Whole-genome sequencing (WGS) for infection prevention has traditionally been used in reaction to a suspected outbreak, usually at the end of an investigation to confirm or to refute the presence of an outbreak. In contrast, WGS surveillance of selected healthcare-associated pathogens regardless of whether an outbreak is suspected can be used to identify outbreaks that are not detected by traditional hospital epidemiologic methods. High costs and needed infrastructure for implementation have been historic barriers to widespread use of WGS surveillance. However, the cost of WGS has fallen, and the expansion of genomic surveillance due to coronavirus disease 2019 (COVID-19) may enable healthcare institutions to establish WGS surveillance programs for other pathogens. Additionally, our work and studies from Australia have identified cost benefits to implementing a WGS surveillance program with effective intervention. Reference Gordon, Elliott and Forde1
Although WGS surveillance is effective in identifying transmission, it does not provide information on the responsible transmission route, which is crucial for interrupting an outbreak. Traditional epidemiologic methods for identifying where transmission occurs have relied on geotemporal clustering within the hospital, which is inadequate for identifying more complex patterns of transmission. Reference Sherry, Lee and Gorrie2,Reference Ward, Hoss and Kolde3 Automated analysis of electronic health records (EHRs) creates an opportunity to use machine learning or statistical modeling approaches for determining the outbreak transmission routes identified by WGS surveillance. Reference Sundermann, Babiker and Marsh4–Reference Miller, Chen and Sundermann8 These automated approaches would assist hospital infection prevention departments by providing systematic methods to investigate outbreaks and identify transmission routes.
In this systematic review, we provide a summary of prior studies utilizing WGS surveillance in healthcare settings for outbreak detection, as well as the use of machine learning and statistical modeling technologies to identify transmission routes. The purposes of this review were to summarize the current literature in this field, to identify barriers to widespread implementation, and to synthesize current knowledge on this topic to help guide decision making about implementation of WGS surveillance.
Methods
Two search terms were utilized in PubMed from inception until March 15, 2021 (Figs. 1 and 2). The WGS surveillance terms “(whole genome sequenc*) AND (surveillance OR routine) AND (healthcare OR hospital) AND transmission” returned 767 results. Article abstracts were screened to exclude studies that were solely community based, non–infection related, utilized non-WGS methods (eg, older molecular subtyping methods such as pulsed-field gel electrophoresis), or only utilized reactive WGS in response to suspected outbreaks. Genomic and epidemiologic data on organisms, number of isolates sequenced, percentage of isolates that were related, number of outbreaks, and epidemiological links were extracted and summarized. Articles were excluded if the data were not sufficiently detailed for extraction.
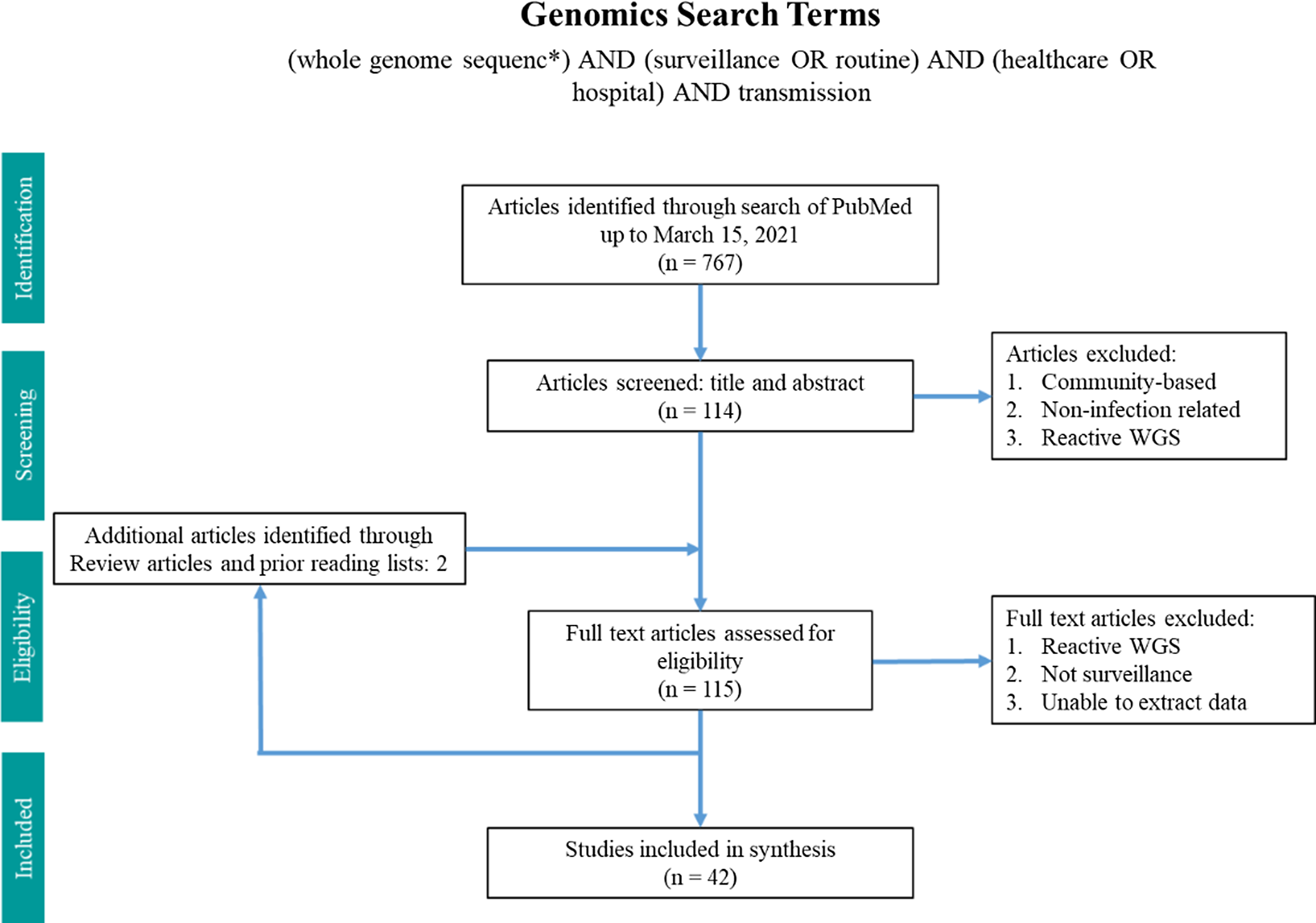
Fig. 1. Search terms in PubMed for whole-genome sequencing surveillance.
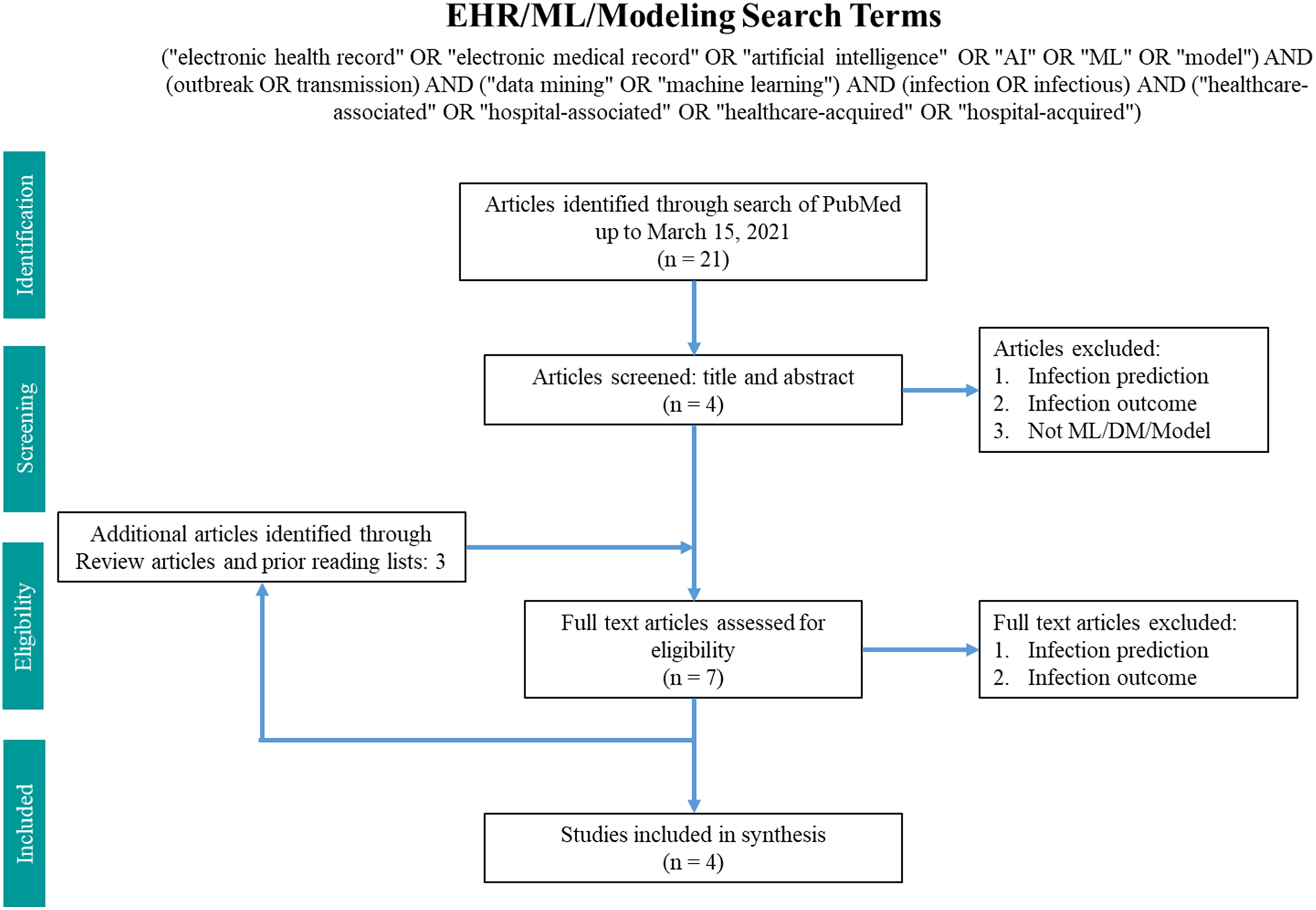
Fig. 2. Search terms in PubMed for machine learning and modeling.
The machine-learning search terms utilized were “(“electronic health record” OR “electronic medical record” OR “artificial intelligence” OR “AI” OR “ML” OR “model”) AND (outbreak OR transmission) AND (“data mining” OR “machine learning”) AND (infection OR infectious) AND (“healthcare-associated” OR “hospital-associated” OR “healthcare-acquired” OR “hospital-acquired”)” and returned 21 results. Article abstracts were screened to exclude infection prediction and outcome studies. Data on the methodology and findings of outbreak and transmission detection models were extracted and summarized.
Results
In total, 42 articles on WGS surveillance were included in the final review. Reference Ward, Hoss and Kolde3–Reference Sundermann, Chen and Miller5,Reference Tosas Auguet, Stabler and Betley9–Reference Wendel, Malecki, Otchwemah, Tellez-Castillo, Sakka and Mattner47 Among these studies, only 2 employed machine learning or statistical modeling to investigate transmission, which were also captured in the machine learning search. From 2013 to 2016, there was only one article per year, with a substantial increase thereafter (Fig. 3). Moreover, 12 studies were from the United States; 10 were from the United Kingdom; 5 were from Australia; 4 were from Germany; 2 were from Japan; and 1 study came per country came from China, Denmark, Finland, France, India, Italy, Netherlands, Spain, Sweden, and Thailand.
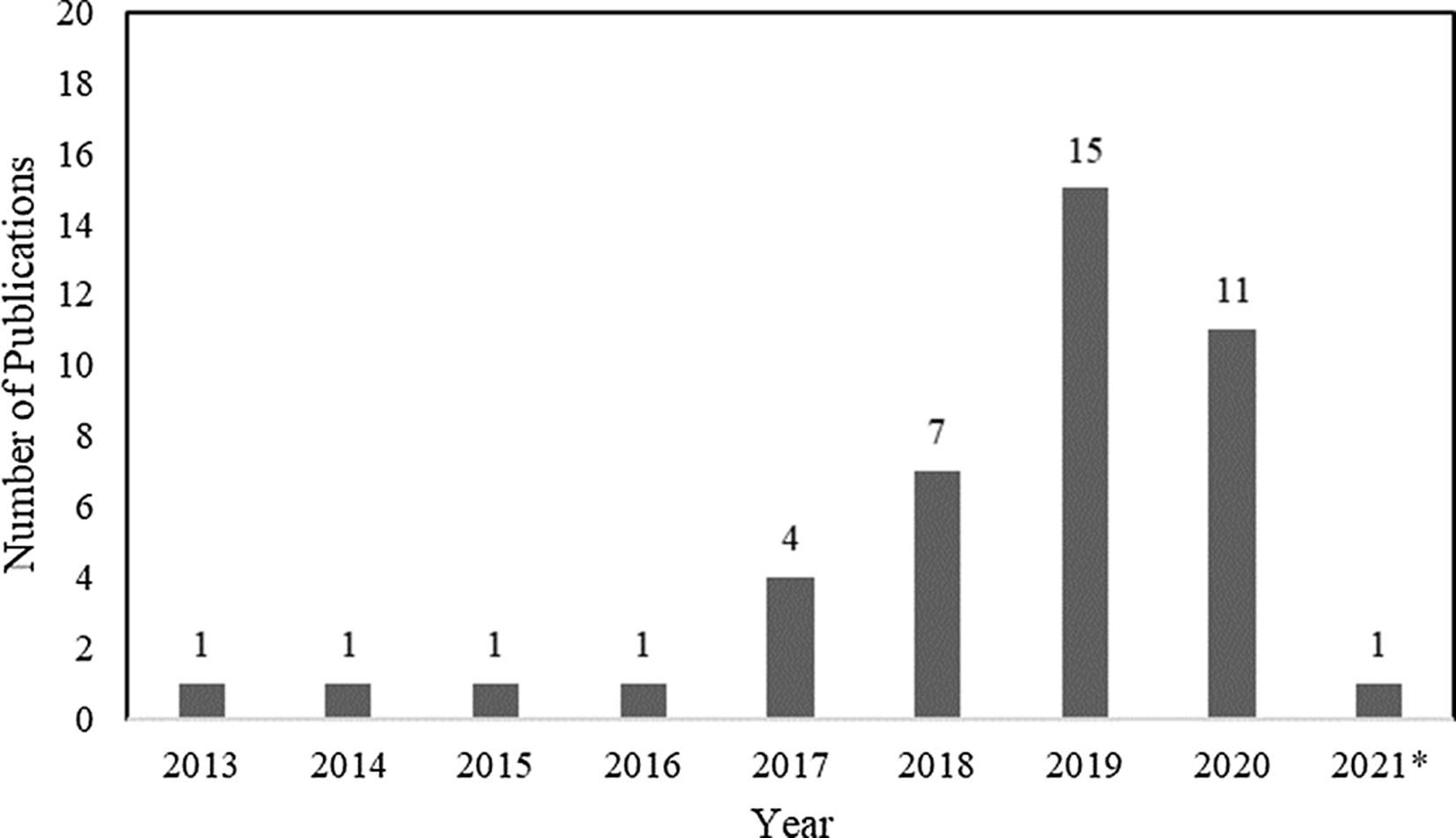
Fig. 3. Summary by year of 42 whole-genome sequencing (WGS) surveillance studies in PubMed through March 15, 2021. *Through March 15, 2021.
The duration of WGS surveillance varied substantially by study, with a median of 12 months and a range of 1–73 months (Supplementary Table S1). Only 2 (4.8%) studies were performed in real time; all other studies were performed retrospectively. Moreover, 39 studies included a single pathogen and 3 studies included multiple pathogens (Table 1). Staphylococcus aureus was the most commonly studied organism (12 studies, 28.6%), with 4 additional organisms present in >2 studies: 2 Klebsiella pneumoniae, 7 Clostridioides difficile, 6 Enterococcus faecium, and 3 Pseudomonas aeruginosa. Organisms selected for sequencing (eg, by anatomic site of infection, multilocus sequence type, and antibiotic resistance phenotype) were diverse across studies.
Table 1. Studies by Date, Organism, and Outbreaks Detected Utilizing WGS Surveillance
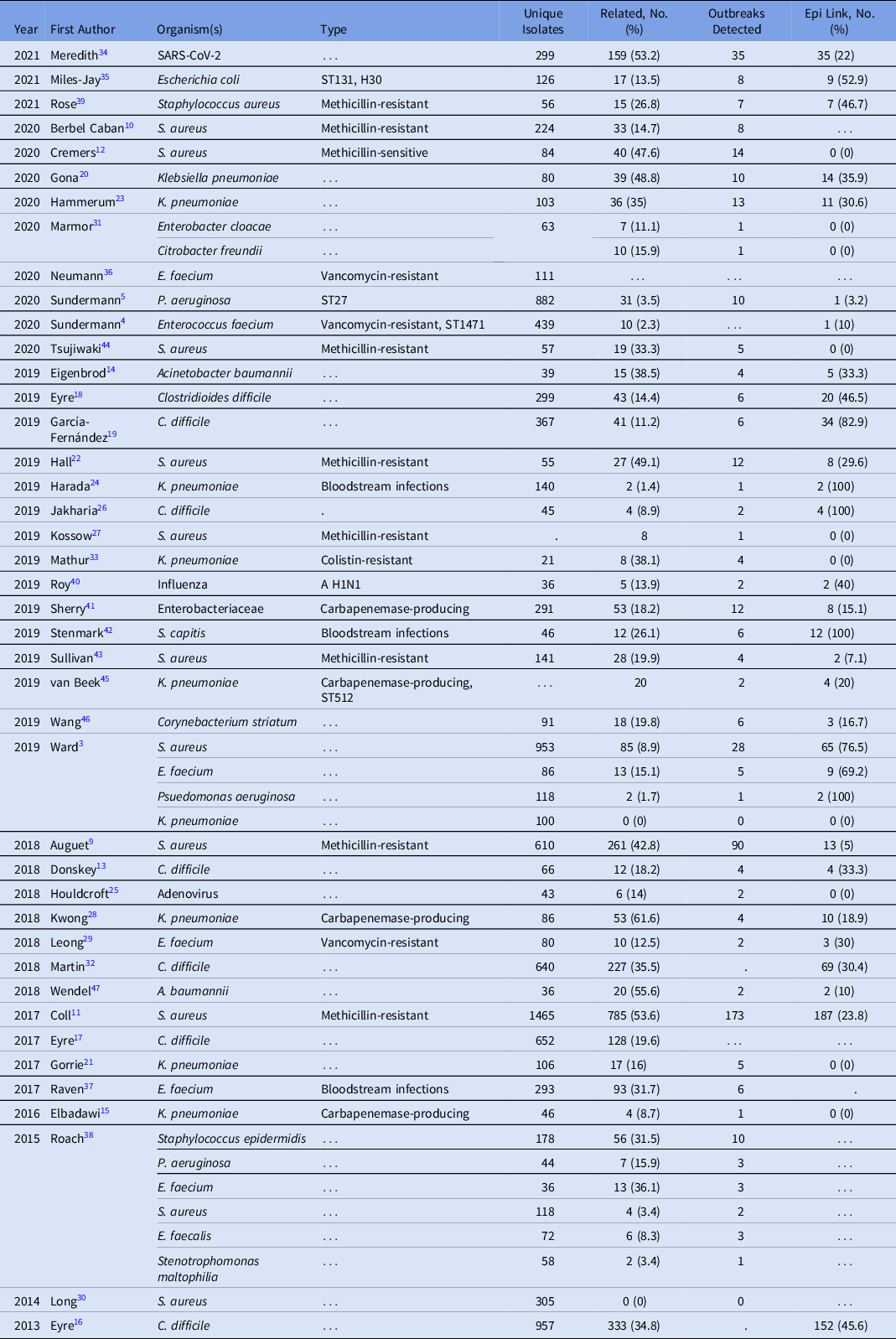
Criteria for defining genetic relatedness were also highly variable between studies and were generally based on the number of single-nucleotide polymorphism (SNP) differences between genomes (Supplementary Table S1 online). Among organisms present in >2 studies, C. difficile had the most consistent SNP cutoff at 2 SNPs, and 1 study used 10 SNPs to identify related isolates (Fig. 4). Furthermore, S. aureus had the widest distribution of SNP cutoffs, ranging from 7 to 50 SNPs.
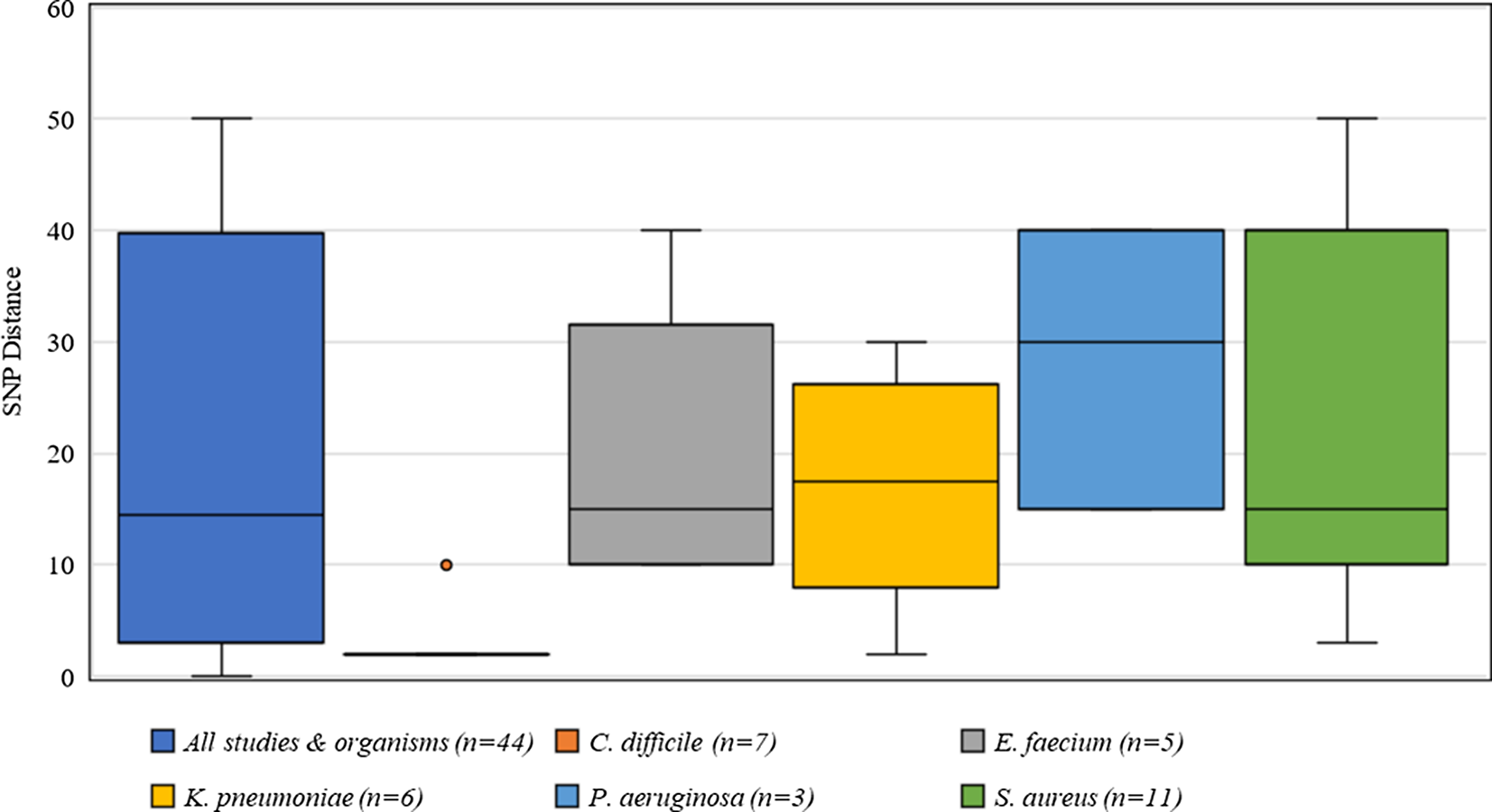
Fig. 4. Distribution of single nucleotide polymorphisms (SNPs) for defining genetic relatedness from 42 studies.
An analysis of the proportion of sequenced isolates that were determined to be genetically related to one another in each study revealed an average of 23.8% of isolates (range, 0%–61%). There were 525 outbreaks detected among 2,837 related isolates (average 5.4 isolates per outbreak). In addition, 41 studies (97.6%) found some level of genetic relatedness between the sequenced isolates.
We examined the methods employed to identify the responsible transmission routes for outbreaks that were detected by WGS. Overall, 35 studies (83.3%) restricted attempts to identify transmission routes to the same hospital unit during a defined period. Reference Tosas Auguet, Stabler and Betley9,Reference Coll, Harrison and Toleman11–Reference Kossow, Kampmeier, Schaumburg, Knaack, Moellers and Mellmann27,Reference Leong, Cooley and Anderson29–Reference Roach, Burton and Lee38,Reference Roy, Hartley, Dunn, Williams, Williams and Breuer40–Reference Stenmark, Hellmark and Söderquist42,Reference Tsujiwaki, Hisata and Tohyama44–Reference Wendel, Malecki, Otchwemah, Tellez-Castillo, Sakka and Mattner47 Only 7 studies (16.7%) examined other possible routes such as medical procedures or healthcare workers. Reference Ward, Hoss and Kolde3–Reference Sundermann, Chen and Miller5,Reference Berbel Caban, Pak and Obla10,Reference Kwong, Lane and Romanes28,Reference Rose, Nolan and Moot39,Reference Sullivan, Altman and Chacko43
Several studies were notable for uncovering otherwise unidentified transmissions, which is the main goal of WGS surveillance. Sullivan et al Reference Sullivan, Altman and Chacko43 were prompted by an outbreak in a neonatal intensive care unit (NICU) to retrospectively investigate all MRSA bloodstream infections for 16 months. Their investigation uncovered isolates related to the NICU outbreak from adult patients in a separate tower. Further investigation revealed shared ventilators between the adult unit and the NICU, which was believed to have caused transmission. Separately, Roy et al Reference Roy, Hartley, Dunn, Williams, Williams and Breuer40 performed sequencing of influenza A H1N1for 6 months and found that traditional infection prevention practice falsely identified outbreaks, and WGS surveillance data were able to connect cases that were previously not believed to be epidemiologically related. Lastly, Berbel Caban et al Reference Berbel Caban, Pak and Obla10 utilized WGS surveillance of MRSA over 2 years and found multiple undetected outbreaks within 2 New York City hospitals. One cluster of 24 isolates from 16 patients spanned 21 months and 9 different hospital wards with patterns of shared healthcare workers. In this study, the authors emphasized the limitations of investigating only geotemporal clustering in outbreak detection and investigation.
Furthermore, 4 articles identified using the machine-learning search terms included in the final synthesis, 2 of which overlapped in the WGS surveillance search terms. Reference Sundermann, Chen and Miller5,Reference Kwong, Lane and Romanes28,Reference Julia, Vilankar, Kang, Brown, Mathers and Barnes48,Reference Stachel, Pinto and Stelling49 Table 2 lists the methods and limitations of each study. One study utilized imputation of cultures to model transmission dynamics from environmental sink contamination, 2 studies used Bayesian methods to model transmission, and 1 study combined WHONET and SaTScan tools to detect outbreaks. In all of these studies, tools were implemented to supplement outbreak detection or investigation, yet the importance of manual or expert input to further investigate the transmissions or outbreaks detected was noted in each of these studies. In the study by Satchel et al, Reference Stachel, Pinto and Stelling49 45 outbreaks were identified, but only 6 were confirmed by an IP investigation. However, these researchers stated that the tool helped to streamline investigation efforts, which reduced time spent by the IP team.
Table 2. Studies Utilizing Machine Learning or Modeling to Detect outbreaks Or Transmission
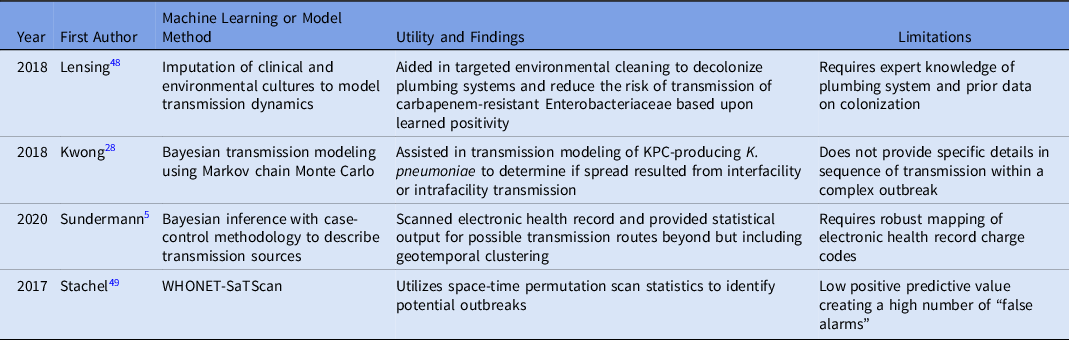
Discussion
In this systematic review, we synthesized studies that demonstrate the utility of WGS surveillance in finding cryptic outbreaks in healthcare settings. Nearly all studies (97.6%) identified outbreaks, but few (4.8%) utilized machine learning or statistical modeling methods to investigate transmission routes. WGS surveillance, while uncommon but increasingly utilized, aided infection prevention practice in these studies by uncovering outbreaks and enabling intervention.
Studies utilizing WGS surveillance have primarily relied on geotemporal linkage to identify transmission routes. Restricting investigations to geotemporal linkage fails to identify potential transmission by procedures that are performed in areas of the hospital other than patient nursing units or healthcare workers, as shown in some of the studies in this review. Some studies stated the limitations of relying solely on geotemporal parameters for identifying the transmission route for related isolates. Regardless, WGS surveillance enabled many of these studies to uncover substantial and significant previously undetected outbreaks that likely affected patient outcomes and associated healthcare costs.
Almost all of the studies reviewed were retrospective in nature, which limits the potential impact of WGS surveillance on healthcare epidemiology and infection prevention. If performed in real time, IP teams have an opportunity to perform an investigation (eg, audit practices, collect environmental cultures, and interview staff), which is not possible in retrospective studies. Furthermore, many studies focused on 1 pathogen, which is less sensitive for detecting outbreaks than WGS surveillance of multiple pathogens. For example, a single transmission route can lead to the spread of multiple pathogens.
Substantial investment and infrastructure are needed to establish real-time WGS surveillance. Healthcare institutions must have appropriate laboratory capacity, bioinformaticians, and genomic epidemiologists to interpret the data. A recent paper by Parcell et al Reference Parcell, Gillespie, Pettigrew and Holden50 discussed barriers to instituting a WGS surveillance program for outbreak detection from an economic and systemwide perspective. Indeed, it is often difficult to prove estimates of cost-effectiveness when considering prevention interventions, but 2 studies have demonstrated the cost-effectiveness of WGS surveillance programs. Reference Gordon, Elliott and Forde1,Reference Kumar, Sundermann and Martin7
We identified very few studies on the utility of machine learning or statistical modeling methods for identification of outbreak transmission routes by WGS surveillance. In our experience, machine learning adds value in detecting transmission routes that do not involve geotemporal clustering such as invasive procedures, healthcare workers, outbreaks separated by unit, and outbreaks of longer duration. Reference Sundermann, Chen and Miller5,Reference Sundermann, Miller and Marsh6,Reference Miller, Chen and Sundermann8 The use of machine learning in combination with WGS surveillance is clearly an understudied area of healthcare epidemiology and infection prevention. Barriers such as interoperability of electronic health records and adoption of WGS surveillance prevent the implementation of such programs. However, adoption of public health WGS surveillance for COVID-19 may expedite the use of this technology by healthcare institutions.
The combination of prospective WGS surveillance, EHR data, and machine learning has the potential to dramatically transform the paradigm of outbreak detection and investigation for infection prevention and control by identifying outbreaks quicker and enabling early intervention to halt transmission. This approach will both improve patient safety and reduce healthcare costs. However, healthcare institutional investment into establishing WGS surveillance programs will be key to expansion and implementation of this approach.
Supplementary material
To view supplementary material for this article, please visit https://doi.org/10.1017/ash.2021.241
Financial support
This study was funded in part by the National Institute of Allergy and Infectious Diseases, National Institutes of Health (NIH grant nos. R21Al109459 and R01AI127472).
Conflicts of interest
All authors report no conflicts of interest relevant to this article.