The pervasiveness of modern digital media in the lives of children and teens has raised important questions about how exposure to, and involvement with, such media might interact with the developing brain. The framing of these questions reflects differing implicit beliefs about the direction of causality in this relationship. Some questions assume that facets of brain development can predispose youth to digital media involvement. It is tempting to ask, for example, whether maturational processes taking place within the brain might prejudice a given age group to become especially enmeshed with digital media, or whether individuals whose brain development lags behind (or is relatively more precocious than) that of their peers also incur greater vulnerability to the consequences of digital media use. Conversely, questions can be posed with the underlying assumption that digital media environments can themselves impact subsequent brain development. We might wonder, for instance, whether digital media experiences have the potential to fundamentally “rewire” the cabling patterns in developing brains, or to stunt or alter the normative developmental processes that lead to a “mature” functional brain. Seeking answers to questions like these carries obvious importance in informing how we, societally and individually, approach the introduction and management of digital media in the lives of our children and teens.
In this chapter, we explore evidence that shapes our current understanding of the relationship between the developing brain and digital media experiences. Although there are many tools that can be applied to the question of how the developing brain affects, and is affected by, digital media behaviors, noninvasive magnetic resonance imaging (MRI) methods have produced many of the key insights, and are the central source of evidence discussed in this chapter. Broadly, MRI methods can be broken down into structural and functional approaches. Structural MRI methods are used to characterize the static anatomical and structural properties of an individual’s brain, and include morphometric methods that detail the specific qualities (e.g., thickness, volume) of the gray matter that comprises the brain’s outer cortex and major subcortical nuclei, and diffusion-weighted imaging methods that can characterize the cabling patterns formed by the brain’s white matter pathways. Meanwhile, functional MRI methods, including task-based functional MRI (fMRI) and intrinsic connectivity approaches such as resting-state fMRI (rsfMRI), allow us to observe dynamic temporal variation in the activation of individual brain regions, and in the coactivation of regions cooperating as part of interconnected brain networks. The evidence from these MRI-based approaches can be considered alongside data derived from complementary methods that render a sharper view of the temporal structure and dynamics of key neural events, especially electroencephalography (EEG).
Combining evidence obtained from these different modalities allows us to consider where and how the findings coalesce, and to evaluate the extent to which various perspectives on the relationship between brain development and digital media behaviors are supported. We focus on three emergent perspectives on the developmental factors that drive, and may be influenced by, digital media habits, and attempt to link these perspectives to evidence on the specific brain networks implicated in these facets of development. One perspective derives from theoretical and empirical work suggesting that the differences in the ability to exert self-regulatory control might account for variation in digital media involvement. Broadly, the idea is that those who struggle to control their thoughts, actions, and the orientation of their attention may be more prone to form digital media habits and be more vulnerable to any impacts of the behavior (Brand et al., Reference Brand, Young and Laier2014; Wei et al., Reference Wei, Zhang, Turel, Bechara and He2017; Wilmer et al., Reference Wilmer, Sherman and Chein2017). As we will detail in the next section of the chapter, there are specific brain regions thought to support the capacity for self-regulatory control, and evidence suggesting that the structure and function of these regions might be relevant in the development of digital media habits. A second perspective on digital media involvement focuses on variation across age groups and individuals in the valuation of, and responsiveness to, environmental rewards. Under this view, normative developmental shifts in reward-relevant processes may introduce periods of particular susceptibility to the appetitive, novel, and arousing properties of digital media interactions, especially for those who possess (or who come to develop) a particularly acute sensitivity to those rewards (Firth et al., Reference Firth, Torous and Stubbs2019). Once again, this perspective orients us to the specific brain regions we know to be involved in the coding of reward value and in processing the outcomes of pursuing (or not pursuing) environmental rewards. A third perspective arises from evidence highlighting conspicuous developmental shifts in the importance of nonfamilial social relationships, and associated changes in orientation and response to social influence (Blakemore, Reference Blakemore2018; Mills et al., Reference Mills, Lalonde, Clasen, Giedd and Blakemore2014; Sutter et al., Reference Sutter, Zoller and Glätzle-Rützler2019). Since digital media, and especially social media, have become such an important source of socially relevant information, some argue that developmental changes in the structure and function of the “social brain” might be especially important for understanding what motivates digital media behaviors, and how they might impact subsequent brain development (Meshi et al., Reference Meshi, Turel and Henley2020).
Importantly, the brain systems that support control, reward, and social information processes are thought to follow distinct trajectories of development. Whereas the mechanisms involved in self-regulatory control mature in a gradual and protracted manner across the period from middle childhood into early adulthood, the brain regions that subserve valuation of, and sensitivity to, rewards are thought to undergo more rapid reconfiguration during early adolescence, in response to the hormonal changes of puberty (Sisk & Zehr, Reference Shulman, Smith and Silva2005; Smith et al., Reference Smith, Chein and Steinberg2013; Spear, Reference Spear2010). This asynchronous developmental timing has important implications for how these systems interact with one another, and may be fundamental to understanding their roles in relation to emerging digital media behaviors. Meanwhile, regions associated with social information processing evince mixed developmental timing patterns (Atzil et al., Reference Atzil, Gao, Fradkin and Barrett2018; Kilford et al., Reference Kilford, Garrett and Blakemore2016; Mills et al., Reference Mills, Siegmund and Tamnes2021; Richardson et al., Reference Richardson, Lisandrelli, Riobueno-Naylor and Saxe2018), with some areas showing marked change in periods of childhood and adolescence, and others showing a more protracted developmental trajectory resembling that of self-regulatory control regions.
While this is a quickly advancing area of scientific inquiry, and the tools of modern neuroimaging have afforded valuable insights into the structure and function of the developing human brain, the reader should be warned from the outset that conclusive answers to the types of questions we posed in the opening paragraph of this chapter are still on the horizon. Rather, perspectives on how digital media experiences might interact with brain development currently derive from only a sparse corpus of fundamentally limited research. Perhaps the most obvious limitation is that very few studies are able to address the directionality of observed relationships. This is because, at present, the vast majority of relevant data originates from purely cross-sectional or correlational work, and very few true experiments or longitudinal studies exist to more adequately clarify causal patterns. It is worth noting, however, that the patterns of association and group differences observed in correlational and cross-sectional studies do help to guide alternative causal hypotheses, and the absence of predicted patterns can serve as key counterevidence against causal claims. Another clear limitation of the literature is that surprisingly few neuroinvestigative studies explore the brain correlates of digital media habits as they arise during the course of development. Rather, most of the evidence derives from studies of brain–behavior relationships observed at a relatively late point in development (in late adolescent and young adult cohorts). This state of affairs is due, in part, to the fact that many digital media behaviors (e.g., smartphone and social media account ownership, online gaming) often only begin to take hold in middle to later adolescence (Lauricella et al., Reference Lauricella, Cingel, Beaudoin-Ryan, Robb, Saphir and Wartella2016), and also to the inherent challenges involved in collecting neuroimaging data from younger participants (e.g., excessive movement and difficulty with task compliance). In our consideration of the brain systems implicated in digital media habits, we therefore rely primarily on the findings from later developmental periods, with the hope that this work contains reliable clues to how digital media experiences might interact with earlier brain development. Finally, as is discussed elsewhere in this handbook, the ever-changing technological landscape makes it difficult to conduct studies on digital media and the brain in a way that sufficiently addresses developmental cohort effects (i.e., the specific digital milieu available to a given developing cohort) and that anticipates consequential changes in the character and function of the digital media environment (e.g., the introduction of new social media forms). As such, much of what we can conclude to date is based on untested assumptions about the stability of observed brain–behavior relationships across varying digital media modalities and ecosystems.
With these caveats in mind, we can consider what the brain science tells us about the brain–behavior relations that surround digital media use. In the sections that follow, we consider, in turn, whether the brain regions and networks implicated in control, reward, and social processing are specifically relevant to digital media experiences. For each perspective, we weigh whether the extant neuroinvestigative evidence is corroborative or not, and consider how the specific pattern of evidence might sharpen or refine current explanatory theories. Rather than attempt an exhaustive review, we walk the reader through some seminal and informative findings, focusing first on studies from mostly nondevelopmental samples of adults, and then visiting the sparse but instructive patterns that have emerged in the developmental neuroscientific literature.
Digital Media and the Brain’s Control and Attention Networks
A growing body of work points to associations between digital media behaviors and the capacity for top-down self-regulatory control over thoughts, emotions, and behavior. Behavioral scientists often subdivide this skillset into separate psychological constructs with different labels (e.g., executive functioning, response inhibition, working memory, attention control, emotion regulation), and use a varied array of tasks and surveys to index its subcomponents. The general finding from across behavioral studies is that groups (and individuals) who demonstrate a weaker capacity for control also tend to exhibit higher levels of digital media use and more problematic involvements (e.g., excessive or addiction-like1 use) with various media forms. This is presumably because the inability to reliably exert control makes one more prone to impulsive engagement with digital media (e.g., frequent phone checking), greater attentional distractibility in response to media-associated cues (e.g., notifications), and greater difficulty with sustaining goal-relevant behaviors in the presence of digital media (Ward et al., Reference Ward, Duke, Gneezy and Bos2017). In our own lab, we have found that poorer performance on self-report and behavioral measures of response and impulse control is associated with increased smartphone and social media use habits among young adults (Wilmer & Chein, Reference Wilmer and Chein2016) and that early signs of this relationship are already present in much earlier stages of development (i.e., in a cohort of 6- to 8-year-olds, unpublished data). Several other studies detail similar relationships between poorer cognitive and attentional control and varying forms of digital media involvement, including greater social media use (Alloway & Alloway, Reference Alloway and Alloway2012), internet dependency (Choi et al., Reference Choi, Park and Roh2014; Dong et al., Reference Dong, Zhou and Zhao2011), increased media-multitasking (the concurrent use of alternate digital media modalities; Baumgartner et al., Reference Baumgartner, Weeda, van der Heijden and Huizinga2014; Lopez et al., Reference Lopez, Heatherton and Wagner2020; Minear et al., Reference Minear, Brasher, McCurdy, Lewis and Younggren2013; E. Ophir et al., Reference Ophir, Nass and Wagner2009), and excessive smartphone habits (Liebherr et al., Reference Liebherr, Schubert, Antons, Montag and Brand2020), at various points in development.
Given the apparent links between the behavioral expression of self-regulatory control processes and a range of digital media behaviors, an obvious place to begin looking for brain–behavior relationships tied to digital media use is within the brain regions and networks thought to support control processes. Considerations of where “control” arises in the brain often emphasize the lateral prefrontal cortex, but a more extensive characterization of how control is enacted might consider three complementary brain networks (Cole & Schneider, Reference Cole and Schneider2007; Dixon et al., Reference Dixon, De La Vega and Mills2018; Dosenbach et al., Reference Dosenbach, Fair, Cohen, Schlaggar and Petersen2008; Gratton et al., Reference Gratton, Sun and Petersen2018). The most prominent of these networks, the fronto-parietal “executive” network (FP; see Figure 5.1), is comprised of the dorsolateral prefrontal cortex (dlPFC, found in the middle frontal gyrus), the posterior parietal cortex (PPC, spanning the supramarginal gyrus and neighboring cortex extending into the intraparietal sulcus), and a dorsomedial prefrontal (dmPFC) region covering the dorsal extent of the anterior cingulate cortex (dACC) and extending into the midline superior frontal gyrus. While the FP network is thought to orchestrate the initiation and adjustment of control, the cingulate component of this network, along with a mid-anterior cingulate (mACC) area found slightly more rostral (in front of) and inferior to (below) the dACC, also functions as a hub region that dynamically coordinates its activity with the bilateral operculum (including the anterior insula and the neighboring posterior segment of the inferior frontal gyrus) to form a cingulo-opercular network (CO; see Figure 5.1). The CO network is thought to drive sustained control over behavior, and to orchestrate reactions to salient (goal-relevant or attention-grabbing) external and interoceptive (coming from inside the body) events, thus giving the network its alternative name – the “salience” network (Menon, Reference Menon and Toga2015). The ability to intentionally orient attention toward specific external and internal (mental) events is also known to involve an additional attention control network that has been dubbed the dorsal attentional network (also shown in Figure 5.1), which includes superior portions of the bilateral parietal association cortex (superior parietal lobule) as well as the bilateral frontal eye fields (found where the middle frontal gyrus intersects with the precentral gyrus). The brain regions encompassed in these control networks are generally understood to undergo a gradual, and particularly protracted, period of maturation that extends from childhood into at least the mid-twenties, which may explain why the ability to exert self-regulatory control over arousing and distracting stimuli is not fully formed until young adulthood (Sherman et al., Reference Sherman, Payton, Hernandez, Greenfield and Dapretto2016).
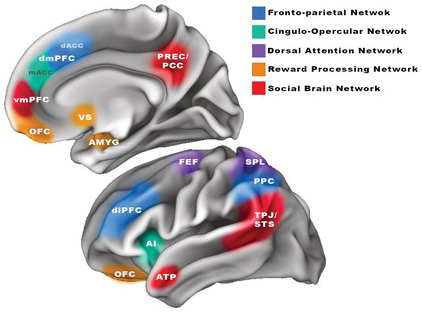
Figure 5.1 Visualization of regions comprising the brain networks thought to be associated with digital media behaviors. Key control regions are shown for the fronto-parietal “executive” network, the cingulo-opercular control network, and the dorsal attentional network, including the frontal eye fields (FEF) and superior parietal lobule (SPL). Also shown are regions strongly implicated in reward processing and those thought to be connected to social processing in the brain.
These control and attentional networks in the brain may be important to the manifestation of digital media behaviors. Some research implicating the neural correlates of control in digital media habits relies on basic structural MRI measurements of regional gray matter volume/density in the brain. Various studies on excessive internet use and online gaming behaviors, for example, offer evidence of reduced gray matter in key regions of the FP and CO networks, including the lateral prefrontal cortex (Q. He et al., Reference He, Turel, Wei and Bechara2020; Yuan et al., Reference Yuan, Qin and Wang2011), the dACC (X. Lin et al., Reference Li, Li and Yang2015; Yuan et al., Reference Yuan, Qin and Wang2011), and the insula (Turel et al., Reference Turel, He, Wei and Bechara2020); though studies in these populations occasionally show the opposing pattern of relationship (cf. Li et al., Reference Li, Li and Yang2015). Decreased gray matter volume has also been observed in the lateral prefrontal cortex, dACC, and anterior insula of individuals with smartphone “addiction” (Horvath et al., Reference Horvath, Mundinger and Schmitgen2020; Y. Wang et al., Reference Wang, Zou, Song and Xu2016), and in the dACC of individuals exhibiting a strong tendency to engage in media-multitasking (Loh & Kanai, Reference Loh and Kanai2014). While some investigators interpret these associations as evidence of consequential long-term impacts of digital media habits on the structural maturation of the brain’s control centers, such causal conclusions are simply untenable on the basis of this correlational evidence (e.g., we cannot know whether different habits lead to differentiated brain maturation, or whether different brains lead to differentiated habits). Moreover, it is challenging to translate evidence of altered structure into functional terms. For instance, while reduced gray matter volume does at times coincide with disrupted functioning, decreases in gray matter over the course of adolescent development are also thought to reflect the normative and desirable removal of unneeded neuronal connections through synaptic pruning (Gogtay & Thompson, Reference Gogtay and Thompson2010).
To try to clarify the nature of the relationship, we can consider evidence from studies of brain activity and connectivity, many of which highlight the same control-relevant brain regions. One early study on the functional correlates of internet gaming (Sun et al., Reference Sun, Ying and Seetohul2012) found that visual cues designed to elicit cravings among heavy video-gamers induced activation of the bilateral dlPFC and dACC. Subsequent work found that heavy media-multitasking was linked to both poorer performance and relatively increased right lateralized PFC activity when attempting an attentionally demanding task, which suggested that media-multitaskers might experience more difficulties when recruiting cognitive control resources (Moisala et al., Reference Moisala, Salmela and Hietajärvi2016). More recently, similarly aberrant lateral and dorso-medial PFC activation was reported in association with excessive smartphone usage (Schmitgen et al., Reference Schmitgen, Horvath and Mundinger2020), with smartphone addicts exhibiting increased activity in these areas when viewing smartphone-relevant visual cues (perhaps indicative of a need for greater effort in order to inhibit cue-related responses). Studies examining functional connectivity within and between the brain’s control networks provide further clarification of the relationship between control and digital media behaviors. The same group of smartphone addicts studied in Schmitgen et al. (Reference Schmitgen, Horvath and Mundinger2020) also evinced weaker coordination between the dmPFC and the left PPC, and between the anterior insula and the right lateralized PPC (Horvath et al., Reference Horvath, Mundinger and Schmitgen2020). Other recent work in heavy and excessive smartphone users has likewise indicated weaker intra-network connectivity in the FP and CO networks (Chun et al., Reference Chun, Park and Kim2020), and decreased functional (Chun et al., Reference Chun, Park and Kim2020) and structural (Wilmer et al., Reference Wilmer, Hampton, Olino, Olson and Chein2019) connectivity between key centers of the brain’s control networks and the ventral striatum (VS), a region of the brain where mesolimbic dopamine is released to signal the value of potential rewards. Thus, studies of brain activity and connectivity suggest that individuals who are more enmeshed with digital media also have a harder time (or need to devote more effort) initiating and sustaining self-regulatory processes, and may not be as facile at controlling responses to appetitive and potentially rewarding cues.
Evidence from EEG studies conducted on groups of heavy and addicted digital media users provides further support for the involvement of self-regulatory control mechanisms in these behaviors. Early work demonstrated that deficient executive abilities (assessed behaviorally) found in heavy internet users were paralleled by differences in the evoked potentials produced during a “Go/NoGo” response inhibition task (Dong et al., Reference Dong, Zhou and Zhao2011). The specific pattern exhibited by the heavy-use group reflected a relatively lower amplitude N2 (a frontally generated electrical potential that the authors associated with the conflict monitoring process that triggers the need to engage control), followed by a higher amplitude and delayed latency P3 (an evoked potential often associated with attention and response control). The authors interpreted these findings as evidence that the addicted group was less efficient at engaging control mechanisms during the task. Some subsequent work on internet use has replicated the reduced N2 potential during inhibitory control (Chen et al., Reference Chen, Liang, Mai, Zhong and Qu2016), while recent studies in excessive social media network users (Q. Gao et al., Reference Gao, Jia, Zhao and Zhang2019) and problematic smartphone users (L. Gao et al., Reference Gao, Zhang, Xie, Nie, Zhao and Zhou2020) point to a reversed pattern in which the more digitally engaged groups were found to evince a higher amplitude N2, and weaker P3 (specifically in the smartphone group) when trying to inhibit impulsive responses. While it can be challenging, even for experts in the field, to interpret the meaning of these differentiated components in the electrophysiological record, they do provide yet another source of evidence connecting digital media habits with the mechanisms underlying control.
So, though far from conclusive and based exclusively on correlational observations, the evidence seems to be broadly consistent with the notion that relative weaknesses in the brain systems supporting control may act as a gateway to digital media habit formation, and that diminished control could be a downstream consequence of prolonged or intensive periods of digital media involvement. However, some variation in the particular sites that emerge as significant across studies, and the occasionally reversed directionality of the findings (e.g., increases vs. decreases in regional volume, activity, connectivity, or evoked potentials), certainly warrant further consideration. One plausible explanation is that this variability is the result of unique brain-behavior relationships that exist for the diverse digital media experiences covered in this work. While the findings most consistently implicate key anterior and frontal nodes of the FP and CO networks, there is also considerably less evidence pointing to the involvement of parietal subregions of the FP and DAT networks (cf. Kei et al., Reference Kei, Naoya and Sayaka2020). This might indicate that digital media use is more closely tied to frontally mediated aspects of control – such as the establishment and maintenance of goal-state representations, and less connected to the parietal processes that dictate the shifting and orientation of attention (Chein & Schneider, Reference Chein and Schneider2005).
Digital Media and the Brain’s Reward Circuitry
A somewhat different perspective stems from the belief that digital media habits are connected to approach motivational and reinforcement processes. Under this view, the appetitive and rewarding features of digital media technologies – often embedded intentionally into digital platforms by their developers in order to stimulate more intense usage habits (Harris, Reference Harris2016) – might drive increased engagement with these platforms, disrupt the normal development of reward circuits, and at the extremes, give rise to maladaptive and addiction-like behaviors. Indeed, much of the work on digital media use draws upon the language and theories of addiction and reward dysregulation, and such diagnostic labels as internet-use disorder, internet addiction, internet gaming disorder, social network use disorder, and smartphone addiction are commonly applied in the literature to groups and individuals who exhibit seemingly excessive, problematic, or dependent use habits (Griffiths et al., Reference Griffiths, Kuss, Demetrovics, Rosenberg and Curtiss Feder2014; Gutiérrez et al., Reference Gutiérrez, de Fonseca and Rubio2016; Kirby et al., Reference Kirby, Dapore, Ash, Malley and West2020; Yao et al., Reference Yao, Liu and Ma2017).
As with claims regarding control, theories linking aberrant reward processing and responsivity to digital media habits also gain some purchase in correlational behavioral evidence. Across several studies exploring the behavioral and trait correlates of different digital media modalities, there is compelling evidence that individuals who tend to be more engaged with these media also tend to exhibit greater general reward sensitivity and responsivity (Sanbonmatsu et al., Reference Sanbonmatsu, Strayer, Medeiros-Ward and Watson2013) – particularly with respect to more immediate rewards (Hadar et al., Reference Hadar, Hadas, Lazarovits, Alyagon, Eliraz and Zangen2017; Tang et al., Reference Tang, Zhang, Yan and Qu2017; Wilmer & Chein, Reference Wilmer and Chein2016), and have greater difficulty with reward reinforcement learning (Meshi et al., Reference Meshi, Elizarova, Bender and Verdejo-Garcia2019).
If the development of reward-relevant processes plays a role in the formation of digital media habits, or results in reward dysregulation that causes greater vulnerability to relevant problematic outcomes, then we might expect to observe such effects within the brain’s reward circuitry (see Figure 5.1). This circuitry includes the dopaminergic pathways that connect the ventral tegmental area of the brainstem (where dopamine is produced) to the nucleus accumbens of the VS, the amygdala, and the ventromedial prefrontal cortex (vmPFC) – especially its ventral-most extent comprising the orbitofrontal cortex (OFC), a region linked to aberrant reward processes in patients with other substance-related and behavioral addictions (Kuss et al., Reference Kuss, Pontes and Griffiths2018). From a developmental perspective, these reward-processing structures are known to undergo a rapid period of change around the onset of puberty, which is thought to explain why adolescence constitutes a period of particularly heightened reward responsivity (Blakemore & Robbins, Reference Blakemore and Robbins2012; Sisk & Zehr, Reference Shulman, Smith and Silva2005; Spear, Reference Spear2010).
Neuroimaging work suggests that the dysregulation of reward-relevant regions, especially the OFC, VS, and amygdala, may indeed be a hallmark for the addiction-like behaviors found in association with a range of digital media forms (Kuss et al., Reference Kuss, Pontes and Griffiths2018; Lin & Lei, Reference Lin, Lei, Montag and Reuter2015; Turel et al., Reference Turel, He, Xue, Xiao and Bechara2014). A study on addicted players of the online video game World of Warcraft (Ko et al., Reference Ko, Liu and Hsiao2009) was among the first to show this relationship. Specifically, the study found that excessive gamers, relative to a comparison group of game novices, evinced increased activity not only in self-regulatory processing regions (dlPFC, dmPFC), but also in the OFC and VS, when presented with game-related cues that aroused the urge to play. Several subsequent studies examining brain structure in participants engaged in especially high levels of internet and smartphone use have found corroborating evidence of gray matter abnormalities in the OFC (Hong et al., Reference Hong, Kim and Choi2013; Lee et al., Reference Lee, Namkoong, Lee, Lee and Jung2019; Lin & Lei, Reference Lin, Lei, Montag and Reuter2015; Zhou et al., Reference Zhou, Montag and Sariyska2019).
Studies examining the number of social relationships that one forms by way of online social networking sites also point to the relevance of reward processing centers in the brain. Building on prior work examining the neural correlates of both online and offline social network size (Bickart et al., Reference Bickart, Wright, Dautoff, Dickerson and Barrett2011; Kanai et al., Reference Kanai, Bahrami, Roylance and Rees2012), Von der Heide and colleagues demonstrated that having a larger online social network, as measured by participants’ actual number of Facebook friends, was associated with greater gray matter volume in multiple reward-relevant brain regions, including the bilateral amygdala and OFC (Von der Heide et al., Reference Von der Heide, Vyas and Olson2013). A related study on the structural brain correlates of actual Facebook use – this time indexed by participants’ mobile device Facebook use over a five-week period (Montag et al., Reference Montag, Markowetz and Blaszkiewicz2017) – found that higher frequency and duration of mobile Facebook use were both associated with decreased gray matter volume of the bilateral VS. Other related work has found evidence of decreased gray matter volume in the bilateral amygdalae of those reporting generally heavier use of social networking sites (i.e., not focused on a particular platform; W. He et al., Reference He, Turel and Bechara2017). As we noted earlier, relative increases and decreases in the volume of regional gray matter can be difficult to interpret in functional terms, but such findings, at the very least, suggest that there are relevant linkages between digital media habits and the processes enacted within these reward-processing centers.
Here again, we can turn to fMRI studies involving task-based manipulations of the digital media environment to corroborate and clarify the structural findings. In one early neuroimaging study on social media behavior (Turel et al., Reference Turel, He, Xue, Xiao and Bechara2014), heavy Facebook users were scanned while performing a task that required them to respond to Facebook-relevant cues (iconography taken from the Facebook platform) while withholding responses to irrelevant cues (traffic signs), or vice versa. Among the regions tested, only one exhibited a pattern of activity that predicted individual differences in Facebook addiction – the VS. That is, the level of one’s Facebook addiction selectively related to how strongly this central reward value processing center responded in association with Facebook images. The importance of the VS in social media behaviors was similarly underscored in another early study of Facebook users in which social feedback given to participants was experimentally manipulated in a simulated social media environment (Meshi et al., Reference Meshi, Morawetz and Heekeren2013). In this study, the authors found that actual Facebook usage was associated with how active the VS became when participants received positive social feedback for themselves (compared to others) in the simulated platform. Another widely cited neuroimaging study deployed a simulated version of the Instagram social networking platform (Sherman et al., Reference Sherman, Payton, Hernandez, Greenfield and Dapretto2016). In the study, high school students submitted photos from their own actual Instagram accounts and were told that their photos, along with photos provided by others, would be viewed by the participants in the study, and either liked or not liked; in reality, the number of likes and the content (neutral or risky behaviors) of the photos were manipulated by the researchers as part of their experimental design. FMRI evidence showed increased activation in the VS, along with several other regions, when participants saw that their own images had received a higher number of likes, and also when viewing neutral photos that were more liked by others. These findings suggest that receiving positive social feedback via social media, and evaluating the relative social value of the information (e.g., photos) others post on these platforms, engages the same brain processes that generally signal rewarding experiences. A related study conducted in adolescents (Cascio et al., Reference Cascio, O’Donnell, Bayer, Tinney and Falk2015) investigated whether these same processes might influence online decisions about whether or not to conform with others’ expressed preferences. During a scanning session, subjects were shown the recommendations (ratings) that they and others had given for a set of smartphone apps, and were then given the chance to revise their own prior rating. Analyses indicated greater activation in both the VS and OFC when participants changed, rather than maintained, their initial rating, which suggests once again that reward valuation signals play a role in dictating this facet of online behavior. Indeed, two recent companion studies exploring the neural processes underlying the selection and sharing of digital media content likewise implicate this same reward valuation network (Baek et al., Reference Baek, Scholz, O’Donnell and Falk2017; Scholz et al., Reference Scholz, Baek, O’Donnell, Kim, Cappella and Falk2017). Specifically, these studies found that the VS and OFC were among the most strongly engaged regions when participants opted to share news headlines via social media in a simulated task, and in association with headlines that are actually the most “viral” (i.e., shared in real-life media) at the population level.
Functional and structural connectivity approaches provide still further evidence of reward circuitry involvement in mediating the nature and intensity of one’s digital media habits, though the directionality of these findings is somewhat nuanced. While some studies suggest that heavier digital media involvement is tied to disrupted (weaker) integration among the brain regions that process reward-relevant information (e.g., functional connectivity with the VS is reduced in internet addicts; Zhang et al., Reference Melchers, Li, Chen, Zhang and Montag2015), other studies find that heavier digital media use is associated with stronger interconnectivity among reward regions (e.g., the integrity of white matter pathways connecting the VS and OFC is stronger in heavy smartphone users [Wilmer et al., Reference Wilmer, Hampton, Olino, Olson and Chein2019]; functional connectivity of the amygdala to other regions is a correlate of adolescent smartphone dependence [Tymofiyeva et al., Reference Tymofiyeva, Yuan and Kidambi2020]).
EEG studies examining online gaming and smartphone addiction also lend support to the idea that heavy digital media use is associated with altered neural activity in the reward system. Relevant studies on internet behavior have found, for instance, that online gaming addicts produce an attenuated P300 in response to receiving rewards (Duven et al., Reference Duven, Müller, Beutel and Wölfling2015), and that individuals who report excessive internet use evince both a smaller feedback-related negativity in response to reward gains, and a larger P300 in response to losses (W. He et al., Reference He, Turel and Bechara2017), which could indicate stronger reinforcement sensitivity and weaker punishment sensitivity, respectively. Recent work on smartphone habits similarly observed an altered reward positivity potential among heavier smartphone users, but no association between intensity of use and the amplitude of the parietal P3 (which the authors considered an index of higher-level decision processing), leading to the conclusion that smartphone addiction may be selectively correlated with reward processing, and not higher-level deliberative processes (Kirby et al., Reference Kirby, Dapore, Ash, Malley and West2020).
Overall, the behavioral and imaging findings connect reward-related brain systems to a range of digital media behaviors. This work spans early forms of digital media, including video gaming and internet use habits, but also harnesses one of digital media’s currently most widespread and time-consuming manifestations – social media networking. While some studies find that digital media behaviors are selectively associated with reward-related signals and locations in the brain (e.g., Kirby et al., Reference Kirby, Dapore, Ash, Malley and West2020), many of the relevant studies also contain evidence for the involvement of regions thought to undergird other, more disparate, functions (e.g., Ko et al., Reference Ko, Liu and Hsiao2009; Sherman et al., Reference Sherman, Payton, Hernandez, Greenfield and Dapretto2016). Indeed, as we consider in greater depth in the next section, several studies highlighting the relevance of reward circuitry in digital media habits (e.g., Cascio et al., Reference Cascio, O’Donnell, Bayer, Tinney and Falk2015; Horvath et al., Reference Horvath, Mundinger and Schmitgen2020; Sherman et al., Reference Sherman, Payton, Hernandez, Greenfield and Dapretto2016) also indicate the relevance of regions more typically associated with social information processes (rather than reward processes, per se).
Digital Media and the Brain’s Social Processing Systems
Increasingly, many of our day-to-day social interactions take place on digital platforms, and it has been argued that social networking sites now serve as an independent medium for developing and maintaining social connectedness despite being devoid of direct face-to-face interactions (Grieve et al., Reference Grieve, Indian, Witteveen, Anne Tolan and Marrington2013; Spies Shapiro & Margolin, Reference Spies Shapiro and Margolin2014). As such, researchers have leveraged the quantification of social interactions supported by online social networking sites like Facebook and Instagram to explore whether, and how, this type of digital social context relates to psychological and brain functioning.
The strength and directionality of the influence of digital screen engagement and social media networking on psychosocial functioning in developmental populations is a subject of significant debate (Coyne et al., Reference Coyne, Rogers, Zurcher, Stockdale and Booth2020; Przybylski et al., Reference Przybylski, Orben and Weinstein2020; Twenge et al., Reference Twenge, Haidt, Joiner and Campbell2020), and evidence from both longitudinal and large-scale secondary data analysis suggests that the relationship is likely smaller and more nuanced than has sometimes been claimed (Coyne et al., Reference Coyne, Rogers, Zurcher, Stockdale and Booth2020; Przybylski et al., Reference Przybylski, Orben and Weinstein2020). Any impact of social media behaviors is also likely to vary across different social networking platforms. For example, some studies in adolescent and young adult samples find that heavier use of Facebook, but not YouTube or Twitter, is related to higher self-reported levels of social connectedness (Alloway et al., Reference Alloway, Horton, Alloway and Dawson2013; Alloway & Alloway, Reference Alloway and Alloway2012), potentially due to the built-in features in Facebook that facilitate more sharing of personal content.
The expectation that aspects of digital media involvement could be motivated by, or have an impact on, social exchange, has led some investigators to pursue evidence of associations between the brain’s social information processing networks (see Figure 5.1) and digital media habits. There is, however, only partial consensus regarding which specific brain regions participate selectively in social information processes. The brain regions most consistently implicated in social information processing are the temporoparietal junction and neighboring (posterior) superior temporal sulcus along with the midline (ventromedial) prefrontal cortex. Some treatments of the “social brain” also variably include the precuneus and adjacent posterior cingulate cortex, and the anterior temporal poles (Adolphs, Reference Adolphs2009; Becht et al., Reference Becht, Wierenga and Mills2021; Mills et al., Reference Mills, Lalonde, Clasen, Giedd and Blakemore2014). Together, these regions are thought to support empathy, mentalizing, social perspective taking, and the processing of social feedback. The conspicuous proximity of the medial prefrontal areas implicated in social feedback processing and those associated with the valuation of primary rewards, such as food and sex (Bartra et al., Reference Bartra, McGuire and Kable2013; Lieberman & Eisenberger, Reference Lieberman and Eisenberger2009) has led some researchers to emphasize the functional overlap between these systems (Bhanji & Delgado, Reference Bhanji and Delgado2014; Braams et al., Reference Braams, Peters, Peper, Güroğlu and Crone2014), as have studies showing engagement of mesolimbic “reward” regions in putatively “social” tasks. Likewise, colocalization of regions implicated in social information processing tasks with areas that also exhibit increased engagement when our minds are supposed to be at rest – comprising a so-called default mode network – has spurred additional theorizing on the specific operations that are supported by these brain areas (Mars et al., Reference Mars, Neubert, Noonan, Sallet, Toni and Rushworth2012).
Work exploring the links between the magnitude of one’s online social network size and brain structure points not only to the involvement of nodes in the brain’s reward system, as was noted earlier, but also to the involvement of social processing areas such as the temporoparietal junction (Kanai et al., Reference Kanai, Bahrami, Roylance and Rees2012). The involvement of the social brain in digital media experiences is further suggested by work examining the association between intrinsic functional organization in the brain and individual differences in the sharing of personal (self-related) information on Facebook (Meshi et al., Reference Meshi, Mamerow, Kirilina, Morawetz, Margulies and Heekeren2016). Specifically, analyses focused on how social processing centers in the vmPFC and precuneus connect up with the rest of the brain. The study revealed that the strength of connectivity between these regions and the lateral PFC predicted a greater tendency to share information with others on Facebook, while stronger connectivity between the precuneus and ATP predicted less sharing. Such findings suggest that the decision to broadcast personally relevant information via social media may depend in part on how one mentally represents social relationships (interactions between the self and others) in these regions.
Other recent work has explicitly investigated whether the structure of social brain regions might also explain the overall amount of time one spends on social media (Turel et al., Reference Turel, He, Brevers and Bechara2018). Based on behavioral findings establishing that the effort to maintain and navigate online social relationships is subjectively perceived as demanding (Turel et al., Reference Turel, He, Brevers and Bechara2018, Exp. 1), the authors wondered whether the social skills used to keep up with these demands might also be reflected in the neuroanatomical correlates of social information processing. Seeming to confirming this hypothesis, structural MRI analyses revealed a significant positive correlation between overall Facebook usage and the gray matter volume of a superior temporal site near the temporoparietal junction (Turel et al., Reference Turel, He, Brevers and Bechara2018, Exp. 2). That is, those with more gray matter in a temporal region of the social brain network reported spending more time on Facebook, which the authors thought could reflect the relative sophistication of the social skills that they rely on to maintain extended online social networks, or their relative adeptness at deploying these skills.
The idea that possessing stronger social skills might promote greater digital media involvement is, however, in an interesting juxtaposition with findings suggesting that individuals with lower social empathy also tend to be more enmeshed with certain digital media (Decety & Lamm, Reference Decety and Lamm2006; Engelberg & Sjöberg, Reference Engelberg and Sjöberg2004; Melchers et al., Reference Melchers, Li, Chen, Zhang and Montag2015). As such, while heavier media use might coincide with a stronger ability to understand others’ perspectives (i.e., advanced social processing skills), it may also coincide with less actual concern for others’ emotional states. Evidence from EEG studies aimed at investigating the neural basis of empathic processing among individuals who report heavy internet use reinforces this speculation. Specifically, multiple studies have found that participants with high internet addiction scores (compared to healthy controls) exhibit an undifferentiated electrical response when viewing images of others in painful versus nonpainful circumstances, whereas the EEG record in healthy controls shows discrimination of these conditions (Jiao et al., Reference Jiao, Wang, Peng and Cui2017; T. Wang et al., Reference Wang, Ge, Zhang, Liu and Luo2014). That is, internet-addicted individuals exhibit (at least in their EEGs) a relative absence of empathy for others’ discomfort.
The evidence considered above demonstrates that some digital media experiences are associated with brain regions and patterns implicated in social information processing. Namely, they show that key nodes in the social brain, including the temporoparietal junction, the precuneus, and the vmPFC, are likely to play a role in determining how digital media users navigate through the complexities of online social networking space and how they form representations of others’ perspectives and feelings. We note, however, that the supportive findings in this domain may be less abundant in the literature than those implicating self-regulatory control and reward-relevant processes. This state of affairs could indicate the differential contributions of these systems to digital media behaviors, or could simply reflect the fact that few studies have thus far deliberately tried to disentangle the social facets of digital media interactions from its inherent rewards and high-level processing demands. We anticipate that this will be a focal aim of future work in this space.
Digital Media Use in the Developing Brain
Above, we harnessed evidence from the young adult literature in order to establish the involvement of control, reward, and social brain systems in digital media experiences. Guided by this evidence, and with some knowledge of the expected trajectory of development within these systems, we turn now to a limited body of developmental neuroscientific work that might help us to understand how these brain–behavior relationships manifest in earlier stages of development: to discern whether the patterns observed in young adulthood are already present in earlier life, whether brain–behavior relationships emerge specifically in conjunction with the maturation of the three systems (or with other brain systems), or whether the patterns discussed above are only characteristic of later stages of development.
Studies in Early Life and Childhood
While early childhood screen and digital media exposure is a widely researched topic, only a handful of studies have deployed noninvasive brain imaging methods in the effort to illuminate potential interrelationships with brain development. Perhaps the earliest developmental glimpse comes from MRI and EEG studies conducted in preschoolers (Hutton et al., Reference Hutton, Dudley, Horowitz-Kraus, Dewitt and Holland2020; Zivan et al., Reference Zivan, Bar, Jing, Hutton, Farah and Horowitz-Kraus2019). One MRI-based diffusion tractography study (Hutton et al., Reference Hutton, Dudley, Horowitz-Kraus, Dewitt and Holland2020) found that, already by preschool (ages 3–5), screen time exposure is associated with widespread reductions in white matter integrity, a sign that these children have less well-developed structural connections between brain regions. While several tracts (pathways) exhibited this association, those associated with executive function, multimodal association, visual processing, and language were especially implicated. The same research group also conducted an EEG study of children aged 4–6 (Zivan et al., Reference Zivan, Bar, Jing, Hutton, Farah and Horowitz-Kraus2019), which found that six weeks of exposure to screen-based, digitally recorded, stories, compared to live human storytelling, resulted in weaker attentional gains and a resting-state EEG pattern characteristic of attentional disruption (increased theta/beta power ratio). Meanwhile, a study exploring screen-based media habits in a group of older children (aged 8–12; Horowitz-Kraus & Hutton, Reference Horowitz-Kraus and Hutton2018) indicated that increased media exposure might be associated with decreased resting-state connectivity between both cognitive control and language regions of the brain and the visual word form area, a region known to be important in the acquisition and execution of reading skills. The authors speculated that this pattern might have arisen because substantial screen time disrupts the normal development of the regions that support reading skill in the brain. Recently, Horowitz-Kraus and colleagues (Reference Horowitz-Kraus, DiFrancesco and Greenwood2020) followed up on this discovery to investigate whether functional connectivity patterns in this age range might also relate to the ratio of time that children spend in front of screens versus reading; this time considering these relationships for both typical readers and children with reading difficulties. The two reading ability groups exhibited similar screen-to-reading time ratios but, selectively for the children with reading difficulties, a relatively greater proportion of screen time activity was related to increased functional connectivity in the salience and executive control networks. The authors suggested that this pattern might reflect inefficient engagement of control processes when reading (and presumably when engaging in other cognitively challenging tasks), which might ultimately lead these children to greater screen dependency (though see Y. Ophir et al., Reference Ophir, Tikochinski and Rosenberg2020). Through we still only have correlational evidence from these studies, the findings are at least consistent with the idea that screen time exposure, particularly during earlier stages of dynamic brain growth and development, might be intertwined with the processes supporting self-regulatory control, especially among those with existing developmental deficits.
Paulus and colleagues (Reference Paulus, Squeglia and Bagot2019) recently reported findings from the first large-scale investigation aimed at relating screen media activity to structural brain characteristics in prepubescent youth (ages 9 and 10 at recruitment), using the structural imaging and survey data from a 4,277-participant subset of the first cross-sectional release of the Adolescent Brain Cognitive Development (ABCD) study (Volkow et al., Reference Volkow, Koob and Croyle2018). The authors characterized and quantified screen media activity via multivariate analyses of survey responses provided by parents and youth. Overall, these analyses produced significant but complex patterns of relationship between structural brain indices (cortical thickness, sulcal depth, and gray matter volume) and screen media activity. In particular, the factor accounting for the most variance in screen media activity showed that greater screen involvement was linked to widespread cortical thinning and gray matter volume reductions (along with greater levels of externalizing psychopathology and lower crystallized intelligence). Interestingly, this pattern held for regions supporting both early sensory processing and higher order functions. However, the specific pattern of relationship was also found to depend on the type of screen media behavior (e.g., social media vs. gaming) – for instance, greater exposure to gaming-related activities was associated with thinner cortex, but also larger regional volume (e.g., OFC) and higher crystallized intelligence. Moreover, other latent factors capturing variance in the screen media activity data suggested disparate patterns of relationship between screen activity and brain structure. In light of this diversity of findings, the authors cautioned that screen media activity cannot be reduced to being simply “good” or “bad” for brain structure and function.
Studies in Adolescents
There is a widely observed rise in digital media involvement during adolescence. With adolescence, pubertal processes advance the brain into a period characterized by rapid change in both the midline dopaminergic reward system and in the extended network of brain regions involved in social information processing (Blakemore, Reference Blakemore2008, Reference Blakemore2012). It is, accordingly, tempting to speculate that the changes taking place in these brain systems might explain the escalation of digital media use during this period, and earlier in this chapter we presented some relevant and corroborative findings (e.g., Cascio et al., Reference Cascio, O’Donnell, Bayer, Tinney and Falk2015; Sherman et al., Reference Sherman, Payton, Hernandez, Greenfield and Dapretto2016; Tymofiyeva et al., Reference Tymofiyeva, Yuan and Kidambi2020; Yuan et al., Reference Yuan, Qin and Wang2011). Unfortunately, much of the additional literature on brain-to-digital-media relationships during “adolescence” has involved either very late adolescent cohorts, or participant samples spanning a wide age range that may include some younger adolescents but also extends into young adulthood (i.e., participants in their early to mid- twenties; F. Lin et al., Reference Lin, Zhou and Du2012; Moisala et al., Reference Moisala, Salmela and Hietajärvi2016, Reference Moisala, Salmela and Hietajärvi2017; Von der Heide et al., Reference Von der Heide, Vyas and Olson2013; H. Wang et al., Reference Wang, Jin and Yuan2015). Thus, it can be difficult to draw meaningful developmental conclusions from this corpus of work.
The few studies using somewhat more constrained age cohorts (i.e., including only adolescents aged 18 or under) produce intriguing, but varied outcomes. One study, for instance, observed that internet gaming habits among a group of 14- to 17-year-olds related to disrupted blood flow patterns (as measured by MRI-based arterial spin labeling) in a large number of brain areas, including some linked to reward-relevant processing (e.g., amygdala) (Feng et al., Reference Feng, Chen and Sun2013). Using functional connectivity methods, another study found that a group of gaming addicts, aged 12–17, also evince relatively increased connectivity between the posterior cingulate cortex and several other social- and reward-relevant regions, including the precuneus and the nucleus accumbens (Ding et al., Reference Ding, Sun and Sun2013). However, more recent work (Chun et al., Reference Chun, Choi and Cho2018) on excessive smartphone use among adolescents aged 12–18 found that smartphone usage intensity related to significantly weaker intrinsic resting-state connectivity within the reward network (OFC to VS) and between the control and reward systems (OFC to mACC), with weakened OFC–VS functional connectivity also found to be predictive of the severity of smartphone withdrawal symptoms reported by the group. Thus, evidence on the relationship between digital media behaviors and functional connectivity across regions of the reward and social processing networks appears to be nuanced, and difficult to align neatly with specific theories of development.
Age Group Comparisons and Longitudinal Studies of Youth
Cross-sectional evidence comparing digital media use among different age cohorts could help us to determine whether observed brain–behavior relationships simply track with the trajectory of normative brain development, or rather, contain evidence for a causative effect of digital media behavior on brain development. Unfortunately, the literature is almost completely lacking studies that directly compare one age group to another. The only notable exception is Sherman et al.’s (Reference Sherman, Greenfield, Hernandez and Dapretto2018) replication and extension of their earlier work in adolescents (Sherman et al., Reference Sherman, Payton, Hernandez, Greenfield and Dapretto2016), in which they leveraged the same simulated Instagram paradigm to collect comparison data from an older young adult cohort comprised of university students (Sherman et al., Reference Sherman, Greenfield, Hernandez and Dapretto2018). Similar to their prior findings in adolescents, the young adults evinced greater activation in social- and reward-relevant brain regions, including the precuneus, vmPFC, and VS, when viewing images from their own Instagram accounts that had received more versus fewer likes. Indeed, the direct contrast between adolescents’ and young adults’ brain activity when these groups received social feedback on their own images produced no significant differences, other than a small region of the visual cortex. This congruency across the two age cohorts suggests that adolescents’ elevated concerns toward “popularity” likely persist into young adulthood. That is, the sensitivity of the brain’s social and reward circuitry might rise with adolescence, but then plateau in young adulthood. When viewing others’ images, however, important age differences did emerge in control-relevant regions of the brain. Namely, while the adolescent sample had exhibited diminished engagement of control regions when viewing risky compared to non-risky/neutral images, young adults showed equivalent activity in the two conditions. In other words, the young adults responded to the images of risky activities by activating the self-regulatory control regions that inhibit actual involvement in such behaviors, while the adolescents did not appear to do so. Indeed, a direct contrast across the two age groups indicated significantly greater activation for young adults in both the dmPFC and dlPFC when viewing risky images. Together, these findings accord with a dual systems framework (Shulman et al., 2016; Steinberg, Reference Steinberg2008), wherein the sensitivity of reward circuitry levels off as the brain’s control and attention systems reach young adult maturity, and show that changes in these interacting systems likely hold relevance for developing digital media habits.
Longitudinal examinations of brain structure and function spanning different stages of development could be especially fertile territory for furthering our understanding of the origins and effects of digital media use. Though relevant longitudinal studies are currently underway (e.g., ABCD; Volkow et al., Reference Volkow, Koob and Croyle2018), the findings available to date generally come from relatively short-term longitudinal investigations that are not specifically informative with respect to development. In one study, for instance, a six-week internet gaming exposure enacted with naïve and experienced young adult gamers resulted in short-term longitudinal reductions in left OFC volume (Zhou et al., Reference Zhou, Montag and Sariyska2019), which could be interpreted as evidence that video game play affects one of the important centers for reward processing. Another short-term intervention study found that when internet-naïve adults were given four weeks of increased internet access, they started to exhibit higher rates of media-multitasking, but there were no significant changes detected in brain structure (Loh et al., Reference Loh, Chakraborty and Sadhu2019). A more extended longitudinal undertaking involved a three-year study conducted in a large sample of Japanese children and adolescents (aged 5–18) aimed at exploring how various digital media behaviors (TV viewing, video gaming, internet use) might prospectively impact brain development (Takeuchi et al., Reference Takeuchi, Taki and Hashizume2015, Reference Takeuchi, Taki and Hashizume2016, Reference Takeuchi, Taki and Asano2018). While the basic prospective longitudinal approach represents the type of method that could inform our understanding of digital media’s causal impacts on brain development, the outcomes are quite challenging to put into a coherent narrative. Notably, the work assessed digital media behaviors only at the start of the study, with no follow-up assessment of how habits may have changed over the longitudinal period. There was also no consistency or specificity in the findings with respect to the particular brain areas whose longitudinal change was predicted by baseline digital media habits, and disparate MRI modalities (gray/white matter volume, mean diffusivity of diffusion MRI) were needed to obtain significant brain–behavior relationships across media types. Most important, there was no reported attempt to delineate specific developmental patterns, despite the longitudinal nature of the data and the wide age range of the participants at entry to the study. Finally, as was alluded to by the authors themselves, the cohort project began in 2008, which predates the widespread availability and popularity of smartphones, social media, and online games in Japan. This observation underscores how work of this nature may be subject to cohort effects introduced by the ever-changing technology climate.
Conclusions: What We Know Now and Where We May Be Headed
Alongside rapid advancements in digital technology, recent years have witnessed a growing body of work dedicated to understanding the potential impact of digital media behaviors on psychological and brain functions. In this chapter, we reviewed a growing literature deploying various MR imaging and complementary electrophysiological methods that might inform our understanding of the links between brain development and digital media behaviors. Broadly, we sought to examine whether the data accord with current perspectives on digital media involvement that emphasize maturing self-regulatory control skills, a heightened sensitivity to rewards, and shifts in responsivity to socially relevant inputs. Acknowledging important limitations in the available developmental evidence, we first considered how well these perspectives address the body of data obtained primarily from young adult populations, and then surveyed the findings from earlier life for evidence that might provide traction in clarifying the developmental origins of observed brain–behavior relationships.
Overall, there is corroborative evidence denoting each of the three highlighted systems (control, reward, social). That is, for each perspective, there appear to be an ample number of supportive findings from across different types of digital media (e.g., internet behaviors, smartphone use, social media involvement, media-multitasking, etc.) and from multiple neuroinvestigative modalities (various MRI-based approaches, EEG). There are, likewise, some examples from research conducted in younger developmental samples pointing to digital media interactions with some of the same neural substrates of control, reward, and social processes that are featured in the young adult literature.
However, we also come across findings that compel more nuanced accounting of the relationships between digital media involvement and brain development. First, across studies, modalities, and age groups, even the most affirming observations – that is, those implicating expected neural correlates of control, reward, or social processing – place differential emphasis on separate regions/subcomponents within a given brain system, and moreover, at times appear to indicate opposing directional patterns (e.g., increases vs. decreases in regional volume/activity/connectivity, positive vs. negative correlations with digital media involvement, stronger vs. weaker engagement across development). These differences may just be the consequence of noisy measurement approaches (e.g., in the characterization of digital media behaviors or the indexing of brain structure/function), but could also reflect actual, and potentially meaningful, differences in the brain–behavior relationships that exist for certain digital media experiences and particular populations. The outcomes may depend, for example, on whether one is examining the addiction-like or excessive digital media behaviors that are emphasized in the disease-oriented approach that dominates much of the field, or whether one is examining more normative day-to-day patterns of engagement with digital media technologies.
We should also be mindful of some specific limitations in how we have approached this review. First, while we present the findings as though each of the three emphasized systems (control, reward, social) can be considered independently, this assumption is plainly fraught, not only because there is imperfect agreement about which specific regions contribute to each system as well as some neuroanatomical overlap between them (e.g., medial PFC, parietal cortex), but more importantly, because the real story of digital media’s relationship with brain development almost certainly lies in the complex and dynamic interactions that take place between these systems, and in how these interactions shift over the course of development. Second, this approach to review reflects a form of confirmation bias. That is, with the expectation that the brain areas associated with control, reward, or social information processing might be relevant to the link between brain development and digital media behaviors, we sought out examples in the literature that could affirm this expectation, while being less attentive to evidence that could potentially lead us toward a different, perhaps overlooked, explanation. By way of example, though we proffered the work conducted by Horvath et al. (Reference Horvath, Mundinger and Schmitgen2020), Sherman et al. (Reference Sherman, Payton, Hernandez, Greenfield and Dapretto2016), and Turel et al. (Reference Turel, He, Brevers and Bechara2018) as examples implicating control, reward, and social mechanisms, respectively, each of these studies also reported significant findings in the medial temporal lobe (the hippocampus or neighboring cortices), which might encourage us to consider the relevance of episodic memory mechanisms enacted within the medial temporal lobe in the relationship between digital media habits and brain development. Likewise, our review could have devoted greater attention to emerging evidence of digital-media-dependent effects on primary visual and somatosensory cortices, and the possibility that daily intensive digital media use is leading to the plastic reshaping of these cortical areas (Gindrat et al., Reference Gindrat, Chytiris, Balerna, Rouiller and Ghosh2015).
Despite the negative attitudes toward digital media involvement often emphasized in public outlets (Bennett, Reference Bennett2017; Parks, Reference Parks2020), the causal impacts of digital media habits on the developing brain remain unclear, due in part to the relative absence of longitudinal work and largely correlational nature of cross-sectional studies, and to the challenges that naturally arise with neuroscientific work conducted with younger populations. Emerging technologies beyond fMRI and EEG could be helpful in circumventing some of these practical limitations. For instance, functional near-infrared spectroscopy, a wearable and relatively low-cost tool that is used across a wide range of populations from preterm infants to the elderly (Pinti et al., Reference Pinti, Tachtsidis and Hamilton2020; Rahimpour et al., Reference Rahimpour, Noubari and Kazemian2018) could be fruitfully applied to examine the brain correlates of digital media habits as they arise in real-world settings. Meanwhile, noninvasive brain stimulation methods could help us to close the causal chain by revealing how experimentally induced alteration of brain states affects digital media behaviors. Findings demonstrative of behavioral change following brain stimulation in other relevant contexts, such as inhibitory control (Cai et al., Reference Cai, Li and Liu2016; Stramaccia et al., Reference Stramaccia, Penolazzi, Sartori, Braga, Mondini and Galfano2015) and risk taking (Figner et al., Reference Figner, Mackinlay, Wilkening and Weber2009; Gilmore et al., Reference Gilmore, Dickmann, Nelson, Lamberty and Lim2018), suggest that it may even be possible to use brain stimulation technologies to alter the course of digital media habit formation or to ameliorate impacts on other behaviors (Hadar et al., Reference Hadar, Hadas, Lazarovits, Alyagon, Eliraz and Zangen2017).
So, where does this leave us? To put it plainly, despite a now sizable literature on associations between the brain and digital media behavior, it is clear that there is much still to be learned. Within an ever-changing media technology landscape, it has proven challenging to address the essential questions that motivate work in the field. Are there specific brain markers present during the course of development that can reliably predict subsequent digital media habits, or that might signal greater susceptibility to any harmful outcomes of these habits? Is brain development influenced in any particularly meaningful way by earlier, or more extended, exposure to digital media technologies? As much as we would like to forward conclusive answers to these questions, the only answer we can justifiably offer as a field is that we do not yet know. But, armed with the many valuable insights provided by the extant literature, and with clarifying evidence that will most certainly emerge through longitudinal and convergent methodology studies on the near horizon, we are optimistic that the field will continue to narrow the gaps in our understanding, and bring us closer to more edifying answers.