In 2010, the World Psychiatric Association published a review reporting that the general depiction of psychiatry in the news and entertainment media was predominantly negative.Reference Sartorius, Gaebel, Cleveland, Stuart, Akiyama and Arboleda-Flórez1 Since then, various public campaigns and strategies have been implemented to address and reduce this stigma.2,3 In England, ‘Time to Change’, which began in 2007, was the largest programme aimed at reducing stigma and discrimination against people with mental health conditions.Reference Sampogna, Gehlen, Giallonardo, Robinson, Thornicroft and Henderson4,Reference Evans-Lacko, Corker, Williams, Henderson and Thornicroft5 National surveys on attitudes towards mental illness surveys examined a decade of trends, from 2003 to 2013, in public attitudes in England. These surveys reported a significant increase in positive attitudes related to prejudice and exclusion following the launch of the Time to Change anti-stigma campaign. However, there was no notable improvement in tolerance or support for community care.Reference Evans-Lacko, Corker, Williams, Henderson and Thornicroft5 In the USA, campaigns such as ‘WhatMakesUs’, a digital media initiative in the Greater Omaha–Council Bluffs metropolitan area, were evaluated by a cross-sectional online survey, which found that respondents who were aware of the campaign exhibited lower stigmatising attitudes and beliefs towards people with mental health conditions.Reference Alvarado-Torres, Silesky, Helgenberger, Anderson, Granillo and Nared6 Australia has also seen multiple campaigns aimed at reducing stigma towards people with mental health conditions, including SANE Australia and Livin. A systematic review of anti-stigma campaigns in Australia found 61 programmes, although these appear to be localised rather than widely implemented at present.Reference Morgan, Wright and Reavley7
A literature review of attitudes towards psychiatry and psychiatrists in 2009 found that among patients who did not comply with a referral to a psychiatrist, the most frequently mentioned reason was the fear of mental illness stigma, rather than negative expectations about the treatment and its quality.2
Stigma and discrimination are well-known barriers that prevent individuals from seeking treatment for mental health conditions.Reference Byrne8 These factors can also prevent people from accepting help when offered or continuing with ongoing treatments.Reference Knaak, Mantler and Szeto9 Given the significant mental health burden, with an estimated 970 million people living with a mental disorder in 2019,2 it is crucial to investigate public perceptions of mental health professionals. Understanding whether stigma extends to health care professionals themselves is essential, as this could have profound clinical and policy implications.
Social media has become a valuable tool for capturing public opinion on a large scale. Between 2012 and 2023, the number of social media users grew by approximately 3.5 billion people, underscoring its central role in communication.Reference Gong, Stump and Li10,11 In July 2023, Twitter rebranded as X, with over 500 million active users in 2024.Reference Dean12 Throughout the article, we will refer to the platform as Twitter/X.
This study aimed to use social media, specifically Twitter/X, to (a) assess whether the public perceptions of different mental health professionals are positive or negative, (b) analyse how these perceptions changed over time and (c) examine engagement levels with tweets about mental health professionals over time.
Method
Collection of Twitter/X data and exploration of data
In this retrospective study, we collected and analysed all tweets posted in English between 2007 and 2023 that included any of the following keywords: psychiatry, psychology, neurology, mental health, psychiatrist, psychologist or neurologist. This data was retrieved using Tweet Binder, a search engine with access to 100% of publicly available tweets. The study period covers the entirety of Twitter/X existence, from its launch in 2007 to 2023, allowing for a thorough exploration and analysis of public opinion over a significant timeframe. In addition to the tweet content, we collected other data such as the date it was posted, number of retweets, number of likes and user descriptions. The number of retweets and likes were used as indicators of user engagement and interest in the tweeted content. Tweets with at least 100 retweets were classified as viral.
Each tweet was assessed on whether the content was classifiable or not. Tweets were not considered classifiable if they were not written entirely in English (some tweets contained multiple languages, and Tweet Binder collected all those Tweets in which the keyword was written in English) or if the content was not complete enough to understand the meaning of the tweet. Finally, we classified the perception of the tweet as positive, negative or neutral.
Machine learning application
Advancements in technology have facilitated the widespread use of artificial intelligence and machine learning across various domains, with social listening being particularly prominent.Reference Domingo-Espineira, Varaona, Montero, Lara-Abelenda, Gutierrez-Rojas and Fernandez Del Campo13,Reference Correia Lopes, Pinto da Costa, Fernandez-Lazaro, Lara-Abelenda, Pereira-Sanchez and Teo14 Machine learning, a subset of artificial intelligence, enables computer systems to learn from data, thereby mimicking human reasoning and performing tasks on a much larger scale.Reference Wang, Fu, Du, Gao, Huang and Liu15 This capability is essential for analysing vast data-sets that would be impractical to evaluate manually. Machine learning encompasses three primary types: supervised, unsupervised and semi-supervised learning. In this study, we utilise semi-supervised learning, which integrates elements of both supervised and unsupervised approaches by using both labelled and unlabelled data.Reference Riese, Keller, Prasad and Chanussot16
Before applying machine learning, a researcher manually classified 1500 tweets in English into categories based on their classification and sentiment. The tweets were then preprocessed, which involved normalising text by removing special characters, splitting negative contractions and converting emojis into classical text. The manually classified data-set was then randomly divided into a training subset (comprising 80% of the samples) and a test subset (comprising the remaining 20%).
For machine learning, we fine-tuned a transformer-based model known as BERTweet,Reference Nguyen, Vu and Nguyen17 using Python 3.8 (developed by Dat Quoc Nguyen and collaborators; see https://huggingface.co/vinai/bertweet-base/tree/main) using the training subset. BERTweet is built on the BERT architecture and trained on 80 Gb of text data, including over 860 million tweets in English. This model was selected because of its widespread use in the literatureReference Jhakal, Singal, Suri, Chaudhary, Kumar and Gorton18,Reference Bonetti, Martínez-Sober, Torres, Vega, Pellerin and Vila-Francés19 and its pretraining on tweets in English similar to those examined in this study. Fine-tuning involves retraining a pretrained model with task-specific data to adjust the parameters.Reference Sun, Qiu, Xu and Huang20 This process leverages the extensive knowledge acquired during the model's pretraining on large, unlabelled data-sets to tackle more specialised tasks effectively. One challenge during fine-tuning is the imbalance of the number of samples between options in both categories. To address this, we employed the Easy Data Augmentation pipeline, using Python 3.8 and the TextAttack (version 0.3.10) library (developed by John Morris and collaborators; see https://github.com/QData/TextAttack/tree/master), which generates additional tweets to balance the data-set by using techniques such as synonym replacement, random word removal and word position swapping. These strategies ensure an equal number of tweets for each option within the same category.
The model's performance was evaluated using the test set, with the fine-tuned BERTweet achieving a mean F1-score of 0.79 in the classifiable category and 0.72 in the sentiment category. These results demonstrate the model's efficacy in accurately classifying tweets. Subsequently, the fine-tuned model was used to classify the remaining 301346 unlabelled tweets in English, showcasing its ability to efficiently handle large-scale text classification tasks.Reference Wei and Zou21
Statistical analysis
We first conducted a descriptive analysis of the tweets based on different key terms. Engagement levels were measured by counting the number of likes and retweets. Given the strong negative skew resulting from the high number of low-impact tweets, we separately analysed those tweets that generated more than 100 retweets (viral tweets). For these viral tweets, we calculated their percentage of the total, as well as the median and interquartile range of likes and retweets.
For each key term, we analysed and reported the percentage of tweets with positive and negative perceptions. Additionally, we graphically evaluated the trends in these perceptions over time, using histograms. We compared the change in perception before and after 2020.Reference Tawil, Haque and Salameh22 Chi-squared tests were used for these comparisons.
We further evaluated the impact of tweets based on positive and negative perceptions. We described the number of likes and retweets with the mean and s.d., and performed a Welch's t-test to compare the means between tweets with positive and negative perceptions. We also analysed the change in likes and retweets according to perception in tweets before and after 2020.
Ethical approval
This study used publicly available tweets. This study was approved by the Research Ethics Committee at King's College London (reference number: MRSU-22/23-35453).
Results
The characteristics of the tweets included in the analysis are reported in Table 1. Tweets containing the key term ‘mental health’ had the largest number of tweets (n = 45 892) and the greatest engagement, with 29.4% classified as viral tweets, having a median of 839 retweets and 2546 likes. Among the other key terms (‘psychiatry’, ‘psychiatrist’, ‘psychology’, ‘psychologist’, ‘neurology’ and ‘neurologist’), there was a smaller range of retweets, with a median between 170 and 255 among tweets classified as viral.
Table 1 Descriptive characteristics of the original tweets included in the analysis, according to the keyword and impact

IQR, interquartile range.
The key terms ‘psychiatrist’ and ‘psychologist’ appeared in 41 669 and 22 755 tweets, respectively, making them the second and third most used terms, significantly higher than the other key terms.
Table 2 shows that tweets containing the key terms ‘mental health’, ‘psychology’, ‘psychologist’, ‘neurology’, ‘neurologist’ and ‘psychiatry’ had a higher percentage with a positive perception compared with a negative perception, both before and after 2020. However, tweets containing the key term ‘psychiatrist’ had a higher proportion with a negative perception. Before 2020, there was a higher proportion of tweets about psychiatrists with a negative perception (n = 4872, 39.52%), compared with a positive perception (n = 1972, 15.99%). Although this gap narrowed after 2020, negative perceptions still prevailed (n = 5505, 36.10% negative v. n = 3472, 22.77% positive). The increase in positive perceptions and decrease in negative perceptions over time was statistically significant (P < 0.001).
Table 2 Perception of the tweets for each keyword and over time
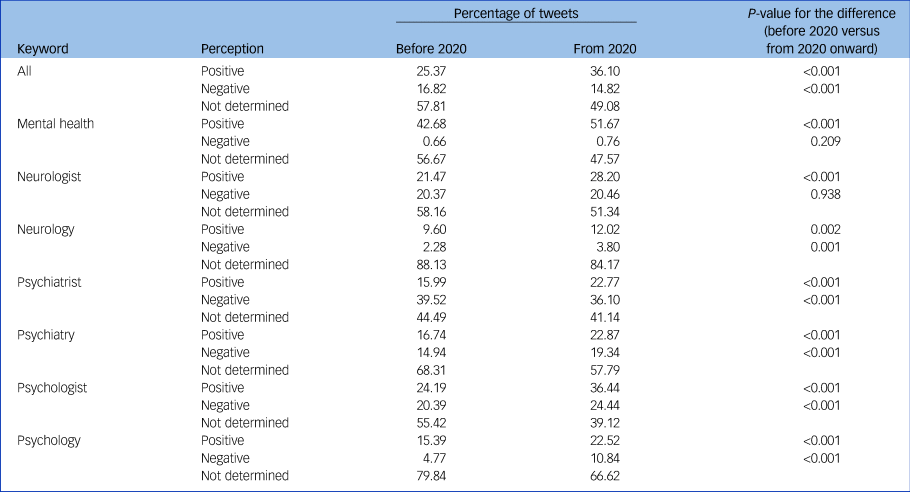
The key term ‘mental health’ had the highest proportion of tweets with a positive perception, with 42.68% before 2020 and 51.67% after 2020. The term ‘psychologist’ was the second highest key term with regard to proportion of tweets with a positive perception (24.19% before 2020 and 36.44% after 2020). Conversely, the term ‘psychiatrist’ had the largest proportion of tweets with a negative perception, with 39.52% before 2020 and 36.10% after 2020, whereas ‘mental health’ had the smallest proportion of negative perceptions, with 0.66% before 2020 and 0.76% after 2020 (Table 2).
There was a statistically significant increase in positive perceptions for all key terms from before 2020 to after 2020, particularly for ‘mental health’, ‘psychiatry’, ‘psychiatrist’, ‘psychology’, ‘psychologist’ and ‘neurologist’ (P < 0.001), and for ‘neurology’ (P = 0.002). A statistically significant decrease in negative perceptions was observed for all key terms overall, particularly for ‘psychiatrist’ (P < 0.001), although there was a statistically significant increase for ‘neurology’, ‘psychiatry’, ‘psychology’ and ‘psychologist’.
Figure 1 illustrates the annual number of tweets according to perception, both for all tweets and for the key terms ‘mental health’, ‘psychologist’ and ‘psychiatrist’. Figure 1(a) shows a year-on-year increase in positive perceptions, alongside a large increase in the number of tweets using these terms. Figure 1(b) highlights that a large proportion of tweets using the key term ‘mental health’ had a positive perception, with very few having a negative perception. It also demonstrates the rise in positive perceptions over time, alongside the number of tweets about mental health. When comparing Figs 1(c) and 1(d), tweets using the term ‘psychologist’ showed a larger proportion of positive perceptions, whereas tweets using the term ‘psychiatrist’ had a higher proportion of negative perceptions.

Fig. 1 Number of tweets posted each year according to perception, separated by key term used.
The impact of the tweets according to their perception of mental health over time is reported in Table 3. Overall, tweets with a positive perception of the key terms analysed had higher engagement with, on average, more retweets (mean 293) and likes (mean 1103). In contrast, tweets with a negative perception had lower engagement with an average of 124 retweets and 434 likes. There was a statistically significant difference (P < 0.001) in the number of likes and retweets between tweets with a positive perception and those with a negative perception. Over time, there was a statistically significant decrease in the mean number of retweets for tweets with a positive perception across all key terms (P < 0.001). Conversely, the number of likes for tweets with a negative perception significantly increased over time (P < 0.001). Despite this, tweets with a positive perception continued to attract more engagement compared with those with a negative perception.
Table 3 Impact of the tweets according to their perception of mental health and over time

The differences between the number of likes and retweets between those expressing a negative and a positive perception were statistically significant for all times (P < 0.001).
Discussion
Key findings
There was a predominance of positive perceptions for the key terms analysed, including ‘mental health’, ‘psychiatry’, ‘psychology’, ‘psychologist’, ‘neurology’ and ‘neurologist’, with the exception of the term ‘psychiatrist’. Although the perception of ‘psychiatrist’ has improved over time, a great proportion of tweets still carry a negative perception compared with a positive one.
Over time, the key terms ‘mental health’, ‘psychology’, ‘psychologist’, ‘psychiatry’ and ‘psychiatrist’ have shown a statistically significant increase in positive perceptions. There has also been a statistically significant decrease in negative perceptions for the key terms ‘psychiatry’, ‘psychiatrist’, ‘psychology’ and ‘psychologist’.
The term ‘mental health’ had the highest number of tweets and the greatest level of engagement, and the largest proportion of tweets with a positive perception, whereas ‘psychiatrist’ had the highest proportion of tweets with a negative perception.
For all key terms, tweets with a positive perception attracted higher engagement compared with those with a negative perception. Over time, there has been a decrease in the number of retweets for tweets with a positive perception, alongside an increase in number of likes for tweets with a negative perception.
Strengths and limitations
A key strength of this study is the extensive data-set, comprising 301 346 tweets collected over a 16-year period. This long-term data collection enabled a comprehensive analysis of trends and shifts in public perception, including the impact of the COVID-19 pandemic on attitudes towards mental health. Social media research helps to mitigate some of the biases often found in survey-based studies, where results can be skewed by the self-selecting nature of participants.
However, this study also has some limitations. First, Twitter/X users are predominantly individuals with internet access, which tends to exclude certain populations, such as the older generation or people in lower-middle-income countries, limiting the generalisability of our findings. Second, our analysis focused solely on tweets in English; there may be differences in perceptions expressed in other languages.
Comparison with other literature
Our study found a higher percentage of negative tweets about psychiatrists compared with positive ones. This is in line with the findings of a multicentre survey conducted in 12 countries, which showed that psychiatrists report significantly higher perceived stigma and discrimination experiences than general practitioners.Reference Gaebel, Zäske, Zielasek, Cleveland, Samjeske and Stuart23
A vignette-based trend study in Germany, which used structured interviews and population surveys from 1990 to 2020, reported an increase in favourable attitudes towards mental health professionals over the past three decades,Reference Angermeyer, Schindler, Matschinger, Baumann and Schomerus24 attributed to broader cultural changes in Germany during that period.Reference Angermeyer, Schindler, Matschinger, Baumann and Schomerus24 There were already positive attitude towards mental health professionals three decades ago, and these have continued to grow.Reference Angermeyer, Schindler, Matschinger, Baumann and Schomerus24 The most pronounced increase in the German public's views to seek help from mental health professionals occurred in the 1990s, before the onset of anti-stigma campaigns or public awareness initiatives. In contrast, our study, which focused on tweets in English, found that before 2020, there were more negative tweets about psychiatrists than positive.
There has also been research on COVID-19 and the spread of ‘fake news’ on Twitter/X, which could be relevant when analysing perceptions of mental health.Reference Castioni, Andrighetto, Gallotti, Polizzi and De Domenico25 There may be specific incidents, or high-profile famous cases about treatment perceived negatively, that may have a significant impact when shared widely on Twitter/X.Reference Castioni, Andrighetto, Gallotti, Polizzi and De Domenico25 However, our research indicates that positive tweets have higher engagement than negative ones, suggesting that isolated negative experiences may not have significantly affected the overall data.
Implications of the findings for future policies and research
This research underscores the importance of improving the public perception of psychiatrists. In 2010, the World Psychiatric Association recommended that depiction of psychiatry in the media is crucial for shifting public opinion.2 This has significant clinical implications and, if followed, could contribute to increased positive perceptions over time.
Psychiatry itself has evolved in many ways over time and, given the extensive amount of time that people spend on social media, platforms like Twitter/X present a critical opportunity for research and global outreach. This study could contribute to leveraging Twitter/X to enhance public understanding about psychiatrists and reduce stigma, thereby facilitating access to mental health support.
Understanding the reasons behind the increasing positive perceptions of mental health over time is essential to maintaining this positive trend. Various campaigns and awareness initiatives, such as incorporating mental health education into school curricula in England,26 may have contributed to this shift.Reference Knaak, Mantler and Szeto9 The COVID-19 pandemic also played a significant role in raising mental health awareness.Reference Nealon27 During the pandemic, the role of psychiatrists expanded considerably, with some even being even redeployed to other services to meet the wider healthcare demands of the crisis.Reference Pereira-Sanchez, Adiukwu, El Hayek, Bytyçi, Gonzalez-Diaz and Kundadak28 In addition to managing patients with pre-existing mental health conditions who faced new challenges related to the virus, psychiatrists had to advocate for those at increased risk of infection. They were also required to support healthcare colleagues who were distressed and exhausted by the unprecedented nature and volume of work. Furthermore, many individuals without mental health diagnoses before the pandemic sought psychiatric help because of the effects of isolation, economic uncertainty and the trauma and grief experienced during the pandemic.Reference Baldwin, Gordon and Marlowe29,Reference Shalev and Shapiro30 Broader cultural shifts over time may also explain the improvement in public attitudes towards mental health.Reference Angermeyer, Schindler, Matschinger, Baumann and Schomerus24
The discrepancy in perceptions of psychologists and psychiatrists is noteworthy. Patients typically seek help from psychologists voluntarily, whereas psychiatrists often see patients who are brought for treatment involuntarily under mental health legislation. Future research including interviews with the general population and patients with lived experience could provide deeper insights into these differences in attitudes.
It is also important to further understand the difference in public perceptions of psychiatrists compared with neurologists. A prospective cohort study conducted in Scotland in 2000 assessed 300 newly referred patients at a neurology out-patient clinic, and found that 47% met the DSM-IV criteria for anxiety or depressive disorder, yet few patients wished to receive psychiatric or psychological treatment despite being willing to see a neurologist.Reference Carson, Ringbauer, MacKenzie, Warlow and Sharpe31 Another study, conducted in the USA at an epilepsy tertiary care clinic, found that of 63 patients symptomatic of anxiety or depression, 83% preferred a neurologist to prescribe medication for these symptoms rather than be referred to a psychiatrist.Reference Clary, Croxton, Snively, Brenes, Lovato and Sadeghifar32
Stigma and discrimination against people with mental health conditions are pervasive across societies and cultures.Reference Knaak, Mantler and Szeto9 However, our findings suggest that the stigmatisation of professionals themselves appears to be improving over time. Future research could investigate these trends among non-English speaking countries, to provide a more global perspective.
In conclusion, using Twitter/X to understand the public perception of mental health and key mental health professionals provides valuable insights into the evolving nature of public opinion over time. Although there is evidence of a general shift toward more positive views on mental health, significant stigma persists, particularly towards psychiatrists. The term mental health has the highest proportion of tweets with a positive perception and the greatest level of positive engagement, which continues to increase overtime. Understanding these trends and continuing to develop strategies to target stigma among psychiatrists could have a positive impact in improving access to treatment.
Data availability
The data that support the findings of this study are available on request from the corresponding author, M.P.d.C.
Author contributions
H.B. and M.P.d.C. wrote this article. M.P.d.C. and M.A.A.-M. conceptualised this study. F.J.L.-A. applied computational analysis. R.P.-A. conducted the statistical analysis. All authors reviewed the article.
Funding
The study (FIS-PI22/00653) was supported by the Instituto de Salud Carlos III (grant number Estatal de I+D+I 2020–2027) and co-financed by the European Development Regional Fund ‘A way to achieve Europe’, as well as the Comunidad de Madrid (grant number P2022/BMD-7321). The funders were not involved in the study design, collection, analysis, interpretation of data, the writing of this article or the decision to submit it for publication.
Declaration of interest
M.P.d.C. is a member of the British Journal of Psychiatry Editorial Board and did not take part in the review or decision-making process of this article. All other authors have none to declare.
eLetters
No eLetters have been published for this article.