There is growing interest in investigating whether contextual variables, such as those representing the built environment, can influence the prevalence of common mental disorders after accounting for individual variables (Reference WeichWeich, 2005). The built environment encompasses all those aspects of our habitat that are created or modified by people, such as homes, schools, parks and roads (Reference Srinivasan, O'Fallon and DearrySrinivasan et al, 2003). Up to now most studies of the built environment and mental illness have used indirect environmental measures such as individuals' perceptions (Reference Ellaway, Macintyre and KearnsEllaway et al, 2001) or aggregated data (Reference RossRoss, 2000; Reference Weich, Holt and TwiggWeich et al, 2003). A few studies have assessed the built environment directly and independently (Reference Weich, Blanchard and PrinceWeich et al, 2002; further details available from the authors), finding little or no variation in prevalence of common mental disorders across small or medium-sized areas (Reference WeichWeich, 2005).
We are unaware of any other Latin American study assessing the contextual effect of the built environment directly and using multilevel models to investigate its association with mental illness. We tested the hypothesis that contextual measures reflecting the quality of the built environment in Santiago, Chile would be associated with common mental disorders independent of individuals' characteristics.
METHOD
Santiago, the capital of Chile, has a population of approximately 6 million people, representing 42% of the total Chilean population. Although Chile is considered to be a middle-income country, with a gross per capita annual income of £2600, it is one of the ten most unequal countries in the world in terms of income (World Bank, 2001). The city is geographically extended, with a mean population density of 392 persons per square kilometre. Geographically the city is neatly compartmentalised according to socio-economic groups, with wealthier people living mainly in the eastern suburbs and the poorest in the southern and northern fringes. Most of the city has basic amenities, including electricity, sanitation and drinkable water, but there are visible differences between more and less affluent sectors. Houses in the wealthier areas are bigger and of better quality. Facilities including better roads and pavements (sidewalks), green areas, overall cleanliness and abundant shops are noticeable. However, crime seems to be present in all sectors, and crime reported to police is especially common in wealthier sectors of the city because criminals target these areas and people report more incidents for various reasons, including private insurance claims.
Sampling strategy
A cross-sectional survey was conducted between 1996 and 1998. The sampling framework was the adult population, restricted to ages 16–64 years, living in private households in the Greater Santiago area. The sampling strategy involved a three-stage design, which included all the 35 boroughs of Greater Santiago, 248 sectors and 4300 households randomly selected with a probability proportional to the size of the sampling units. The number of households within each sector varied from 26 to 5. One person per household was chosen randomly for interview using Kish tables (Reference KishKish, 1965). Individuals from sectors with fewer than five observations were excluded from this analysis. Responses were obtained from 3870 households (response rate 90%). Further details of the sampling design can be found elsewhere (Reference Araya, Rojas and FritschAraya et al, 2001).
Mental health, social and demographic questionnaire
Psychiatric symptoms were assessed with the Revised Clinical Interview Schedule (CIS–R; Reference Lewis, Pelosi and ArayaLewis et al, 1992), a structured and detailed psychiatric interview used extensively in primary care and community studies in Chile and elsewhere. This interview has 14 items assessing the severity of the most common psychiatric symptoms. Each item is given a score, which can then be summed to yield a total score. This continuous measure, reflecting psychiatric symptom severity, was used as our main outcome. The mean weighted κ across all 16 sections of the CIS–R was 0.87 (s.d.=0.08). The validity and reliability of the CIS–R are comparable with the Composite International Diagnostic Interview (CIDI; Reference Lewis, Pelosi and ArayaLewis et al, 1992; Reference Andrews and PetersAndrews & Peters, 1998; Reference Brugha, Meltzer and JenkinsBrugha et al, 2005).
The gender and age of respondents were recorded. Individuals also answered questions regarding their socio-economic status including marital status, educational level and monthly per capita income. The latter was estimated as the sum of net monthly salaries and other income (pensions, dividends, interests or rents) contributed by each household member, divided by the number of residents regardless of age. Interviewers rated the quality of housing through visual inspection as luxurious, good, average, poor or very poor. In order to do this, interviewers used the same criteria and received the same training as for the Chilean national census. The presence of a self-reported physical disease was ascertained from the response to an open-ended question: ‘Do you suffer from any physical problem or disability at present?’. Two independent physicians assessed if the physical problem would require medical attention, in which case they classified it according to the bodily system involved. The self-reported number of friends or relatives who could provide emotional or practical support if needed was determined with a single, open-ended question. The number of alcohol units consumed daily was entered as a continuous variable. All violent crimes reported to the local police station in each one of the sectors were added and this figure was divided by the population of the sector to create an index of violence. The following borough variables were also included: education and health budget per capita, and number of social organisations divided per population size in the borough.
Built Environment Assessment Tool
The Built Environment Assessment Tool (BEAT) was developed to collect data through visual inspection on a wide range of features of small geographical sectors. The sectors included in the assessment were the clusters used in the survey and are those that the Chilean Office of National Statistics (INE) had chosen to maintain updated statistics of the population between censuses. Although the sectors vary in size, they usually represent homogeneous areas of approximately ten small contiguous streets whose maps were prepared by the INE.
The main purpose was to try to create a score that reflected the desirability or attractiveness of a sector. Although many characteristics of a local sector are likely to affect satisfaction, the study focused on those that could be easily and reliably assessed by a walk through the area. Most items were derived from the Residential Environment Assessment Tool (REAT; Reference Dunstan, Weaver and ArayaDunstan et al, 2005) used to measure area characteristics in Wales. Some additional items were included because they were thought to be important locally, such as the presence of stray dogs or bad odours. The final instrument (available from the authors) included items relating to the following characteristics, with the number of items in parentheses: public lighting (2), state of roads (6), sidewalks (4), public green areas (5), green elements on sidewalks and front gardens (4), dirtiness (1), traffic and noise (2), bad odours (1), general maintenance of properties (1), general use of the sector (4), empty sites (2), external beautification (1), presence of homeless people (1), presence of stray dogs (1), access to properties (2), balconies (1), street signs (1), public transport (4), security and safety devices (6), and a list of facilities including:
-
(a) essential facilities: primary and secondary schools, other type of schools, crèches, primary care clinics, hospitals and private clinics;
-
(b) leisure facilities: public gymnasium, swimming pool, football pitch, sports club, cultural centre, library, community centre, corner shops, pharmacies, cinema, theatre, restaurants, coffee shops, bars;
-
(c) Other facilities: petrol station, petrol station shops and kiosks.
Some of the items required dichotomous ratings, for example the presence of lamp-posts; others had a range of possible values that could be ordered from high to low, such as the level of maintenance of front gardens. Ratings for all items were converted into a score between 0 and 1, with the value of 1 always representing either a more desirable feature (e.g. cleaner roads) or more of that particular item (e.g. more essential facilities). For example, the item for ‘beauty of front garden’ was initially coded 1, 2 or 3 and subsequently recoded as 0, 0.5 or 1, with 1 representing ‘very beautiful’ front gardens. The presence of any of the listed facilities in the sector was rated as ‘1’ and individual scores were summed to generate three facility indices. These total scores for the facilities indices were subsequently recoded into three scales with scores ranging from 0 to 1.
Ratings were made for observations of the sector as a whole, for example the extent of street litter within the sector rather than in any particular street. Operational definitions were provided for each item, including a set of photographs to illustrate different degrees of items such as litter. The tool was initially piloted in a few neighbourhoods in Santiago of varying socio-economic status. Raters were university students of architecture and psychology trained over 2 days to ensure consistency in their understanding and ratings of items. During this training, instructions were given and photographs used to discriminate between ratings.
Two independent raters assessed each geographical sector. Each one scored all items, and after the assessment both raters met and agreed on a consensus rating, which is the one used in this study. There were ten pairs of raters in all. The walk-through assessment was undertaken over a period of 30 days in January 2002. All assessments were conducted in the mornings in order to avoid potential differences due to timing, for instance in ratings of noise. Raters were given a map of the sector with clear boundaries and instructions to follow a predetermined route, specified in the map, when walking through the area and to cover all streets in the sector. The assessment of each sector took approximately 60 min to complete. In certain areas it was necessary to arrange for someone to accompany the raters for reasons of safety, but the raters were instructed not to talk about the neighbourhood to anyone until the ratings had been completed.
Statistical analyses
Composite score for the BEAT scale
All variables with 95% or more of respondents in one category were eliminated. Subsequently we performed factor analysis with varimax rotation of all the remaining items to assess if and how these items loaded into common factors. Cronbach's α was estimated for all the items in the scale and for the items within each one of the newly derived factors after the factor analysis. All variables were entered, including the facilities indices, into the factor analysis model and those with loadings lower than 0.4 after varimax rotation or high uniqueness values were also excluded. The scores of each item (0, 1) within a factor were added to generate a total composite score for the factor. A higher score in the factor reflected a more attractive area. We generated an alternative total score applying weights to the individual item scores according to the loadings of that particular item in the factor analysis. The total unweighted and weighted scores for each factor were compared using correlation coefficients to explore for possible differences, depending on the methods used to estimate total factor scores. Finally, for all regression models, the four factor scores were rescaled to a minimum of 0 and a maximum of 10 to facilitate comparison of effect sizes between the factors.
Testing associations across levels
Associations between sector (level 2) and borough (level 3) exposures and individual CIS–R scores were investigated using multilevel regression models (MLwiN version 2.02, Institute of Education, University of London, UK, and Stata Release 9), before and after adjusting for individual (level 1) predictor variables. All analyses excluded individuals from sectors with fewer than five replies and those with incomplete data for any of the individual, sector-level and borough-level variables to be included in the models. We did this because we felt that using data from only a handful of individuals might not offer a fair representation of the sector. Individuals included and excluded from the analyses were compared in terms of age, gender and socio-economic characteristics. The modelling strategy consisted of first fitting a simple variance components null model to quantify the three components of residual variation in CIS–R score as a continuous variable: borough, sectors and individuals. Analyses involving CIS–R score as a continuous outcome were based on a normally distributed multilevel model using the observed and log-transformed CIS–R scores. Estimation in all models was based on iterative generalised least squares. As the associations between individual-level variables and mental health are already well known, the primary aim of this study was to investigate the effects of the local and wider neighbourhood on mental health using the CIS–R total scores, after taking individual factors into account. Therefore the modelling strategy we adopted was to investigate sector-level predictors of CIS–R first, then to add individual and finally borough-level variables to the model and to note changes in the components of variance and coefficients for the sector-level factors. We investigated whether there were any differential associations between factor 1 and CIS–R for categories of selected individual variables by fitting appropriate interaction terms in the regression models.
RESULTS
Development of the Built Environment Assessment Tool
Twenty-seven items were removed from the original list for two reasons: 14 did not show sufficient ability to discriminate (95% or more of the answers fell into one category) and the remainder had loadings below 0.4 after varimax rotation or high uniqueness values so that they did not fit well with any of the factors. Among the items left out were several questions on the type of roads, external beautification of properties, parking on sidewalks, predominant type of properties, protection on balconies, security fences, presence of taxis, bad odours, the size of green areas on sidewalks, and presence of vagrants. Twenty-five items remained, including three representing the sum of the list of facilities. These were grouped into four main factors:
-
(a) general quality of the area;
-
(b) facilities, noise and traffic in the area;
-
(c) public green areas;
-
(d) empty sites.
These four factors all had eigenvalues over 1 and together explained 90% of the total variance (Table 1). The mean Cronbach's α for the items in the scale was 0.87. There were small differences between these values of α and those generated using only the items within each factor. Lower α values for the items contained in factors with lower eigenvalues are explained because the first factor with the highest eigenvalue contains the most items, thus making the greatest contribution to the overall variation in scores. Kappa coefficients for items between pair of interviewers fluctuated from 0.69 to 0.92, with 78% of the estimated κ coefficients above 0.85 and full agreement for 70% of the items. Simply summing items to get a total factor score assumes equal weighting of each item and that ‘non-loading’ items are not important. For this reason we compared weighted (according to eigenvalues) and unweighted scores for each of the factors. We found high correlations between these two different ways of scoring the factors (correlation coefficients for factors 1, 2, 3 and 4 were 0.99, 0.87, 0.92 and 0.88 respectively), and therefore we decided to use the simple, unweighted scores in all analyses. Correlation coefficients between the four sector-level factors are presented in Table 2.
Table 1 Factor analysis of items included in the Built Environment Assessment Tool

Items | Factor number | Factor 1 General quality | Factor 2 Facilities | Factor 3 Green areas | Factor 4 Empty sites |
---|---|---|---|---|---|
Proportion variance, % | 50.1 | 20.3 | 12.6 | 8.2 | |
Eigenvalues | 6.2 | 2.5 | 1.5 | 1.0 | |
Loadings presented after varimax rotation | |||||
Width of sidewalks | 1 | 0.45 | –0.24 | –0.18 | 0.29 |
General maintenance of sidewalks | 1 | 0.69 | –0.012 | 0.07 | –0.04 |
Additional features on sidewalks (wheelchairs, bicycles, etc.) | 1 | 0.71 | –0.02 | 0.07 | 0.12 |
State of front gardens | 1 | 0.70 | 0.03 | 0.03 | –0.01 |
Trees on sidewalks | 1 | 0.54 | –0.07 | –0.01 | 0.16 |
Size of trees on sidewalks | 1 | 0.44 | –0.17 | –0.13 | 0.16 |
Green area on sidewalk | 1 | 0.74 | –0.15 | –0.04 | 0.27 |
Dirtiness of street | 1 | 0.63 | –0.00 | 0.18 | –0.08 |
General maintenance of properties | 1 | 0.67 | –0.11 | 0.17 | –0.07 |
Type of properties | 1 | 0.41 | –0.14 | 0.08 | 0.18 |
Stray dogs | 1 | 0.57 | –0.08 | 0.19 | 0.14 |
Signs for orientation | 1 | 0.41 | –0.37 | –0.10 | 0.05 |
Other public signs | 1 | 0.67 | –0.16 | 0.07 | 0.12 |
Security badges on houses | 1 | 0.41 | –0.29 | –0.24 | 0.25 |
Guards | 1 | 0.42 | –0.24 | –0.08 | 0.33 |
Level of traffic | 2 | –0.05 | 0.80 | 0.01 | –0.17 |
Noise of traffic | 2 | 0.02 | 0.71 | –0.02 | –0.06 |
Bus stop | 2 | 0.08 | 0.54 | –0.18 | 0.09 |
Essential facilities | 2 | 0.27 | 0.41 | –0.18 | –0.03 |
Leisure facilities | 2 | 0.12 | 0.52 | –0.15 | –0.01 |
Other facilities | 2 | 0.14 | 0.47 | –0.11 | 0.16 |
Public green areas | 3 | –0.11 | –0.12 | 0.78 | 0.12 |
State of public green areas | 3 | 0.28 | –0.10 | 0.74 | 0.09 |
Presence of empty sites | 4 | 0.08 | 0.03 | 0.08 | 0.80 |
Empty sites occupied illegally | 4 | 0.11 | 0.07 | 0.08 | 0.82 |
Table 2 Pearson correlation coefficients of the scores in the four derived factors of the Built Environment Assessment Tool

General quality | Facilities | Green areas | Empty sites | |
---|---|---|---|---|
General quality | 1.00 | |||
Facilities | 0.11 | 1.00 | ||
Green areas | 0.31 | –0.03 | 1.00 | |
Empty sites | 0.10 | 0.07 | 0.17 | 1.00 |
Characteristics of the sample surveyed
A total of 3870 interviews were completed to give a response rate of 90%. These 3870 individuals clustered into 248 sectors and 35 boroughs. A total of 488 individuals (12.6%) were excluded because of missing data or because they lived in a sector with fewer than five respondents, leaving 3382 observations from 210 sectors within 31 boroughs for analysis. Excluded individuals were no different to those included in terms of age (P=0.56), gender (P=0.17) or marital status (P=0.59), but had lower median income (in Chilean pesos, CLP62 500 v. CLP100 000, P<0.0001), were less likely to be educated to university level (20 v. 36%, P<0.001), were more likely to live in very poor or poor quality housing (22 v. 15%, P<0.001), have fewer supportive individuals (3.7 v. 4.2, P=0.01) and have lower alcohol consumption (1.5 v. 1.8, P=0.005). Excluded individuals also had higher mean CIS–R scores (8.5 v. 7.2, P<0.001). There was no evidence that excluded sectors were any different from those included in terms of the four factors generated from the BEAT scores or violent incidents reported to police. The number of individuals per sector in the final data-set ranged from 5 to 26 and the number of sectors per borough from 2 to 26. Characteristics of the individuals, sectors and boroughs are presented in Table 3.
Table 3 Characteristics of individuals, sectors and boroughs in the sample
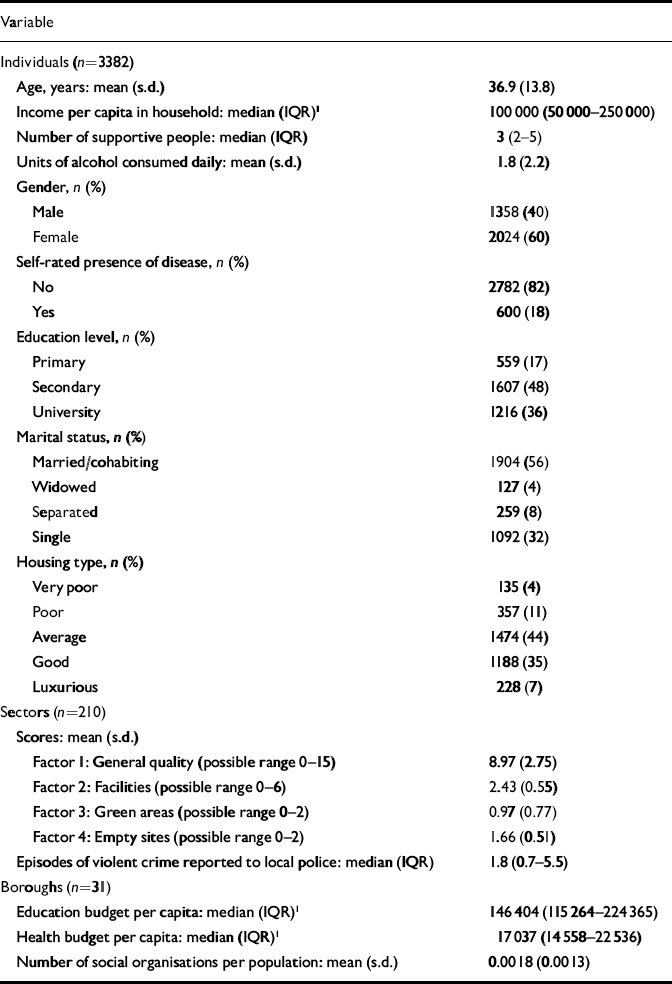
Variable | |
---|---|
Individuals (n=3382) | |
Age, years: mean (s.d.) | 36.9 (13.8) |
Income per capita in household: median (IQR) 1 | 100 000 (50 000–250 000) |
Number of supportive people: median (IQR) | 3 (2–5) |
Units of alcohol consumed daily: mean (s.d.) | 1.8 (2.2) |
Gender, n (%) | |
Male | 1358 (40) |
Female | 2024 (60) |
Self-rated presence of disease, n (%) | |
No | 2782 (82) |
Yes | 600 (18) |
Education level, n (%) | |
Primary | 559 (17) |
Secondary | 1607 (48) |
University | 1216 (36) |
Marital status, n (%) | |
Married/cohabiting | 1904 (56) |
Widowed | 127 (4) |
Separated | 259 (8) |
Single | 1092 (32) |
Housing type, n (%) | |
Very poor | 135 (4) |
Poor | 357 (11) |
Average | 1474 (44) |
Good | 1188 (35) |
Luxurious | 228 (7) |
Sectors (n=210) | |
Scores: mean (s.d.) | |
Factor 1: General quality (possible range 0–15) | 8.97 (2.75) |
Factor 2: Facilities (possible range 0–6) | 2.43 (0.55) |
Factor 3: Green areas (possible range 0–2) | 0.97 (0.77) |
Factor 4: Empty sites (possible range 0–2) | 1.66 (0.51) |
Episodes of violent crime reported to local police: median (IQR) | 1.8 (0.7–5.5) |
Boroughs (n=31) | |
Education budget per capita: median (IQR) 1 | 146 404 (115 264–224 365) |
Health budget per capita: median (IQR) 1 | 17 037 (14 558–22 536) |
Number of social organisations per population: mean (s.d.) | 0.00 18 (0.00 13) |
IQR, interquartile range
1. Chilean pesos
Variance components null model for common mental disorder
Mean CIS–R score for the total sample was 7.19 (s.d.=8.00, range 0–49). We estimated that approximately 5.6% (95% CI 1.8–9.4) of the residual variation in total CIS–R score lies at the borough level, 3.8% (95% CI 1.8–5.7) at the sector level and 90.6% (95% CI 86.2–95.1) at the individual level (Table 4). In view of the non-normal distribution of CIS–R scores we also undertook all multilevel modelling using log-transformed scores and the results in all these models were almost identical to those presented here (further details available from the authors).
Table 4 Components of variance in total Revised Clinical Interview Schedule score (as a continuous variable) at the individual, sector and borough level: multilevel modelling

Null model | Model 1 (null+sector variables) 1 | Model 2 (model 1+individual variables) 2 | Model 3 (model 2+borough variables) 3 | |
---|---|---|---|---|
Variance (s.e.) | ||||
Level 3 (borough) | 3.59 (1.25) | 0.08 (0.21) | 0.00 (0.00) | 0.00 (0.00) |
Level 2 (sector) | 2.42 (0.64) | 2.15 (0.60) | 0.51 (0.36) | 0.50 (0.36) |
Level 1 (individual) | 58.28 (1.46) | 58.33 (1.46) | 51.29 (1.28) | 51.29 (1.28) |
1. Sector variables in model 1 were the four derived factors from factor analysis
2. Individual variables were age, gender, presence of disease, income, education, marital status, housing type, number of supportive individuals and alcohol use. Model 2 also included the sector-level variable episodes of violent crime reported to local police
3. Borough variables were education budget per capita, health budget per capita and number of social organisations
Effect of including individual, sector and borough characteristics in the model
Sector-, individual- and borough-level fixed effects were added to the null model in a cumulative manner (Table 4). Inclusion of the sector-level exposures reduced the total residual variation. The estimated percentage of residual variation at borough level decreased to 0.13%, whereas the percentage residual variation at sector (3.55%) and individual (96.32%) levels remained similar and increased respectively. Additional inclusion of individual-level variables reduced the overall variance further, with none of the residual variation now explained at the borough level. Estimates remained unchanged after the addition of borough-level variables. Estimated associations between sector-level factors and mental health were therefore based on a simpler, two-level model that included only sector (level 2) and individual (level 1) variables.
Effect of neighbourhood quality on common mental disorders
Table 5 shows the crude and adjusted associations between each of the sector-level factors (rescaled so that the possible range for each is 0–10) and CIS–R total score. After adjusting for other sector-level and individual-level variables, factor 1 (overall quality of the built environment) was inversely associated with total CIS–R score: that is, there was strong evidence that individuals living in sectors with more desirable features such as better roads or more green areas had better mental health, after taking into account individual characteristics. There was also a significant association with factor 4 in the adjusted model only, but this was in the opposite direction; higher factor scores were associated with higher CIS–R scores.
Table 5 Association between sector-level factors according to Built Environment Assessment Tool and Revised Clinical Interview Schedule total score as a continuous variable: two-level regression models

β (95% CI) | Adjusted P | |||
---|---|---|---|---|
Unadjusted | Adjusted 1 | |||
Sector level | ||||
Factor 1 | –0.96 (–1.14 to –0.77) | –0.30 (–0.49 to –0.11) | 0.002 | |
Factor 2 | –0.18 (–0.73 to 0.37) | –0.04 (–0.38 to 0.31) | 0.84 | |
Factor 3 | –0.14 (–0.25 to –0.03) | –0.01 (–0.09 to 0.06) | 0.77 | |
Factor 4 | 0.12 (–0.04 to 0.28) | 0.17 (0.06 to 0.28) | 0.002 | |
Individual level | ||||
Age | –0.02 (–0.03 to 0.004) | –0.08 (–0.10 to –0.06) | <0.001 | |
Female gender | 3.03 (2.50 to 3.55) | 3.16 (2.61 to 3.70) | <0.001 | |
Income 2 | –0.03 (–0.04 to –0.03) | –0.01 (–0.02 to –0.005) | 0.001 | |
Education | ||||
Primary | Reference | |||
Secondary | –2.24 (–3.00 to –1.49) | –1.42 (–2.16 to –0.67) | ||
University | –4.41 (–5.22 to –3.59) | –1.86 (–2.74 to –0.97) | <0.001 3 | |
Marital status | ||||
Married | Reference | |||
Widowed | 0.37 (–1.02 to 1.77) | 0.29 (–1.06 to 1.64) | ||
Separated | 2.36 (1.36 to 3.37) | 1.53 (0.58 to 2.47) | ||
Single | –0.60 (–1.18 to –0.02) | –1.26 (–1.90 to –0.61) | <0.001 3 | |
Housing | ||||
Very poor | Reference | |||
Poor | –2.06 (–3.60 to –0.53) | –1.58 (–3.02 to –0.14) | ||
Average | –3.79 (–5.16 to –2.43) | –2.51 (–3.81 to –1.22) | ||
Good | –6.40 (–7.80 to –5.01) | –3.37 (–4.76 to –1.98) | ||
Luxurious | –8.98(–10.67 to –7.30) | –4.25 (–6.03 to –2.48) | <0.001 3 | |
Social support (no. of supportive people) | –0.33 (–0.40 to –0.27) | –0.24 (–0.30 to –0.18) | <0.001 | |
Alcohol use | 0.11 (–0.01 to 0.23) | 0.39 (0.27 to 0.52) | <0.001 | |
Disease | 3.88 (3.20 to 4.56) | 3.75 (3.08 to 4.41) | <0.001 |
1. Adjusted for other sector-level factors and sector-level variable episodes of violent crime reported to local police, and individual-level variables age, gender, presence of disease, income, education, marital status, housing type, number of supportive individuals and alcohol use
2. Per 10 000 pesos
3. Value from Wald test
We tested interactions between factor 1 and the following individual variables: gender, income and education. The only significant interaction was for gender and factor 1 (–0.32, 95% CI –0.58 to – 0.05, P=0.02) in which male respondents living in less desirable areas had significantly lower CIS–R scores than female respondents. No significant interaction was found for income (0.004, 95% CI –0.001 to 0.008, P=0.10) or education (secondary, 0.23, 95% CI –0.26 to 0.72; university, 0.16, 95% CI –0.35 to 0.68; overall χ2=0.91, d.f.=2, P=0.63).
Although our primary interest was to investigate the association of these factors with mental health, which was best represented by the continuous distribution in CIS–R total scores, we also explored associations with the most common ICD–10 disorders (World Health Organization, 1992), anxiety and depressive disorders, using logistic regression models. There were 154 (4.6%) cases of depression and 309 (9.1%) of anxiety. There was no evidence of any association with depression for factors 1, 2, or 4 (factor 1, OR=1.00, 95% CI 0.87 to 1.13, P=0.92; factor 2, OR=0.91, 95% CI 0.71 to 1.17, P=0.46; factor 4, OR=1.05, 95% CI 0.99 to 1.13, P=0.16) but some evidence of an association with factor 3, representing public green areas (OR=0.94, 95% CI 0.90 to 0.99, P=0.01). For anxiety disorders there was some evidence of an association with factor 4 (empty sites, OR=1.05, 95% CI 1.00 to 1.11, P=0.04), but no association was detected for the other factors (factor 1, OR=0.94, 95% CI 0.86 to 1.03, P=0.20; factor 2, OR=1.01, 95% CI 0.85 to 1.21, P=0.87; factor 3, OR=0.99, 95% CI 0.95 to 1.02, P=0.38).
DISCUSSION
This is the first large population-based study of common mental disorders and the built environment of small geographical sectors of a Latin American city using a directly assessed contextual measure and multilevel modelling in the analysis. We found strong evidence of an association between the quality of the built environment in these sectors and common mental disorders, before and after adjusting for individual variables. However, in line with previous reports, the contribution of these sectors to the total variance in common mental disorders was small and most of it was explained by individual factors. None the less, these results represent probably some of the most persuasive evidence found so far to establish an association between the quality of our surrounding built environment and the presence of common mental disorders.
The Built Environment Assessment Tool
We developed and tested a quick and reliable method to assess the built environment using a walk-through method. The great majority of the items reflected the built environment, but there were a few –such as the presence of stray dogs –that represented the observable residential environment rather than something built. We had previous experience with developing a similar instrument for a study in South Wales (Reference Dunstan, Weaver and ArayaDunstan et al, 2005) and we studied carefully other similar instruments (Reference Sampson, Raudenbush and EarlsSampson et al, 1997; Reference Cohen, Spear and ScribnerCohen et al, 2000; Reference Weich, Blanchard and PrinceWeich et al, 2002; Reference Hembree, Galea and AhernHembree et al, 2005). Our measure showed good interrater reliability and internal consistency; in the absence of a gold standard, however, it is difficult to assess its criterion validity. Overall, the psychometrics of this tool are comparable with those found for the two similar tools developed in the UK (Reference Weich, Burton and BlanchardWeich et al, 2001; Reference Dunstan, Weaver and ArayaDunstan et al, 2005).
Variance in mental disorders according to geographical aggregation
Studies in Western countries using multilevel models to estimate area-level variation in common mental disorders have found little or no variation at higher levels of aggregation, after accounting for individual differences (Reference WeichWeich, 2005). The contribution of smaller area effects to the total variance in common mental disorders usually fluctuates between 0.5% and 4% before adjusting for individuals' characteristics, and drops to levels between 0% and 1% after adjustment (Reference WeichWeich, 2005). Our findings are in keeping with the higher end of previous estimates; we found that 3.8% of the variance in common mental disorders was explained at the small sector level in the unadjusted models, reducing to nearly 1% in the adjusted models.
As eloquently argued by Weich (Reference Weich2005), there may be a number of reasons to explain this lack of positive findings. For instance, sectors that are large or heterogeneous tend to yield negative results. However, in a previous UK study in South Wales using small geographical units (postcodes with approximately 150 people) we also found little variation at this level (further details available from the authors). It is possible that using geographical units identified on the basis of an arbitrary geographic classification, which might not reflect neighbourhood unity, may influence the results. In this respect, Reijneveld et al (Reference Reijneveld, Verheij and de Bakker2000) found that the clustering of common mental disorders was higher at neighbourhood level (sectors with similar types of building delineated by natural boundaries) than at postcode level using arbitrary geographical boundaries. We tried to deal with both of these possibilities, so we used small geographical sectors of approximately 300 people that were sufficiently homogeneous in terms of their neighbourhood. Our study did not measure the outcome (CIS–R) aggregated at household level and so it is possible that some of the variance found at higher (sector) or lower (individual) levels might reflect variance present at household level. In our previous study in Wales we found that 37% (95% CI 27–49) of variance existed at household level (further details available from the authors). Although the CIS–R variance at borough level appears greater than at sector level in Table 4, the confidence intervals of these estimates (5.6%, 95% CI 1.8–9.4% at the borough level and 3.8%, 95% CI 1.8–5.7 at the sector level) show that one cannot reach this conclusion. More importantly, once adjustment for other variables are introduced this borough variance comes close to nil but the sector variance remains only slightly attenuated. Yet the variance we found at borough level in adjusted models is considerable in comparison with other studies. The most likely explanation is that Santiago, like other cities in Latin America, is quite compartmentalised in terms of the quality and socio-economic status of the geographical areas, with little variation within but more variation between boroughs.
Previous studies have been criticised because they tend to rely entirely on brief psychiatric self-reported questionnaires to measure the outcome. Our study used a detailed structured psychiatric interview to overcome this limitation. So, as it stands, we have to conclude that there seems to be little variation in prevalence variation in prevalence of common mental of common mental disorder explained at area level and much of this variance resides at individual level. However, even if small sectors contribute little to this overall variance, is it still possible that some features of these areas may be associated with common mental disorders?
Quality of residential environment and common mental disorder
There have been only a handful of mental health studies that have used truly contextual and independent measures of the built environment throughout the world. In the UK there have been only two such studies. Weich et al (Reference Weich, Blanchard and Prince2002) found a significant association between the prevalence of depression and properties with predominantly deck access (OR=1.28, 95% CI 1.03–1.58) and of recent construction (OR=1.43, 95% CI 1.06–1.91). It is worth noting that this was a cross-sectional study in which no multilevel modelling was used in the analysis (Reference Weich, Blanchard and PrinceWeich et al, 2002). Using a similar contextual assessment of the built environment as in the study we report here and multilevel modelling in the analysis we did not find any significant association between the total score of an index depicting quality of the residential environment and the prevalence of common mental disorders in our study in South Wales (further details available from the authors). It must also be borne in mind that both Weich et al (Reference Weich, Blanchard and Prince2002) and our previous study used brief questionnaires to measure mental disorder and studied smaller samples than in this study (Weich et al, 76 sectors, n=1887; our previous study, 51 sectors, n=1500). A larger number of sectors could help to improve the accuracy of the estimates and provide greater power to test smaller effects.
We found strong evidence of an association (P⩽0.05) between two factors of our index of quality of the built environment (BEAT) and common mental disorders, after adjusting for individual differences. These factors represented almost two-thirds of the total variance in the quality of the built environment and thus one can confidently conclude that they are good indicators of the built environment in the city of Santiago. We used a similar method as in the South Wales study (REAT; Reference Dunstan, Weaver and ArayaDunstan et al, 2005), but there were some differences that might help explain the discrepant results. The REAT assessed mainly the more private built environment such as houses, gardens or housing density. The BEAT assessed extensively other aspects of the built environment such as roads, pavements and public facilities. The REAT provided a total score reflecting the quality of the residential environment, whereas we used four factors with their corresponding individual scores. Although it may seem intuitive that a better built environment might help us feel better, the precise mechanism by which the built environment influences our mental health is still a matter of conjecture.
Why did only two factors show significant associations in our study? The first factor represented the largest proportion of the variance and it was the most comprehensive indicator of the quality of the neighbourhood and built environment. Although the relative contribution of this factor to change in CIS–R score is approximately ten times smaller than that associated with individual variables such as being female, it is a factor amenable to change and it is widely spread. Interestingly, the only significant interaction across levels showed that women were more affected (higher CIS–R scores) than men when living in less desirable areas. This would be in keeping with our hypotheses because the women –especially those who did not work outside the home –probably spent more time in the areas studied than men and were therefore more exposed.
We found, rather surprisingly, that factor 4 (empty sites) was associated in the opposite direction: fewer sites were associated with higher CIS–R scores. However, factor 4 was not a key indicator of the area environment, contributing only 8% of the variance in our factor analysis, and in the unadjusted model (see Table 5) this association was not significant at a 5% level. Our assumption was that fewer empty sites, especially if they were unoccupied, would be a good feature of the sector; however, it is possible that our assumptions were baseless and that empty sites in Santiago may not represent abandoned, derelict places where rubbish accumulates, as in other settings. We expected that factor 2, representing 20% of the total variance, would be significantly associated with CIS–R scores. However, this factor was a rare combination of essential and leisure facilities and noise and traffic in the area. Our assumption here was that an increased number of facilities would represent an asset for the locality, but it may be that more facilities bring more noise and traffic to the area and that this is more important. Nevertheless, overall it is reassuring that the strongest and clearest association is for the best and most comprehensive indicator of the quality of the built environment. When we explored associations of these factors with ICD–10 categorical disorders the results were puzzling. We found that there was no association between these disorders and factor 1, representing the overall quality of the neighbourhood. Even more surprisingly, individuals who were depressed were more likely to live in areas with more public green areas, an association that we did not find when using CIS–R total scores. More in keeping with the other results, individuals living in areas with fewer empty sites were less likely to have an anxiety disorder, an association that we found for CIS–R total scores but in the opposite direction. It is difficult to find a reasonable explanation for these disparate findings, especially for those related to depressive disorders. However, our interest was to focus on population changes in mental health (symptom scores) rather than concentrate on specific subgroups, mainly because the former approach would be more informative for public health decision-makers (Reference RoseRose, 1993).
Santiago is fairly well compartmentalised according to socio-economic grouping. Wealthy people live in areas completely removed from the areas where poorer people live, something not always found in UK cities with a much more mixed socio-economic distribution within geographical sectors. This clear and distinct geographical distribution might have helped reduce ‘contamination’ and accentuated the differences between the sectors selected in our clustered sampling strategy. We selected the sectors in our sample to represent an adequate spread of neighbourhood deprivation, so we expected this would ensure an adequate spread of residential quality. We think that a drop of one point in the total CIS–R score attributable to living in the sectors with better built environment quality is a meaningful change, bearing in mind the large proportion of people who might potentially benefit from interventions to reduce this difference. When a common threshold of common mental disorder caseness with the CIS–R (⩾12) is used, those living in areas with better built environment are approximately 20% less likely to meet caseness criteria than those living in areas with poorer built environments. Leventhal & Brooks-Gunn (Reference Leventhal and Brooks-Gunn2003) found that families who moved from a very poor neighbourhood to a non-poor neighbourhood showed better mental health than control families who did not move. A similar issue related to mobility is whether or not individuals with poorer mental health may selectively move to more deteriorated areas rather than poorer areas making individuals unhappier (causation v. selection). Unfortuantely the design of our study does not allow adequate testing of this theory, and the stability of residence was not recorded.
Strengths and limitations
Our study benefited from using a truly contextual and independent assessment of the built environment rather than measures derived from aggregating individual data. The small size of our surveyed areas ensured reasonable homogeneity within sectors. We used multilevel modelling to account for the hierarchical structure of the data. The study was large but its unique setting means its results are not necessarily generalisable to other cities throughout the world. Our independent measures at the highest level concentrated on the physical aspects of the environment, mostly because we thought that these could be measured reliably. Of course, the quality of the built environment also reflects something of the psychosocial environment, but we did not include these aspects in this study. This study should be taken as an invitation to explore this field further.
The assessment of the geographical sectors was undertaken almost 4 years after we finished the survey of the individuals. Although it is possible that the conditions in those neighbourhoods could have changed in the interim period, we did not find evidence that sectors had experienced major structural changes during the interval according to a survey of local government authorities (Secretaría Regional Ministerial de Planificación y Coordinación, n, 2005). A few sectors with a larger proportion of socially disadvantaged individuals were excluded from the analysis. The main reason for sector exclusion was the small number of people in the sector or the lack of data. Common mental disorders are more prevalent among socially deprived individuals; thus our estimates may be an underrepresentation of the true association. Finally, this is a cross-sectional study and as such we cannot infer the direction of causality. Equally, this kind of design cannot account for factors related to selective migration or population instability.
In conclusion, measuring the impact of the quality of neighbourhoods on mental health and understanding the complex interrelationships between individuals' characteristics and their local environment are challenges that should be confronted, so that appropriate and effective interventions can be implemented to improve the mental health of the population.
Acknowledgements
We thank the School of Architecture, Universidad Central de Chile, Santiago, for their support and cooperation in carrying out this study, and to the European Union for funding the Santiago Mental Disorder Survey. This research was supported by a grant from the Wellcome Trust.
eLetters
No eLetters have been published for this article.