1. Introduction
Previous work on language acquisition has drawn attention to the complexity of the task of learning verb meaning (e.g., Gentner Reference Gentner1978). In order to acquire a new verb, the child needs to identify how many entities are involved in the event depicted by the verb, and how they relate. Paying attention to the contexts in which verbs occur does not help the child to achieve this task: they might allow many possible mappings to verb meaning in a particular situation (Naigles Reference Naigles1996).Footnote 1
Based on the systematic correspondence between verb meaning and the syntactic structures or frames they can occur in, it has been postulated that children learn the former by paying attention to the latter (e.g., Gleitman Reference Gleitman1990, Fisher Reference Fisher1996). Children infer that novel verbs in different syntactic structures have different meanings. For example, causative verbs typically occur in transitive frames and non-causative verbs typically occur in intransitive frames. Thus, syntactic structure is a source of information that helps narrow the semantic hypothesis space licensed by the observation of the extralinguistic context – other proposed sources are prosody, function words (Cauvet et al. Reference Cauvet, Limissuri, Millotte, Skoruppa, Cabrol and Christophe2014, de Carvalho et al. Reference de Carvalho, He, Lidz and Christophe2019) and morphology (He and Lidz Reference He and Lidz2017). This is the basic claim of the syntactic bootstrapping hypothesis.
Notwithstanding, children under the age of two fail to assign appropriately different interpretations to verbs presented in transitive and intransitive frames (Hirsh-Pasek et al. Reference Hirsh-Pasek, Golinkoff, Naigles, Hirsh-Pasek and Golinkoff1996, Bavin and Growcott Reference Bavin, Growcott, Perkins and Howard2000). Hence, some studies have investigated the developmental origins of the ability to use sentence frames to guide verb learning. These studies claim that children use sentence structure to learn about meaning even before they can identify verb subcategorization frames. That is, they can obtain semantic information from a partial, probably imperfect pre-syntactic representation of the sentence (with dropped arguments, incomplete or incorrect parsings; see Fisher et al. Reference Fisher, Geoffrey Hall, Rakowitz and Gleitman1994 and Fisher Reference Fisher1996). Thus, syntactic bootstrapping is claimed to begin with a structure or analogical mapping mechanism (hereafter referred to as SAMM) that projects a set of referential terms in a sentence onto a set of participants in the conceptual representation of the event expressed by the verb (Fisher Reference Fisher1996; Yuan et al. Reference Yuan, Fisher and Snedeker2012).
Although the SAMM account does away with some of the strong assumptions of the syntactic bootstrapping hypothesis, it makes one strong assumption on its own. It posits that learners can identify some familiar nouns in speech, interpret them as possible verb arguments and use them to build an abstract representation of the sentence equivalent of the conceptual representation of verb meanings, that is, the predicate-argument structure (Fisher Reference Fisher1996).
Several experiments, mostly conducted on white, middle-class English-learning populations, have shown that children as young as 19 months assign different interpretations to novel verbs in sentences containing one noun (e.g., “He's gorping”) versus sentences containing two nouns (e.g., “He's gorping him”; Fisher Reference Fisher1996, Reference Fisher2002; Yuan and Fisher Reference Yuan and Fisher2009; Yuan et al. Reference Yuan, Fisher and Snedeker2012). For instance, as part of a forced-choice task, 3- and 5-year-olds (Fisher Reference Fisher1996) and 2- and 3-year-olds (Fisher Reference Fisher2002) were exposed to caused-motion events in which one female active participant directly caused the motion of an also female passive participant in either transitive (with two nominals (NP or DP arguments of the verb), e.g., “She's pilking her fast”) or intransitive sentence contexts (with one nominal, e.g., “She's pilking fast”). Then, children were shown a still picture of the midpoint of the videotaped event and instructed to point to the person performing the action described by the verb. Their responses were significantly affected by sentence context: they were more willing to choose agents of motion as the subjects of transitive sentences and patients of motion as the subjects of intransitive sentences. These results suggest that the number of nominals in the sentence influenced their interpretation of some aspects of verb meaning.
Similar results were found using a different experimental paradigm. Yuan et al. (Reference Yuan, Fisher and Snedeker2012) showed 21- and 19-month-olds two simultaneous events: a two-participant caused-motion event and a one-participant action event. These were accompanied by a novel verb in one of the following sentence contexts: transitive (“He's gorping him!”), intransitive (“He's gorping!”) or neutral (e.g., “That looks fun!”). The results supported the SAMM proposal: children who had heard the novel verb in transitive sentences looked longer at the two-participant event than those who had heard the new verb in intransitive sentences. In another study, Yuan and Fisher (Reference Yuan and Fisher2009) exposed 2-year-old English-learning children to dialogues in which interlocutors used a novel verb in transitive (“Jane blicked the baby!”) or intransitive sentences (“Jane blicked!”). They found that, if exposed to transitive dialogues, children looked longer at the two-participant event in the test trial than those who had been exposed to intransitive dialogues. They confirmed that children were not merely matching people in a screen with nominals in a sentence and, further, that syntactic structures are meaningful to children even without a concurrent scene.
In addition, some experimental studies (see Lidz et al. Reference Lidz, Gleitman and Gleitman2003, for Kannada; Lee and Naigles Reference Lee and Naigles2008, for Mandarin; and Göksun et al. Reference Göksun, Küntay and Naigles2008, for Turkish) suggest that children learning argument-dropping languages use the SAMM to infer verb meanings as well. For example, in Mandarin, Turkish or Kannada the number of noun phrases (NPs) is not an entirely reliable cue: these languages allow ellipsis of noun arguments in appropriate discourse contexts. Further, in Kannada and Turkish, other morphological devices, such as the use of causative morphology or case markers, are better predictors of causative meaning than the number of NPs. Nonetheless, 2- and 3-year-old Mandarin-learning children (Lee and Naigles Reference Lee and Naigles2008), 2- to 5-year-old Turkish-learning children (Göksun et al. Reference Göksun, Küntay and Naigles2008) and 3-year-old Kannada-learning children (Lidz et al. Reference Lidz, Gleitman and Gleitman2003) interpreted verbs as causative by paying attention to the number of overt nominals in the sentences. In these experiments, authors found effects of argument number and of the presence of the accusative marker (for Turkish), but no effects of causative morphology (for Turkish and Kannada).
These results had led researchers to conclude that the interpretational bias to map nouns in a sentence to participants in an event is innate: from birth, children are biased to interpret that a verb combined with two nouns involves two participants, whereas a verb combined with one noun involves one participant. Consequently, children would not learn these patterns from the input, as evidenced by the fact that Mandarin-learners apply a pattern that is rather weak in their input and, conversely, Turkish and Kannada-learners do not apply a highly strong pattern in these languages (i.e., the presence of the causative morpheme). Notwithstanding, Göksun et al. (Reference Göksun, Küntay and Naigles2008) and Lee and Naigles (Reference Lee and Naigles2008) report that children's experimental behaviour matches their language input patterns: the presence of a post-verbal noun phrase with intransitives has a stronger effect on English-learners than Mandarin or Turkish-learners.
Even though the aforementioned studies have investigated the impact of causative or case-marking morphology on verb interpretation, no attention has been given to the role of agreement morphology. In Göksun et al. (Reference Göksun, Küntay and Naigles2008), for instance, non-causative enactments by Turkish-learning children were increased by the presence of a single NP accompanying a transitive verb (e.g., inek it-sin ‘cow push.opt.3sg’), despite the fact that there were two referential expressions in total, since the verb was inflected for 3rd person singular. There are two alternatives: (a) children did not interpret agreement morphology at all, although previous studies suggest that by 20 months, Turkish children have grasped the basic patterns of verb inflection (Aksu-Κος and Ketrez Reference Aksu-Κος, Ketrez, Bittner, Dressler and Kilani-Schoch2003); (b) children interpreted agreement morphology as having the same reference as the overt noun.
In addition to the experimental research, the SAMM has been addressed by computational studies that have modelled a learner following its assumptions and obtained results that parallel children's behaviour in laboratory tasks (e.g., Connor et al. Reference Connor, Gertner, Fisher, Roth, Clark and Toutanova2008, Christodoulopoulos et al. Reference Christodoulopoulos, Roth, Fisher, Korhonen, Lenci, Murphy, Poibeau and Villavicencio2016). For instance, Connor et al. (Reference Connor, Gertner, Fisher, Roth, Clark and Toutanova2008) trained a system to label semantic roles automatically using samples of parental speech and written text, but only on sentences with eight words or fewer. So as to assign role labels for each potential argument (i.e., each noun in a sentence), the system used one of the following features: the number and order of nouns, the position of the noun relative to the verb, and so forth. Every version of the system was tested on invented sentences with novel verbs and nouns that appeared more than twice in training, but also over real child-directed speech and a sample of written text. The learner that took into account the number and order of nouns generalized the pattern: Noun 1 of 2 = agent and Noun 2 of 2 = patient to new verbs. Hence, it generated the error (also observed in children: Hirsh-Pasek et al. Reference Hirsh-Pasek, Golinkoff, Naigles, Hirsh-Pasek and Golinkoff1996, Bavin and Growcott Reference Bavin, Growcott, Perkins and Howard2000) of interpreting “A and B gorp” as an event relating an agent with a patient. The occurrence of this error decreased after including the position of the noun relative to the verb, indicating that children's early sentence comprehension is dominated by less sophisticated representations of word order.
In order to simulate more closely the human learner, Christodoulopoulos et al. (Reference Christodoulopoulos, Roth, Fisher, Korhonen, Lenci, Murphy, Poibeau and Villavicencio2016) trained a model incrementally: to assign words to lexical categories, the learner accessed information progressively, throughout the exposure to the utterances of the dataset. They found that an incremental model requires a higher number of seed nouns to achieve the same level of precision as that obtained by a batch model.
To summarize, the literature on the SAMM provides evidence that the number of nouns in a sentence is one of the sources of information that guide early verb learning: children can infer some aspects of verb meaning by aligning the nominals in a sentence with the participants performing an event. Hence, a verb combined with two nouns expresses a two-participant event, whereas a verb combined with one noun expresses a one-participant event. In this sense, the SAMM “predicts early success in distinguishing transitive from intransitive verbs” but only “if the sentences are simplified so that the number of nouns in the sentence is informative” (Yuan et al. Reference Yuan, Fisher and Snedeker2012:1384; see also Fisher Reference Fisher1996). That is why previous studies have tested children on simplified sentences with up to two nouns and, in the case of computational studies, why lengthy sentences were excluded from the analyzed database.Footnote 2
Accordingly, real speech poses several challenges to the SAMM. Unlike experimental utterances, in natural linguistic exchanges utterances may have more than two nominals. For instance, the presence of adjuncts can increase the number of nouns beyond the expected count. Participants can also be omitted if they can be retrieved from previous discourse or the extralinguistic situation (for instance, in imperative sentences, such as tomá ‘take’, in which objects are frequently dropped) and if they are implied: El bebé ya comió (algo) ‘The baby has already eaten (something)’.Footnote 3
The purpose of the present study is to explore the performance of the SAMM using real input to children learning Spanish. To do so, we evaluate the strength of the number of nominals in the utterance as a cue to verbal syntactic classes. Four possible parsing conditions are considered and, instead of using invented sentences with up to two nouns, we use utterances from everyday exchanges. In doing so, we consider phenomena previously overlooked, such as lengthy utterances, agreement morphology and ditransitive verbs.
2. Method
The following sections introduce the data under study (section 2.1), including the transcription and segmentation methods used (section 2.1.2), morphological and nominal parsing methodology (sections 2.1.3 and 2.1.4), and verb transitivity classification (section 2.1.5). Section 2.2 then discusses how the data were processed and the statistical analyses used.
2.1. Data
This study assessed the SAMM over data collected, transcribed and segmented in utterances by Rosemberg, Alam, Stein, Migdalek, Menti, and Ojea (Rosemberg et al. Reference Rosemberg, Alam, Stein, Migdalek, Menti and Ojea2015–2016). It was gathered as part of a longitudinal study following typically-developing Spanish-learning infants living in the city of Buenos Aires and surrounding areas. These data included naturalistic interactions at home (and occasionally outside) in which the speech from the target child, their caregivers and sometimes other children and adults was recorded in four-hour sessions.Footnote 4
Our sample is made up of transcribed audio-recordings from caregivers to twenty subjects (11 females, 9 males; average age: 19 months; age range: 16–23 months). Two pieces of evidence suggest that this is an appropriate age for analyzing the information provided by the input. First, at this age children know and can discriminate between nouns and verbs. Although verbs start featuring in children's productive vocabularies after 24 months of age (Goldin-Meadow et al. Reference Goldin-Meadow, Seligman and Gelman1976), verb comprehension precedes production. Thus, before 24 months, children already understand many verbs (Goldin-Meadow et al. Reference Goldin-Meadow, Seligman and Gelman1976, Bates et al. Reference Bates, Benigni, Bretherton, Camaioni and Volterra1979; Golinkoff et al. Reference Golinkoff, Hirsh-Pasek, Cauley and Gordon1987, Smith and Sachs Reference Smith and Sachs1990, Caselli et al. Reference Caselli, Bates, Casadio, Fenson, Fenson, Sanderl and Weir1995). Moreover, before producing and comprehending many nouns and verbs, shortly after 1 year of age, children can perform basic grammatical categorization (Gómez and Lakusta Reference Gómez and Lakusta2004, Höhle et al. Reference Höhle, Weissenborn, Kiefer, Schulz and Schmitz2004, Gerken et al Reference Gerken, Wilson and Lewis2005. Mintz Reference Mintz, Hirsh-Pasek and Golinkoff2006). Second, as mentioned earlier, 19-month-old English-learning children use the number of nouns in a sentence to interpret novel verbs heard in experimental settings (Yuan et al. Reference Yuan, Fisher and Snedeker2012). Given that the SAMM has not been attested at earlier stages and that children later increase their syntactic parsing abilities and might use more advanced strategies, we have focused on the input they are exposed to at this age of development.
Children in our sample were socioeconomically diverse: 10 from low-income families whose parents had less than 12 years of education, and 10 from middle-class families whose parents had 16 or more years of education.
2.1.1. Transcription and segmentation
Two of the four recorded hours from each participant (i.e., a total of 40 hours) were orthographically transcribed by Rosemberg and colleagues following the CHAT (MacWhinney Reference MacWhinney2000) format. Transcribed speech was segmented into utterances meeting at least two of the following three criteria: (a) that they be bounded by a pause longer than two seconds, (b) that they have a distinct intonational contour, and (c) that they be syntactically complete.Footnote 5
2.1.2. Morphological parsing and utterance filtering
Transcribed and segmented speech was processed with CLAN's MOR for Spanish (MacWhinney Reference MacWhinney2000) to add a layer of morphosyntactic information, further disambiguated by POST (Parisse and Le Normand Reference Parisse and Le Normand2000).
Only utterances with one finite verb identified by MOR were sampled. Finite verb inflections are quite salient in Spanish morphology, which led us to think that they might act as a strong cue to the identification of verbs by children learning Spanish. Previous studies on the SAMM did not explore which verb forms children apply this mechanism to, so there is no evidence as to whether children apply the SAMM to verbs that do not function as actual predicates, such as auxiliaries, copular verbs and so forth. We have therefore excluded them from the analyses: whenever the finite verb was a copula or an impersonal verb, or if it was part of an idiom (e.g., cagar ‘shit’ in cagar a piñas ‘beat up’) the utterance was filtered out. Utterances containing more than one verb (e.g., verb periphrasis with auxiliaries), non-finite verbs or no verbs at all were excluded from the analyses. In this way, we avoided assessing the SAMM over a range of overwhelmingly diverse phenomena and, nevertheless, fulfilled the purpose of analysing a more realistic sample of speech. The analyzed sample contained 27.3% of the utterances in the original dataset (i.e., 5,400 utterances from the original 19,791 utterances).
2.1.3. Nominal parsing conditions
In a subsequent step, we varied the inclusion criteria for “nominal” to allow for four different hypotheses about children's identification of this category:
1. Stimuli-nominals (SN): In this condition we only labelled as nominals word categories previously tested in experimental studies on the SAMM, that is, common and proper nouns, personal pronouns in the nominative, accusative, dative, prepositional and reflexive case as well as demonstrative pronouns. This strategy does not extend the SAMM beyond the lexical categories over which it has already been attested.
2. Stimuli-nominals-and-inflections (SNI): Same as SN, above, but with the addition of verb inflections for person agreement.
3. All-nominals (AN): This one constitutes a sophisticated strategy by which almost every word functioning as a nominal was labelled as such. Not only were common and proper nouns included but also personal, demonstrative, possessive, indefinite, interrogative/exclamative and relative pronouns functioning as nouns. Phrases headed by adjectives acting as nouns (for instance, la vieja adj ‘the old lady’) were computed as well. Elements with several non-pronominal uses were excluded, to avoid introducing undesired noise: the reflexive pronoun in the 3rd person singular and 2nd/3rd person plural form (se) and the relative pronoun que.Footnote 6 Possessive pronouns were only considered if preceded by articles (e.g., el mío ‘mine’) and not in predicate contexts with copulative verbs, for instance, Es mío ‘It's mine’. Indefinite elliptical constructions were left out, for instance, indefinite numeral or quantitative phrases (e.g., Tengo mucho ‘I have a lot’, Quiero más ‘I want more’ or Encontré bastante ‘I found plenty’).
4. All-nominals-and-inflections (ANI): The same parsing strategy as AN, but this time identifying as nominals person-agreement morphemes occurring in the verb.
Table 1 summarizes the nominal elements included in each of the four parsing conditions.
Table 1: Summary of the nominal categories included in the four parsing conditions. SN: stimuli-nominals; AN: all-nominals; SNI: stimuli-nominals-and-inflections; ANI: all-nominals-and-inflections.

2.1.4. Verb transitivity
Following previous experimental studies on syntactic bootstrapping, we classified each finite verb according to its transitivity. Although transitivity is a complex notion that concerns several features of the clause (Hopper and Thompson Reference Hopper and Thompson1980), we categorized verbs based on their typical syntactic subcategorization frames (Jackendoff Reference Jackendoff1990, Fisher et al. Reference Fisher, Gleitman and Gleitman1991; Levin Reference Levin1993) and their case-marking behaviour. Thus, verbs were classified as:
1. Intransitives: if they usually occur in the scheme subject + verb;
2. Transitives: if they usually occur in the scheme subject + verb + direct complement. (Only verbs which assign accusative case to their internal argument were classified as transitives);
3. Ditransitives: if they usually occur in the scheme subject + verb + direct complement + indirect complement.
There are no available databases with detailed information about the syntactic behaviour of individual verbs in the variety of Spanish spoken in Argentina. Hence, we hand-coded 30% of the occurrences of verbs with a frequency greater than 10, and 100% of the occurrences of verbs with a frequency below 10 (a total of 2,208 utterances). The authors read the utterances with the purpose of identifying which meaning of the verb was at play. For instance, the verb pasar can be used either as an intransitive verb (meaning ‘happen’ or ‘go by’) or as a ditransitive verb (meaning ‘hand something to somebody’). Once the meaning of the verb was identified, the authors determined the transitivity value on the basis of their native knowledge of the language and sometimes consulted with another source (Real Academia Española 2019).
In two cases, verbs were not ascribed to any category and the utterance was discarded: (a) the utterance provided only partial or incomplete information such that it was not possible to decide which category the verb belonged to; (b) due to parsing errors, verbs in the utterances were incorrectly identified as such. Ambiguous utterances, in which verbs could be interpreted as belonging to different syntactic categories, were assigned to the class requiring fewer nouns. As the number of nominals tends to be less than, rather than more than, the quantity required by the verb, this decision aimed at setting a favorable test-field for the heuristic under study.Footnote 7
To assess the reliability of the coding process, the authors independently coded 20% of the hand-coded sample. Inter-rater agreement was strong, as indicated by Cohen's kappa correlation coefficient (κ = .94, 95% CI [0.91, 0.98]).
In the next step, we generalized the pattern found in the hand-categorized portion to the remaining 70% of the utterances in the sample. For example, if (out the 30% of the utterances manually coded) a verb occurred 20% of the time in a transitive frame and 80% in an intransitive frame, the remaining 70% utterances were automatically assigned a class tag replicating that distribution.
2.2. Procedure
Data processing and statistical analyses were conducted using the R (R Core Team 2019) packages: caret (Kuhn Reference Kuhn2020), ggplot2 (Wickham Reference Wickham2016), graphics (R Core Team 2019), psych (Revelle Reference Revelle2019), stats (R Core Team 2019), vcd (Meyer et al. Reference Meyer, Zeileis and Hornik2020) and yardstick (Kuhn and Vaughan Reference Kuhn and Vaughan2020). For each of the four noun parsing conditions, we calculated how many words were tagged as nominals in every utterance, as shown in the examples of Table 2.
Table 2: Example calculation of the amount of nominal elements in each parsing condition. SN: stimuli-nominals; AN: all-nominals; SNI: stimuli-nominals-and-inflections; ANI: all-nominals-and-inflections.

The nouns were counted separately, disregarding whether they were part of a bigger structure. There is some experimental evidence suggesting that, at this early age, children interpret every noun in an utterance as an argument of the verb with a certain semantic role, without taking their position into account (e.g., Bavin and Growcott Reference Bavin, Growcott, Perkins and Howard2000). As a result, every utterance was tagged for the number of nominals it contained.
2.2.1. Analysis of overall performance
We assessed the performance of a classifier that categorizes verbs into classes based on the number of nominals in the utterance by measuring the degree of agreement between the class predicted and the actual class of the verb. We did so in each of the four noun-parsing conditions and over the two samples: the hand-coded sample and the complete sample (i.e., hand-coded 30% plus automatically-coded 70%). As a first step, we built confusion or error matrices that report the frequency of each of the four possible outcomes: true positives (TP, i.e., hits), true negatives (TN, i.e, correct rejections), false positives (FP, i.e., false alarms) and false negatives (FN, i.e., misses). On this ground we calculated the following widely-used measures of overall performance:
1. Recall, precision and F1 score
2. Overall accuracy
3. Cohen's kappa and Matthews correlation coefficient
Recall – also known as hit rate, sensitivity or true positive rate – measures the proportion of actual positives that are correctly identified as such. In other words, it reports the degree of sensitivity of the classifier to the event of interest. Recall = 1 means that the model predicts all actual positives as such (and does not generate any misses or FNs). Precision – also known as positive predictive value or true discovery rate – assesses the proportion of predicted positives that are truly positive in the reference sample. Precision = 1 means that the model does not generate any FP or false alarms. These two measures share an inverse relationship with each other: improving the precision score often results in lowering the recall score, and vice versa. In turn, the F1 score combines precision and recall. Classifiers with higher F1 scores detect most of the actual positive observations (high recall) and refrain from predicting as positive observations that are actual negatives (high precision). F1 score = 1 means that recall and precision are at ceiling and, thus, perfectly balanced. Just like recall and precision, the F-score does not take the TNs into account. These three measures were macro-averaged across classes.
Accuracy indicates how many observations, both positive and negative, were correctly classified (i.e., agreements between actual and predicted) out of the total number of observations. This measure considers all four possible outcomes in the confusion matrix and provides information about the overall effectiveness of the classifier. Accuracy = 1 means that none of the observations were misclassified. We compared overall performance between nominal parsing conditions pairwise by the use of McNemar's statistical tests (Dietterich Reference Dietterich1998). An exact binomial test was computed to assess whether the overall accuracy rate was significantly higher or lower than the ‘no-information rate’ (NIR, i.e., the accuracy rate yield by a naive classifier that always predicts the most frequent outcome).
Cohen's kappa was calculated to compare the obtained agreement between predicted and actual values against chance agreements expected by class frequencies. If κ is zero, the classifier performs no better than a chance prediction. Values close to −1 and +1 indicate performance much worse than chance, and much better than chance, respectively. As some concerns have been raised regarding the use of kappa as a performance measure in classification (Delgado and Tibau Reference Delgado and Tibau2019), we also provide Matthew's Correlation Coefficients (MCC).
2.2.2. Analysis of performance by verb class
One-versus-all confusion matrices were built for each verb class (i.e., intransitive, transitive and ditransitive) in all the parsing conditions and analyzed samples combined, i.e., the hand-coded 30% plus the automatically-coded 70%. Then, we calculated the prevalence or frequency of each verb class in the total sample and compared it against the detection prevalence or frequency of predicted verb classes. In addition, we assessed the performance of the classifier on each verb class, and across parsing conditions and samples by calculating the recall, precision and F1 scores for intransitive, transitive and ditransitive verbs individually.
3. Results
This section details the results of the analyses described in section 2.
3.1. Performance overall
Since the hand-coded and complete samples display similar results, this section will focus on the former. Figure 1 plots the confusion matrix obtained after classifying every verb in the hand-coded sample according to the number of nominals they occur with. In the four parsing conditions, many observations fall outside the diagonal that shows matching actual and predicted classes (i.e., [I, I], [T, T] and [D, D]), thus performance is rather low. In addition, nominals tend to be insufficient, as suggested by the fact that many transitive and ditransitive verbs are classified as intransitives in the conditions without agreement morphology (in SN and AN). Adding agreement morphology (in SNI and ANI) improves the performance, since transitive and ditransitive verbs are not classified as often as intransitives, and the size of the blocks that make up diagonal of matching actual and predicted classes increases – although not for all verb classes (see section 3.2, Performance by verb class).
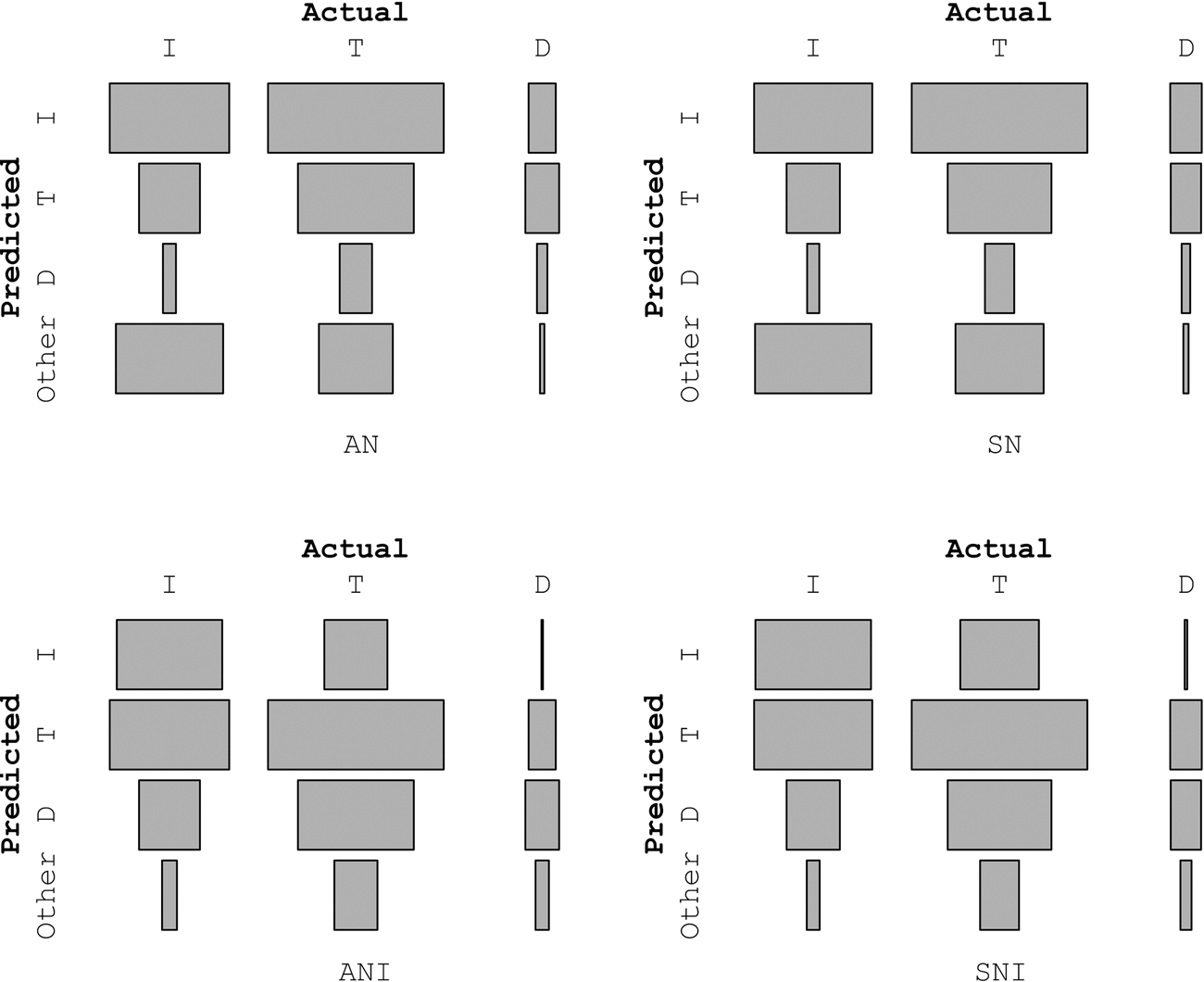
Figure 1: Confusion matrix of verb classification across nominal parsing conditions (hand-coded sample). I: Intransitives; T: Transitives; D: Ditransitives.
SN: stimuli-nominals; AN: all-nominals; SNI: stimuli-nominals-and-inflections; ANI: all-nominals-and-inflections.
Note: True (i.e., actual) verb classes are displayed as columns and predicted verb classes as rows.
3.1.1. Macro-recall, macro-precision and macro-F1 scores
Table 3 shows the performance measures for each of the conditions and analyzed samples. Conditions without inflections (SN and AN) behave similarly and perform much more poorly than conditions with inflections (SNI and ANI), which also behave alike. In addition, parsing all the nominals (AN and ANI) helps achieve only slightly higher macro-recall and macro-precision rates than parsing exclusively the nominals used to build experimental stimuli (SN and SNI).
Table 3: Performance measures of verb classification across nominal parsing conditions and analyzed samples. SN: stimuli-nominals; AN: all-nominals; SNI: stimuli-nominals-and-inflections; ANI: all-nominals-and-inflections.
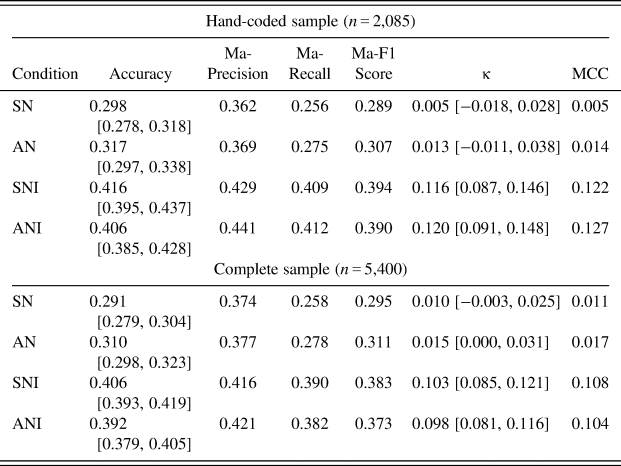
Note: 95% CI are indicated between square brackets.
Less than 30% of the TPs were classified as such in SN and AN. In conditions with inflections, this percentage increases to 40%. Thus, macro-recall scores indicate that less than half of the TPs are detected in the conditions analyzed. Although macro-precision scores are slightly higher than macro-recall scores, they are low too: in all the conditions FPs always exceed TPs. Around 35% of the detected positives are TPs in conditions without inflections. Once agreement morphology is included, the classifications reach macro-precision rates of 45%.
The fact that precision is always higher than recall – i.e., classification yields more FNs than FPs – suggests that in general the threshold chosen to classify verbs is more conservative than liberal. In other words, the number of nominals required to detect verb categories is rather demanding. In conditions with agreement morphology, in which the required number of independent NPs is lowered, macro-precision and macro-recall scores are closer to each other.
Macro-F1 scores indicate that the SNI condition achieves the highest performance, closely followed by the ANI condition. In turn, the poorest performance is observed in the SN condition.
3.1.2. Overall accuracy
In every condition analyzed, overall accuracy is less than 50%. In other words, more than half of the utterances were incorrectly classified. By comparing the parsing conditions, it appears that SN (.29) and AN (.31), on the one hand, and SNI (.41) and ANI (.40), on the other, perform similarly. However, the proportion of errors is significantly higher in AN compared to SN, as indicated by McNemar's test (X 2 (1) = 13.342, p < 0.001). On the other hand, while classification accuracy is higher in SNI than ANI, their proportion of errors is not significantly different (X 2 (1) = 2.694, p = 0.100).Footnote 8 In general, SNI and ANI significantly outperform conditions without agreement morphology: ANI > AN (X 2 (1) = 22.666, p < 0.001), ANI > SN (X 2 (1) = 34.439, p < 0.001), SNI > AN (X 2 (1) = 30.541, p < 0.001), and SNI > SN (X 2 (1) = 40.285, p < 0.001). Just as the F1 scores indicated, the highest accuracy is achieved by SNI, closely followed by ANI, and the lowest accuracy is achieved in the SN condition.
To further understand the performance of the classifier, it is informative to compare its performance against a no-information rate (NIR). In our sample, transitive verbs have the highest frequency: they account for 51% of the observations. Thus, a naive classifier that labels every observation as transitive already performs better than a classifier that considers the number of nominals in the utterance. Indeed, one-tailed binomial tests showed that classification performance in our four parsing conditions is significantly worse than the NIR (p-value < 2.2e-16).
3.1.3. Cohen's kappa and MCC coefficients
As verb syntactic classes are not equally frequent in the input, we have also calculated other measures such as Kappa and MCC coefficients. These allow us to assess how much better the classification is performed under each condition analysed (SN, AN, SNI, ANI) compared to the performance of a classifier that simply assigns verb classes randomly according to the frequency of each class. Both measures yielded similar results (see Table 3), so only the former are mentioned here. In SN (κ = .005) and AN (κ = .01) the kappa coefficients are close to zero. In fact, κ = 0 and even κ < 0 are part of the 95% confidence intervals. Thus, we cannot affirm that the accuracy achieved in these conditions is not due to chance. In SNI (κ = .11) and ANI (κ = .12), the accuracy is greater than chance and zero lies below the lower confidence limit. Still, performance is really very poor, as κ < .2, which means there is only slight agreement between actual and predicted classifications.
3.2. Performance by verb class
The following sections detail the performance of our models by transitivity class with and without inflections.
3.2.1. Prevalence, detection prevalence and detection rate
As shown in Table 4, the prevalence or frequency of the positive event in the sample varies among verb classes. Transitives are the most frequent and intransitives are fairly frequent as well, with the former accounting for 10% more occurrences than the latter. The occurrence of a ditransitive verb, on the other hand, is a rare event in the sample: only 9% of the utterances contain a ditransitive verb. This is apparent in Figure 2, in which the fourth quadrant – representing TNs – of ditransitives’ one-versus-all confusion matrix is much larger than the rest.
Table 4: Performance measures of verb classification across verb classes, nominal parsing conditions and analyzed samples. SN: stimuli-nominals; AN: all-nominals; SNI: stimuli-nominals-and-inflections; ANI: all-nominals-and-inflections.

Note: 95% CI are indicated between square brackets.

Figure 2: Outcome of verb classification according to verb class and nominal parsing condition (hand-coded sample). SN: stimuli-nominals; AN: all-nominals; SNI: stimuli-nominals-and-inflections; ANI: all-nominals-and-inflections.
Note: Values C (= Class) and O (= Other) are relative to each class. For instance, for intransitive verbs in the first column, “C” stands for “Intransitive” and “O” for “Transitive/Ditransitive”.
By comparing the detection prevalence against the actual prevalence, we can anticipate that transitive verbs in all four parsing conditions are underdetected. Their detection prevalence increases by adding inflections as suggested by the different number of TPs in the second quadrant of the conditions with and without inflections in Figure 2. Ditransitives are underdetected in conditions SN and AN. After adding agreement morphology, their detection prevalence exceeds by far their actual prevalence, anticipating many FPs as well as TPs (compare the first quadrants of ditransitives between conditions with and without inflections in Figure 2). In contrast, for intransitive verbs, their actual prevalence is lower than their detection prevalence – which yields many TPs but also FPs in conditions SN and AN as shown in the first quadrants in Figure 2. The opposite is observed after adding agreement morphology.
3.2.2. Recall, precision and F1 score
Parsing conditions without inflections (AN and SN) yield similar recall, precision and F1 scores. The same happens in the case of conditions with inflections (SNI and ANI).
Whenever agreement morphology is not counted (in SN and AN conditions), intransitive verbs obtain the highest recall scores, ditransitive verbs the lowest, and transitives lie somewhere in the middle. In addition, transitive verbs yield the highest precision scores, ditransitive verbs the lowest scores, and intransitive verbs fall between them. With the exception of ditransitive verbs, these precision scores were expected considering the lower (more liberal) or higher (more conservative) threshold required for each class. Compared to intransitive verbs, transitive verbs need to reach a higher threshold to be classified as such (i.e., two nominals). As a counterpart of having a higher threshold – and thus higher precision scores – the classification of transitive verbs yields more FNs than the classification of intransitive verbs, which, as we have mentioned, lowers their recall. The classification of intransitive verbs produces more TPs, as well as more FPs. Ditransitives are the exception: although their threshold is the highest, their precision is the lowest. The combination of these measures, that is, the F1 score, indicates that the classifier performs similarly when classifying intransitive and transitive verbs (AN) or slightly better in the case of intransitives (SN). Once again, ditransitive verbs display the lowest F1 score.
By adding inflections (in conditions SNI and ANI), recall scores are expected to improve in general since more NPs are being counted. This is especially so in the case of ditransitive verbs: in SNI they obtain the second highest score after transitives and in ANI the highest score, almost equal to the score of transitives. Transitive and ditransitive verbs, that in conditions without inflections (SN and AN) were frequently classified as intransitives (see Figure 1), are much better classified (see transitive and ditransitive TPs in Figure 2) once agreement inflections are counted. Interestingly, the recall scores of intransitive verbs do not improve after adding agreement morphology into the count. In fact, they yield the lowest scores among the three classes (see remarks on Figure 3 below). With regard to precision scores, the results obtained by intransitive and transitive verbs in the conditions without agreement morphology are now swapped: intransitives show the highest precision rates. In contrast, adding inflections does not increase precision when it comes to classifying transitive verbs. Ditransitive verbs in turn obtain the lowest precision scores. In general, F1 scores improve after adding inflections. Transitive verbs obtain the highest score, closely followed by intransitives, while ditransitives show the lowest scores.

Figure 3: Frequency of utterances with different numbers of nominals (across verb classes and nominal parsing conditions) (hand-coded sample)
SN: stimuli-nominals; AN: all-nominals; SNI: stimuli-nominals-and-inflections; ANI: all-nominals-and-inflections.
On the whole, agreement morphology improves the precision of the model when classifying intransitive verbs, but maintains their recall score. In the case of transitive verbs, recall scores increase while precision scores stay equal. Finally, recall scores for ditransitives improve greatly after adding agreement morphology, but precision stays the same as in the conditions without inflections.
To understand the different behaviour among verb classes it is useful to examine the distribution of utterances containing each verb class in terms of their number of nominal elements in Figure 3.
As both utterances with zero and one nominal are balanced and highly frequent in the case of intransitives verbs, adding inflections yields many FNs. It is also noticeable that the proportion of utterances with one independent nominal stays constant across verb classes (see SN and AN in Figure 3). Instead, utterances with zero nominals decrease as we transition from intransitive to ditransitive verbs, and the opposite is true as regards utterances with two, three, four and more nominals.
4. Discussion
The purpose of this investigation was to explore the performance of the structure or analogical mapping mechanism (SAMM) on naturalistic input to children learning Spanish. This heuristic was previously assessed in experimental designs, in which the researcher can resort to simple syntactic constructions and prototypical referential expressions to build the linguistic stimuli. Yet in real speech, people make use of the entire complexity of the linguistic system to communicate. In this study, we have explored the performance of the SAMM on real speech produced in the environment of young children and, on that basis, we discuss its bearing on verb learning. As we still need to learn how children under the age of two interpret and parse referential expressions, we considered four noun-parsing conditions: the stimuli-nominals condition (SN) (which was minimally demanding and resembled the experimental stimuli over which the SAMM was previously assessed), the stimuli-nominals-and-inflections condition (SNI), the all-nominals condition (AN), and the all-nominals-and-inflections condition (ANI). The last two probably overestimate children's knowledge at early stages in development. Additionally, we have analyzed the performance of the SAMM on different verb classes: intransitive, transitive and ditransitive.
In regard to the proposed parsing conditions, in general we found that conditions without agreement morphology (SN and AN) perform similarly, as was also the case for conditions with agreement morphology (SNI and ANI). The parallel behaviour of conditions with stimuli nominals and with all the nominals suggests that adding independent NPs over which the SAMM was not tested does not change the performance of the classifier noticeably. Thus, whether children parse all the nominals or not would not affect the performance of the mechanism on naturalistic data. On the contrary, the results showed that adding agreement morphology greatly improves the performance measures. So, although we may not be concerned with assessing experimentally whether children consider other independent (non-argument) NPs, it is truly important to know whether they factor in agreement morphology.
The measures that evaluated the performance of the classifier in each of the four parsing conditions showed that performance is poor, especially in the conditions that do not include agreement morphology. In all the conditions overall accuracy is less than 50% (i.e., more than half of the utterances are incorrectly classified). Moreover, classification performance is significantly worse than the no-information rate: interpreting every verb as transitive without paying attention to the number of nominals yields a better performance (51% accuracy). As indicated by F1 scores and overall accuracy, the SNI condition achieves the highest performance and the SN condition, including only experimental stimuli, the lowest. Kappa scores revealed that agreement (i.e., accuracy) in SN and AN conditions is not greater than agreement by chance. In SNI and ANI conditions, the agreement is greater than chance, but still, performance is very poor. Results not better than chance translate into a situation in which the child assigns verbs to syntactic classes randomly. Such an outcome leads us to think that the SAMM plays only a minor role in spontaneous verb exchanges.
Recall and precision scores were also considered. The former indicate that less than half of the TPs are detected as such in all the conditions analyzed. Precision is slightly higher but is still low (always under 50%). The fact that precision is higher than recall suggests that the threshold chosen to classify verbs in all the conditions is high. The number of nominals required to detect verbs is rather demanding, especially when agreement morphology is not considered. In natural exchanges, the missing NPs can be recovered from contextual and interactional cues, such as gestures or gaze direction. In that respect, it has been shown that children as young as 13 months old understand the referential nature of deictic gestures (and use them as cues to the meaning of co-occurring words; see Baldwin Reference Baldwin1993, Gliga and Csibra Reference Gliga and Csibra2009) and take part in episodes of joint attention (Tomasello Reference Tomasello, Moore and Dunham1995).
The fact that parsing conditions without inflections (SN and AN) achieve low or barely adequate outcomes probably relates to the typological features of Spanish, an inflectional language which allows the expression of some grammatical categories (e.g., person and number) as verb suffixes. As a pro-drop language, the subject does not need to be overtly expressed and can be recovered from the verb form. Utterances with zero nominals (i.e., with agreement morphology only) are allowed. Thus, by considering agreement morphology, performance improves considerably. However, research on the SAMM has rarely assessed children's interpretation of verb inflections for person agreement, since they were mostly conducted in English, which has an impoverished verb morphology. Some studies (Göksun et al. Reference Göksun, Küntay and Naigles2008, for Turkish; Lidz et al. Reference Lidz, Gleitman and Gleitman2003, for Kannada), however, investigated the effect of other verb morphemes and suggested that causative morphemes (unlike case markers) have no bearing in the causative interpretation of verbs.
The little evidence available about the acquisition of agreement morphology in Spanish suggests we should consider its bearing on the process of verb learning postulated by the SAMM with caution. For example, some studies have found that the acquisition of verb morphology in Spanish takes place gradually. Children build verb inflection paradigms piece by piece. For instance, they start producing a person contrast in one tense but not in others. Hence, a single moment in development cannot be established when Spanish children learn the category of “person” or “number” (e.g., Aguirre Reference Aguirre, Bittner, Dressler and Kilani-Schoch2003, Mueller et al. Reference Mueller Gathercole, Sebastián and Soto1999).Footnote 9 Thus, we ought to say that parsing conditions ANI and SNI (that assume a generalized mastery of verb inflections) overestimate what children know about morphology at the age of 19 months. We know that children this age produce some person and number contrasts on the verb only in some cases (e.g., with some tenses) and that after the second year there is a drastic growth in the production of these forms.
The weak performance of the SAMM over naturalistic input is related, in part, to the assumption that the child exclusively parses nouns before tackling verbs. In contrast, previous investigations have shown that children not only pay attention to nouns in order to recover syntactic information and the meaning of unknown words: they can also use function words and phrasal prosody (Soderstrom et al. Reference Soderstrom, Blossom, Foygel and Morgan2008, de Carvalho et al. Reference de Carvalho, He, Lidz and Christophe2019). In addition, proposals such as Rispoli (Reference Rispoli2019) suggest a developmental sequence of syntactic learning not determined by the acquisition of certain grammatical categories before others (e.g., nouns before verbs) but by the addition of syntactic layers to clause structure.
The second part of the study focused on investigating whether the SAMM performs differently according to verb classes (transitive, intransitive, and ditransitive) in the four analyzed conditions. One contribution of this study is the inclusion of ditransitive verbs, which have not been considered in previous studies of the SAMM (e.g., Fisher Reference Fisher2002, Lee and Naigles Reference Lee and Naigles2008). In conditions without agreement morphology (SN and AN), our results indicate that intransitive verbs, followed by transitives, are the most frequently identified and yield the highest recall, while ditransitives yield the lowest scores. The highest amount of FPs – and thus, the lowest precision – is produced in classifying ditransitive verbs, and the scarcest in classifying transitives. F1 scores indicate that in these conditions the performance of intransitive and transitive verbs is equivalent, although for different reasons. Intransitive verbs yield a higher recall score but are less precisely classified than transitive verbs which, in turn, yield a lower recall score but are more precisely classified than intransitives. Last, the F1 score of ditransitive verbs is the lowest.
When agreement morphology is factored in (in conditions SNI and ANI), transitive and ditransitive verbs are more frequently identified, as shown by the fact that their classification produces more TPs (i.e., higher recall). Intransitives achieve the same recall as in conditions without inflections. The highest precision is reached by intransitive verbs, transitives achieve a slightly lower precision, and ditransitive verbs the lowest (i.e., ditransitives yield the highest number of FPs). Finally, intransitive and transitive F1 scores are the highest and ditransitive the lowest, just as was observed in conditions without agreement morphology.
On the whole, the assessment of different verb classes shows that agreement morphology has a differential impact according to the class under consideration. Although in all the conditions parsing more nominals improves the performance of the classification overall, this is only specifically true for the classification of transitive and ditransitive verbs. Adding verb inflections is detrimental to the classification of intransitives. This is likely due to the fact that intransitives tend to occur frequently with overt subjects, and thus, adding inflections exceeds the number of nominals required. In contrast, transitive and (mainly) ditransitive verbs do not frequently occur with overt subjects, and take more arguments. Thus, it is not surprising that adding inflections is beneficial for the latter two classes. Secondly, the inverse relationship between intransitive and transitive verbs regarding recall and precision scores is a result of the different threshold or number of nominals required to classify them as one or the other. This yields equivalent performances as indicated by the F1 scores of these classes. In contrast, ditransitive verbs display an unexpected behaviour: their precision is the lowest, although their threshold is the highest.
The fact that the performance of the SAMM varies according to verb class could relate to a difference in the pace of acquisition. However, the available evidence on this matter is sometimes contradictory and varies among languages. Some studies (e.g., De Bleser and Kauschke Reference De Bleser and Kauschke2003 for German) found that intransitive verbs are generally acquired earlier than transitive ones, while others (e.g., Davidoff and Masterson Reference Davidoff and Masterson1995 for English) found the reverse pattern. More studies on different languages are needed to gain conclusive evidence on this subject.
As a limitation to this study, we should mention that the identification of nouns and verbs was not accomplished in stages: from the beginning, every verb and every noun (according to the parsing condition) was identified and considered in the classification. This does not align with the possibility that children need to hear a novel verb several times before learning it. In addition, the classification of a verb does not take into account information gathered in previous encounters with that verb. In the future, we could implement an incremental learning model (Christodoulopoulos et al. Reference Christodoulopoulos, Roth, Fisher, Korhonen, Lenci, Murphy, Poibeau and Villavicencio2016) and test it on our sample of naturalistic data, which contains lengthier utterances than those over which the SAMM was previously assessed (e.g., Connor et al. Reference Connor, Gertner, Fisher, Roth, Clark and Toutanova2008). In addition, the SAMM could be assessed on variation sets (e.g., Küntay and Slobin Reference Küntay, Slobin, Slobin, Gerhardt, Kyratzis and Guo1996), which have been shown to maximize children's comprehension.
We should return now to our initial question, namely how does the SAMM perform on naturalistic input. Before answering, a further remark is in order. Given that the use of the SAMM is only warranted on one-predicate utterances, it could only be applied to 30% of our naturalistic data, that is to say, a small portion of children's input. Overall, the performance in the four parsing conditions is poor. The parsing condition that most closely resembles the stimuli of the studies that have investigated the SAMM experimentally achieves the worst performance (i.e., SN). Still, in all the conditions – and especially in those without agreement morphology – children would arrive at inaccurate interpretations more than half of the time. We might ask then what is the contribution of the SAMM to verb learning? In that respect, the results presented in this study do not allow us to assert that young children acquiring Spanish strongly rely on the SAMM when they start learning verb meanings in natural linguistic settings. On the contrary, the results pose several doubts regarding the degree to which such a mechanism contributes to early verb learning. In principle, it suggests that other mechanisms or cues are involved as well and/or that in natural linguistic interactions, the SAMM operates under certain restrictions.
In essence, the SAMM comes down to the discovery of the referential nature of nouns which are regarded as potential participants in the event expressed by the verb. This is a necessary step in order to use syntax to learn verb meanings, but we cannot take it for granted that children learning Spanish use the SAMM in real exchanges, because our study has shown that it is not reliable. Other sources of information – prosody, function words – are also necessary to recover the syntactic structure of sentences which, once recovered, might guide the identification of some aspects of verb meaning, as suggested by classical studies in the framework of syntactic bootstrapping (SB).
Although this study mainly addresses some problems within the SAMM framework in particular and the SB in general, there are broader challenges to these approaches, importantly, as regards their relationship with linguistic theory. It has been claimed that experiments that have studied syntactic bootstrapping are compatible with both Lexical Projectionist and Non-projectionist representations of syntactic structure, assuming sometimes one type of approach and sometimes the other (Arunachalam Reference Arunachalam, de Almeida and Manouilidou2015). Therefore, solving problems such as what kind of learning is promoted by these mechanisms (semantic or syntactic), how do predicates and syntax relate to each other in language acquisition, etc. are crucial to our understanding of what these mechanisms actually account for.