Introduction
Non-native tones are often hard to acquire, even to those from a tone language background (Hao, Reference Hao2012; Lee & Hung, Reference Lee and Hung2008; Li & Zhang, Reference Li and Zhang2010). Research has suggested that musicianship enhances non-native tone perception (music-to-language transfer, Alexander et al., Reference Alexander, Wong and Bradlow2005; Choi, Reference Choi2020; Delogu et al., Reference Delogu, Lampis and Belardinelli2010), motivating the OPERA hypothesis (Patel, 2011, Reference Patel2014). Methodologically, the preponderance of research has treated musicianship as a binary variable (e.g., Cooper & Wang, Reference Cooper and Wang2012; Wong & Perrachione, Reference Wong and Perrachione2007 Zheng & Samuel, Reference Zheng and Samuel2018). As such, a musician group often contained learners of diverse musical instruments. Different types of musical instruments have different pitch processing demand, so it is possible that they have different effects on tone perception (FitzGerald & Paulus, Reference FitzGerald, Paulus, Klapuri and Davy2006). Even though OPERA explicitly acknowledges the heterogeneity nature of musicianship, no study to our knowledge has directly tested this claim. To enrich OPERA and its body of evidence, we investigated the effect of pitched and unpitched musicianship on tone identification and word learning.
OPERA predicts that music training enhances the neural encoding of speech when the training meets five conditions: overlap, precision, emotion, repetition, and attention (Patel, 2011, Reference Patel2014). Specifically, the processing of an acoustic feature in music and language should involve overlapping neural networks. Moreover, the music training must require greater precision in acoustic processing than speech perception. Lastly, the musical activities must be associated with strong positive emotion, require repeated practice, and entail focused attention.
Evidence of music-to-language transfer in tone perception undergirds OPERA. Several studies found that musicians from non-tone language backgrounds (e.g., English and Italian) outperformed their non-musician counterparts on Mandarin tone discrimination and identification (Alexander et al., Reference Alexander, Wong and Bradlow2005; Delogu et al, Reference Delogu, Lampis and Belardinelli2010; Lee & Hung, Reference Lee and Hung2008). This musical advantage was also evident in musicians with tone language background (i.e., Thai) (Cooper & Wang, Reference Cooper and Wang2012). Yet, these studies investigated music-to-language transfer based on a small number of lexical tones (i.e., four). Choi (Reference Choi2020) examined musical advantage in tone perception in Cantonese which has a larger number of tones (i.e., six). Results revealed that English musicians outperformed non-musicians on discriminating half of the Cantonese tones (i.e., high-rising, high level, and mid-level).
Beyond tone identification and discrimination, musical advantage extends to the higher perceptual levels as reflected by tone sequence recall and tone word learning (Choi, Reference Choi2020; Cooper & Wang, Reference Cooper and Wang2012; Wong & Perrachione, Reference Wong and Perrachione2007). Tone sequence recall involves forming and storing representations of tones in the memory while tone word learning involves the use of tonal contrasts to differentiate meaning. Choi (Reference Choi2020) found that English musicians outperformed non-musicians on recalling Cantonese contour tone sequences. Wong and Perrachione (Reference Wong and Perrachione2007) found that English musicians achieved higher attainment than non-musicians in Mandarin tone word learning. Building on Wong and Perrachione (Reference Wong and Perrachione2007) which had a small sample size (n = 17) and only included three Mandarin tones, Cooper and Wang (Reference Cooper and Wang2012) conducted a larger word learning study (n = 54) with five Cantonese tones. While English musicians outperformed English non-musicians, Thai musicians (mean accuracy = 63%, no ceiling effect) did not outperform Thai non-musicians (mean accuracy = 75%, no ceiling effect) on tone word learning. This suggested that musicianship did not facilitate tone word learning in listeners from a tone language background. The authors argued that music-to-language transfer was not as straightforward as assumed, but must take L1 background and other factors into account. To summarize, existing research on music-to-language transfer has identified the benefit of musicianship in tone perception across perceptual levels. As described below, different types of musical instruments have different demands on pitch processing. To extend the literature, we investigated a potential factor undergirding music-to-language transfer – the choice of musical instruments. In this study, we define pitched musicians as instrumentalists whose musical instruments involve pitch control (e.g., piano and violin). We define unpitched musicians as percussion instrumentalists whose instruments are not normally used to produce melodies (e.g., simple drums, FitzGerald & Paulus, Reference FitzGerald, Paulus, Klapuri and Davy2006).
Existing studies on music-to-language transfer have largely ignored the heterogeneity of musicianship (e.g., Alexander et al., Reference Alexander, Wong and Bradlow2005; Cooper & Wang, Reference Cooper and Wang2012; Lee & Hung, Reference Lee and Hung2008; Wong & Perrachione, Reference Wong and Perrachione2007). Although OPERA acknowledges the heterogeneity nature of musicianship, empirical evidence of this claim is absent. OPERA states that music training should demand higher precision of an acoustic feature than speech for music-to-language transfer to occur. In speech communication, the primary goal of listeners is to understand the semantic content. In an ecological context, there are many redundant cues to meaning (e.g., semantic context and transitional probabilities). Even without precise auditory perception (e.g., F0), listeners can still successfully engage in speech communication (Patel, Reference Patel2012). By contrast, pitched musicians need to carefully monitor and regulate the pitches produced along a continuous spectrum (e.g., violin) or an equal-tempered scale (e.g., piano) (Alexander et al., Reference Alexander, Wong and Bradlow2005; Parker, Reference Parker1983). Unlike speech communication, the lack of redundant cues for pitch monitoring poses an upward pressure on the auditory system to refine its F0 encoding. Therefore, we hypothesize that pitched musicians exhibit an advantage in Thai tone identification and word learning, relative to non-musicians.
Furthermore, we hypothesize that such advantage is only specific to pitched musicians. Unlike pitched musicians, unpitched musicians need not monitor and regulate the pitches that they produced. While unpitched music training has a high demand on rhythm and timing, it has minimal demand on pitch precision (FitzGerald & Paulus, Reference FitzGerald, Paulus, Klapuri and Davy2006). Since pitch but not rhythm cues Thai tones (Burnham et al., Reference Burnham, Kasisopa, Reid, Luksaneeyanawin, Lacerda, Attina, Rattanasone, Schwarz and Webster2015), we anticipate that unpitched musicians would not enjoy pitched musicians’ advantage.
Our further goal is to examine whether the specificity (or universality) of musicianship applies to both perceptual and higher-level linguistic processing. Tone identification task only requires participants to match the tones with their tone diagrams (see Figure 1). Participants only need to form temporary representations of the tone and compare it with the visual information. Span across seven sessions on different days, tone word learning task requires participants to perceptually learn and associate the speech information with meanings (Cooper & Wang, Reference Cooper and Wang2012). Unlike tone identification task, participants have to form a long-term phonological representation of the target sound and link it with the semantic representation. In the session tests, participants have to utilize tonal and segmental information to retrieve the semantic representations. Thus, we tested the participants on tone identification and word learning.
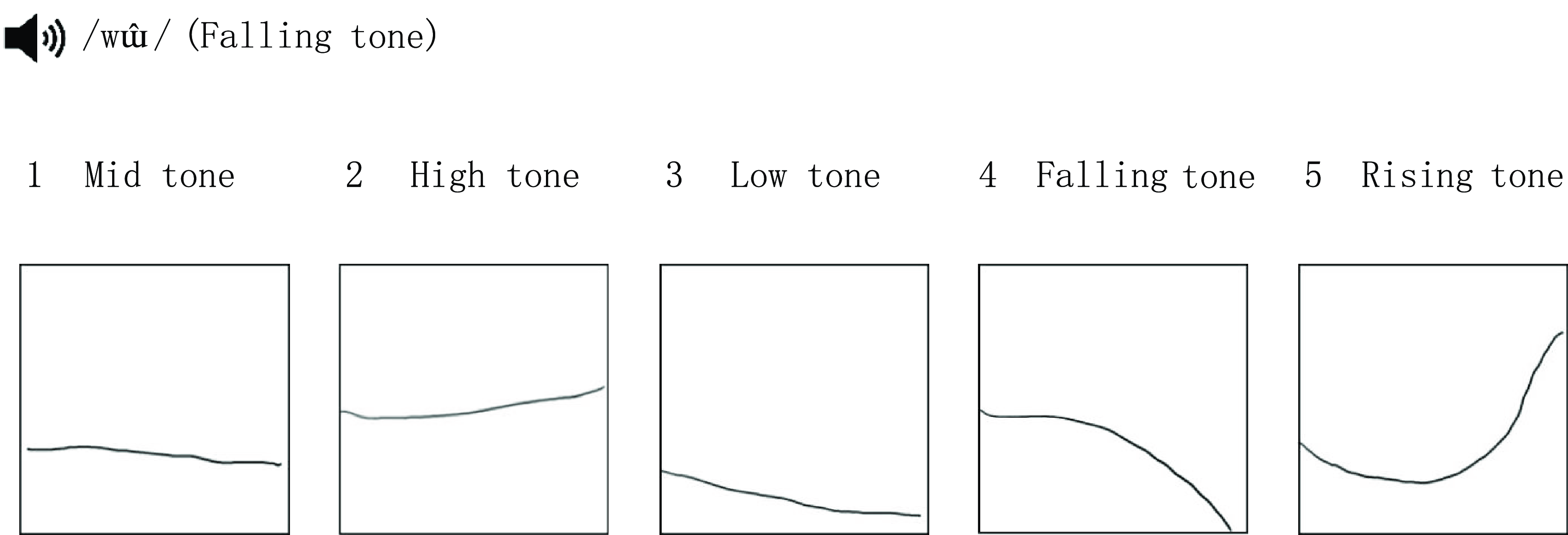
Figure 1. The Familiarization Phase of Tone Identification Task.
In the present study, we subdivided the musicians into pitched musicians and unpitched musicians (Cooper & Wang, Reference Cooper and Wang2012; Wong & Perrachione, Reference Wong and Perrachione2007). To control for within-group differences, we only included pianists and violinists in the pitched instrument group as they have similar pitch detection ability (Parker, Reference Parker1983). Regarding the choice of tone language, most studies have chosen Cantonese (Choi, Reference Choi2020; Cooper & Wang, Reference Cooper and Wang2012) and Mandarin tones (Alexander et al., Reference Alexander, Wong and Bradlow2005; Wong & Perrachione, Reference Wong and Perrachione2007; Zheng & Samuel, Reference Zheng and Samuel2018). In Hong Kong, Mandarin learning is a compulsory at school. Thus, it is impractical to find Cantonese listeners who are naïve to Mandarin tones. Unlike Mandarin, Thai language is rarely used in Hong Kong, making it a perfect probe for naïve tone perception (Choi & Lai, Reference Choi and Laiin press). Testing the Cantonese listeners with naïve tones can avoid the possible confound of Mandarin proficiency differences between groups. Phonologically, Thai has a rich tonal inventory of five lexical tones which includes three level tones (mid, low, and high) and two contour tones (falling and rising) (Abramson, Reference Abramson1978; Morén & Zsiga, Reference Morén and Zsiga2006). Working memory and non-verbal intelligence may influence language learning success (Miyake & Friedman, Reference Miyake, Friedman, Healy and Bourne1998), so we controlled for them. In short, the following research questions motivated our study:
-
(1) Do pitched musicians outperform non-musicians on Thai tone identification and word learning?
-
(2) Do unpitched musicians outperform non-musicians on Thai tone identification and word learning?
We predict that pitched – but not unpitched – musicians would outperform non-musicians on Thai tone identification and word learning. To address the potential concern about null results interpretation (i.e., unpitched musicians and non-musicians are expected to perform similarly), where appropriate, we would supplement any null hypothesis significance testing with Bayesian hypothesis testing (Kruschke, Reference Kruschke2018).
Method
Participants
We recruited 44 Cantonese listeners (19 males and 25 females) in Hong Kong via mass emails and posters. The sample size was modest given the upper limit of the ethics approval typically granted to undergraduate students at The University of Hong Kong. According to language and music background questionnaires, all participants received or were receiving tertiary education, had typical hearing, and had no Thai learning experience nor absolute pitch (Choi, Reference Choi2022a, Choi & Chiu, Reference Choi and Chiu2022; Choi et al., Reference Choi, Tong, Gu, Tong and Wong2017). The participants were sorted into three groups, that is, pitched musician (n = 15), unpitched musician (n = 13), and non-musician (n = 15). Adopting pre-established criteria in previous studies (Choi, Reference Choi2021, Reference Choi2022b, Reference Choi2022c; Cooper & Wang, Reference Cooper and Wang2012), the pitched musicians had at least 7 years of continuous piano and/or violin training, less than 2 years of unpitched percussion training, and could play their instruments at the time of testing. The unpitched musician had at least 7 years of continuous unpitched percussion training, less than 2 years of pitched musical training, and could play their instruments at the time of testing. The non-musicians had less than 2 years of musical training, no musical training in the past 5 years, and could not play any musical instrument at the time of testing. One unpitched musician was excluded from the study due to excessive pitched music training. The final sample consisted of 15 pitched musicians, 13 unpitched musician, and 15 non-musicians, with mean ages of 23.47 years (SD = 3.18 years), 25.54 years (SD = 4.99 years), and 24.13 years (SD = 2.59 years) respectively.
Tables 1 and 2 summarize the music background of the pitched musicians and the unpitched musicians, respectively. The mean onset age of music training was 5.93 years (SD = 1.39 years) for the pitched musicians, 13.73 years (SD = 4.22 years) for the unpitched musicians, and 8.33 years (SD = 4.16 years) for the non-musicians. On average, the pitched musicians and the unpitched musicians had received 11.77 years (SD = 3.87 years) and 10.81 years (SD = 3.68 years) of music training, respectively, while three non-musicians had only received 1 year (SD = 0 year) of music training.
Table 1. Music background of the pitched musicians
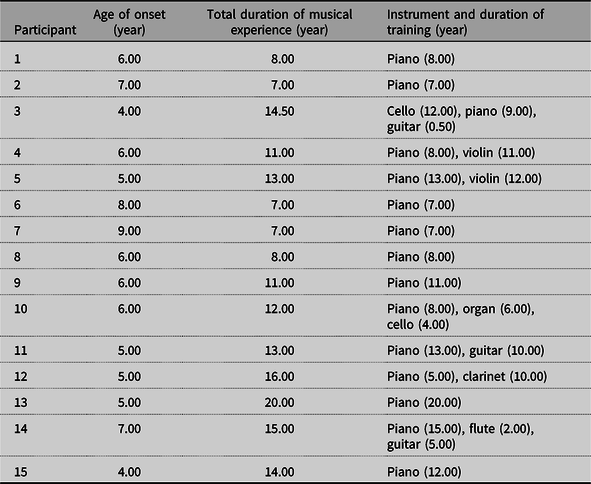
Table 2. Music background of the unpitched musicians
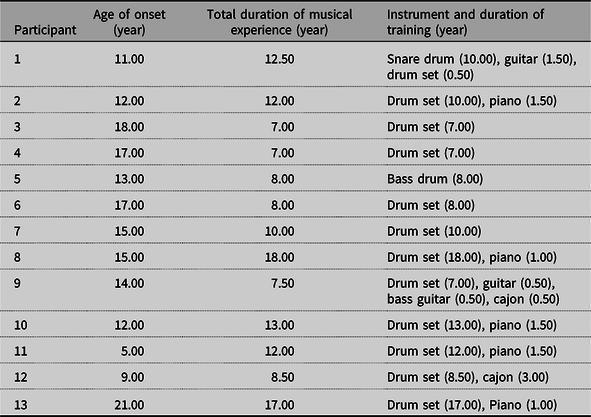
Procedure
Participants completed all the tasks using a computer with Sennheiser HD280 PRO headphones in a soundproof room at The University of Hong Kong. All the auditory stimuli were recorded by two native Thai speakers (one male, one female) via Shure SM58 at 48 kHz sampling rate in the same soundproof room.
Working memory task
We administered a backward digit span task. Participants listened to sequences of digits beginning from two digits in length up to eight digits and recalled in reverse order. At each length, there were two sequences. The task terminated upon failure in recalling both sequences at the same length. The maximum sequence length that participants could recall accurately was recorded. The sample-specific internal consistency was satisfactory (Cronbach’s α = .62).
Non-verbal intelligence task
We administered the adapted short form of Raven’s 2 Progressive Matrices (Raven et al., Reference Raven, Rust, Chan and Zhou2018). Participants were asked to choose an appropriate picture to complete a matrix in 24 trials. Each correct response yielded one point. The sample-specific internal consistency was fair (Cronbach’s α = .49).
Tone identification task
Familiarization phase. Participants first listened to the female-produced /wɯ1/, /wɯ2/, /wɯ3/, /wɯ4/, and /wɯ5/ for three times while viewing the corresponding tone diagram. Participants then engaged in a 15-trial identification task (1 syllable × 5 tones × 1 speaker × 3 repetitions). As Figure 1 shows, participants indicated the tone by pressing the number of the corresponding tone diagram. Feedback on accuracy and the correct answer were given.
Main tone identification task. The task assessed participants’ ability to identify Thai tones. It had the same format as the familiarization phase except that there were 100 trials (5 syllables (/tia/, /dɯa/, /ŋɤ/, /ruj/, /few/) × 5 tones × 2 speakers × 2 repetitions). Participants’ response accuracy was collected without feedback given. All the syllables were formed by Thai consonants and vowels. The sample-specific internal consistency was very high (Cronbach’s α = .92).
Tone word identification training
Participants underwent seven 30-min training sessions using E-prime 3.0 software (Psychology Software Tools, 2016), on 3 days within 2 weeks. There were two sessions on each of the first two days and three sessions on the third day. They learned 15 Thai words which included three CV monosyllables (/hϵː/, /mu:/, and /piː/) produced in the five Thai tones. Unfamiliar phonotactic structures impair word learning, so the monosyllables contain consonants and vowels common to Cantonese and Thai (Ellis & Beaton, Reference Ellis and Beaton1993). For each session, we used two tokens of each word per speaker (/piː-H-female/ two tokens;/piː-H-male/ two tokens).
In each session, participants completed five training blocks in randomized order, followed by two review blocks and one session test. In each training block, participants first listened to four randomized repetitions (2 speakers × 2 repetitions) of three words of different CV structures and tones while viewing pictures depicting the words’ meanings. Then, participants completed a 12-trial quiz (3 words × 2 speakers × 2 repetitions) on the three words with feedback given. Participants identified the meaning of the stimulus by selecting the correct picture among three options.
Review 1 comprised 15 trials. Participants listened to the 15 words blocked by syllable structure produced by the female speaker and selected a picture depicting the word’s meaning from six options. Review 2 consisted of 30 randomized trials (15 words × 1 repetition × 2 speakers) in a similar format except that the participants selected a picture from 15 options. Feedback on accuracy and correct answer were given with the stimulus replayed.
The session test comprised 60 randomized trials (15 words × 2 repetitions × 2 speakers) in a similar format as Review 2 except that no feedback was given. Participants’ mean percent accuracy in session tests of sessions 1 and 7 was collected to evaluate the improvement in tone word learning proficiency from training. The sample-specific internal consistencies were high in the session tests of session 1 (Cronbach’s α = .87) and session 7 (Cronbach’s α = .95).
Results
Preliminary analysis
Prior to the main analyses, we first ascertained whether the three groups differed in working memory and non-verbal intelligence. Thus, we conducted two sets of one-way ANOVAs with group (pitched musician, unpitched musician, and non-musician) as the between-subjects factor. ANOVAs revealed non-significant main effect of group in working memory, p = .296, and non-verbal intelligence, p = .495. These reflect that the three groups matched on working memory and non-verbal intelligence. To be empirically stringent, we still controlled for these variables in the main analyses.
Tone identification
Our first hypothesis is that pitched – but not unpitched – musicians outperform non-musicians on Thai tone identification. The mean accuracy was normally distributed with no extreme skewness or kurtosis (Rheinheimer & Penfield, Reference Rheinheimer and Penfield2001). We further computed the mean accuracy of the level tones by averaging the accuracies of the high, mid, and low tones, and the mean accuracy of the contour tones by averaging the accuracies of the falling and the rising tones (see Figure 2).
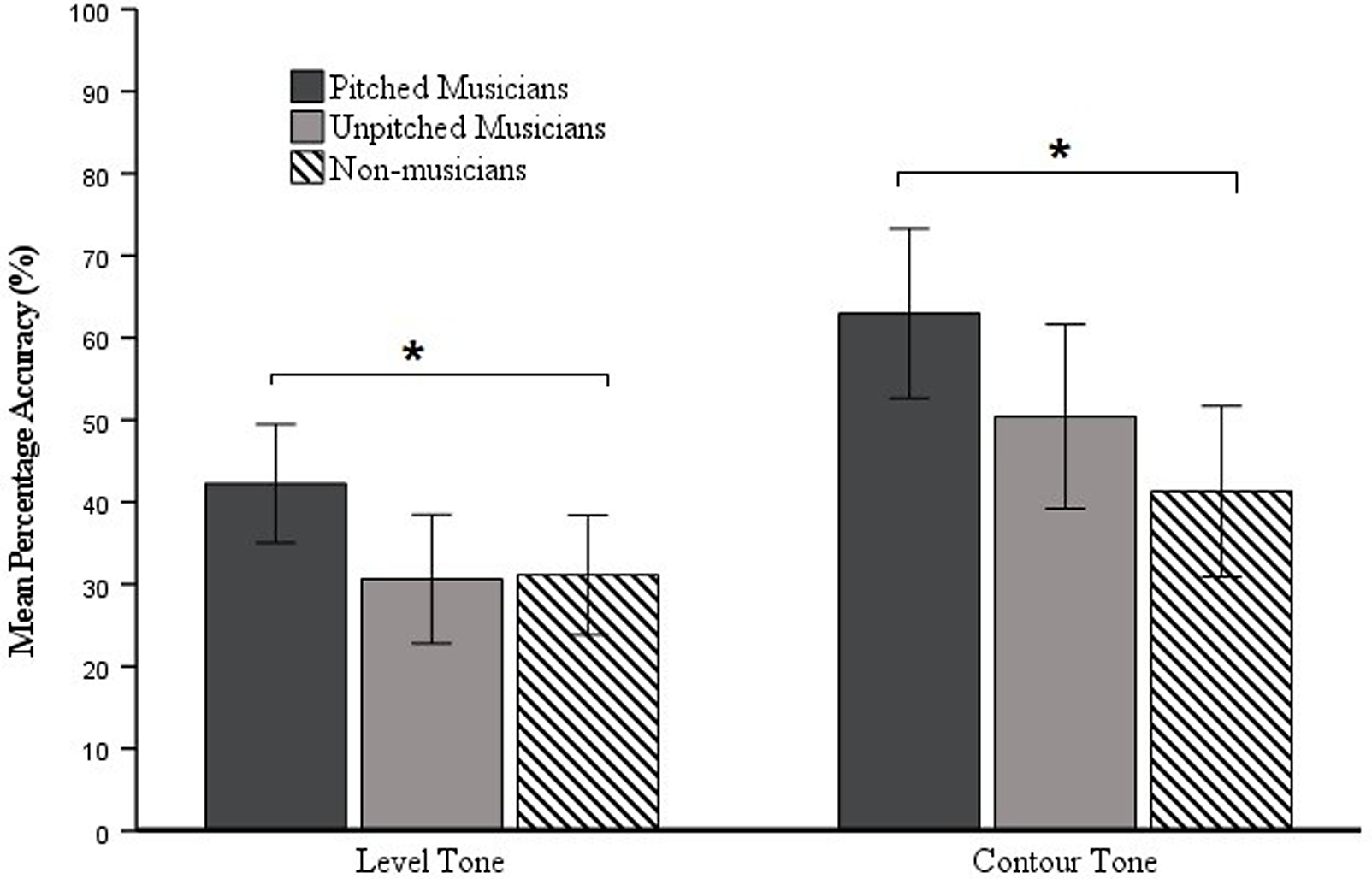
Figure 2. Mean Percentage Accuracy of Each Group for Level Tone and Contour Tone Identification. * p < .05. The error bars denote 95% confidence intervals.
To examine the effect of musicianship type on tone identification, we conducted a two-way mixed ANCOVA on mean accuracy with tone type (level and contour) as the within-subject factor, group (pitched musician, unpitched musician, and non-musician) as the between-subjects factor, and working memory and non-verbal intelligence as the covariates. ANCOVA showed a significant main effect of group, F(2, 38) = 4.74, p = .015, η p 2 = 0.20. Post hoc pairwise comparisons with Bonferroni adjustments revealed that the pitched musicians significantly outperformed the non-musicians, p = .015, while the unpitched musicians did not, p = 1.00. This indicated that the pitched musicians but not the unpitched musicians exhibited a musical advantage in tone identification. However, the pitched musicians did not significantly outperform the unpitched musicians, p = .126. The main effect of tone type, p = .602, and the two-way interaction, p = .170, were not significant.
Tone word learning
Our second hypothesis is that pitched – but not unpitched – musicians outperform non-musicians on Thai tone word learning. Based on their skewness and kurtosis, the mean accuracies in sessions 1 and 7 were normally distributed (Rheinheimer & Penfield, Reference Rheinheimer and Penfield2001).
To examine the effect of musicianship type on word learning performance, we conducted a two-way mixed ANCOVA on mean accuracy with session (session 1 and session 7) as the within-subject factor, group (pitched musician, unpitched musician, and non-musician) as the between-subjects factor, and working memory and non-verbal intelligence as the covariates. The analysis yielded significant main effects of session, F(1, 38) = 9.36, p = .004, η p 2 = 0.20, and group, F(2, 38) = 5.11, p = .011, ηp 2 = 0.21. Most importantly, the interaction between session and group was significant, F(2, 38) = 5.46, p = .008, ηp 2 = 0.22.
Next, we conducted simple effects analysis to unpack the interaction between session and group (see Figure 3). In session 1, all groups performed similarly, ps > .05. In session 7, the pitched musicians significantly outperformed the non-musicians, p = .002, but the unpitched musicians did not, p = .152. As in tone identification, the pitched musicians but not the unpitched musicians exhibited a musical advantage in tone word learning. No significant difference was found between the pitched and the unpitched musicians in session 7, p = .389.
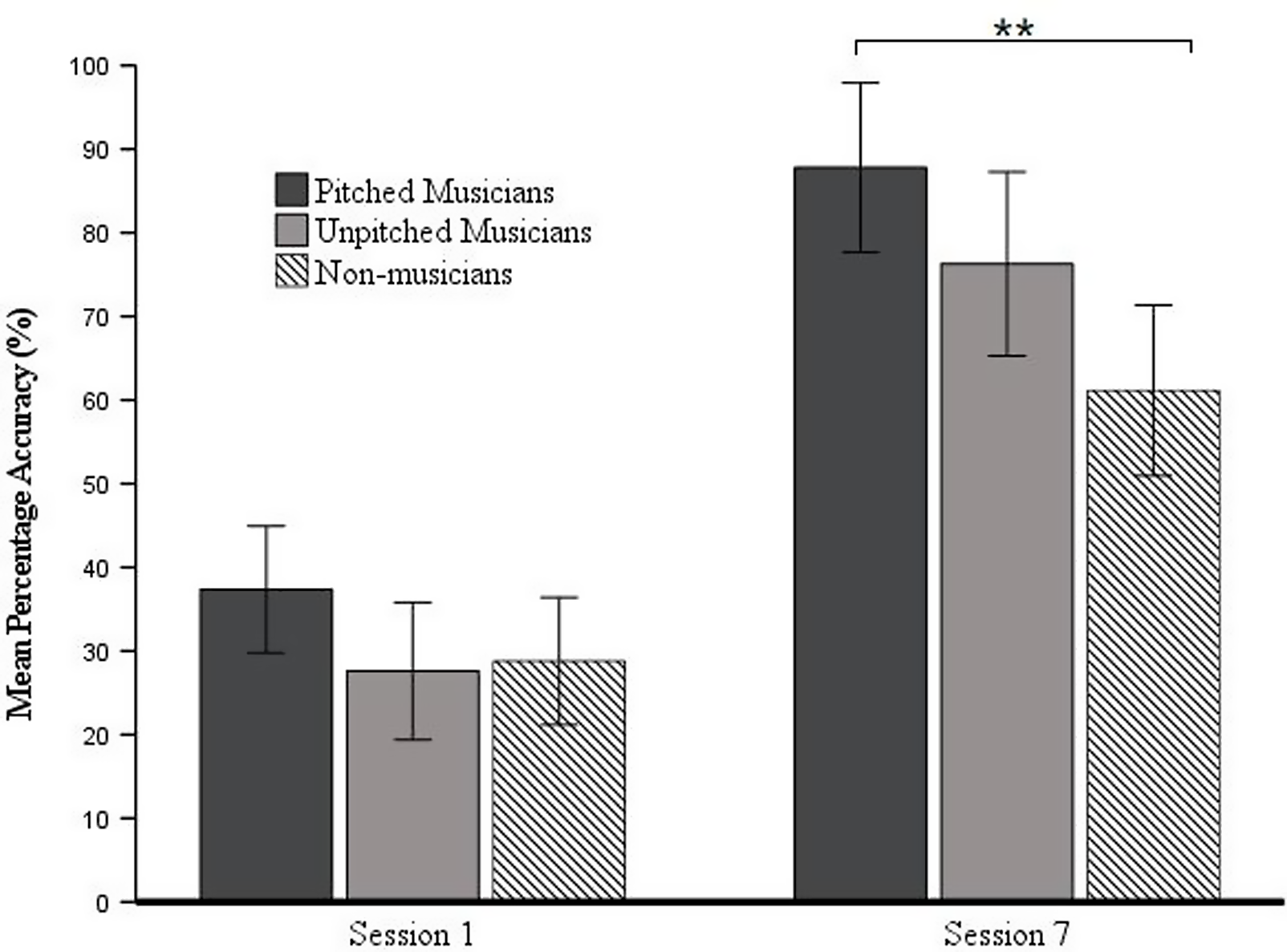
Figure 3. Mean percentage accuracy of each group for tone word identification session 1 and session 7 tests. ** p < .01. The error bars denote 95% confidence intervals.
Supplementary Bayesian analysis
Our central finding is that the pitched musicians outperformed the non-musicians while the unpitched musicians did not. Although this finding is based on statistically significant main effect and interaction, null hypothesis significance testing cannot differentiate between absence of evidence and evidence of absence (Ly & Wagenmakers, Reference Ly and Wagenmakers2022). Specifically, one might argue that the unpitched musicians had outperformed the non-musicians, but the difference was too small to register in ANCOVA. To supplement the main analysis, we adopted Bayesian hypothesis testing which quantifies evidence for both alternative and null hypotheses (Dienes, Reference Dienes2014, Reference Dienes2016; Wagenmakers, Reference Wagenmakers2007). For the tone identification task, we conducted a Bayesian independent samples t test on the overall accuracy with group (unpitched musicians and non-musicians) as the independent variable. The Bayes factor (BF01) was 2.14 with a very small error (0.003%). This indicates that the data are 2.14 times more likely under the null than the alternative hypothesis. The same set of analysis on level tone identification accuracy and contour tone identification accuracy yielded consistent results (annotated.jasp files are uploaded to OSF https://osf.io/f2gsd). Based on Lee and Wagenmakers’ (Reference Lee and Wagenmakers2013) suggested cut-off values of BF01, the analysis yielded anecdotal evidence for equal performance between the unpitched musicians and the non-musicians on tone identification. For the word learning task, we conducted the same set of analysis on the mean accuracy in session 7. The BF01 was 0.999 with 0.004% error, indicating equal evidence for both hypotheses. Since the BF01 has very little implication on word learning, we will only interpret the significant interaction reported in the main analysis. At the very least, Bayesian t test has offered anecdotal evidence for equal performance between the unpitched musicians and the non-musicians on tone identification.
Discussion
The principal finding is that the type of musicianship drives music-to-language transfer. Relative to the non-musicians, only the pitched musicians exhibited a musical advantage in tone identification and word learning. Pitch is an important acoustic correlate of tones. For the pitched musicians, their expertise in musical pitch processing has positively transferred to pitch processing in the linguistic domain. Compared with the non-musicians in the word learning task, the pitched musicians were better able to (i) form long-term phonological representations of segmental and tonal information, (ii) link it with semantic representations, and (iii) use it for lexical access. This indicates that their enhanced tone identification can feed forward to higher-level linguistic processing. Even though the unpitched musicians had extensive music training, they had very little expertise in musical pitch processing. As such, they did not exhibit any musical advantage relative to the non-musicians in tone identification and word learning.
From a theoretical perspective, the above finding is consistent with OPERA’s precision element. For music-to-language transfer to occur, music training must demand higher precision of the acoustic feature at question than speech does (Patel, Reference Patel2011, Reference Patel2014). This is indeed our case. Specifically, pitched music training has a high demand on pitch whereas unpitched music training has a low demand. In line with OPERA, the pitched musicians showed a musical advantage in tone perception and word learning whereas the unpitched musicians did not. Previous studies only investigated the benefit of musicianship as a whole without considering its heterogeneity (e.g., Alexander et al., Reference Alexander, Wong and Bradlow2005; Cooper & Wang, Reference Cooper and Wang2012; Lee & Hung, Reference Lee and Hung2008, Wong & Perrachione, Reference Wong and Perrachione2007). Here, we provide more fine-grained empirical support for OPERA’s precision element. In particular, it is not musicianship per se that matters but the musical experience in using the relevant acoustic feature.
An incidental finding is that music-to-language transfer applies to tone language listeners. Departing from previous studies, our pitched musicians showed a musical advantage despite their L1 tone language background (Cooper & Wang, Reference Cooper and Wang2012; Maggu et al., Reference Maggu, Wong, Liu and Wong2018). The discrepancy may be due to coarse versus nuanced classifications of musicianship. While the previous studies included a great variety of pitched instrumentalists and percussionists in their musician group, our study divided musicians into pitched and unpitched musicians. Furthermore, we only included pianists and violinists in our pitched musician group to control for within-group differences (Parker, Reference Parker1983). Extending the previous studies, our results suggest that tone language experience does not limit music-to-language transfer as long as the musical expertise is relevant (e.g., pitched musicianship) to the speech information (e.g., tones). Since the preponderance of related studies focused on East Asian tone languages, Creel et al. (Reference Creel, Obiri-Yeboah and Rose2023) advocated the need to test African tone languages such as Akan. We believe that our findings can apply to speakers of any tone language as long as pitched musicianship enhances their musical pitch sensitivity.
Methodologically, our findings reflect the importance to consider the type of musical experience when studying music-to-language transfer. Our central finding is that not all types of musicianship can benefit tone perception. Having a heterogenous group of musicians may mask the music-to-language transfer that could have otherwise manifested in a homogenous sample (e.g., Cooper & Wang, Reference Cooper and Wang2012; Maggu et al., Reference Maggu, Wong, Liu and Wong2018). Likewise, pitch expertise alone may not benefit speech timing aspects such as voice onset time (Lisker & Abramson, Reference Lisker and Abramson1964; Mohajer & Hu, Reference Mohajer and Hu2003; Lin & Wang, Reference Lin and Wang2011). Thus, it is also possible that percussion-based instruments with a high rhythmic demand can enhance the discrimination of aspirated and unaspirated consonants. A nuanced grouping of musicians can certainly help elucidate the specificity of music-to-language transfer.
This study’s correlational nature inherently limits its causal inference (Schellenberg, Reference Schellenberg2015). Yet, having the groups predefined ensured the musicians have long-term musical experience for studying music-to-language transfer (Patel, Reference Patel2011). In our study, the musicians and non-musicians matched on cognitive factors such as working memory and non-verbal intelligence. To be empirically stringent, we explicitly controlled for them in the analyses. Nevertheless, our non-verbal intelligence task had a fair internal consistency, so we encourage future studies to adopt a more reliable measure. Given the small population size of unpitched musicians in Hong Kong, it was impractical to match their music onset age with the pitched musicians. To our knowledge, there is no direct evidence suggesting that the onset age of musical training influences tone perception or tone word learning ability (Liang & Du, Reference Liang and Du2018; Vaquero et al., Reference Vaquero, Hartmann, Ripollés, Rojo, Sierpowska, François, Càmara, van Vugt, Mohammadi, Samii, Münte, Rodríguez-Fornells and Altenmüller2016). That said, we would encourage future research to match the music onset ages of musician groups as far as practical.
In conclusion, pitched musicians but not unpitched musicians enjoy a unique musical advantage in tone identification and word learning. This suggests that music-to-language transfer hinges on the type of musicianship rather than musicianship per se. From a theoretical perspective, our findings (i) support the precision element of OPERA and (ii) suggest that researchers should consider the heterogeneity of musicianship when studying music-to-language transfer. Of note, our study focuses on musical advantage relative to non-musicians, so a direct comparison between pitch and unpitched musicians is not of key interest. We believe that the lack of significant difference between the pitched and the unpitched musicians does not undermine the pitched musicians’ advantage over the non-musicians. Instead, it simply implies that pitched musicians exhibit a musical advantage but having that advantage is not enough to outdo unpitched musicians. Practically, the current study suggests the potential use of pitched music training to enhance Cantonese listeners’ non-native tone language learning. Furthermore, our finding indicates that the choice of musical instrument may matter to music-to-language transfer. Lastly, we do not dismiss the possibility of self-selection into different musical instruments based on predisposed pitch acuity (see Schellenberg, Reference Schellenberg, Thomas, Mareschal and Dumontheil2020). Thus, we call for randomized controlled trials to scrutinize the above implications.
Acknowledgements
This work was supported by The University of Hong Kong (Seed Fund for Basic Research for New Staff [202107185043] and Project-Based Research Funding [supported by Start-up Fund]). We appreciate Kusol Im-Erbsin and Ratana-U-Bol for their assistance in stimuli development. We also thank Mei Sze Wong for her technical support in data collection.
Replication package
All study materials, data, and analysis codes are available online at https://osf.io/f2gsd.