. While the coefficient values are lower, the relationship remains robust. The margin plots capturing the conditional effect of health care equity and corruption are reported in Figures A and B. These results remain similar to those reported in our main estimations. Second, we use an alternate dependent variable instead of COVID deaths, since some scholars argue that mortality data are contingent upon the quality of systems of registration and could be under-reported by some countries (Beaney et al. Reference Beaney, Clarke, Jain, Golestaneh, Lyons, Salman and Majeed2020). The quality of reporting systems, however, does not always correlate with the quality of COVID testing data, which form the basis for data on mortality numbers (Baccini et al. Reference Baccini, Rocco, Paganini, Mattei, Sani, Vannucci, Bisanzi, Burroni, Peluso, Munnia, Cellai, Pompeo, Micio, Viti, Mealli and Carozzi2021). We use COVID-19 fatality rate data instead of COVID deaths per million as an alternative dependent variable to test the robustness of our findings. Furthermore, the fatality rate might better reflect the performance of healthcare systems compared with deaths per capita. While the mean value of the fatality rate is about 2%, the maximum value is 19.7% in our sample. Our results using the fatality rate is reported in Table B and Figure C and D capturing conditional effects (online appendix). The results, once again, remain robust to using the fatality rate as a dependent variable. It is noteworthy that these results are robust to using IV estimations and that we obtain a higher joint F-statistic and Kleibergen-Paap F-statistic with these alternative estimations.
Next, we use alternative operationalisations of the health spending measures. We replace health spending as a share of GDP with per capita health spending (log) measure. The denominator, GDP, which is somewhat volatile, might affect the share of health spending even though we use the 5-year average. Our results are displayed in Table C (Figure E and F, conditional plots) of the online appendix, and they remain robust to using the health spending per capita (log) measure. The IV estimations and conditional effects reflect our baseline results in Tables 1 and 2. Our results are not sensitive to how we operationalise our main independent variable of interest.
Fourth, we include a range of other variables into the model to control for important omissions from our baseline models. Accordingly, we include a dummy variable capturing countries which are classified as highly indebted by the World Bank. This variable takes the value 1 if debt is over 50% of GDP and 0 otherwise. The data are sourced from the World Bank (2020) WDI. We also include a measure of trade openness (total trade to GDP log) capturing the interconnectivity of business sourced from the WDI (2020). We also capture interconnectivity of people using international air travel passengers (log) of each country during last 5 years (average) sourced from the WDI (2020). We also include two dummy variables capturing island nations and whether or not a country is tropical to control for the degree of geographical isolation and climatic conditions. We also include a measure of ruggedness of the terrain using the terrain ruggedness index, 100m (log) developed by Nunn and Puga (Reference Nunn and Puga2012). We also create a dummy variable using information from the WHO (2003) to capture the extent to which a country was affected by the previous SARS pandemic during the 2002–2004 period. This variable is expected to capture previous experience of dealing and preparing for pandemics. Lastly, we include the COVID-19 vaccination rate measured as a percentage of the population that is fully vaccinated, sourced from Basta and Moodie (Reference Basta and Moodie2020), to control for the effect of vaccinations on COVID outcomes. New research suggests that vaccine deployment reduces new COVID-19 infections (Deb et al. Reference Deb, Furceri, Jimenez, Kothari, Ostry and Tawk2021). The results are reported in Table D (conditional plots in Figures G and H). The inclusion of these controls in alternative models makes little difference to the basic results reported in Tables 1 and 2.
Fifth, we use alternative instruments, namely CO 2 emissions per capita (log). Previous studies show that CO2 emissions significantly increase healthcare expenditures (Ullah et al. Reference Ullah, Ali, Shah, Yassim, Rehman and Al-Ghazali2019; Gündüz Reference Gündüz2020). Our results remain robust to using these alternative instruments. These variables pass the instrument relevance test with joint F-statistics above the threshold of 10. Finally, we use the Global Health Security (GHS) index developed by Bell and Nuzzo (Reference Bell and Nuzzo2021) from Johns Hopkins as our main variable of interest to estimate all our baseline models (including the interaction models).Footnote 14 Our results are presented in Table F in the online appendix. The results remain robust when utilising the GHS, which seems to correspond very well with the size of health budgets. Thus, one might suggest that higher spending in societies with equitable health access and lower corruption might even serve well as a measure of health security capacity that can be used to measure preparedness for facing future crises. Preparedness indices based simply on the size of budgets are likely to provide a biased picture.
Conclusion
Governments across the world have committed to spending on health as a way to deal with the COVID pandemic, presumably to build resilience against any such future pandemic. Global financial institutions are making available vast amounts of money for alleviating the pain from COVID-related economic and social crises. While spending is a primary tool for governments to address crises, such as health crises, it is not at all certain that money alone matters in terms of effecting favourable outcomes (Lindert Reference Lindert2021). Our results show that higher levels of public spending on health in the past have not cushioned societies from the worst outcomes of the COVID-19 pandemic. Indeed, higher spending on average in the 5 years prior to 2020 has resulted in higher COVID-19 deaths per million, controlling for a host of relevant factors, including employing an IV approach for addressing potential endogeneity. Compared to spending, having an equitable health system seems to matter negatively on death, suggesting that what matters is having an infrastructure capable of reaching and treating people, or conducting effective public action for preventing the spread of the virus among the truly vulnerable. Conditionally, higher spending seems to matter for reducing deaths only among societies with equitable health access as is higher spending accompanied by lower regime corruption. Our results are in contrast to that of Bosancianu et al. (Reference Bosancianu, Hanno Hilbig, Humphreys, Sampada, Lieber and Scacco2020), who find that state capacity does not influence COVID mortality, in so far as corruption and equity relate also to state capacity.
International and local policies aimed at building resilient health systems against future pandemics might do well to focus less on quantities spent than on the details of building equitable systems that allow greater access, paying attention to questions of governance and targeted ways in which viruses might be combated. If issues of governance and equity are not addressed, they would place an increasing burden on those from lower-income groups, further aggravating inequalities and health outcomes in the future. Even democratic societies might not object too greatly to frivolous spending and may focus more on other priorities over health, which means that more targeted mechanisms would have to be deployed for transforming public health spending towards delivering more effective health systems. Finally, studies also show that a country’s ability to deal with crises could depend on the level of government involved in making the decision (Treisman Reference Treisman2002; Kumar and Prakash Reference Kumar and Prakash2017; Bosancianu et al. Reference Bosancianu, Hanno Hilbig, Humphreys, Sampada, Lieber and Scacco2020). According to these studies, decentralisation improves health service provision by increasing accountability. The difficulty in obtaining data at the sub-national level precludes us from capturing the level of government responsible for allocating health service expenditures. We believe this could be a line of inquiry for future research as more disaggregated data become available.
Supplementary material
To view supplementary material for this article, please visit https://doi.org/10.1017/S0143814X22000216.
Data availability statement
de Soysa, Indra, 2022, “Replication Data for: Can Bigger Health Budgets Cushion Pandemics? An Empirical Test of COVID-19 Deaths Across the World,” https://doi.org/10.7910/DVN/HKT3LO, Harvard Dataverse, V1, UNF:6:hhgi/lEAhmfKkfqNGFTcsQ== [fileUNF]
Acknowledgements
A preliminary version of this study was published within the UNU-WIDER project on “Academic Excellence.” We thank the Editor and two anonymous referees for providing extremely constructive suggestions. We also thank Paul Patrick Walsh, Samuel Brazys, Aidan Regan, Yoo Sun Jung, Artur Tamazian, the research group on health inequalities (CHAIN) at the Dept. of Sociology & Political Science (NTNU), and participants at the WEAI conference for valuable comments. The usual caveat applies.
Appendix 1. List of countries

Appendix 3. Descriptive statistics

Appendix 4. Data sources and definitions
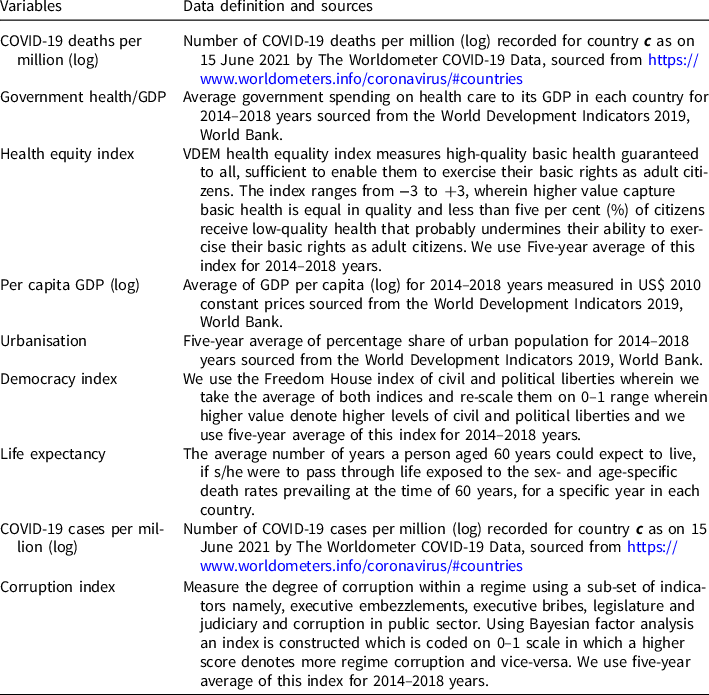