Functioning impairments and risk for psychosis and depression
Loss of functioning is linked to reduced quality of life, and they combine to negatively influence the disease course of many psychiatric conditions, especially psychosis and major depression.Reference Bora, Harrison, Yucel and Pantelis1 Early functional deficits are already present in clinical high-risk states (CHRs).Reference Ruhrmann, Paruch, Bechdolf, Pukrop, Wagner and Berning2,Reference Schultze-Lutter, Michel, Ruhrmann and Schimmelmann3 More specifically, deficits in role functioning (educational and occupational) within the CHR period are particularly relevant because they frequently develop in CHRs irrespective of transition to psychosis, and lead to problems associated with inability to attend school, unemployment, social impairments and lasting financial consequences.Reference Harvey and Strassnig4,Reference Yung, Yuen, McGorry, Phillips, Kelly and Dell'Olio5 Notably, although outcomes in social functioning could recently be promisingly predicted by machine learning modelsReference Dwyer, Falkai and Koutsouleris6 constructed on clinical and structural magnetic resonance imaging (sMRI) baseline data in up to 83% of patients in CHRs,Reference Koutsouleris, Kambeitz-Ilankovic, Ruhrmann, Rosen, Ruef and Dwyer7 outcomes related to role functioning could not accurately be determined, thus calling for a broader investigation of potential predictors. Evidence shows that social and role functioning may be fundamentally different phenomena, differentially linked to symptoms,Reference Burton, Tso, Carrion, Niendam, Adelsheim and Auther8 neurocognitive deficitsReference Carrion, Goldberg, McLaughlin, Auther, Correll and Cornblatt9 and adverse outcomes.Reference Carrion, Auther, McLaughlin, Addington, Bearden and Cadenhead10,Reference Velthorst, Zinberg, Addington, Cadenhead, Cannon and Carrion11 More specifically, recent views posited that role functioning may be more strongly associated with concurrent environmental factors, compared with social functioning.Reference Cornblatt, Carrion, Addington, Seidman, Walker and Cannon12,Reference Evert, Harvey, Trauer and Herrman13 This would be coherent with the notion that environmental adverse events during maturational/developmental periods (e.g. trauma experiences, repeated negative social interactions, maladjustments in developmental goals) are central for psychosis,Reference Howes and Murray14 depressionReference Kwong, Lopez-Lopez, Hammerton, Manley, Timpson and Leckie15 and bipolar disorderReference Schmitt, Malchow, Hasan and Falkai16 pathophysiology. Moreover, such adverse events have been associated with brain structure and function alterations.Reference Baker, Williams, Korgaonkar, Cohen, Heaps and Paul17–Reference Popovic, Ruef, Dwyer, Antonucci, Eder and Sanfelici19
Notably, impairments in role functioning also concern individuals in early illness stages outside the psychosis risk spectrum, such as depression.Reference Schultze-Lutter, Schimmelmann and Michel20 This is particularly relevant since the CHR may evolve in different psychiatric disorders.Reference Fusar-Poli, Nelson, Valmaggia, Yung and McGuire21 Indeed, 35–68% of patients in CHRs develop or maintain non-psychotic disorders,Reference Schultze-Lutter, Schimmelmann and Michel20,Reference Fusar-Poli, Nelson, Valmaggia, Yung and McGuire21 thus calling for research on CHRs to broaden the scope of risk estimation to detect not only psychosis, but also other adverse outcomes. Consistently, patients in CHRs often experience affective symptoms, to the extent that 41% of have a depressive disorder.Reference Fusar-Poli, Nelson, Valmaggia, Yung and McGuire21,Reference Fusar-Poli, Salazar de Pablo, Correll, Meyer-Lindenberg, Millan and Borgwardt22 Furthermore, studies showed that psychosis risk is detectable also in affective conditions beyond the traditional ‘at-risk’ construct.Reference Fusar-Poli, Tantardini, De Simone, Ramella-Cravaro, Oliver and Kingdon23,Reference Lee, Lee, Kim, Choe and Kwon24 These findings highlight that multiple conditions are associated with role impairments since their early stages. Thus, the prediction of such functional outcomes should be targeting both CHRs and affective samples, to obtain more realistic, reliable and potentially transdiagnostic prediction models of future risk for further functional impairments and, ultimately, disability, in a more heterogeneous help-seeking population.Reference Koutsouleris, Kambeitz-Ilankovic, Ruhrmann, Rosen, Ruef and Dwyer7,Reference Koutsouleris, Worthington, Dwyer, Kambeitz-Ilankovic, Sanfelici and Fusar-Poli25,Reference Rosen, Betz, Schultze-Lutter, Chisholm, Haidl and Kambeitz-Ilankovic26
Employing machine learning to identify reliable functioning predictors
So far, research on early identification of patients who subsequently develop psychosis or other adverse outcomes has produced favourable results, yet further improvement is required. Few studiesReference Antonucci, Pergola, Pigoni, Dwyer, Kambeitz-Ilankovic and Penzel27,Reference Cannon, Yu, Addington, Bearden, Cadenhead and Cornblatt28 have tested the predictive value of environmental adverse events on functional impairments across concurrent psychiatric conditions, as frequently present in psychosis risk syndromes. In this context, machine learning could harness the interacting effects of different risk factors by building prognostic models using multiple data domains, rather than using only one domain at a time. This form of multimodal learning has been shown to improve prognostic/predictive performance in various fields of medicine, such as affective disorders,Reference Chekroud, Zotti, Shehzad, Gueorguieva, Johnson and Trivedi29,Reference Kessler, van Loo, Wardenaar, Bossarte, Brenner and Cai30 Alzheimer's diseaseReference Grassi, Perna, Caldirola, Schruers, Duara and Loewenstein31 and stroke.Reference Feng, Badgeley, Mocco and Oermann32 Also, in the CHR field, multimodal risk calculators outperformed unimodal prediction models.Reference Cannon, Yu, Addington, Bearden, Cadenhead and Cornblatt28,Reference Bodatsch, Ruhrmann, Wagner, Muller, Schultze-Lutter and Frommann33–Reference Koutsouleris, Upthegrove and Wood35 Notably, these multimodal risk calculators also showed generalisability,Reference Koutsouleris, Kambeitz-Ilankovic, Ruhrmann, Rosen, Ruef and Dwyer7,Reference Koutsouleris, Kahn, Chekroud, Leucht, Falkai and Wobrock36 even when applied to outcome prediction.Reference Kambeitz-Ilankovic, Meisenzahl, Cabral, von Saldern, Kambeitz and Falkai37 Thus, embracing a multimodal predictive approach could facilitate the identification and characterisation of people at risk for adverse functioning outcomes, which might, in turn, lead to differential managements that are tailored on a patient's individual needs and impairments,Reference Lin, Wood, Nelson, Beavan, McGorry and Yung38 irrespective of a later transition to psychosis.Reference Lin, Wood, Nelson, Beavan, McGorry and Yung38 Parallel to the development of generalisable predictive models, a deeper investigation into the prognostic power of single data domains and how each domain individually influences the final prediction is also of central importance.Reference Dwyer, Falkai and Koutsouleris6
Study aim
The aim of this study was therefore to expand existing role functioning prediction models,Reference Koutsouleris, Kambeitz-Ilankovic, Ruhrmann, Rosen, Ruef and Dwyer7 which operated on clinical and sMRI data, by adding information regarding environmental factors previously associated with psychosisReference Loewy, Corey, Amirfathi, Dabit, Fulford and Pearson39–Reference Upthegrove42 and depression.Reference Hill, Mellick, Temple and Sharp43–Reference Vocisano, Klein, Keefe, Dienst and Kincaid46 We analysed two populations with overlapping courses of functional impairments (i.e. CHR and recent-onset depression (ROD)), drawn from the database of the Personalized Prognostic Tools for Early Psychosis Management study (PRONIA; https://www.pronia.eu/). We hypothesised that, by adding environmental information to the clinical and sMRI data domains, follow-up role functioning impairments could be more accurately predicted in CHR and ROD samples separately, as well as transdiagnostically. As a first step, we investigated the predictive power of environmental factors occurring before baseline, alone and in combination with clinical (i.e. retrospectively collected scores of social and role functioning) and sMRI data. Then, we investigated the models’ transdiagnostic potential and generalisability to unseen individuals. We evaluated the predictive importance of each environmental variable in the respective predictive models, and then investigated whether the best multimodal predictive model generalised to the prediction of other clinically relevant trajectories. As a final step, we employed multivariate regression techniques to assess whether the environmentally determined predictions of role functioning were associated with the clinical and sMRI data domains, and could therefore act via clinical vulnerability or sMRI abnormalities.
Method
Sample determination
Individuals were recruited within the European Union's Seventh Framework Programme project PRONIA.Reference Koutsouleris, Kambeitz-Ilankovic, Ruhrmann, Rosen, Ruef and Dwyer7 The cohort is divided based on the date of recruitment in CHR (n = 92) and ROD (n = 95) discovery samples (i.e. individuals recruited between February 2014 and May 2017, at seven sites; Table 1), for model generation, and in CHR (n = 74) and ROD (n = 66) replication samples, for generalisability assessments (i.e. individuals recruited after May 2017 and July 2019 at the same seven discovery sites, plus three new sites; Supplementary Appendix 1 and Supplementary Table 1 available at https://doi.org/10.1192/bjp.2022.16). Individuals meeting the criteria for CHR or ROD were recruited according to internationally established diagnostic criteria;Reference Koutsouleris, Kambeitz-Ilankovic, Ruhrmann, Rosen, Ruef and Dwyer7 20% of CHR and 16% of ROD individuals were recruited at the three new replication sites.
Table 1 Study-associated, sociodemographic, physical, clinical, functional and environmental differences at baseline in discovery individuals with clinical high-risk states, and in individuals with recent-onset depression, with lower versus higher Global Functioning: Role scale outcomes at follow-up
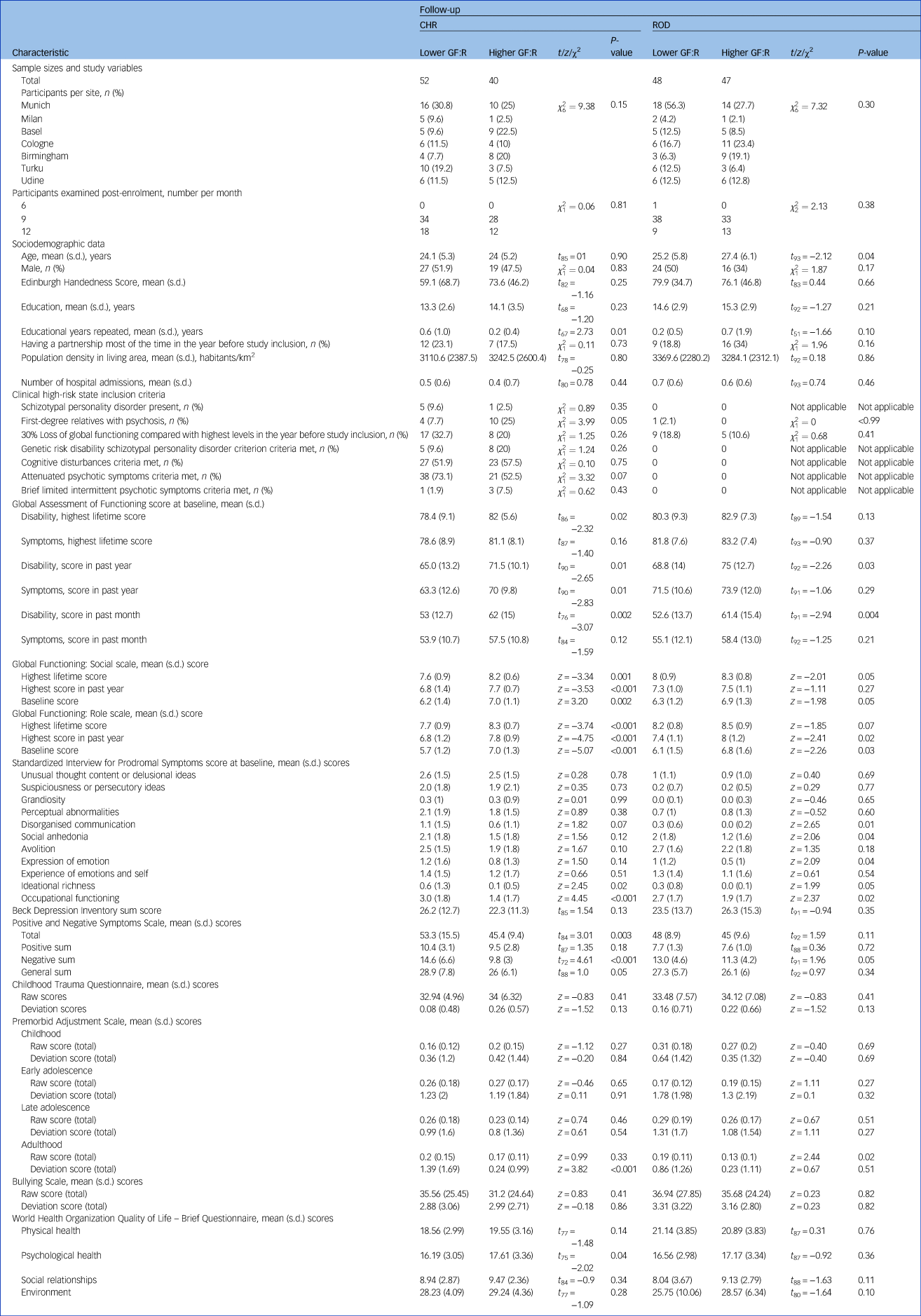
Significance was defined at α = 0.05. CHR, clinical high-risk; ROD, recent-onset depression; GF:R, Global Functioning: Role scale.
For all individuals, baseline sMRI and environmental information, as well as baseline and follow-up social and role functioning scores between the 6- and 12-month timepoints of the study (clinical data), were available. Written informed consent was obtained from all participants. The Global Functioning: Role (GF:R) scaleReference Cornblatt, Auther, Niendam, Smith, Zinberg and Bearden47 was used to define lower versus higher role functioning at a literature-based threshold,Reference Koutsouleris, Kambeitz-Ilankovic, Ruhrmann, Rosen, Ruef and Dwyer7 using the participants’ latest examination within the 6- to 12-month follow-up period. Based on the literature,Reference Cornblatt, Auther, Niendam, Smith, Zinberg and Bearden47 a score of >7 points indicated higher outcome and a score of ≤7 points indicated lower outcome, as a score of 7 points marks initial mild, but already persistent, role functioning impairment.
Two-sample t-tests, z-tests and chi-squared tests were used to investigate potential across-sites demographic and clinical baseline differences in CHR and ROD. Furthermore, we investigated the prevalence comparisons of DSM-IV-TR diagnoses in CHR and ROD with lower versus higher role functioning at baseline (T0) and follow-up examinations 9 months after baseline (T1), through chi-squared tests. P-values were group- and timepoint-wise false discovery rate (FDR)-corrected (α = 0.05).
Unimodal classifiers
The combined clinical and sMRI role functioning prediction models reported in the previous study from our group,Reference Koutsouleris, Kambeitz-Ilankovic, Ruhrmann, Rosen, Ruef and Dwyer7 which we aimed to expand by adding environmental information, informed the present analysis with respect to the choice of predictors and the machine learning pipelines for sMRI and clinical classifiers, which were not altered in any part. The environmental classifier was not informed from the previous study,Reference Koutsouleris, Kambeitz-Ilankovic, Ruhrmann, Rosen, Ruef and Dwyer7 except for the machine learning pipeline, which was implemented consistently with the clinical and sMRI ones. However, it should be noted that our samples do not completely overlap with those used in that study, because 24 CHR and 25 ROD individuals in the discovery samples skipped the environmental assessment (see Supplementary Appendix 1, Section 1). Therefore, only individuals with environmental assessments (besides clinical and sMRI) were retained in the present study. Based on this rationale, we trained the following classifiers:
(a) A ‘clinical’ classifier based on eight baseline Global Functioning: Social (GF:S) and GF:R scores (i.e. highest social and role functioning lifetime score, highest and lowest social and role functioning scores in the past year, and current social and role functioning scores), based on the Global Functioning Scale.Reference Cornblatt, Auther, Niendam, Smith, Zinberg and Bearden47
(b) An ‘environmental’ classifier, using six summary scores derived from the Childhood Trauma Questionnaire (CTQReference Scher, Stein, Asmundson, McCreary and Forde45), the Bullying Scale for Adults,Reference Haidl, Schneider, Dickmann, Ruhrmann, Kaiser and Rosen48 and time-window scores (childhood, early adolescence, late adolescence and adulthood) of the Premorbid Adjustment Scale (PASReference Shapiro, Marenco, Spoor, Egan, Weinberger and Gold49), which measures the relative level of harmony between an individual's needs and environmental characteristics and requests.Reference Garcia, Al Nima and Kjell50 Notably, although the PAS does not strictly measure adverse events, its derived scores reflect how environmental challenges and risk factors may modulate the capacity of people to adjust in different periods of life.Reference Shapiro, Marenco, Spoor, Egan, Weinberger and Gold49 All summary scores entering the algorithm were derived by normalising total raw scores by using the published psychometric norms of each instrument (Supplementary Appendix 1, Section 2).
(c) An ‘sMRI’ classifier, including baseline whole-brain grey matter volume (GMV) individual data. We employed open-source CAT12 toolbox (version r1155 for Linux; Christian Gaser, University of Jena, Germany; see http://dbm.neuro.uni-jena.de/cat12/) to pre-process and analyse individual GMV maps. Detailed consortium-wise pre-processing and site correction procedures of MRI data is reported in Supplementary Appendix 1, Section 3 and elsewhere.Reference Koutsouleris, Kambeitz-Ilankovic, Ruhrmann, Rosen, Ruef and Dwyer7
Classifiers were based either on continuous variables (i.e. environment and sMRI) or ordinal variables (i.e. clinical), which were treated as continuous variables based on previous literature.Reference Rhemtulla, Brosseau-Liard and Savalei51 All predictor assessments were made without knowledge of outcome data, and the outcome label was determined without knowledge of predictor information. Two-sample t-tests and z-tests were used to investigate potential clinical and environmental differences between CHR and ROD with regards to lower versus higher role functioning (P < 0.05). Results for discovery cohorts are reported in Table 1, and results for the replication cohort are reported in Supplementary Table 1. For MRI, we ran checks to rule out any role functioning or site effects, as well as their interaction, on GMV estimates. The results of these checks highlighted the absence of any main effect of role functioning, site and their interaction, on GMV maps (all P > 0.05, family-wise error-corrected k = 10; Supplementary Appendix 1, Section 4).
Machine learning pipeline
The overall analytic strategy (Supplementary Fig. 1) was to first quantify the unimodal prognostic performance of each classifier (clinical, environmental, sMRI), and then to understand whether environmental information would improve prediction performance when combined with the clinical model and/or the sMRI model. Therefore, for each cohort (CHR and ROD), we built six machine learning models to predict higher versus lower GF:R outcome: three using unimodal classifiers and three using combinations of individual classifiers (multimodal classifiers). To facilitate comparability and interpretation of our findings, both for unimodal and multimodal classifiers, we chose to implement the same machine learning pipelines reported to generate the combined clinical and sMRI prediction models we aimed at expanding.Reference Koutsouleris, Kambeitz-Ilankovic, Ruhrmann, Rosen, Ruef and Dwyer7 With this aim, we implemented a mixed inner k-fold/outer leave-site-outReference Koutsouleris, Kambeitz-Ilankovic, Ruhrmann, Rosen, Ruef and Dwyer7 cross-validation strategy based on our machine learning platform NeuroMiner, version 1.0 for Linux (Nikolaos Koutsouleris, Munich, Germany; see https://github.com/neurominer-git). Per each population (CHR, ROD), we obtained unimodal risk calculator predictions based on environmental, clinical and sMRI baseline features. On the basis of these unimodal predictions, we built the three multimodal classifiers described above, using stacked generalisation (Supplementary Appendix 1, Section 5).Reference Wolpert52 We purposefully did not investigate the joint predictive ability of clinical and sMRI classifiers, as this was already explicitly addressed in a recent publication from our group.Reference Koutsouleris, Kambeitz-Ilankovic, Ruhrmann, Rosen, Ruef and Dwyer7 To measure the discriminative utility of the input variables within each unimodal classifier, we computed the probability of being selected for classification purposes within the inner cross-validation loop for each feature,Reference Antonucci, Pergola, Pigoni, Dwyer, Kambeitz-Ilankovic and Penzel27 following a forward feature selection procedure that occurred only in the inner cycle (CV1) of training data. Of note, this procedure was performed only for significant unimodal classifiers (see Results). A detailed description of our machine learning pipeline is provided in Supplementary Appendix 1, Sections 5 and 6. P-values reflecting the permuted significance of models (Supplementary Appendix 1, Section 6) are reported in Table 2. Permutation-based pairwise comparisons between discovery unimodal and multimodal classifier performance are reported in Supplementary Table 5. As a check, we have calculated the expected calibration error (ECE)Reference Koutsouleris, Worthington, Dwyer, Kambeitz-Ilankovic, Sanfelici and Fusar-Poli25,Reference Guo, Pleiss, Sun and Weinberger53 to estimate calibration for the models achieving best accuracy and generalisability in our discovery cohorts (see Results). The ECE was relatively low, although not perfect (mean ECE = 0.21). Methods and results of this check are fully described in Supplementary Appendix 1, Section 10 and Supplementary Fig. 2.
Table 2 Performance of all unimodal and multimodal classifiers tested in clinical high-risk, recent-onset depression and the pooled sample, for global functioning role outcome at follow-up

Results are reported for both discovery and replication cohorts. Model significances were assessed by computing BAC in 1000 random label permutations and comparing them with the observed BAC of the respective model. P-values were adjusted for multiple comparisons, using the false discovery rate (FDR). FDR-corrected significant models are reported in the significance column in bold. Significances could not be calculated for any replication analysis, as the out-of-sample validation mode of NeuroMiner, different from the discovery mode, does not allow us to calculate significance for models that are generated in one cohort and then applied to another sample. For these analyses, ‘not assessed’ is reported in the table. The range from minimum to maximum BAC across all Outer Cross-Validation (CV2) folds. FPR, false positive rate; PPV, positive predictive value; NPV, negative predictive value; AUC, area under the curve; BAC, balanced accuracy; CHR, clinical high-risk; sMRI, structural magnetic resonance imaging; ROD, recent-onset depression.
Assessment of generalisability
Transdiagnostic potential of risk calculators
To investigate whether the hypothesised unimodal and multimodal risk calculators that we computed separately for CHR and ROD could have transdiagnostic potential, we repeated the same pre-processing, training and testing pipeline (Supplementary Appendix 1, Sections 5 and 6) on the combined CHR-ROD population, comprising 187 individuals (92 CHR, 95 ROD).
Validation of risk calculators
To test for the generalisability of all prognostic models derived from CHR, ROD and the pooled CHR and ROD sample, we validated CHR discovery models in the CHR replication cohort (n = 74); ROD discovery models in the ROD replication cohort (n = 66); and transdiagnostic (CHR + ROD) discovery models in the pooled CHR and ROD replication sample (n = 140), without any re-training (Supplementary Appendix 1, Section 5).
Despite the employment of leave-site-out cross-validation, we used sanity checks to rule out whether the discovery and validation performance of our unimodal and multimodal classifiers could be affected by any latent site effects. Results of these checks are reported in Supplementary Appendix 1, Section 5.
Prognostic generalisation of risk calculators to clinical trajectories
To assess our role functioning predictor's generalisability to the development of other clinical outcomes over time, we used linear mixed effects models (see Results). We therefore generated trajectories based on three longitudinal timepoints for three clinical readouts (Supplementary Appendix 1, Section 7): number of psychiatric hospital admissions across timepoints; prodromal positive and negative symptoms, drawn from the Structured Interview for Psychosis-Risk Syndromes;Reference Miller, McGlashan, Rosen, Cadenhead, Cannon and Ventura54 and quality of life, drawn from the World Health Organization Quality of Life – Brief Questionnaire.Reference Skevington, Lotfy, O'Connell and Group55 For each clinical variable of interest, baseline, T1 (6–12 months after baseline) and T2 assessment (18 months after baseline) evaluations were entered into the analyses (Supplementary Appendix 1, Section 7). A multiple comparisons correction was carried out with FDR (α = 0.05).
Environmental feature knock-out analysis
To quantify the predictive contribution of each of the environmental variables included in the algorithm, we ran new GF:R outcome prediction models based on environmental features, but we removed each of the six features originally included in the individual classifier, one at a time, without altering the original machine learning pipeline employed for the environmental classifier. This led to six independent Support Vector Machine analyses for CHR and ROD, each comprising five features.
Investigation of between-classifiers relationships
To understand whether environmentally determined predictions could act via clinical vulnerability or sMRI abnormalities in increasing the risk for worse outcome in CHR and ROD – that is, to preliminarily investigate whether the predictive power of our environmental model on follow-up occupational functioning might be partially explained either by baseline global functioning impairments or baseline sMRI anomalies – we ran support vector regression analyses (Supplementary Appendix 1, Section 9).
Ethic statement
The authors assert that all procedures contributing to this work comply with the ethical standards of the relevant national and institutional committees on human experimentation and with the Helsinki Declaration of 1975, as revised in 2008. All procedures involving human patients were approved by the German Clinical Trials Register (DRKS00005042) and approved by the local research ethics committees in each centre.
Results
Demographic, clinical and environmental site-level characteristics are reported separately for CHR and ROD discovery patients in Table 1, and for CHR and ROD replication patients in Supplementary Table 1. Table 3 and Supplementary Table 2 report prevalence comparisons of DSM-IV-TR diagnoses in CHR and ROD samples with lower versus higher GF:R at baseline (T0) and T1 follow-up examinations.
Discovery results
CHR cohort
In the CHR group (Table 2, Fig. 1 and Supplementary Appendix 1, Section 4), only environmental and clinical risk calculators predicted GF:R outcomes significantly better than chance, according to leave-site-out cross-validated balanced accuracy (BACLSOCV) (environmental model: BACLSOCV = 66.4%, P FDR = 0.01; clinical model: BACLSOCV = 67.3%, P FDR = 0.04) and area under the curve (AUC) (environmental model: 0.63; clinical model: 0.76). The multimodal classifier integrating environmental, clinical and sMRI predictions was more accurate than all unimodal classifiers (BACLSOCV = 71.2%, P FDR = 0.01, AUC = 0.75), followed by the model integrating environmental and clinical predictions (BACLSOCV = 65.4%, P FDR = 0.04, AUC = 0.70). Permutation-based pairwise comparisons between discovery unimodal and multimodal classifier performances are reported in Supplementary Table 5. In the environmental domain, higher deviation scores for premorbid adjustment in adulthood, self-reported bullying victimisation and self-reported experiences of childhood trauma were predictive of poor role functioning outcomes (Fig. 2a). In the clinical domain, lower GF:R lifetime scores predicted poor role functioning outcomes (Fig. 2b).

Fig. 1 Plots representing the balanced accuracies (BACs) across models and samples. Dots indicate the mean BAC per model, and bars indicate the range (minimum to maximum) of the BACs per models across all of the cross-validation outer cycle folds. sMRI, structural MRI.
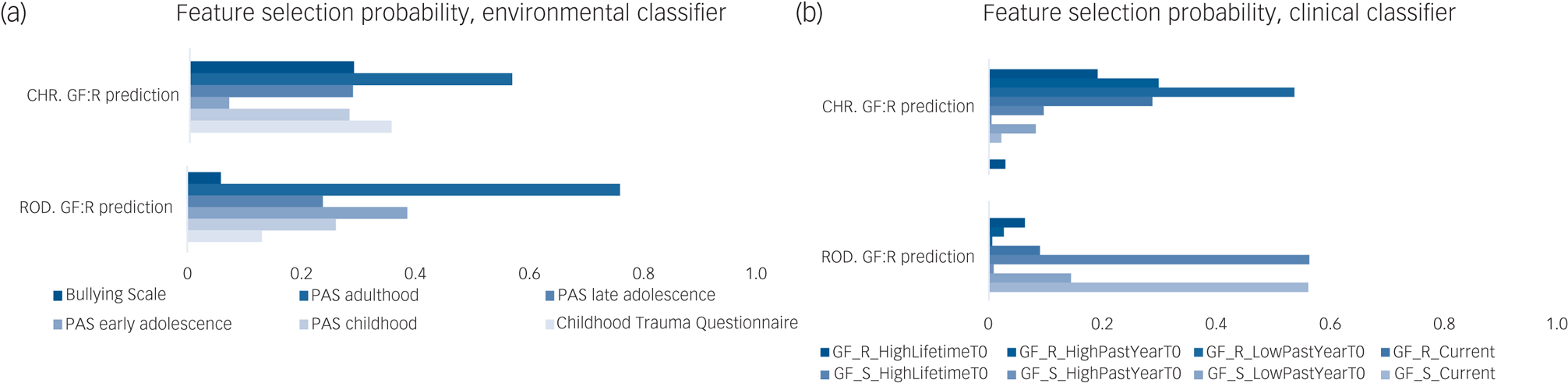
Fig. 2 Probability of each feature for being selected in our mixed k-fold/leave-site-out cross-validation framework by (a) the environmental classifier, (b) the clinical classifier and (c) the sMRI classifier. For (a) and (b), a value of 1 indicates that all models had retained the given variable (Supplementary Appendix 1, Section 5). Feature permutation testing results are reported in Supplementary Table 10. CHR, clinical high-risk; GF:R, Global Functioning: Role; GF:S, Global Functioning: Social; PAS, Premorbid Adjustment Scale; ROD, recent-onset depression; sMRI, structural magnetic resonance imaging; GF_R_HighLifetimeT0, GF:R highest lifetime score measured at T0; GF_R_HighPastYearT0, GF:R highest score in the last year before T0; GF_R_LowPastYearT0, GF:R lowest score in the last year before T0; GF_R_Current, GF:R score at T0 examination; GF_S_HighLifetimeT0 , GF:S highest lifetime score measured at T0; GF_S_HighPastYearT0, GF:S highest score in the last year before T0; GF_S_LowPastYearT0, GF:S lowest score in the last year before T0; GF_S_Current, GF:S score at T0 examination.
ROD cohort
In the ROD group (Table 2, Fig. 1 and Supplementary Appendix 1, Section 4), no unimodal risk calculator predicted a GF:R outcome above chance. Only the stacked model integrating environmental and clinical predictions provided significant prediction performance (BACLSOCV = 58.9%, P FDR = 0.04, AUC = 0.60). Lower GF:R outcomes were predicted by the PAS adulthood, PAS early adolescence and PAS childhood features of the environmental risk calculator (Fig. 2a), as well as by GF:R highest scores during the past year and GF:R highest lifetime scores in the clinical risk calculator (Fig. 2b). Permutation-based pairwise comparisons between discovery unimodal and multimodal classifier performances are reported in Supplementary Table 5.
Assessment of generalisability
Transdiagnostic potential of risk calculators
In the pooled CHR and ROD discovery sample (Table 2 and Fig. 1), the clinical risk calculator predicted a GF:R outcome above chance and with significance (BACLSOCV = 64.8%, P FDR = 0.02, AUC = 0.72). In the environmental domain, higher deviation scores for PAS adulthood and PAS late adolescence were predictive of poor role functioning outcomes. In the clinical domain, lowest current GF:R score and lowest GF:S score during the past year predicted poor role functioning outcome. The multimodal classifier combining clinical and environmental predictions, as well as the model integrating all unimodal models, performed significantly above chance (combined clinical + environmental model: BACLSOCV = 62.4%, P FDR = 0.03, AUC = 0.71; combined clinical + enviornmental + sMRI model: BACLSOCV = 61.1%, P FDR = 0.04, AUC = 0.67).
Validation of risk calculators
The study-group-specific clinical models, as well as multimodal risk calculators combining environmental and clinical data, performed above chance when applied to the respective CHR and ROD replication samples (CHR: BACLSOCV = 67.3% and AUC = 0.76 for the clinical model, BACLSOCV = 67.7% and AUC = 0.76 for the environmental plus clinical model; ROD: BACLSOCV = 70.5% and AUC = 0.81 for the clinical model, BACLSOCV = 62.5% and AUC = 0.72 for the environmental plus clinical model). Notably, the model integrating environmental, clinical and sMRI predictions, which achieved the best BACLSOCV in the CHR discovery sample, achieved much lower BAC (58.7%) when applied to the CHR replication sample, but the performance difference between CHR discovery and validation samples was not significant (P = 0.12; Supplementary Table 6). The transdiagnostic risk calculator built with the clinical data of the pooled CHR and ROD groups performed above chance in the pooled replication cohort (BACLSOCV = 69.7% and AUC = 0.74), in CHR alone (BACLSOCV = 61.1% and AUC = 0.75) and in ROD alone (BACLSOCV = 75% and AUC = 0.72) (Table 2 and Supplementary Table 4). Models combining clinical and environmental decision scores, and those combining clinical, environmental and sMRI decision scores, were those reaching the highest performance in all cohorts, and performed similarly (clinical + environmental model: BACLSOCV = 68.2% and AUC = 0.74 for CHR + ROD, BACLSOCV = 62.1% and AUC = 0.76 for CHR alone, and BACLSOCV = 71.5% and AUC = 0.73 for ROD alone; clinical + environmental + sMRI model: BACLSOCV = 69.6% and AUC = 0.75 for CHR + ROD, BACLSOCV = 67.4% and AUC = 0.77 for CHR alone, and BACLSOCV = 69.3% and AUC = 0.72 for ROD alone) (Table 2, Supplementary Tables 4 and 6).
Potential generalisation of risk calculators to relevant clinical trajectories
Linear mixed models results revealed that the prognostic role functioning assignments produced by the environmental plus clinical model stratified clinical trajectories of the ROD cohort, with respect to negative symptoms (P FDR = 0.02) and environmental quality of life (P FDR = 0.02; Fig. 3b). It did not stratify any clinical readout trajectory in the CHR group (all P FDR > 0.4; Fig. 3a).

Fig. 3 Results of the linear mixed effects models used to compare main and slope differences between trajectories of prognostic or observed assignments (lower versus higher) for role functioning in CHR (a) and ROD (b). Linear fits are depicted in each plot. Significant main effects are highlighted in bold. Leave-site-out BAC percentage of all environmental models generated in CHR (c) and ROD (d) through recursive elimination of one environmental variable at a time. BAC, balanced accuracy; CHR, clinical high-risk; FDR, false discovery rate; GF:R, Global Functioning: Role; PAS, Premorbid Adjustment Scale; ROD, recent-onset depression; SIPS, Structured Interview for Psychosis-Risk Syndromes; WHOQOL, World Health Organization Quality of Life – Brief Questionnaire.
Environmental feature knock-out analysis
In the CHR group, the removal of one environmental variable at a time did not produce models superior to the original environmental classifier (Fig. 3, Supplementary Appendix 1, Section 8 and Supplementary Table 7), whereas in ROD, the model without the PAS childhood variable was superior to the original one (BACLSOCV = 60.1%). All other models performed similarly to the original one (Fig. 3d, Supplementary Appendix 1, Section 8 and Supplementary Table 7).
Investigation of between-classifiers relationships
In the CHR group, the sMRI-based model significantly predicted environmental decision scores, explaining 6.56% of the observed variance (P FDR = 0.02; Supplementary Table 7). However, the clinical regression model could not predict environmental decision scores (explained variance 1.99%, P FDR = 0.24). In the ROD group, the model predicting environmental-related decision scores for GF:R according to clinical data was significant (P FDR = 0.01), and explained 9.18% of the observed variance (Supplementary Table 7). However, the sMRI regression model was non-significant (explained variance 0.03%, P FDR = 0.87). Full performance metrics from each multivariate regression performed are reported in Supplementary Table 8.
Discussion
We demonstrated that, by combining environmental, clinical and sMRI baseline predictions, we could predict outcome in role functioning in CHR with 71.2% BACLSOCV, and significance, across seven geographically different European sites, but with much less accuracy and lower AUC than a CHR replication sample (BACLSOCV = 58.7%). On the other hand, by combining clinical and environmental predictions without sMRI, we could predict outcome role functioning in CHR with 65.4% BACLSOCV in the discovery sample and 67.7% BACLSOCV in the replication sample, in both cases with an AUC of around 0.75. Therefore, our results support our hypothesis that environmental variables inform prediction of outcome in role functioning. Furthermore, they encourage future research to employ, set up or redefine machine learning algorithms for worse outcome prediction in a complex, superordinate and multimodal, rather than unimodal perspective.Reference Koutsouleris, Kambeitz-Ilankovic, Ruhrmann, Rosen, Ruef and Dwyer7 Despite the good performance of our combined environmental and clinical risk calculator in CHR discovery and validation samples, linear mixed model analysis revealed that prognostic assignments (lower versus higher role outcome) were not associated with any other clinical trajectory. This finding suggests that the prognostic validity of this classifier seems limited to functional deficits and does not generalise to other clinical readouts. However, future studies involving the investigation of less state-affected clinical variables (i.e. academic functioning, work skills, resilience) should further clarify the extent of prognostic relevance of this model.
In ROD, only the combination of environmental and clinical variables predicted role outcome with significance and a modest BACLSOCV (58.9%), with an AUC of 0.60. It also pointed to a great extent of generalisability to the ROD replication sample (BACLSOCV = 62.5% and an even higher AUC of −0.72). Notably, despite lower BACLSOCV scores compared with CHR, linear mixed effects models used to calculate clinical trajectories in ROD revealed that role functioning outcome assignments based on environmental and clinical predictions stratified negative symptoms and environmental quality-of-life trajectories. This finding may indicate prognostic generalisation of this multimodal predictive model outside of its original role functioning domain.
Notably, accuracies in both discovery and replication samples were slightly lower in ROD than in CHR. A possible explanation emerges from the analysis of the prevalence of DSM-IV-TR diagnoses in both samples. A greater percentage of ROD individuals, relative to CHR, meet diagnostic criteria for at least one DSM-IV-TR psychiatric disorder. However, in our CHR samples, several and diverse DSM-IV-TR conditions are present, especially in mood and anxiety domains, consistent with previous literature.Reference Woods, Powers, Taylor, Davidson, Johannesen and Addington56 Unlike CHR, in ROD, the most represented DSM-IV-TR category across individuals is major depressive disorder. This pattern is observable in both discovery and replication samples, and across both timepoints (Table 3 and Supplementary Table 2). However, this observed homogeneity of our ROD group is only partially consistent with previous literature, which indeed reported frequent comorbidities between depression and other psychiatric disorders.Reference Thaipisuttikul, Ittasakul, Waleeprakhon, Wisajun and Jullagate57,Reference Hasin, Sarvet, Meyers, Saha, Ruan and Stohl58 We might speculate that the higher clinical variability in our CHR samples might have led to a more accurate and more representative multimodal predictive model for this population, and that the higher clinical homogeneity in our ROD samples may have driven the better generalisation of ROD models to other clinical trajectories. However, these hypotheses need to be tested by future studies; for example, studies using subtyping/clustering procedures.Reference Dwyer, Cabral, Kambeitz-Ilankovic, Sanfelici, Kambeitz and Calhoun59
Table 3 Prevalence comparisons of the DSM-IV-TR diagnoses in the CHR and ROD discovery samples characterised by lower versus higher role functioning at baseline and follow-up examinations 9 months later

Analyses were performed for diagnoses in the domains of mood, anxiety and substance misuse. Presence of threshold diagnostic criteria in the past month before respective timepoint was examined, using χ 2-tests. For dysthymic disorder, lifetime presence of threshold and subthreshold criteria were combined and compared against absence of lifetime criteria. P-values were group- and timepoint-wise corrected for multiple comparisons, using the false discovery rate. Significance was defined at α = 0.05. T0, baseline; T1, follow-up examinations 9 months after baseline; CHR, clinical high-risk; ROD, recent-onset depression; GF:R, Global Functioning Role; AWOPD, agoraphobia.
Taken together, our CHR and ROD findings suggest that individualised prediction of role functioning outcomes is possible, although with modest accuracy, in a replicable and geographically validated framework based on environmental and clinical information. sMRI, however, did not seem to play an important role in this prediction, as highlighted by the fact that the combined clinical-environmental-sMRI prediction model developed here could predict role outcome very well in the CHR discovery sample, but with much lower accuracy in the CHR validation sample, although the difference was marginally significant. This aligns with recent views cautioning researchers about neuroimaging-based machine learning findings, because of their excessively high dimensionality, especially in presence of small sample sizes and heterogeneous clinical phenomena.Reference Vieira, Gong, Scarpazza, Lui, Huang and Crespo-Facorro60 However, a recently published study has highlighted that sMRI models show great predictive power when applied to transition-to-psychosis prediction.Reference Koutsouleris, Dwyer, Degenhardt, Maj, Urquijo-Castro and Sanfelici61 Considering our findings and this recent evidence, we may speculate that structural neuroimaging-based information could be more informative for diagnostic prediction, rather than for transdiagnostic outcomes. Future studies are strongly warranted to validate this hypothesis.
Consistently, our assessment of transdiagnostic generalisability supported the prognostic relevance of the combination of environmental and clinical risk calculators for role prediction, showing accuracy (62.4% BACLSOCV and 0.71 AUC), significance and generalisability to any replication sample combination. Notably, also the combination of environmental, clinical and sMRI decision scores led to accurate (61.1% BACLSOCV and 0.67 AUC), significant and generalisable findings, and its performance metrics were very similar to those of the combined clinical and environmental risk calculator (Table 2 and Supplementary Table 6). These findings further support the view that MRI data carry a negligible amount of predictive information when applied to the longitudinal investigation of transdiagnostic outcomes.
Interestingly, investigation of the reliability of features within predictive models built on both separate and pooled on CHR and ROD samples revealed that premorbid adjustment in adulthood was transdiagnostically important for outcome prediction. This suggests that this environmental feature might be associated with role functional outcome regardless of the clinical population tested, and that the transdiagnostic potential of our combined environmental and clinical risk calculator is mainly driven by this environmental feature. Notably, the PAS scale measures the degree of achievement of developmental goals over time, according to gender, socioeconomic status and age.Reference Cannon-Spoor, Potkin and Wyatt62 Therefore, adult maladjustments to developmental goals may be more predictive compared with other features, because they represent the most proximal-to-baseline environmental feature in the algorithm, and may indeed be a result of earlier environmental adverse events.Reference Howes and Murray14,Reference Vocisano, Klein, Keefe, Dienst and Kincaid46 Consistently, besides premorbid adjustment in adulthood, the combinations of environmental adverse events with the highest predictive value were different between CHR- and ROD-based risk calculators, but all included the presence of earlier developmental maladjustment. Indeed, after PAS adulthood, the most predictive feature for role functioning prediction in the CHR sample was the occurrence of childhood trauma,Reference Loewy, Corey, Amirfathi, Dabit, Fulford and Pearson39 and, in ROD, early adolescence maladjustment to environment.Reference Tyborowska, Volman, Niermann, Pouwels, Smeekens and Cillessen63 Findings therefore highlight the existence of both transdiagnostic and syndrome-specific environmental adverse events able to predict role outcome in different clinical populations.Reference Oliver, Radua, Reichenberg, Uher and Fusar-Poli64 Notably, environmental adverse events occurred in different time periods may increase the risk for disease in a composite/compounding way.Reference Bhavsar, Boydell, McGuire, Harris, Hotopf and Hatch65 For example, it may be hypothesised that childhood adversities (e.g. childhood trauma) may increase the risk for subsequent maladjustment (e.g. lower levels of environmental adjustment in adulthood) by increasing the risk of exposure to further environmental stressors (e.g. bullying victimisation), thus acting as triggers of a causal environmental path.Reference Morgan, Reininghaus, Fearon, Hutchinson, Morgan and Dazzan66 However, childhood adversities may either predict subsequent adversities, or interact with other adult adversities,Reference Hafeman and Schwartz67 thus making the picture even more complex. This view is consistent with findings from our recursive feature elimination procedure, which showed that the most accurate environmental model in CHR was the one constructed on all environmental adverse events, hence reiterating the importance of taking into account the complex gestalt of environmental maladjustments and adverse events.
Finally, we observed that environmental decision scores predicting follow-up role outcome were significantly associated in CHR, with sMRI baseline data, and in ROD, with clinical data. These preliminary findings partially support the hypothesis that the history of environmental adverse events may mediate differential associations of baseline clinical and sMRI predictors with follow-up role outcome. However, future path analysis studies investigating the specific mediating or moderating role of environmental events in the relationship between clinical data, GMVs and follow-up outcome are warranted.
Limitations
This study has some limitations. Although our study was based on an extension of previously published prediction models,Reference Koutsouleris, Kambeitz-Ilankovic, Ruhrmann, Rosen, Ruef and Dwyer7 we could not directly compare the two sets of results, as the samples did not exactly overlap. This was because complete lack of environmental assessments for some of the patients in the CHR and ROD samples. Furthermore, it should be noted that the small sample size did not allow us to investigate potential gender effects on role functioning predictions. As gender is differentially linked to environmental adversity effects,Reference Evans, Grella and Upchurch68 future studies should further investigate this relationship. Also, calibration results (Fig. 1 and Supplementary Appendix 1, Section 10) revealed relatively low, although not perfect, ECEs. This seems to be frequent with Support Vector Machine algorithms.Reference Huang, Li, Macheret, Gabriel and Ohno-Machado69 Future studies might take into account calibration already the model-building phase, e.g. via Bayesian Binning into Quantiles, to try to achieve better calibration performance.Reference Naeini, Cooper and Hauskrecht70 Moreover, the type of environmental information collected might represent a limit. As well as developmental maladjustments, childhood traumatic experiences and bullying victimisation have been all previously associated with psychosisReference Loewy, Corey, Amirfathi, Dabit, Fulford and Pearson39,Reference Tarbox, Addington, Cadenhead, Cannon, Cornblatt and Perkins41 and depression;Reference Hill, Mellick, Temple and Sharp43,Reference Negele, Kaufhold, Kallenbach and Leuzinger-Bohleber44,Reference Vocisano, Klein, Keefe, Dienst and Kincaid46 information regarding other adverse life events that are known risk factors for psychosis and other mental disorders were not collected within this study.Reference Stilo and Murray71 Furthermore, our environmental predictive model was based on variables reflecting the occurrence of environmental adverse events, as well as variables reflecting the level of environmental adjustment to such adverse events (and many others), as measured by PAS. It cannot be excluded, therefore, that PAS score variations may be the result of not only the occurrence of environmental adversities across the lifespan, but also individual differences in the ability of adapting to adverse environmental exposures, thus having a close relationship with core adjustment-related psychological attributes, such as coping strategies and resilience.Reference Holz, Tost and Meyer-Lindenberg72 This relationship needs to be thoroughly and experimentally investigated by future studies.
Importantly, it should be noted that our validation sample was recruited within the same study of the discovery sample, although a part of the validation sample was recruited at different sites to the discovery ones. Furthermore, we employed the NeuroMiner software to carry out our machine learning pipeline, as one of the main aims of the PRONIA consortium was to facilitate open science and validation of findings via the NeuroMiner Model Library (see Data availability). However, the current NeuroMiner version allowed us to perform permutation testing only on the discovery cohorts, without providing significance estimates for replication performance. Future validation of the model in completely independent populations from other consortia and countries is warranted, to better account for optimism in the performance estimate and further characterise the performance of our models. Another important limitation of our study is the small sample size. CHR is a difficult population to recruit and keep in a longitudinal study, because of the risk-related aspect of their condition. This issue is quite common; indeed, our sample size is in line with other samples employed in recent machine learning studies conducted on CHR populations.Reference Haining, Brunner, Gajwani, Gross, Gumley and Lawrie73,Reference Mongan, Focking, Healy, Susai, Heurich and Wynne74 Moreover, in our case, it should be noted that findings are further limited by the number of features employed in the models. Machine learning-based predictions require a large amount of data,Reference van der Ploeg, Austin and Steyerberg75 but the number of features we used was limited. Consistently, within our machine learning pipeline, the number of events per predictor variable is lower than recently recommended.Reference Riley, Snell, Ensor, Burke, Harrell and Moons76 However, this is not uncommon in the CHR field. Indeed, although a large number of input features reduces the risk of overoptimistic results, it could be difficult to translate models based on a large number of features into clinical, real-world settings, where data obtained are usually limited because of patient adherence, drop out or time constraints. With this regard, it has been previously suggestedReference Sanfelici, Dwyer, Antonucci and Koutsouleris77 to employ double-cycle, nested, leave-site-out cross-validation techniques as a gold-standard strategy to mitigate overfitting and optimism of models’ performance, especially in cases of limited numbers of features and/or of individuals, as in this study. Nevertheless, taking all of these limitations into account, it should be noted that the understanding of the clinical usefulness of the presented models is strictly dependent on the results of further validation on larger and geographically diverse cohorts. Future studies are warranted to thoroughly investigate the stability and generalisability of our models, and further test the potential of translation into clinical practice of our models. With respect to the clinical implementation of our models, it should be also noted that the threshold we have chosen for the classification metrics was not determined based on clinical grounds. Indeed, CHR and ROD are very heterogeneous clinical states, and obtaining consensus-based risk estimates for these help-seeking populations, especially for transdiagnostic outcomes like occupational functioning, is a challenge. An online machine learning-based strategy has been recently proposed in a publication aiming at developing psychosis predictive models in diverse at-risk populations across different consortia.Reference Koutsouleris, Worthington, Dwyer, Kambeitz-Ilankovic, Sanfelici and Fusar-Poli25 Future studies are warranted to test the feasibility of such solution, and to direct efforts toward the generation of a public library of machine learning-based estimated risk distributions reflecting diverse help-seeking populations.
In conclusion, we explored syndrome-specific and transdiagnostic predictive models combining clinical and environmental information, which could predict role outcome with moderate accuracy in several independent samples. For ROD, these predictions seem to be prognostically relevant to non-functioning clinical trajectories, like negative symptoms and quality of life. The modest, although stable across samples, performance of our combined clinical and environmental predictive models (both the syndrome-specific and transdiagnostic) encourage future research to spend significant efforts in further validating existing multimodal risk calculators to fully assess their degree of applicability in healthcare settings, as well as in defining guidelines for models’ comparability and replicability.Reference Rosen, Betz, Schultze-Lutter, Chisholm, Haidl and Kambeitz-Ilankovic26 If geographically and extensively validated, risk calculators built on both CHR and affective populations could benefit patients with a realistic, personalised prognostic estimation of their functioning level irrespective of diagnostic boundaries, thus supporting early rehabilitation and a better integration of patients into their societal environment. However, future studies on environmental adverse events are warranted to define to what extent risk factors interact with each other, with symptoms profiles and with neurobiological alterations, to predispose young individuals for worse role outcomes. Such multimodal frameworks will more likely mirror the complex and heterogeneous architecture of psychosis and depression risk, and would hopefully contribute to provide models with even higher accuracies, closer to real-world scenarios, and with more potential for translation into clinical practice.
Supplementary material
To view supplementary material for this article, please visit https://doi.org/10.1192/bjp.2022.16
Data availability
The combined clinical and environmental prediction models can be found in the NeuroMiner Model Library (http://www.proniapredictors.eu/#). All analysis pipelines from NeuroMiner are publicly available via GitHub (https://github.com/neurominer-git/).
Acknowledgements
The PRONIA consortium:
The authors listed here performed the screening, recruitment, rating, examination and follow-up of the study participants. They were involved in implementing the examination protocols of the study, setting up its IT infrastructure, and organising the flow and quality control of the data analysed in this manuscript between the local study sites and the central study database.
Department of Psychiatry and Psychotherapy, Ludwig-Maximilian-University, Munich, Germany: Shalaila Haas, Alkomiet Hasan, Claudius Hoff, Ifrah Khanyaree, Aylin Melo, Susanna Muckenhuber-Sternbauer, Yanis Köhler, Ömer Öztürk, Nora Penzel, David Popovic, Adrian Rangnick, Sebastian von Saldern, Rachele Sanfelici, Moritz Spangemacher, Ana Tupac, Maria Fernanda Urquijo, Johanna Weiske, Antonia Wosgien, Camilla Krämer.
Department of Psychiatry and Psychotherapy, University Hospital and Faculty of Medicine, University of Cologne, Cologne, Germany: Karsten Blume, Dominika Julkowski, Nathalie Kaden, Ruth Milz, Alexandra Nikolaides, Mauro Seves, Silke Vent, Martina Wassen.
Department of Psychiatry (Psychiatric University Hospital, University Psychiatric Clinics Basel), University of Basel, Switzerland: Christina Andreou, Laura Egloff, Fabienne Harrisberger, Ulrike Heitz, Claudia Lenz, Letizia Leanza, Amatya Mackintosh, Renata Smieskova, Erich Studerus, Anna Walter, Sonja Widmayer.
Institute of Mental Health & School of Psychology, University of Birmingham, UK: Chris Day, Sian Lowri Griffiths, Mariam Iqbal, Paris Lalousis, Mirabel Pelton, Pavan Mallikarjun, Alexandra Stainton, Ashleigh Lin.
Department of Psychiatry, University of Turku, Finland: Alexander Denissoff, Anu Ellilä, Tiina From, Markus Heinimaa, Tuula Ilonen, Päivi Jalo, Heikki Laurikainen, Antti Luutonen, Akseli Mäkela, Janina Paju, Henri Pesonen, Reetta-Liina Säilä, Anna Toivonen, Otto Turtonen.
Department of Psychiatry (Psychiatric University Hospital LVR/HHU Düsseldorf), University of Düsseldorf, Germany: Sonja Botterweck, Norman Kluthausen, Gerald Antoch, Julian Caspers, Hans-Jörg Wittsack.
General Electric Global Research Inc., USA: Ana Beatriz Solana, Manuela Abraham, Timo Schirmer.
Workgroup of Paolo Brambilla, University of Milan, Italy:
Department of Neuroscience and Mental Health, Fondazione IRCCS Ca' Granda Ospedale Maggiore Policlinico, University of Milan, Italy: Marika Belleri, Francesca Bottinelli, Giuseppe Delvecchio, Adele Ferro, Eleonora Maggioni, Marta Re, Letizia Squarcina; Programma2000, Niguarda Hospital, Italy: Emiliano Monzani, Maurizio Sberna; San Paolo Hospital, Italy: Armando D'Agostino, Lorenzo Del Fabro; Villa San Benedetto Menni, Albese con Cassano: Giampaolo Perna, Maria Nobile, Alessandra Alciati.
Workgroup of Paolo Brambilla at the University of Udine, Italy:
Department of Medical Area, University of Udine, Udine, Italy: Matteo Balestrieri, Carolina Bonivento, Giuseppe Cabras, Franco Fabbro.
Author contributions
N.K. had full access to all of the data in the study and takes responsibility for the integrity of the data and the accuracy of the data analysis. L.A.A. and N.K. conceived and designed the study. N.K., R.S. A.P., N.P., L.K.-I., S.R., A.R., D.D., K.C., J.K., T.H., F.S.-L., C.P., S.J.W., P.B., S.B., A.B., R.K.R.S., E.M., R.U., A.F., M.R., I.A., O.F.O. and M.S.D. were responsible for data acquisition, analysis or interpretation. L.A.A., R.S. and N.K. drafted the manuscript. N.P., R.S., G.P., G.B, F.S.-L., T.H., A.P., R.L., A.F., K.C., U.D. and R.U. critically revised the manuscript for important intellectual content. N.K., L.K.-I., E.M., S.R., R.K.R.S., C.P., P.B., S.B., S.J.W., A.B. and R.U. obtained funding. N.K., P.F. and A.B. supervised the study.
Funding
This work was supported by the EU-FP7-HEALTH grant for the project ‘PRONIA’ (Personalized Prognostic Tools for Early Psychosis Management; PI: N.K., agreement number: 602152) and by the Structural European Funding of the Italian Minister of Education and Research (Attraction and International Mobility – AIM - action, grant agreement No 1859959). The AIM action also funds L.A.A.’s salary.
Declaration of interest
A.B. has received lecture fees from Otsuka, Janssen and Lundbeck; and consultant fees from Biogen. N.K. has received honoraria for talks presented at education meetings organised by Otsuka/Lundbeck. N.K. and E.M. hold commercial patents that are related to the present work (https://patents.google.com/patent/US20160192889/). C.P. participated in advisory boards for Janssen-Cilag, AstraZeneca, Lundbeck and Servier; received honoraria for talks presented at educational meetings organized by AstraZeneca, Janssen-Cilag, Eli Lilly, Pfizer, Lundbeck and Shire; and was supported by National Health and Medical Research Council Senior Principal Research Fellowship (grants 628386 and 1105825) and European Union–National Health and Medical Research Council (grant 1075379). G.P.'s position is funded by the European Union's Horizon 2020 research and innovation programme under the Marie Sklodowska-Curie grant agreement number 798181. L.A.A.'s salary is funded from the Structural European Funding of the Italian Minister of Education (Attraction and International Mobility – AIM - action, grant agreement number 1859959).
L.K.-I., D.D., F.S.-L. and R.U. are members of the BJPsych editorial board and did not take part in the review or decision-making process of this paper. No other disclosures were reported.
eLetters
No eLetters have been published for this article.