1 Introduction
Ontology is an agreed and shared formal representation of knowledge, a model of formal specification regarding naming and definition of types, properties and interrelationships of entities that exist in a particular domain of discourse. The well-known formal definition of Gruber on ‘formal explicit specifications of shared conceptualizations’ has been revised and explained in detail (Guarino & Oberle, Reference Guarino and Oberle2009), emphasizing the importance of sharing (agreed) ontologies among stakeholders and communities of practice and knowledge, especially for ontologies intended to support large-scale data/information interoperability, sharing of information and ontology-supported processes such as data integration from heterogeneous data sources, simulation of systems, tracking of behaviour of multicomponent systems via component interrelationships, data analytics and collaborative decision making, to mention some. Ontology engineering (OE) studies the methods and methodologies for developing ontologies and supporting their evolution through their life cycle.
Given the requirements for the ontologies that must be developed in the context of a problem-solving task, we conjecture that, ideally, an ontology engineering methodology (OEM) must support all involved stakeholders during the ontology life cycle, that is, from the development of seed ontologies to the continuous evolution and maintenance of ontologies, keeping them ‘live’ to shape knowledge.
Starting from this conjecture and based on several surveys on OEMs (Uschold & Gruninger, Reference Uschold and Gruninger1996; Fernández-López & Gómez-Pérez, Reference Fernández-López and Gómez-Pérez2002; Corcho et al., Reference Corcho, Fernández-López and Gómez-Pérez2003; Iqbal et al., Reference Iqbal, Murad, Mustapha and Sharef2013; Stadlhofer et al., Reference Stadlhofer, Salhofer and Durlacher2013; Simperl & Luczak-Rösch, Reference Simperl and Luczak-Rösch2014; Yadav et al., Reference Yadav, Singh Narula, Duhan and Jain2016), we focus on methodologies that do meet the following two major requirements that are widely accepted from the OE research community:
(a) Emphasize on the active and decisive involvement of knowledge engineers, workers and domain experts in all phases of OE, that is, in the phases of ontology specification, ontology development, ontology exploitation and ontology evaluation.
(b) Support, via appropriate tooling, a collaborative approach in ontology specification, development, exploitation and evaluation phases, engaging all stakeholders in close collaboration towards shaping commonly agreed knowledge.
It must be emphasized that, beyond the above-stated minimum set of requirements, due to the large volumes of available data being created, the focus has been shifted on requirements regarding the support of a data-driven approach of ontology development: This requisite implies the incorporation of ontology specifications according to the form of data, aiming to associate these specifications to knowledge provided by domain experts towards understanding data, assessing their quality, as well as to bridge heterogeneity among data sources. The incorporation of that requirement in methodologies implies additional steps at early phases of ontology development (e.g., creating a seed ontology), at later phases (e.g., shaping domain knowledge to reflect and integrate data in the forms that it exists) and during the evolution of the ontology (e.g., incorporating new forms of data and/or updating existing), while in many cases emphasizing further the role of knowledge workers, who aim to exploit data in their operational setting. Such a focus is necessary to ensure the wide use of ontologies and their effectiveness to support data-driven processes.
Furthermore, the focus to the importance of exploiting and evaluating the developed ontologies as part of the day-to-day practice remains as an important phase in the ontology life cycle. Ontologies, as they formally shape conceptualizations of the involved stakeholders, are placed in real knowledge creating and consuming environments and under the criticism of their community members who put them under the prism of their own knowledge and experience and/or put them in the context of their own processes (e.g., exploiting them to support knowledge creation and consumption via analytics and decision making). Ontology exploitation and evaluation may result in the discovery of new meanings since concepts are seen under the view of new and evolving contexts (e.g. new data sources).
Last but not least, an OEM must integrate distinct tasks and related tools that support (a) informed participation in argumentations/discussions on issues regarding the ontology specifications and their evolution, (b) tracking of the development history of ontologies at the level of individual concepts/properties/axioms and (c) management tasks such as importing, comparing, aligning and merging shared specifications, populating ontologies with data from heterogeneous data sources, to accelerate the development and facilitate the evolution processes.
In this paper, the following categorization of OEMs is introduced:
Collaborative OEM: a clearly and systematically defined (in phases, tasks and workflows) OEM which emphasizes the active and decisive involvement of knowledge engineers, knowledge workers as well as domain experts in all phases of OE (specification, implementation, exploitation and evaluation) via their close and continuous collaboration, towards shaping commonly agreed knowledge. Representative examples are HCOME (Kotis & Vouros, Reference Kotis and Vouros2006), DILIGENT (Vrandečić et al., Reference Vrandečić, Pinto, Tempich and Sure2005), DOGMA-MESS (de Moor et al., Reference de Moor, De Leenheer, Meersman, Schärfe, Hitzler and Øhrstrøm2006), NeOn (Pérez et al., Reference Pérez, Baonza and Villazón2008), GOSPL (Debruyne et al., Reference Debruyne, Tran and Meersman2013) and SAMOD (Narula et al., Reference Narula, Yadav, Duhan and Jain2018).
Non-Collaborative OEM: an OEM that does not emphasize the collaboration of stakeholders towards engineering commonly agreed ontologies, still it clearly defines phases, tasks and workflows of the OE approach, in a systematic and formal way. Representative examples are METHONTOLOGY (Fernández-López et al., Reference Fernández-López, Gómez-Pérez and Juristo1997), Uschold & King (Reference Uschold and King1995), On-To-Knowledge Methodology (OTKM) (Sure et al., Reference Sure, Staab, Studer, Staab and Studer2004) and FMCLGO (Giunchiglia et al., Reference Giunchiglia, Dutta, Maltese and Farazi2012).
Custom OEM: an OEM that does not necessarily define phases, tasks and workflows in a formal and systematic way, but still engage the active involvement of communities of practice and the use of tools (collaborative or not) towards developing ontologies in an agile, decentralized and most of the times collaborative manner. Most of those approaches use Wiki and GitHub technology as a mean to support tool-based distributed collaboration in the development of commonly agreed ontologies. Representative approaches are included in Rebele et al. (Reference Rebele, Suchanek, Hoffart, Biega, Kuzey and Weikum2016), Calbimonte et al. (Reference Calbimonte, Dubosson, Hilfiker, Cotting and Schumacher2017), Narula et al. (Reference Narula, Yadav, Duhan and Jain2018), Salatino et al. (Reference Salatino, Thanapalasingam, Mannocci, Osborne and Motta2018), Tommasini et al. (Reference Tommasini, Sedira, Balduini, Ali, Le Phuoc and Calbimonte2018) and in the recent related work of Arndt et al. (Reference Arndt, Naumann, Radtke, Martin and Marx2019).
In the following sections, we succinctly present an overview of the OEMs that meet the two major requirements: Not surprisingly, these are collaborative OEMs. However, it should be pointed out that under pragmatic conditions, custom methodologies used for engineering specific ontologies may satisfy these criteria (as will also be discussed in relation to specific ontology development efforts). Therefore, to review the impact of OEMs to the status of ontologies, we have selected a large set of well-known, widely used, published ontologies that claim a methodological approach for their development: The list is by no means inclusive, but, according to our knowledge, there is not any effort that deviates radically from the methodological aspects mentioned with respect to these ontologies.
Our aim is to (a) provide an insight on the impact of collaborative OEMs to the status of developed ontologies, also in contrast to the related impact of other methodologies, (b) update the OE research community on the current status and trends in OEMs, as these are shaped through practice and (c) propose a set of recommendations for ontology engineers to consider for the development and evolution of living and evolving ontologies. The paper does not emphasize the different types of OEMs or a comparison of OEMs to identify the best (if any). As stated in Keet’s OE textbook (pp 91), there are many different use-case scenarios, and it is scientifically hard to prove one methodology is better than another—it is better to pick one of them for developing an ontology than using none at all (Keet, Reference Keet2018).
The paper is structured as follows: Section 2 defines key terminology used in the paper and presents the collaborative OEMs that this survey emphasizes. Section 3 describes in detail the selected ontologies (SOs) used for the presented OE impact analysis and Section 4 discusses the results in terms of specific findings, trends and recommendations. Section 5 discusses related work on similar surveys on researching OEMs and OE tools, and Section 6 concludes the paper.
2 Collaborative OE methodologies
As already pointed out, we require that an OE methodology supports the active involvement of different roles during the ontology lifecycle, providing tools for their active participation in all stages of ontology lifecycle. The roles involved are the following:
(a) Domain experts: These are the persons who have the knowledge/expertise of the domain and/or data sources. These usually are practitioners, not acquainted with ontology languages, specifications, etc.
(b) Ontology engineers: These are the persons who have the knowledge/expertise to make ontological specifications and coordinate an OE task.
(c) Knowledge workers: These are the persons who exploit the ontology in ‘operational’ conditions, so as to solve problems, or perform data-driven analysis tasks. These may be domain experts but, in some cases, this is not necessary.
In addition to the roles, we require that an OE methodology supports the engineering of live, evolved and reusable ontologies. Towards analyzing and presenting this requirement, we characterize an ontology according to its status throughout its lifetime in three corresponding dimensions:
(a) Liveness: An ontology is live at a particular time instant if it is active at that instant. It may be under development, under evolution, maintenance or update, evaluation or in-use.
(b) Evolution: An evolved ontology is an ontology that has been evolved over time, that is, there are available more than one version, recorded during its life cycle.
(c) Reusability: A reused ontology is an ontology that has been used to specify other ontologies or used in applications/projects beyond its origin.
According to the above dimensions of the ontology lifetime, an evolved ontology may be dead, and a live ontology may have been not evolved yet. A reused ontology may be alive or dead, evolved or not. An ontology may have also use other ontologies for its specification but still not been reused by others. Authors propose those three dimensions (liveness, evolution, reusability) for their analysis, based on the requirements for OE as introduced and discussed in the Introduction section. Authors do not claim that these are the only dimensions possible for such an analysis, however, to be able to support the aim of the paper that is, to examine the impact of collaborative OEMs for the evolution of living and reused ontologies, these are the necessary requirements.
In the following, we provide a succinct overview of the most recent collaborative OEMs satisfying our basic requirements for the continuous evolution of living and reused ontologies. The descriptions focus also on characteristics related to tool-supported engineering of ontologies towards live, evolved and/or reused ontologies. Table 1 presents those methodologies in relation to how they meet those requirements and characteristics (labeled in Table 1 and cited in the OEM descriptions below, paragraphs OEM1 to OEM9), as discussed in the Introduction section.
Table 1 Collaborative OEMs and supported features
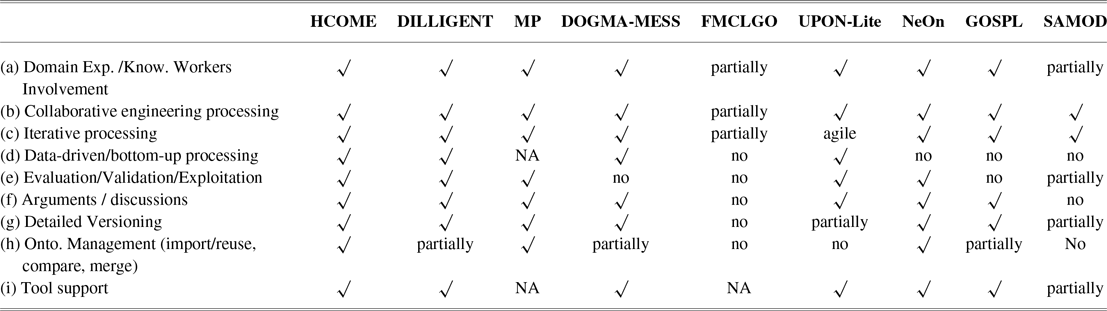
At this point it must be stated that a collaborative OEM generally comprises three main phases: (a) ontology specification, (b) ontology development and (c) ontology exploitation and evaluation phase. Different organizations of OE tasks into phases exist (e.g. as recently included in Keet’s OE textbook (Keet, Reference Keet2018: 92)); however, all include the development of the ontology as one of the OE phases, and the implementation task as an integrated task of this phase (the term ‘implementation’ is used interchangeably with ‘development’ in some cases). Also, the distinction between the isolated, single, stand-alone, ontology development vs. collaborative development of ontologies and ontology networks is highlighted, even in very recent related work (e.g., Keet (Reference Keet2018: 93)). Based on this discussion, we do not present approaches that emphasize only the development phase (and related task) of ontologies, as in data-driven (e.g. (D’Amato et al., Reference D’Amato, Fanizzi and Esposito2010)) or test-driven (e.g. (Maria Keet & Ławrynowicz, Reference Maria Keet and Ławrynowicz2016)) ontology development approaches. We conjecture that such development approaches should be viewed as integrated ontology development tasks in the development phase of a collaborative OEM. Thus, although those approaches are used for the development of ontologies, they should be examined as part (integrated tasks) of an OE methodological approach.
OEM1. The Human-Centered Collaborative Ontology Engineering Methodology (HCOME) (Kotis & Vouros, Reference Kotis and Vouros2006; Kotis & Papasalouros, Reference Kotis and Papasalouros2010) supports the decisive involvement of knowledge workers in an ontology life cycle. It supports a human-centred approach to the collaborative engineering of ontologies, where the active participation of knowledge workers (Table 1(a)) in the ontology life cycle, in close collaboration to domain experts and ontology engineers (Table 1(b)), is emphasized. Ontologies are managed according to the capacities of knowledge workers are developed individually as well as collaboratively and are put in the context of workers’ experiences and their working settings as an integrated part of a ‘knowing’ process. Leveraging the role of knowledge workers by their active participation in the ontology life cycle, this human-centred approach requires the use of tools that provide greater opportunities for managing and interacting with conceptualizations in a direct and continuous mode in threads of discussion, resolving various issues. An iterative approach to the execution of tasks (Table 1(c)) at all three phases (specification, conceptualization, exploitation/evaluation) is supported, emphasizing on tasks related to the argumentation/discussion of conceptualizations, detailed versioning of evolving specifications, and ontology management such as import/export, comparison and merging of ontological definitions. Leveraging the role of knowledge workers, HCOME (although not intended to that when proposed) emphasizes on the exploitation of data in operational contexts. In conjunction to that, a data-driven (bottom-up) conceptualization approach is also proposed (Table 1(d)) in the updated version of the HCOME methodology (Kotis & Papasalouros, Reference Kotis and Papasalouros2010), supported by learning seed ontologies (knowledge in that case is extracted from query logs). In terms of tooling support, the methodology was supported (until 2010) by a stand-alone OE integrated environment (namely, HCONE) to support management and versioning tasks in the personal space of knowledge workers (Table 1(i, g, h)), and a semantic MediaWiki-based shared environment (namely, Shared-HCONE) (Kotis et al., Reference Kotis, Papasalouros, Vouros, Pappas and Zoumpatianos2011) for supporting the shared space collaborative and evaluation tasks (Table 1(i, e, g)) and the argumentation-based discussion model (Table 1(f)). Currently, this gap of support is covered by related tools such as Protégé (for developing of local ontology versions) and Web-Protégé (for a collaborative editing experience) as well as by using e-mail lists and Google technology (groups and documents).
OEM2. Similarly to HCOME, the DIstributed, Loosely-controlled and evolving Engineering of oNTologies (DILIGENT) (Vrandečić et al., Reference Vrandečić, Pinto, Tempich and Sure2005; Pinto et al., Reference Pinto, Tempich, Staab, Staab and Studer2009) methodology focuses on the evolutionary life cycle of ontologies (Table 1(c)) and on a user-centric ontology development. The ontology development process begins with the involvement of different stakeholder groups (Table 1(a)), that is, domain experts, knowledge workers and ontology engineers, as they collaboratively build (Table 1(b)) the first version of an ontology (seed ontology). This version is the result of fast agreement (between all participants) on the high-level terms (Table 1(f)). Furthermore, knowledge workers begin to work with the ontology and locally adapt it—introducing subclasses—to their specific requirements. A control board is introduced, collecting requests for changes to the shared (core) ontology (Table 1(e)). The set of different local ontologies is analyzed by the control board, to find similarities (Table 1(h)). Based on this analysis, a new version of the shared ontology is introduced (Table 1(g)). The control board revises the shared ontology frequently. The ontology engineers are responsible for maintaining the ontology based on the decisions of the board. Knowledge workers are able to locally update the local ontologies by reusing new terms instead of using their previously defined local terms. As a result, ontology reuse (Table 1(h)) is maximized among all knowledge workers. As it is obvious, the approach emphasizes the distributed and collaborative construction of ontologies. As in HCOME, leveraging the role of knowledge workers supports the exploitation of data in operational contexts (Table 1(d)). In terms of tooling support, a standard Wiki is used to allow a traceable discussion between all participants (Table 1(i)). The agreed ontology is placed to the Wiki in order to visualize the ontology and ease the discussion upon it. Evaluation and validation processes are also supported. A data-driven (bottom-up) conceptualization approach (Table 1(d)), supported via appropriate tools (Table 1(i)) for ontology learning, is described at the conceptual level. Ontology management is partially supported.
OEM3. Melting Point (MP) (Garcia et al., Reference Garcia, Neill, Garcia, Lord, Corcho and Gibson2010) is a collaborative and human-centered methodology that has been engineered to support decentralised communities of practice for which the designers of technology and the users may be the same group of stakeholders. MP was developed by taking best practices from earlier methodologies. As in HCOME and DILIGENT methodologies, MP emphasizes the collaborative development (Table 1(b)) of ontologies by communities of practice (Table 1(a)), utilising the collective intelligence of the application domain. In addition, MP is not prescriptive about specific techniques or methods, since it recommends that ontology developers should consider the use of those that best suit their particular situation (Table 1(i)). Managerial activities happen throughout the whole ontology life cycle (Table 1(h)). The iterative interaction (Table 1(c)) amongst stakeholders ensures (a) the quality of the ontology (Table 1(e)) and (b) that a set of predefined control activities take place. This methodology has been shaped from experiences gathered by its authors as best practice within a real OE setting of bio-ontology development, reusing tasks from other methodologies. The recommendation of specific tools to support each task and phase of the methodology is missing.
OEM4. Ontology-Grounded Methods and Applications (DOGMA) (de Moor et al., Reference de Moor, De Leenheer, Meersman, Schärfe, Hitzler and Øhrstrøm2006) and its extension DOGMA-MESS (Leenheer & Debruyne, Reference Leenheer and Debruyne2008) follows a data-driven (bottom-up) approach to OE (Table 1 (d)). It is a collaborative OEM (Table 1(b) that supports the modeling of shared ontologies in stakeholders’ own terminology and context (Table 1(a). To accomplish that, four modes of knowledge conversion are introduced: socialization, externalization, combination and internalization. Technically, at the center of the approach is an Ontology Server, which is embedded in a central ontology evolution support system (Table 1(c)). There are three types of participants (Table 1(a): the core domain expert, the domain expert and the ontology engineer. The ontology evolution process is driven by social knowledge conversion modes. This process is iteratively performed (Table 1(c) until an optimum trade-off between differences and commonalities of organizational and common perspectives is reached. The development task is driven by data (Table 1(d)). Ontology management is partially supported (Table 1(h) via the import and identification of conflicts of ontological definitions. In terms of tooling support, the methodology was supported by DOGMA Studio (Leenheer & Debruyne, Reference Leenheer and Debruyne2008) in all its phases and tasks (Table 1(i, g)).
OEM5. A facet-based OEM for the development of large-scale geospatial ontologies in the space domain (FMCLGO) (Giunchiglia et al., Reference Giunchiglia, Dutta, Maltese and Farazi2012) has been proposed, with a minimal set of ontology development guiding principles inspired by the faceted approach, originally proposed for the library science. A facet is a hierarchy of homogeneous terms describing an aspect of the domain, where each term in the hierarchy denotes a different concept. The approach focuses on the domain and facet aspects, refining and extending existing resources including GeoNamesFootnote 1, WordNetFootnote 2 and the Italian part of Multi-WordNetFootnote 3. The ontology development process is manual in terms of identifying and categorizing all the terms denoting classes into facets, relations and attribute names. On the other hand, the process is automatic in terms of populating the ontology with entities and corresponding attribute values. The terms collected and disambiguated during the identification of the terminology phase were used as building blocks for the construction of the facets that eventually constitute the Space ontology (analysis and synthesis phases). This methodology follows a top-down approach to OE (Table 1(d)). The involvement of different roles in a collaborative processing at all steps (Table 1(b)), emphasizing the participation of domain experts and knowledge workers (Table 1(a)), is missing (apart from typical interviews of domain experts). Iterative processing (Table 1(c)) is limited at the stage of mapping resources to the ontology (by repeating all previous tasks), and this only if disagreements have been identified between engineers. Argumentation, exploitation and evaluation of the ontology are not part of the methodological tasks (Table 1(e, f)), and typical management tasks such as versioning, importing, comparing and merging ontological definitions are not discussed (Table 1(g, h)). In terms of tooling support, there is lack of information regarding specific tools proposed or used for each phase and task of the methodology (Table 1(i)).
OEM6. A recent methodology for lightweight and rapid OE is UPON Lite (De Nicola & Missikoff, Reference De Nicola and Missikoff2016). It has been designed for domain experts, knowledge workers and even casual users in the relevant business domain. The approach aims to reduce the role of ontology engineers, placing the responsibility for ontology development to the community of end-users (knowledge workers) (Table 1(a)). This is achieved through a participative social approach (Table 1(b, f)), supported by easy-to-use methods and tools (Table 1(i)). UPON Lite is designed for rapid ontology prototyping and is based on three main aspects: (a) a user-centered approach that accentuates the role of domain experts, (b) a social approach that accentuates the collective intelligence of domain experts to progressively (Table 1(c)) perform all tasks and (c) an ontology building process that is organized in well-defined steps. The process of ontology building and management is carried out on a social media platform. Provided examples are based on Google DocsFootnote 4 suite, in particular with shared Google Sheets for OE, in conjunction with Google Forms and Google+ for other functions (such as debating and voting). Argumentation and versioning support are proposed (Table 1(f, g)) and supported via inherited functionality of social media technology. In addition to these tools, MappingMaster text mining tool and Protégé OE integrated environment were in use. This methodology follows a data-driven (bottom-up) approach to OE. It involves different roles in a collaborative processing (Table 1(b)) at all steps, emphasizing the participation of knowledge workers, following an agile process (Table 1(c). Exploitation and evaluation of the ontology are included in the methodological tasks (Table 1(e)), but typical management tasks such as importing, comparing and merging ontological definitions are not discussed (Table 1(h)).
OEM7. A scenario-based OEM, NeOn (Pérez et al., Reference Pérez, Baonza and Villazón2008), emphasizes the development of ontology networks as well as the reuse of existing ontological and non-ontological resources to the development of an ontology. One of the key principles of this OEM is the involvement of developers and ontology practitioners at the same time (Table 1(a)). NeOn considers the existence of multiple ontologies in ontology networks, the collaborative development of ontologies (Table 1(b)) and the reuse and re-engineering of knowledge (Table 1(g, h)). NeOn definition has been based on the analysis of a set of nine ontology development scenarios. A data-driven conceptualization approach (Table 1(d)) is not reported. Also, argumentation/discussion (Table 1(f)) for supporting consensus/agreement reach is not explicitly reported, but the methodology was supported by the NeOn toolkit (Table 1(i)), which provided support for discussing issues (based on DILIGENT OEM) via a plugin that was connected to Cicero (Dellschaft et al., Reference Dellschaft, Engelbrecht, Barreto, Rutenbeck and Staab2008). Cicero was a dialogue management platform for keeping track of ontological discussions between ontology developers and users.
OEM8. Grounding Ontologies with Social Processes and Natural Language (GOSPL) (Debruyne et al., Reference Debruyne, Tran and Meersman2013) is a collaborative (Table 1(b)) ontology evolution (Table 1(c)) approach that has been based on the notion of hybrid OE and DOGMA-MESS (Leenheer & Debruyne, Reference Leenheer and Debruyne2008). A hybrid ontology is an ontology where concepts are described both in a formal and an informal manner, where context identifiers refer to communities supporting the social processes leading to agreements (Debruyne et al., Reference Debruyne, Tran and Meersman2013) (Table 1(f)). In hybrid OE, communities of practice are first-class citizens, meaning that the interactions within an evolving community result in series of ontology evolution actors (Table 1(a, c)). All the agreements reached in the involved communities are the result of social interactions (Table 1(f)). Natural language is central and vital to this approach. GOSPL supports involved stakeholders to interpret and model shared ontologies in their own terminology and context and provide feedback of the results to the community. Social processes are explicitly defined. GOSPL is discussion-oriented, that is, the ontology evolves only if the community is reaching an agreement (through a voting system) A data-driven conceptualization approach (Table 1(d)) is not reported. Ontology management is partially supported via the importing of ontologies and the identification of conflicts of ontological definitions (Table 1(h)). In terms of tooling support (Table 1(i)), there is support of a discussion-oriented tool to handle hybrid ontology description and glossary, discussions’ links, the management of communities, the commitments of applications to the ontology, the OWL implementation of the hybrid ontology and a voting system, among other provided functionalities.
OEM9. Very recently, SAMOD (Peroni, Reference Peroni2017) OEM has been proposed for simplified and agile development of ontologies. Such an approach specifies the steps of an iterative processing (Table 1(c)) focusing on the development of documented ontologies starting from typical examples of domain descriptions. This work has been partially inspired by test-driven development processes in software engineering. Although domain experts and ontology engineers are present, knowledge workers are not placed in the loop (Table 1(a)). Collaboration appears in between domain experts and ontology engineers at certain initial steps of the development process (Table 1(b)). The approach is not data driven, but it is driven by motivating scenarios and informal competency questions (Table 1(d)). Example data populating the ontology test the validity of the evolving models iteratively (Table 1(e)). Versioning support exists at the level of the models as a whole (Table 1(g)), but no detailed tracking of ontological definitions is supported explicitly. Typical management tasks such as importing, comparing and merging ontological definitions are not discussed (Table 1(h)), but no arguments/discussions mechanism is reported as a mean for reaching consensus (Table 1(f)). In terms of tooling support, the methodology was partially supported by tools such as transformation of diagrams into OWL and automatic generation of ontology documentation (Table 1(i)).
3 Selected Ontologies
The ontologies presented in this paper were selected from a large set of well-known, widely-used, representative and recently published ontologies. As already pointed out, this list is not by any means exhaustive: It rather presents representative examples of ontologies (domain, generic, upper, application oriented and data driven) at different stages of their life cycles (from mature to very recent and from those with high reusability to those of no-use beyond the original intended one), claiming the use of an OEM, maybe by means of appropriate tools.
Ontologies were selected from (a) ontology repositories (Table 2) and (b) articles from the latest (last 3 yr) International Semantic Web (ISWC) and Extended Semantic Web (ESWC) conferences. In addition, ontologies known very well to us, since they that have been built in our laboratory and have been added to the list (SO13, SO24): These have been published in conference articles (ESWC’17, SEMANTICS’17) and in a journal article (International Journal of Distributed Systems and Technology), representing knowledge for key research domains (semantic trajectories and IoT respectively). Also, prominent (in terms of acceptance, reuse and community engagement) ontologies that have been reused in OE tasks during the last 10 years of our OE experience have been added to the list (SO14, SO15, SO16, SO17, SO19, SO21, SO22, SO30 and SO31). Furthermore, ontologies that are prominent and are presented in articles discussing the followed OE methodologies have been added (SO8, SO11, SO14, SO15, SO16, SO17, SO18, SO19, SO20, SO21, SO22, SO23, SO25, SO26, SO27, SO28 and SO29). Finally, there is a set of ontologies that were selected due to its intersection with other sets, that is, ontologies that are prominent, well-known and widely used (SO8, SO11, SO14, SO15, SO16, SO17, SO19, SO21 and SO22). The description of the ontologies is ordered according to their publication type and year (conferences first—from recent to older events, journals and Web resources afterwards).
Table 2 Ontology repositories that were used for the ontology selection process
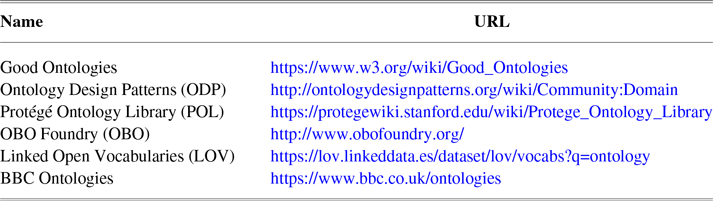
In Table 3, the status of the SOs is presented (a detailed descriptive presentation is provided in the Appendix). More specifically, the table presents: (a) the aim and scope of the ontologies, (b) their life status (alive or dead), (c) their status of evolution, that is, whether they have been evolved so far (or until death) and (d) their status of reuse, that is, how and in what extent they have been reused (or planned to be reused) in other OEM efforts. Also, they are linked to their related published resources (Web and papers in journals or conferences) as well as to the related OEM adopted for their development and/or evolution. Finally, additional information has been gathered to be considered in the analysis, such as the reuse of other ontologies/vocabularies and the use of tools to support a collaborative engineering approach.
Table 3 Selected ontologies, their relation to OEMs, and their status

To validate the details of each SO, knowledge engineers or/and authors of related papers have been contacted by e-mail (sharing the short descriptions). From the thirty contacted ontology development teams/ontologists, eighteen have been explicitly (by providing their feedback by e-mail) verified them, updating the collected information accordingly.
4 OEMs impact Analysis, Findings and Recommendations
4.1 OEMs impact to SOs
In this section, we present the impact of OEMs to the development and evolution of SOs: Specifically, we examine the impact of OEMs to the ontologies’ life status, status of evolution and status of reuse. The analysis involves thirty-two ontologies (single or groups of ontologies as in the case of SPAR and Upon Lite) presented in Section 3, following a pragmatic approach (i.e., based on practical rather than theoretical considerations, since we consider OEMs impact of ontologies in practice, during the engineering of real (pragmatic) ontologies, in real engineering settings).
Starting from our conjecture that, ideally, an OE methodology must support all involved stakeholders during the ontology life cycle, we focus on OE methodologies that support the active and decisive participation of domain experts, knowledge workers and ontology engineers, in all phases of OE, by means of appropriate tooling support. This places some bias towards collaborative ontology specification, development, exploitation and evaluation of ontologies. However, for the purposes of our analysis, we need to comparatively examine the impact of non-collaborative OEMs and other custom OEMs (OEMs that are not classified in the other two types), as these are reported by the ontology engineers in the respected ontology articles.
Therefore, following the pragmatic approach described, we report per ontology examined the following features: (a) collaborative tools’ support, (b) life status, (c) evolution status, (d) reused-in status and (e) reuses status. In addition to these, for each ontology, the analysis involves its classification in one of the three OEM types (collaborative, non-collaborative and custom). Furthermore, we examine the effect of combined features for each ontology, with the aim to identify which of the classified ontologies in a specific OEM type is alive, has been evolved and has been reused or satisfies all these properties.
Subsequently, we report on the number of alive, evolved and reused ontologies, as well as the number of ontologies that are alive, evolved and reused at the same time, out of the number of ontologies that have followed a specific OEM type. Figures 3 and 4 in the following section show how the different OEM types for the SOs satisfy the impact criteria. In Figure 3, the OEMs are comparatively depicted over the three criteria and their combination. In Figure 4, the distribution is shown for all the above criteria taking into account the OEMs that satisfy the criteria, thus providing a view starting from the impact criteria towards the OEMs. As an example of each figure, the following is extracted from the analysis:
Figure 3 example: Number of live SOs from all SOs followed a Collaborative OEM (4/10 = 0.4).
Figure 4 example: Number of live SOs following a Collaborative OEM from all the live SOs per OEM type (4/20 = 0.2).
4.1.1 Analysis
Based on the analysis conducted, five main criteria (OEM type, liveness, evolution, reusability, collaborative tool support) and one secondary (reuses other ontologies), and their distribution, have been derived to report the following results:
(a) 10 ontologies among those examined followed a collaborative OEM, 4 ontologies followed a non-collaborative OEM and 18 ontologies followed a custom OEM. More than the half of them (19) have been supported by collaborative tools, and most of them (14) have been used in the context of custom OEM (Figure 1).
(b) Regardless the OEM followed, from all the SO, 20 ontologies are still alive, 27 ontologies have been evolved, 22 ontologies have been reused and 20 ontologies have reused others in their specifications. Evolution of ontologies (either ended or ongoing) is the feature with the highest percentage (84%) (Figure 2). Out of the alive ontologies, the largest proportion are the ones followed a Non-Collaborative and Custom OEM. Almost all the SOs followed a Custom OEM are live or reused or evolved, while 76% of them exhibit all the above qualities. The latter is very important as a combined metric since it separates the SOs followed a Custom OEM from the rest by a very large margin. This is especially obvious in Figure 3 where the distribution of impact is shown per criterion for the total number of SOs under each criterion instead of the total number of SOs in the study. The SOs followed a Custom OEM are overwhelmingly impactful across the criteria.
(c) Impact of collaborative OEM: from all the SOs, 4 ontologies have followed a collaborative OEM and are alive (13%), 5 ontologies have followed a collaborative OEM and have been evolved (16%), 5 ontologies have followed a collaborative OEM and have been reused (16%) and only 2 ontologies have followed a collaborative OEM and are alive and have been evolved and have been reused at the same time (7%). Evolution of ontologies (either ended or ongoing) is the feature with the highest percentage (16%), with all features to range above 6% (Figures 2 and 3).
(d) Impact of non-collaborative OEM: from all the SOs, 3 ontologies have followed a non-collaborative OEM and are alive (9%), 4 ontologies have followed a non-collaborative OEM and have been evolved (13%), 1 ontology have followed a non-collaborative OEM and has been reused (3%) and none of the ontologies have followed a non-collaborative OEM and is alive and have been evolved and reused at the same time (0%). Evolution of ontologies (either ended or ongoing) is the feature with the highest percentage (13%), with all features to range at 0% (Figures 2 and 3).
(e) Impact of custom OEM: from all the SOs, 13 ontologies have followed a custom OEM and are alive (41%), 17 ontologies have followed a custom OEM and have been evolved (53%), 16 ontologies have followed a custom OEM and have been reused (50%) and 13 ontologies have followed a custom OEM and are alive and have been evolved and reused at the same time (41%). Evolution of ontologies (either ended or ongoing) is the feature with the highest percentage (53%), with all features to range above 41% (Figures 2 and 3).

Figure 1 Collaborate tool support per type of OEM.
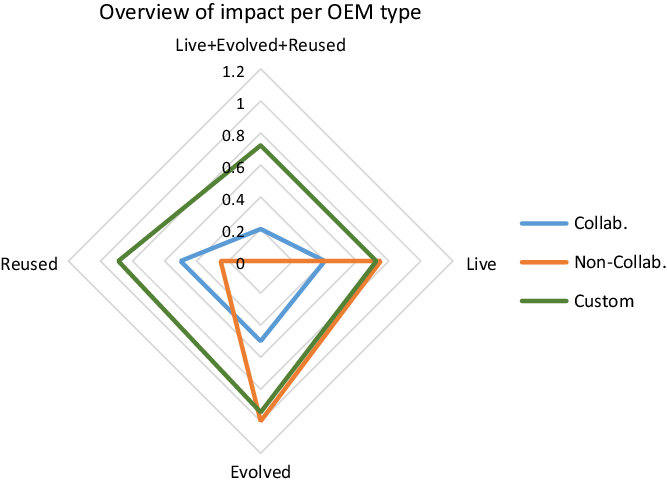
Figure 2 OEM types and impact criteria: live, evolved, reused SOs per OEM.
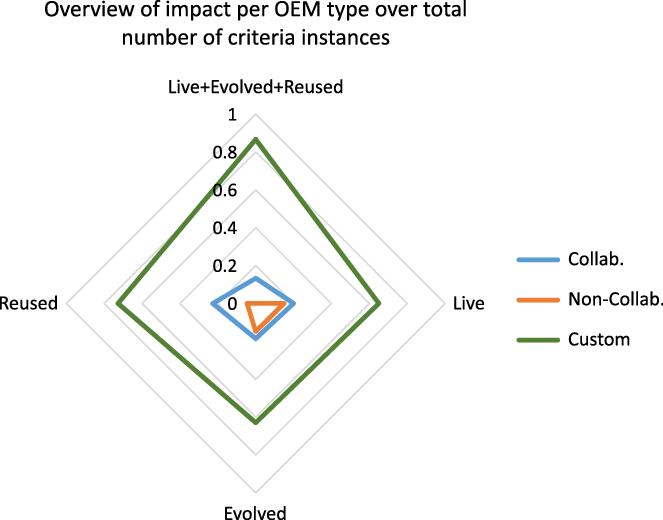
Figure 3 OEM types and impact criteria: live, evolved, reused SOs per OEM per criterion.
By a first reading of the data, it may be surprising that a custom OEM has the highest impact on the status of SOs. However, this may be justified by the fact that most (14 out of 19) of the ontologies in this type have been engineered with the support of collaborative tools, as discussed in paragraph (a) above. However, the need for support tools may have preceded the choice of the developers, who required OEMs that could be easily customized to their needs. What is evident (depicted in Figure 6) is that, across the timeline, almost all of the high impact SOs are custom engineered with tool support. That may well be the cause for their impact, that is, the fact that satisfied broader requirements of the community that made them sustainable and contributed to their longevity. Furthermore, the number of SOs that satisfy all features (alive, evolved and reused) at the same time is observed for those ontologies that followed a custom OEM. This is also justified by the fact that the several different options of tool support (e.g. Wiki, GitHub, Blog, mailing lists, OE tools) were provided to the communities of interest (stakeholders, including domain experts, ontology engineers and knowledge workers).
Another interesting dimension of the analysis concerns correlations between the features of different SOs. The most interesting result of those concerns the number of the evolved ontologies, as well as the number of the ontologies that are alive, evolved and reused at the same time, among the ontologies that have followed a specific OEM type:
Evolution: For the SOs developed using a collaborative OEM (10 ontologies), only the 50% of them have been evolved, whereas for the SOs followed a non-collaborative OEM (4 ontologies) or a custom OEM (18 ontologies), this percentage is above 94%, that is, all the SOs but one was evolved.
Liveness+Evolution+Reuse: For the SOs developed using a collaborative OEM (10 ontologies), only 20% of them are alive, have been evolved and reused at the same time, whereas for the SOs developed using a non-collaborative OEM (4 ontologies), this percentage is zero. However, for the SOs followed a custom OEM (18 ontologies), this percentage is 72% (Figure 3).
Overall, from this survey and analysis conducted, it is found that the custom OEMs have the highest impact on the engineered SOs.
Following an Open Science approach, the data are available on GitHub at https://github.com/KotisK/OEM-survey.
4.2 OEM Trends, Findings and Recommendations
From the presented analysis, the following trends have been identified (looking at the SOs of the last three years):
• The trend is to engineer ontologies following a custom community-driven OEM supported by widely available and known collaborative tools (Figures 4 and 5). This eases the participation of stakeholders who are very well acquainted with these tools.
• The trend is to make the engineered ontologies open (with appropriate licensing schemes) to enable their reuse, evolution and refinement, as much as possible: Sharing is one of the most crucial aspects when devising an ontology, as it is well known. However, this, in conjunction to the use of appropriate tools, provides opportunities for the OE tasks to support evolution and reuse.
• The trend is to use custom, tool-supported OEM supporting collaborative evaluation and validation of evolved versions, integrating feedback from several iterations of evolution and commenting on the published and exploited versions of the ontologies. Thus, agility and multiple iterations are crucial aspects of OEMs, while again, tools for managing the evolution (e.g. versioning, keeping track of changes, justifications etc.) are important.
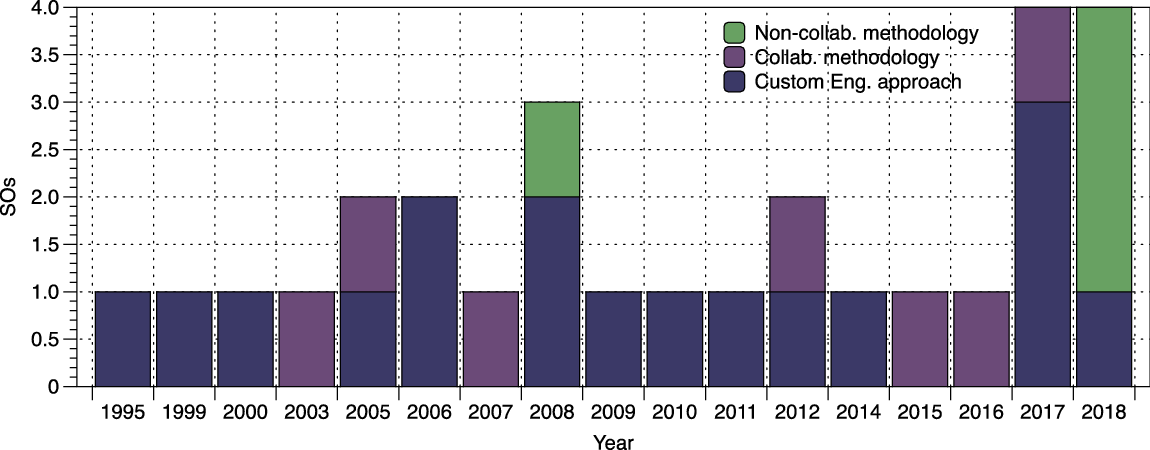
Figure 4 Time distribution of SOs and the OEM followed.
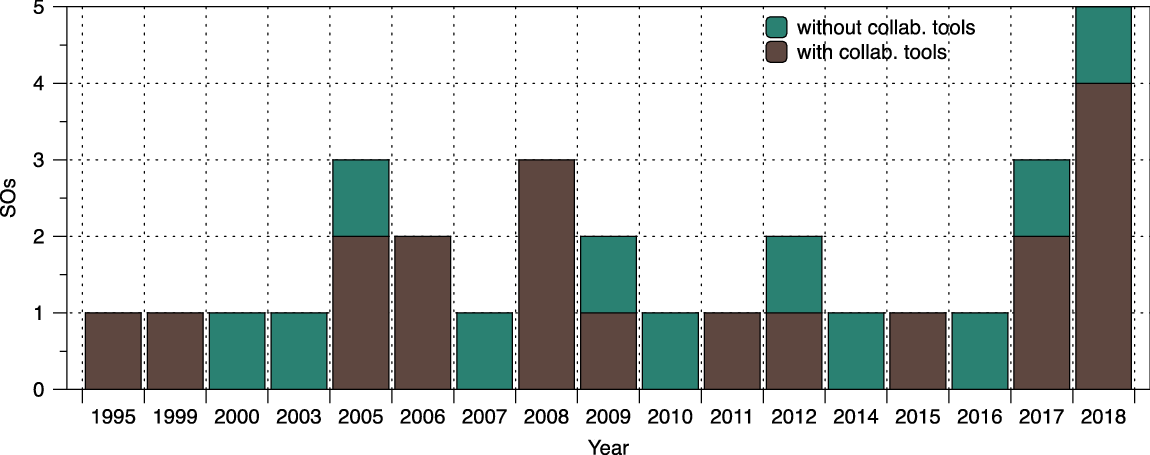
Figure 5 Time distribution of collaborative tool support development, for all SOs.
These trends are evidenced by the following main findings:
Finding 1: The stakeholder needs for collaboration and technology support seem to be the most probable cause for their decision to device and use custom-engineered OEMs and support them by the use of collaborative tools towards achieving their goals. That results in more focused or expert-driven SOs that are desirable for adoption and reuse by the community. Thus, we conjecture that the methodology by itself may not be sufficient for an ontology engineer to perform a set of collaborative tasks. Collaborative tools developed by engineers may be a justified investment to them as well as the key deciding factors for future stakeholders to reuse and evolve. This is evidenced by (a) the high percentages of alive, evolved and/or reused ontologies among those engineered with either collaborative or custom OEMs and (b) the fact that the collaborative and custom OEMs associated with ontologies reported the use of collaborative tools to support all stakeholders at a very high percentage (Figure 6).
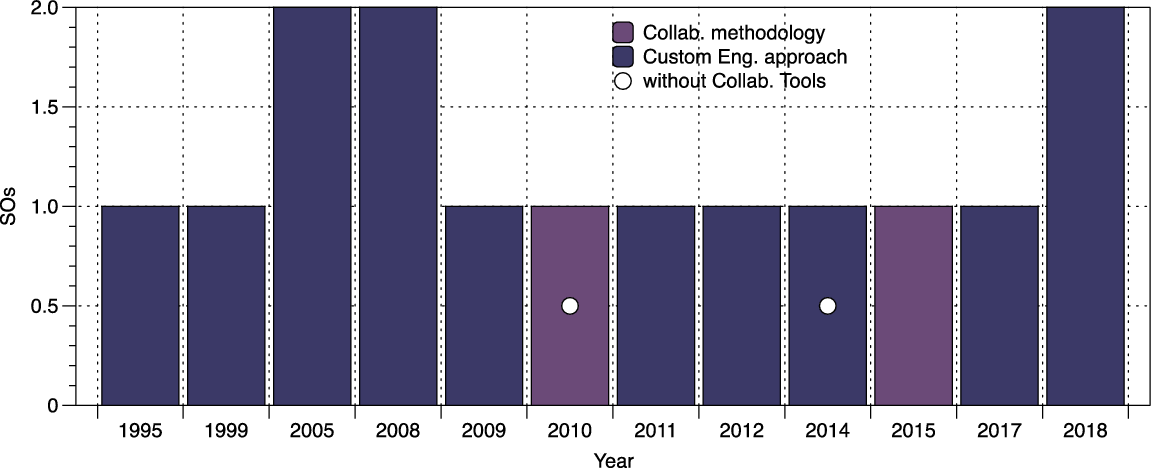
Figure 6 Time distribution of SOs’ full impact (live+ evolved+ reused) and collaborative tool support (support is default).
Finding 2: It is the aim and scope of the ontology that most often determines its reuse status. For instance, if an ontology is developed only for the purpose of enabling the representation and management of specific knowledge within a specific project or application, it is less possible for this ontology to be reused outside the project/application and its related community. This fact may also have a negative impact in the life and evolution of this ontology, without however to be able to predict a certain ‘death’: Other related projects may reuse and further evolve the ontology in their own new/related contexts. The aim and scope of the ten SOs that have not been reused are as follows: Five of them have been devised with a limited project-related aim/scope (SO2, SO13, SO26, SO28, and SO29), while six address a domain-specific aim/scope (SO2, SO5, SO10, SO20, SO26, and SO28). One ontology has an application-specific aim/scope (SO6), while only three have a generic aim/scope (SO12, SO13, SO29).
Finding 3: Although custom OEMs are not rendered to tool-supported tasks for stakeholders to follow and implement, they do demonstrate the use of freely available tools that ease their effort in different ways, from tracking issues related to the refinement of proposed versions by the community (e.g., GitHub) down to the collaborative specification of conceptualizations (e.g., Google spreadsheets, e-mails). The conducted analysis shows that there are several options available in terms of tooling for the support of collaboration in the development of shared and commonly agreed ontologies. This finding is important, given that the availability of free and open source collaborative OE tools (e.g. Web Protégé) and ontology libraries (for reuse of ontologies) nowadays is a ‘silver bullet’ for the engineering of ontologies.
Based on the trends and findings resulted from the analysis made, the following recommendations for the engineering of ontologies can be concluded:
Recommendation 1: Ontologists seem to require custom methods to achieve their immediate OE goals and requirements. Following stakeholders also seem to prefer the custom-engineered OEMs and use them in their OE tasks. With that in mind, the recommendation would be that engineers should develop or reuse an OEM keeping in mind that may be used by others and, therefore, utilize or develop methods and technologies that are widely accepted. Towards this, they need to assure the active, continuous, decisive participation of all interested parties. To do this, ontologists need to investigate the impact of community-driven requirements for the ontologies to methodological features and to choose appropriate, widely-known and used (collaborative) tools, for example, OnToology (Alobaid et al., Reference Alobaid, Garijo, Poveda-Villalón, Santana-Perez, Fernández-Izquierdo and Corcho2019) and WebProtégé (Tudorache et al., Reference Tudorache, Vendetti and Noy2009), for facilitating contributions in a selective, familiar and user-friendly way. This should be done throughout the lifetime of the ontology, to be kept alive, evolved and be reused at the maximum level. This will minimize the risk of developing a single-contributor ontology, with no community consensus, and safeguard the active participation of interested stakeholders.
Recommendation 2: As a general recommendation deduced from the works presented in the papers that describe the SOs, the OEM followed (collaborative/non-collaborative or custom-engineered) should provide early versions of the ontology to be evaluated and validated by knowledge workers, in a continuous, iterative approach. This is very important in the Big Data era, among other things, as multi-source data may necessitate fast adaptation to data sources, and validation of ontological specifications for the needs of data analysis tasks. Early feedback and early involvement of stakeholders are other important aspects of the approach to be followed.
Recommendation 3: The ontology developed should be made available, open and ‘linked’ to other ontologies associated to the interests of a wide (as much as possible) community of domain experts and knowledge workers in a respective field. This means that the ontology should reuse classes/properties and specifications from other ontologies, be shared to the community using appropriate licence schemes and with the appropriate tooling support, as needed, and should assure its reusability either within the ‘strict’ scope concerning its development, but also to a wider (as much as possible) context. The latest can be supported, if appropriate generic constructs and specifications are provided, generalizing upon the terms and specifications used within its ‘strict’ scope. Modularity plays a crucial role here (Grau et al., Reference Grau, Horrocks, Kazakov and Sattler2008; Santipantakis & Vouros, Reference Santipantakis and Vouros2014), and special emphasis should be put to designing ontologies as modular parts of other ontologies, and/or allow splitting ontologies to modular parts that can be reused independently from the rest of the ontological specifications.
Recommendation 4: To support the ontology engineer to follow the previous recommendations, the extension of a modern tool-supported OEM should be recommended in terms of (a) supporting the specification of modular ontologies, w.r.t. formal modularity properties, and supporting mapping between ontologies, at the conceptual and at the instance level and (b) facilitating the agile specification, development, evolution and maintenance of modular ontologies in shared collaborative workspaces with well-known and widely used tools by knowledge workers and domain experts.
5 Related Work
According to our recent search and records, there are few related recent surveys on OE approaches; however, these surveys do not analyze the impact of the OEMs to the status of ontologies. This section presents a chronological overview of related works and their differences/limitations over the analysis presented in this paper.
In Iqbal et al. (Reference Iqbal, Murad, Mustapha and Sharef2013), a survey on OEMs is presented. Authors compare existing methodologies and identify gaps, trends and needs that a modern effort to define a new OE methodology should consider. Based on nine criteria (C1–C9 columns of data analysis, see Excel file on GitHub) identified by the authors, the review concludes that the examined methodologies are not mature enough in terms of the level of provided details for each phase and task of the supported ontological life-cycle. However, the review does not present an analysis of the impact of those methodologies to the already engineered ontologies. More importantly, the briefly presented recommendations are limited to the generic comment that new methods and techniques should be explored for the identification of ontology concepts.
In Stadlhofer et al. (Reference Stadlhofer, Salhofer and Durlacher2013), a survey on OEMs in the domain of public administration is provided. The criteria selected for this analysis are related to general aspects of the ontology development (e.g., strategies for building ontologies) as well as project management-related aspects (e.g., recommended process model or the consideration of collaborative ontology development). The study concludes that none of the examined methodologies were fully mature to serve as an OE methodology for electronic service provisioning in public administration. However, there is not any specific description of a proposed OE methodology, and there is lack of analysis based on the correlation of OE methodologies to engineered ontologies or OE tools.
In Simperl & Luczak-Rösch (Reference Simperl and Luczak-Rösch2014), a survey on the collaborative OEMs is presented, along with a survey on OE environments. Both are compared in terms of specific criteria, and the concluded remarks of this analysis are presented. The presented conclusions are fostering the fact that ontologies should be developed and maintained in a community-driven way, with the support of integrated OE environments, providing explicit and full support to collaboration and user participation. Although this related work follows a different path to the one presented in our paper (since there is no any analysis of the product of the OE approaches that is, the ontologies), the outcome is in line to the trends and concluded recommendations presented in this paper. More importantly, the survey identifies the need to collect and examine detailed case studies and experience reports that might be helpful for defining a research roadmap to collaborative OE for the forthcoming years. Authors emphasize the shift from the development of ontologies from scratch to large-scale reuse of a large number of ontologies, also shifting their focus from a tool-assisted engineering process to a data-driven engineering approach. Finally, authors suggest that the collaborative and consensus-reaching processing, and associated tool support, should be adapted to reflect these changes. The findings, trends and recommendations presented in this paper provide supporting evidence to this survey and they also extend their analysis to non-collaborative and custom OEMs, going beyond the correlation of OEMs to OE tools, by also analyzing the actual impact to ontologies, following a pragmatic approach.
In Yadav et al. (Reference Yadav, Singh Narula, Duhan and Jain2016), a survey on all aspects of OE is briefly presented, that is, ontologies, OE methodologies and OE tools. However, the survey is limited to just a brief presentation of the topic, without going deeper into the analysis, comparison or critical discussion of their correlation.
The analysis of OE communities, among others, helps understanding how ontologies are being built, whether the community follows specific OE processes and if the proposed OE methodologies work. The latest related work presented in Kanza et al. (Reference Kanza, Stolz, Hepp and Simperl2018) contributes to this field of research by analyzing the activities and interactions of the Schema.org community. Although the analysis is deep and extensive, the actual overall impact of the methodological approach in the development and evolution of Schema.org ontology is not discussed. Also, the work is limited only on Schema.org, without comparing the analysis results with the results of other related OE efforts.
6 Discussion
In Corcho et al. (Reference Corcho, Fernández-López and Gómez-Pérez2003), authors research the ‘meeting point’ of methodologies, tools and languages for OE, based on the widely accepted requirement that a methodology must be supported by appropriate tools in all of its phases and tasks, and do so in an integrated way (e.g. IDE such as Protégé). Concludingly, authors emphasize the need for an ontology development workbench. In the more recent related work of Simperl & Luczak-Rösch (Reference Simperl and Luczak-Rösch2014), it is also emphasized that ontologies should be developed and maintained in a community-driven manner, with the help of fully fledged environments providing dedicated support for collaboration and user participation. This review paper discussed several of the methodologies, methods and techniques, and present popular development environments, which can be utilized to carry out, or facilitate specific activities within the methodologies.
Based on the already identified need of OE tools to support OEMs, but in contrast to related work that identifies such a need, the detailed description and analysis of OE tools (or integrated environments) are out of the scope of this paper since the aim is different, that is, to analyze the impact of the OEMs to the status of ontologies. Having said that, the need of OE tools is accentuated in the paper, supported by the review and analysis conducted, focusing on the impact of not only the OEMs followed (or not) for the engineering of the SOs but also on the tools used (or not). The presented analysis identifies the impact of custom-engineered approaches with collaborative tools support (Figures 3 and 4) in the findings. For instance, that the number of SOs that satisfy all features (alive, evolved, and reused) at the same time is observed for those ontologies that followed a custom OEM, with several different options of tool-supported technology (e.g. Wiki, Git, Blog, mailing lists, OE tools) provided to the communities of interest (stakeholders, including domain experts, ontology engineers and knowledge workers).
The argument that tools are not necessarily associated with methodologies but have an important impact in OE; besides, methodologies have been seen in several related works. Recently, the development of a Git-based OE environment called OnToology (Alobaid et al., Reference Alobaid, Garijo, Poveda-Villalón, Santana-Perez, Fernández-Izquierdo and Corcho2019), emphasized the need for automating OE support activities (i.e., documentation, evaluation, releasing and versioning) in a collaborative- and community-oriented manner. While compared to other collaborative ontology development environments such as WebProtégé (Tudorache et al., Reference Tudorache, Vendetti and Noy2009), Moki (Bosca et al., Reference Bosca, Casu, Dragoni and Rexha2014), Neologism (Basca et al., Reference Basca, Corlosquet, Cyganiak, Fernández and Schandl2008), Vocol (Halilaj et al., Reference Halilaj, Petersen, Grangel-González, Lange, Auer, Coskun and Lohmann2016) and Vocbench (Stellato et al., Reference Stellato, Rajbhandari, Turbati, Fiorelli, Caracciolo, Lorenzetti and Pazienza2015), the evaluation was based on specific OE support activities that are however only a subset of the collaborative OEM toolset that is required to support all tasks and phases of the ontology life cycle (as presented in our paper). For instance, the actual development/editing of the ontology (improvise, learn, specify, compare and merge conceptualizations) is not supported by OnToology, but it is supported (partially) by WebProtégé and its offline stand-alone version of Protege. Other tools such as the Neologism (not maintained anymore) support the editing and publishing, but not the evaluation and versioning of ontologies. Another Git-based tool is VoCol, providing support for project management, quality assurance, documentation, visualization and publishing of ontologies. Finally, Moki is based on MediaWiki technology, similar to the discontinued Shared-HCONE (Kotis et al., Reference Kotis, Papasalouros, Vouros, Pappas and Zoumpatianos2011), providing either a light-weight view or a full source-code view of the ontology, but it does not provide any documentation/argumentation functionalities.
7 Conclusions
There are several OE methodologies, each specifying roles, artifacts, stages, processes and tasks related to the systematic engineering of ontologies. In this article, we conjecture that custom engineered OEMs) is a major trend as is the development of collaborative tools. The requirements by the engineers are such that justify the effort for going beyond established methods. Such approaches are not only overwhelming in numbers across the last two decades but also in sustainability and reuse. This also led to continuous evolution and maintenance of ontologies, keeping them ‘live’ to shape knowledge. Starting from this conjecture, we focus on and examine the effectiveness of collaborative methodologies to keep ontologies alive, evolved and increase their potential to be reused. Special emphasis is put to providing support, via appropriate tooling, to a collaborative approach in ontology specification, development, exploitation and evaluation, engaging all stakeholders towards shaping commonly agreed knowledge. Therefore, both the real-world complexity of the requirements and the need for collaboration drive the trends in OEMs.
This paper summarizes a set of collaborative approaches and the features that they support, driven by major requirements that are widely accepted from the OE research community. In addition, but closely related to tools used, a detailed community-driven investigation of the requirements of the ontologies that need to be developed in a project, may result to additional methodological features that need to be considered and incorporated in the final methodological approach to be applied.
In this survey, we have followed a pragmatic approach and examined the impact of OEMs to the status of ‘deployed’ ontologies in terms of their life status, evolution and reuse. The analysis allows for the identification of trends in OE and recommendations for the future ontology engineer and ontologist.
An important conclusion is that, while an OEM must support the active participation of all involved stakeholders during the ontology life cycle, it is more the selection of appropriate OE tools that have a positive impact to the status of the engineered ontologies, supporting collaboration, ontology evolution and reuse. Moreover, the aim and scope of the targeted ontology should be carefully specified at the early stages of the ontology life cycle, emphasizing a generic rather than a specific focus of the conceptualizations to be developed, precluding the limited exploitation of the ontology within a restricted community.
Concluding, non-collaborative OEMs have a negative impact to the status of ontologies, that is, to their liveness, evolution and reusability. Collaborative and custom OEMs, to ensure a positive impact to the status of engineered ontologies, need to be effectively supported by an extended collaboration toolset for the development, maintenance and evolution of modularized ontologies.
Acknowledgements
The authors would like to acknowledge the significant contribution of the engineers of the SOs, as well as the authors of their related papers, who actively participated in this review by contributing their feedback on the description of their ontology used for the presented analysis. Specifically, the authors acknowledge the contribution of the following contributors and their collaborating ontologists/authors: Yannis Tzitzikas, John Breslin, Vincenzo Maltese, Antoine Zimmermann, Pascale Gaudet, Armin Haller, Milen Yankulov, Jean-Paul Calbimonte, Mcgibbney Lewis, Antonio de Nikola, Francesco Osborne, Mauro Dragoni, Evangelos Katis, Felipe Arakaki and Oscar Corcho.
In addition, we acknowledge the significant contribution of the respectful reviewers, experts in OE, for their valuable comments and suggestions.
Appendix
A Selected Ontologies
SO1. The Vocabulary for Cataloging and Linking Streams (VoCaLS) ontology (Tommasini et al., Reference Tommasini, Sedira, Balduini, Ali, Le Phuoc and Calbimonte2018) (https://w3id.org/rsp/vocals# ) aims to represent knowledge related to the publication and consumption of data streams on the Web. VoCaLS has been designed in a generic way, to be reusable and to be possible to combine with domain as well as technology-specific vocabularies. It has been collaboratively engineered following a discussions-based approach, integrating results of the work of the W3C RSP Community Group. It is an open vocabulary, available through a permanent URI, and it has been submitted to the Linked Open Vocabularies (LOV) repository. It is published under a CC-BY 4.0 license. VoCaLS is a live, evolved and reused ontology, and it reuses several other vocabularies for its definition.
SO2. The HELIS ontology (Dragoni et al., Reference Dragoni, Bailoni, Maimone and Eccher2018) (https://perkapp.fbk.eu/helis/ontology/core.html) aims to represent knowledge in the Healthy Lifestyle Support domain. HeLiS represents the core knowledge in a health-related project (PerKApp) that aims to promote healthier lifestyles for preventing chronic diseases (https://perkapp.fbk.eu/helis/). The ontology is implemented in OWL and it is currently live, evolved in version 1.10 (https://perkapp.fbk.eu/helis/ontology/helis_v1.10.owl). Apart from PerKApp project it has been reused in other projects as well (8 projects reported in authors’ feedback); however, this is expected due to that fact that it has been just recently (2018) engineered for the purposes of the specific project. Also, the ontology does not reuse other ontologies. There is an official GitHub repository (https://github.com/maurodragoni/helis); however, there is no any indication of using this as a tool to support a collaborative and iterative OEM. This is also indicated by the fact that METHONTOLOGY (Fernández-López et al., Reference Fernández-López, Gómez-Pérez and Juristo1997) OEM is reported to guide the development process of this SO, which is not a collaborative OEM. Having said that, authors report that both ontology engineers and domain experts are involved in the development process, but the information on how this is done is missing.
SO3. The Computer Science ontology (CSO) (Salatino et al., Reference Salatino, Thanapalasingam, Mannocci, Osborne and Motta2018) (http://skm.kmi.open.ac.uk/cso/ and http://oro.open.ac.uk/55484/) is a large-scale taxonomy of research areas within the domain of Computer Science. It is automatically generated from large datasets, using a custom data-driven OEM, supported by ontology learning and community-based collaborative tools. It can be easily evolved by re-running the ontology learning algorithm on new datasets and by incorporating feedback gathered from the community via a web application. The main ontology conceptualizations are based on BIBO bibliographic ontology (http://bibliographic-ontology.org/bibo/bibo.php) and on SKOS (https://www.w3.org/2004/02/skos/). It is an effort started in 2012 that has been evolved over the years and supported the development of several applications used in both academia and industry. A Web application (CSO PortalFootnote 5) is provided to enable users to explore and provide valuable feedback on the ontology. This feedback is examined by domain experts of an editorial board and is used to generate new versions of the ontology. Some portions of the ontology (e.g., Semantic Web, Software Architecture) were also comprehensively reviewed by domain experts. The CSO is available in various formats (OWL, Turtle and CSV) from https://cso.kmi.open.ac.uk/downloads.
SO4. SPAR ontologies (Peroni & Shotton, Reference Peroni and Shotton2018) (http://www.sparontologies.net) are a set of modular OWL ontologies that represent knowledge about the publishing domain (document description, bibliographic resource identifiers, etc.). The development process of these ontologies has been driven by specific development principles that were later shaped as the SAMOD (Peroni, Reference Peroni2017) OEM (enabling adoption, reuse, modularization, interoperability, minimal logical constraints, tool-support). The ontologies are still live and have been reused by several projects and ontologies (since 2015) that they were published in the Web (http://www.sparontologies.net/uptake). A community-based evolution approach is followed by using GitHub repository (https://sparontologies.github.io/#github-guidelines ) and by publishing specific participation rules for authors to follow (https://sparontologies.github.io/#participation-guidelines ).
SO5. The Audio Commons ontology (George Fazekas, n.d., Reference George Fazekas2018) (http://www.audiocommons.org/ac-ontology/aco.html) represents knowledge about audio content, both musical and non-musical with the aim to facilitate interoperability between repositories and clients. The ontology is live (currently in version 1.2.2) and online for others to reuse under the licence CC-BY 0. Its ontology design follows the METHONTOLOGY (Fernández-López et al., Reference Fernández-López, Gómez-Pérez and Juristo1997) OEM. However, as reported in a recent publication (George Fazekas, Reference George Fazekas2018), the development is performed in an online public GitHub repository (https://github.com/AudioCommons/ac-ontology) maintained by the Audio Commons consortium. The approach is using GitHub’s issue tracking system as a communication channel to support maintenance and evolution of the ontology (at the moment—November 2018—only one contributor has been recorded). The ontology reuses several existing ontologies (to increase interoperability), but there is no any information available related to its reuse in other ontologies or projects (however, this is expected due to that fact that it has been just recently engineered).
SO6. The United Nations System Document ontology (UNDO) (Peroni et al., Reference Peroni2017) (https://unsceb-hlcm.github.io/onto-undo/index.html) is an OWL ontology that formally describes real-world objects and conceptualizations that appear in parliamentary, normative and judicial documents of the UN. The goal is to provide a mechanism for sharing data about any of these documents and its content in RDF format. Furthermore, the objective is to support various UN agencies to reuse and extend the ontology, meeting own domain-specific requirements. The ontology is live (the latest and authoritative versions of UNDO is available at https://w3id.org/un/ontology/undo) and online for others to reuse under the licence CC-BY 0. All source files can be found in the GitHub repository at https://w3id.org/un/repository/undo (only one contributor has been recorded and the latest version is dated June 2017). UNDO has been developed from scratch using SAMOD (Peroni, Reference Peroni2017) OEM (after three iterations) of the process, as documented in the GitHub repository. The ontology reuses a number of existing ontologies (to increase interoperability); however, as reported in the related ISWC’17 paper (Peroni et al., 2017), there is no explicit information of its broad adoption worldwide so far, since it is has been recently released (Reference Peroni2017). Having said that, United Nations and collaborating agencies will use it for describing their documents and their interrelations in RDF.
SO7. The MedRed ontology (Calbimonte et al., Reference Calbimonte, Dubosson, Hilfiker, Cotting and Schumacher2017) (http://w3id.org/medred/medred# ) has been developed in the context of the MedRed acquisition project (MedRed: http://w3id.org/medred/project), with the goal of representing clinical data acquisition metadata. The ontology is live (the latest version of MedRed ontology is available at https://jpcik.github.io/medred/onto/medred/index-en.html) and online for others to reuse under the licence CC-BY 4.0. Source files in OWL can be found in the GitHub repository at https://github.com/jpcik/medred (two contributors have been recorded and the latest release of the ontology, v1.0.1, is dated June 27, 2017) as well as in Linked Open Vocabularies (LOV) repository and Zenodo (https://doi.org/10.5281/zenodo.819875). The ontology has been developed using a custom OEM with the support of GitHub. Also, authors report the use of OnToology (Alobaid et al., Reference Alobaid, Garijo, Poveda-Villalón, Santana-Perez, Fernández-Izquierdo and Corcho2019) and OOPS! Web-based tool (Poveda-Villalón et al., Reference Poveda-Villalón, Gómez-Pérez and Suárez-Figueroa2014), which were used to generate documentation, to perform basic validation, and to aid in the entire process by automating part of the collaborative ontology development process. It reuses several existing ontologies (towards increasing interoperability), and at the same time, it has been used in a number of related pilot projects by the developers.
SO8. YAGO3 ontology (Rebele et al., Reference Rebele, Suchanek, Hoffart, Biega, Kuzey and Weikum2016) is a multilingual extension of YAGO (https://www.mpi-inf.mpg.de/departments/databases-and-information-systems/research/yago-naga/yago/), an open source populated ontology developed to represent knowledge that is derived from Wikipedia, WordNet and GeoNames. It represents knowledge of millions of entities such as persons, organizations, and cities and contains millions of facts about these entities, and more than 350.000 classes. The ontology is live (the latest version of YAGO, version 3.1, is available in .ttl format at http://resources.mpi-inf.mpg.de/yago-naga/yago3.1/yago3.1_entire_ttl.7z) and online for others to reuse under the licence GNU General Public licence, version 3 or later. Source files in .ttl can be found in the GitHub repository at https://github.com/yago-naga/yago3 (eight contributors have been recorded and the latest release of the ontology, v3.1, is dated June 2017). The ontology has been developed using a custom OEM, extracting knowledge (facts) from Wikipedia and constructing the taxonomy using mainly the categories of Wikipedia. Also, the development process is supported by GitHub. It reuses several existing ontologies (towards increasing interoperability), and at the same time, it has been used in a number of related pilot projects by the developers (since first release of YAGO in 2008).
SO9. The Proton ontology (Damova et al., Reference Damova, Kiryakov, Simov and Petrov2010) (https://ontotext.com/products/proton/) is a lightweight upper-level ontology (encoded in OWL light) that has been serving as a modeling basis for tasks such as: (a) ontology generation (new ontologies constructed by extending PROTON), (b) automatic entity recognition from text and (c) semantic annotation. It contains about 500 classes and 150 properties. The development of the ontology has followed a collaborative community-based process organized in accordance with the DILIGENT (Pinto et al., Reference Pinto, Tempich, Staab, Staab and Studer2009; Vrandečić et al., Reference Vrandečić, Pinto, Tempich and Sure2005) OEM. The ontology is not live since 2010 (the latest version of Proton, version 3.0, is available at https://www.ontotext.com/documents/proton/proton-doc.htm) and online for others to reuse under the licence CC-BY 3.0. Source files in can be found at https://www.ontotext.com/proton/protontop.html (for the Top module) and https://www.ontotext.com/proton/protonext.html (for the Extension module). There is no information regarding reuse of other ontologies in Proton (to increase interoperability), but there is available information that it has been used in a number of projects around the world, for example, the semantic search and annotation of The National Archives of UK.
SO10. The Curriculum & Syllabus ontology (Katis et al., Reference Katis, Kondylakis, Agathangelos and Vassilakis2018) (http://xworks.gr/ontologies/ccso.owl) aims to represent knowledge related to an educational and academic setting, modeling the core concepts of curriculum in higher education. Although, as reported in the related paper, the developed ontology aims to be highly reusable, the engineering process follows a combination of two old but popular non-collaborative and iterative OEMs, namely, the one proposed by Uschold & King (Reference Uschold and King1995) and the one proposed by Noy & McGuinness (Reference Noy and McGuinness2001). The ontology is live since 2018 (the latest version available in OWL format at http://xworks.gr/ontologies/ccso.owl is version 0.7) and online for others to reuse but with no licence indication. The ontology can be applicable and freely reused by other schools and universities. It is available online at https://w3id.org/ccso/ccso while HTML documentation is also provided. Due to its little age (March 2018), there is no information on its reuse in other applications or ontologies. However, it has the potential to be reused and shareable among institutions. There is no any support of tools for collaboratively and iteratively engineering future evolved versions of it.
SO11. Schema.org ontology (Guha et al., Reference Guha, Brickley and Macbeth2016) (https://schema.org), as presented and discussed in the latest paper of ESWC’18 event (Kanza et al., Reference Kanza, Stolz, Hepp and Simperl2018), is considered one of the most successful collaborative community-based Semantic Web projects of all times, engineering a schema for the representation of commonly used types (classes and datatype properties) and relations (object properties) with the aim to structure data on the Internet, on Web pages, in e-mail messages, and even more. An OWL version is available at https://schema.org/docs/schemaorg.owl under a CC-BY-SA 3.0 licence. The engineering of the ontology follows a custom OEM, supported by a highly active community, within a DILIGENT-like (Pinto et al., Reference Pinto, Tempich, Staab, Staab and Studer2009; Vrandečić et al., Reference Vrandečić, Pinto, Tempich and Sure2005) tool-supported collaborative workflow, supported by tools such as e-mail service and GitHub repository (https://github.com/schemaorg/schemaorg). It has been heavily reused by other ontologies and Semantic Web applications and is still a live project (latest evolution is version 3.4 dated June 2018). GitHub records 61 contributors and 6 releases so far (since 2014).
SO12. NdFluents is a generic ontology (Giménez-García et al., Reference Giménez-García, Zimmermann and Maret2017) (https://www.emse.fr/~zimmermann/ndfluents.html) that aims to represent metadata about RDF statements (annotated statements), preserving inference capabilities, and also addressing the problem of representing ‘fluents’, that is, relations that hold within a certain time interval and not in other. It is based on the 4dFluents ontology (Welty & Fikes, Reference Welty and Fikes2006), by generalizing it to annotate statements with any number of context dimensions (not only 4). The ontology, as published in http://www.emse.fr/~zimmermann/ndfluents.html, is a generalization from temporal parts to contextual parts of conceptualizations. As authors reported, its development did not go through a specific methodology since it is strictly following the design of 4dFluents. Moreover, the goal with this ontology was not really to describe accurately a field of knowledge, nor to provide the best engineered vocabulary. Authors were more interested in the way the approach of Welty and Fikes can be used to model contextual knowledge and how it behaves with respect to reasoning. The abstract model is fixed, and there is no interest by authors in modifying the concrete artifacts (the OWL file and the documentation). The use of this ontology in a practical project may induce some evolution, but the scope is so narrow that it is likely to stay pretty much the same forever.
SO13. The datAcron ontology (Santipantakis et al., Reference Santipantakis, Vouros, Doulkeridis, Vlachou, Andrienko, Andrienko and Martinez2017) (http://ai-group.ds.unipi.gr/datacron_ontology/) is a generic ontology for the representation of semantic trajectories, events and contextual information. The main contribution of the ontology is: (a) the representation of semantic trajectories at varying and interlinked levels of spatiotemporal analysis and (b) the support of data transformations that facilitate analytics. It has been engineered following the HCOME (Kotis & Vouros, Reference Kotis and Vouros2006) collaborative and iterative OEM, for a period of 12 months, using Protégé, Google Groups and e-mail support tools. The ontology has been engineered for the purposes of the datAcron project in a data-driven way: In doing so, tools for populating it with data from big data heterogeneous data sources have been devised, also supporting validation of constructed knowledge graphs. It is live as it is used to convert data from heterogeneous sources and link them, supporting data transformations for advanced analysis tasks. It is expected that it will be reused by other related projects for the representation of semantic trajectories. dat-Acron is reusing generic and upper ontologies and its engineering process has been supported by collaborative tools. The latest version, version 2.0 (as of April 2017), is available online at http://ai-group.ds.unipi.gr/datacron_ontology/ under CC-BY 3.0 licence.
SO14. The Dublin Core (Arakaki et al., Reference Arakaki, Alves and Santos2018) (http://dublincore.org/, http://dublincore.org/documents/dcmi-terms/) is a vocabulary for describing generic metadata with the aim to provide a consolidated standard with several possibilities for its use, that is, the representation, identification, location and access to Web resources. It is a live and continuously evolving project with highly active and large community for 20 years. Its latest official version has been issued at http://dublincore.org/documents/dcmi-terms/ and is dated in June 2012. It has been heavily and widely reused in other ontologies/vocabularies as an annotation approach of entities using standardized metadata, under a CC-BY 3.0 licence. The engineering approach follows a custom OEM, supported by the Dublin Core Metadata Initiative (DCMI) Usage Board and the DCMI Namespace Policy, and tools such as e-mail lists and wikis. DCMI communities bring together people working on Dublin Core metadata, the use of Dublin Core specifications, and in metadata best practices. Dublin Core is a W3C ‘good ontology’.
SO15. The FOAF ontology (Dan Brickley, Reference Dan Brickley2014; Narula et al., Reference Narula, Yadav, Duhan and Jain2018) (http://www.foaf-project.org/) is a well-known and widely used vocabulary that aims to define people-related terms that can be used in structuring and linking data in the social Web (along with SIOC ontology). The project is not live (latest activity reported in 2015); however, it has been evolved for almost fifteen years (since 2000). The latest version (0.99) is reported at http://xmlns.com/foaf/spec/, dated January 2014. It has been widely reused in other ontologies/vocabularies as metadata for human entities, under a CC-BY licence. The engineering approach followed a custom OEM, with community-based discussions via mailing lists and forums, and W3C-related working groups. FOAF is a W3C ‘good ontology’.
SO16. The SIOC ontology (Berrueta et al., Reference Berrueta2018; Bojars et al., Reference Bojars, Passant, Breslin and Decker2009) (http://sioc-project.org/) is a well-known and widely-used ontology that aims to define terminology related to online social communities structure and content, that can be used in structuring and linking the social Web (along with FOAF ontology). The project is live (latest activity reported in 2018), and it has been evolved for many years (since 2005). The latest version (1.36) is reported at http://rdfs.org/sioc/spec/, dated Feb 2018. It reuses several vocabularies, and it has been widely reused in other ontologies/vocabularies as metadata for human entities, under a CC-BY 1.0 licence. The engineering approach follows a custom OEM, based on a collaborative effort amongst members of the Unit for Social Semantics at the Data Science Institute, NUI Galway and Semantic Web developers on the SIOC developers' mailing list (SIOC-Dev), with IRC channels and Wiki support. GitHub (at https://github.com/rdfs-org/rdfs.org/tree/master/sioc) reports one contributor and an update 9 months ago. SIOC is a W3C ‘good ontology’.
SO17. The Good Relations ontology (Hepp, Reference Hepp2008, Reference Hepp2011) (http://www.heppnetz.de/projects/goodrelations/) aims to represent knowledge related to e-commerce (types of products and services) for publishing details of companies’ products and services to be exploited by search engines, mobile applications and browser extensions. The project is not live (latest revision 1.0 was released in Nov. 2011 at http://www.heppnetz.de/ontologies/goodrelations/v1.html) under a CC-BY 3.0 licence, and it has been evolved since 2008. It has been (re)used in several e-commerce projects and related vocabularies but external ontologies/vocabularies have not been reused in its specification. The engineering approach follows a custom OEM that mixes the Uschold & King OEM (Uschold & King, Reference Uschold and King1995) with collaborative work based on discussions via mailing lists, IRC channels and Wiki. Good Relations is a W3C ‘good ontology’.
SO18. The Music ontology (Raimond et al., Reference Raimond, Abdallah, Sandler and Giasson2007; Raimond & Sandler, Reference Raimond and Sandler2012; M. O. Group, Reference Group2013) (http://musicontology.com/) aims to provide a commonly agreed model to represent the music domain knowledge, for publishing structured data on the Web. The project is not live (latest revision 2.1.5 was released July 2013 at http://musicontology.com/specification/) under a CC-BY 1.0 licence, and it has been evolved since 2006. It has been (re)used in several music-related projects and related vocabularies and external ontologies/vocabularies have been reused in its specification. The engineering approach follows a custom OEM with community-based discussions on Wiki (peer reviewed), GitHub and mailing lists support. GitHub (https://github.com/motools/musicontology.com) reports one contributor 5 years ago. Music ontology is a W3C ‘good ontology’.
SO19. The MarineTLO ontology (Tzitzikas et al., Reference Tzitzikas, Allocca, Bekiari, Marketakis, Fafalios, Doerr and Candela2016; Bekiari et al., Reference Bekiari, Doerr, Tzitzikas, Allocca, Barde, Minadakis and Marketakis2017) (http://www.ics.forth.gr/isl/MarineTLO/) is a top-level ontology for the representation of the marine domain. The aim is to assist research about species and biodiversity. The project is live (latest revision 5.0 was released in January 2017 at https://www.ics.forth.gr/isl/ontology/content-content-MTLO/html/index.html) under a CC-BY 3.0 licence, and it has been evolved since 2014. It has been reused in several related projects and ontologies/vocabularies, and other ontologies/vocabularies have been reused for its specification. The engineering approach follows a custom iterative and incremental OEM, comprising the following steps: collection of Comments and suggestions are collected by email. MarineTLO is a W3C ‘good ontology’.
SO20. BBC ontologies (Raimond et al., Reference Raimond, Scott, Oliver, Sinclair and Smethurst2010) (https://www.bbc.co.uk/ontologies) aim to describe BBC concepts for representing the knowledge related to BBC products and any other corporate content published in the Web as linked data. The majority of those contain more products dedicated in thematic areas, for example Education, Sport, News. It is not clear if the project is live anymore (latest revisions of each BBC ontology can be accessed individually at https://www.bbc.co.uk/ontologies, and the latest one is reported in 2015) under a CC-BY 3.0 or 4.0 licence, and they have been evolved since 2009. Moreover, there is no detailed information regarding their reuse outside BBC organization, and there is no information regarding the reuse of other ontologies for their specification. More important, there is no any report on the specific engineering methodology followed for each ontology.
SO21. The GeoNames ontology (Wick et al., Reference Wick, Vatant and Christophe2015) (http://www.geonames.org/ontology/documentation.html) aims to represent geospatial information needed for describing the geography (names and other information of toponyms) of resources in the Web. The ontology is not live (latest revision 3.1, dated Nov. 2012) can be accessed at http://www.geonames.org/ontology/ontology_v3.1.rdf under a CC-BY 4.0 licence, and it has been evolved since 2006. It has been reused in several related projects and ontologies/vocabularies, and other ontologies/vocabularies have been reused for its specification. The engineering approach follows a custom OEM with a community-based discussion via mailing lists, a forum and a blog.
SO22. The DBpedia ontology (Mendes et al., Reference Mendes, Jakob and Bizer2012) (https://wiki.dbpedia.org/services-resources/ontology and http://live.dbpedia.org/ontology) aims to represent knowledge from the Wikipedia (many specific domains and general world knowledge). It has been created manually based on the most commonly used Wikipedia info-boxes. It currently covers 685 classes, 2.795 different properties and 4.233.000 instances. It is a live ontology (latest release is version 3.7) accessible online under CC-BY-SA 3.0 and GNU Free Documentation licence, and it has been evolved since 2009. Its schema can be separately downloaded as an OWL file (http://downloads.dbpedia.org/2014/dbpedia_2014.owl.bz2) and can also be queried via a SPARQL endpoint (http://dbpedia.org/sparql). It has been reused in several related projects and ontologies/vocabularies, and other ontologies/vocabularies have been reused for its specification. The engineering approach follows a custom OEM that collaboratively generates wiki-based community mappings to Wikipedia info-boxes and supports the wiki-based editing of the ontology. The approach is supported by a DBpedia Mappings Wiki (http://mappings.dbpedia.org) and DBpedia Extraction Framework (http://mappings.dbpedia.org/index.php/Use_the_DBpedia_Extraction_Framework). A GitHub repository is used as an issue tracker for modification requests in the DBpedia Ontology (reports 5 contributors for over a year).
SO23. The Gene ontology (GO) (Carbon et al., Reference Carbon, Dietze, Lewis, Mungall, Munoz-Torres, Basu and Westerfield2017; Dessimoz & Škunca, Reference Dessimoz and Škunca2017; Munoz-Torres & Carbon, Reference Munoz-Torres and Carbon2017; Liu et al., Reference Liu, Liu and Rajapakse2018) (http://geneontology.org) aims to represent knowledge related to the functions of genes and their products. The GO conceptualizes the logical structure of the biological functions and their relations, implemented as directed acyclic graph. The ontology is live since 1999. The latest version is reported in 2018 under a CC-BY 4.0 licence, downloadable from http://geneontology.org/page/download-ontology. It has been reused in several related projects and ontologies/vocabularies, and other ontologies/vocabularies have been reused for its specification. The engineering approach follows a custom community-driven OEM, supported by a GitHub tracker (https://github.com/geneontology/go-ontology). GitHub reports 21 contributors so far. The revisions of the ontology are managed by senior ontology editors, who are experts in biology and computational knowledge representation. Ontology updates are performed in a collaborative manner between the GO Consortium (GOC) ontology team and scientists who request the updates. Requests are submitted by scientists working on GO annotations and from domain experts in particular areas of biology.
SO24. The IoT ontology (Kotis & Katasonov, Reference Kotis and Katasonov2013) (http://ai-group.ds.unipi.gr/kotis/ontologies/IoT-ontology) aims to represent knowledge related to the Internet of Things (IoT) domain, towards supporting the automated deployment of IoT applications in heterogeneous smart environments. It serves as a semantic registry to associations of sensing, actuating and identity, as well as to applications that utilize the services provided by these associations. The ontology is not live, and it has been evolved for approximately one year. The latest online version (version 2.1) can be accessed at http://kotis.epizy.com/Ontologies/IoT/IoT-ontology-v2.1.owl under a CC-BY 3.0 licence. It has been reused in IoT-related projects and ontologies/vocabularies (e.g. IoT-trust), and other ontologies/vocabularies have been reused for its specification (e.g. SSN). It has been engineered following the HCOME (Kotis & Vouros, Reference Kotis and Vouros2006) collaborative and iterative OEM for a period of 12 months, using Protégé and WebProtégé, Google Groups and e-mail support tools.
SO25. The Ontology of Professional Judicial Knowledge (OPJK) (Casanovas et al., Reference Casanovas, Casellas, Tempich, Vrandečić and Benjamins2007a) was developed in the Semantically Enabled Knowledge Technologies (SEKT) EU project (IST-2003-506826) with the aim to represent the legal domain and be used in a web-based intelligent FAQ for judicial use prototype system (namely, Iuriservice) to support professional judges. The ontology was extracted manually from a repository of professional judicial knowledge. Due to the distributiveness of the development environment, controlled discussion and traceability of the ontology engineering process was needed. Thus, the ontology was engineered following the DILIGENT (Vrandečić et al., Reference Vrandečić, Pinto, Tempich and Sure2005; Pinto et al., Reference Pinto, Tempich, Staab, Staab and Studer2009) methodology. The ontology is not live anymore, and there are no any available online resources to be accesses for download and reuse. In 2005, the ontology has been integrated to PROTON ontology (Damova et al., Reference Damova, Kiryakov, Simov and Petrov2010) (see SO8 row in the Selected Ontologies - Table 3).
SO26. The Vocational Competency Ontology (VCO) (De Leenheer et al., Reference De Leenheer, Christiaens and Meersman2010) aims to be used for the reconciliation of contextualised competency models, and exchange competency-annotated employment and training data. The ontology has been used to evaluate the collaborative and iterative methodology of DOGMA-MESS (de Moor et al., Reference de Moor, De Leenheer, Meersman, Schärfe, Hitzler and Øhrstrøm2006; Leenheer & Debruyne, Reference Leenheer and Debruyne2008) in the context of the European project Codrive. The ontology is not live and there are no any available online resources to be accessed for download and reuse. There is no information related to online versions of the ontology (source files, dates, etc.).
SO27. The Space ontology (Giunchiglia et al., Reference Giunchiglia, Dutta, Maltese and Farazi2012), also known as GeoWordNet (Giunchiglia et al., Reference Giunchiglia, Maltese, Farazi and Dutta2010), aims to formally represent places of the world originally taken from GeoNames. This large-scale geospatial ontology has been used to evaluate an ontology engineering methodology based on the faceted approach, as originally developed in library science (Giunchiglia et al., Reference Giunchiglia, Dutta, Maltese and Farazi2012) in the context of the EC FP7 Living-Knowledge. In fact, following the guiding principles of the methodology, classes of GeoNames were formalized into concepts, integrated with WordNet (as well as MultiWordnet for the Italian language) and arranged hierarchically by means of semantic relations. GeoNames places populate the ontology. GeoWordNet has been used to build YAGO2 (Hoffart et al., Reference Hoffart, Suchanek, Berberich and Weikum2013), it is still used to support the semantic geo-catalogue of the Province of Trento in Italy (Farazi et al., Reference Farazi, Maltese, Dutta, Ivanyukovich and Rizzi2013), and as background knowledge for the semantic matching tool S-Match (Giunchiglia et al., Reference Giunchiglia, Shvaiko and Yatskevich2004). The ontology was created in 2010, last update was in 2012, and it is currently available in CSV and RDF format under licence CC-BY 3.0 at the following link: https://sourceforge.net/projects/s-match/files/datasets/.
SO28. Upon Lite ontologies (De Nicola & Missikoff, Reference De Nicola and Missikoff2016) comprise a set of more than 20 ontologies (according to authors) representing different domains of knowledge. The process has started in 2001 and involved a variable number of domain experts up to 100. The majority of ontology teams were formed by non-experts in ontology engineering. This methodology has been mainly adopted by two European Union projects: (a) Collaboration and Interoperability for Networked Enterprises in an Enlarged European Union (COIN) and (b) Business Innovation in Virtual Enterprise Environments (BIVEE). In the former, a trial ontology was developed for the Andalusia Aeronautic cluster for a furniture ecosystem for the Technology Institute on Furniture, Wood, Packaging and related industries in Spain, and for the robotics sector in Italy. In the national Italian project E-Learning Semantico per l’Educazione continua nella medicina (ELSE), a trial ontology for lifelong education of medical doctors in the domain of osteoporosis was developed. Authors/ontologists have provided detailed information regarding the status of these and other Upon Lite-related ontologies. We have included this reference based on the important findings that the authors of the recent (2016) related journal paper (De Nicola & Missikoff, Reference De Nicola and Missikoff2016) have presented, based on their comparison of the Upon Lite methodology to others.
SO29. The Human Resources Management reference ontology (‘About Human Resources Management Ontology | Joinup,’ n.d.; Gómez-Pérez et al., Reference Gómez-Pérez, Ramírez and Villazón-Terrazas2007), composed of 13 modular ontologies, aims to represent employment knowledge and, more specifically, knowledge related to the details of job postings and the CV of job seekers. Its development has been supported by the Single European Employment Market-Place (SEEMP) project, an EC STREP project. The ontology was developed following the NeOn methodology (Pérez et al., Reference Pérez, Baonza and Villazón2008) for Building Ontology Networks and supported by two ontology engineering tools: Web Service Modelling Toolkit (WSMT) https://sourceforge.net/projects/wsmt/) and WebODE (http://www.oeg-upm.net/). The ontology is available in the WSML standard (http://www.wsmo.org/wsml/) at http://www.oeg-upm.net/, under a CC-BY 3.0 licence. The project is not live any more. Last activity is reported in 2012 (for the creation of RDFS versions of the ontologies). Other existing human resources management standards have been reused.
SO30. SWEET ontologies (Raskin, Reference Raskin2003; Raskin & Pan, Reference Raskin and Pan2005; DiGiuseppe et al., Reference DiGiuseppe, Pouchard and Noy2014) (https://sweet.jpl.nasa.gov/) aim to represent knowledge related to the Earth and Environmental Sciences (EES) domain. Semantic Web for Earth and Environmental Terminology (SWEET) ontologies have become the standard ontologies to represent the EES domain formally. It is a live project, currently at version 3.2.0 (as of Aug. 2018), available online in different formats at https://bioportal.bioontology.org/ontologies/SWEET and https://github.com/ESIPFed/sweet, under an Apache Licence v2.0. GitHub reports 6 contributors and 8 releases since 2017. Older revisions (from year 2011 and prior to version 3.1.0) are archived. The latest methodological approach follows a custom engineering of ontologies, supported by online collaborative tools such as the BioPortal and GitHub. The original version was developed by Robert Raskin at JPL (R. G. Raskin & Pan, Reference Raskin and Pan2005) but now stewardship has been taken over by the Earth Science Information Partners Federation (ESIPFed). It has been heavily evolved and reused the last fifteen years (at least).
SO31. The Semantic Sensor Network (SSN) and Sensor, Observation, Sample and Actuator (SOSA) ontologies (Compton et al., Reference Compton, Barnaghi, Bermudez, García-Castro, Corcho, Cox and Taylor2012; Haller et al., Reference Haller, Janowicz, Cox, Lefrançois, Taylor, Le Phuoc and Stadler2018; Janowicz et al., Reference Janowicz, Haller, Cox, Phuoc, Lefrançois, Janowicz and Lefranc2018) (https://www.w3.org/TR/vocab-ssn/) aim to represent knowledge related to sensors and their observations, the involved procedures, features of interest, samples used to do so and the observed properties, as well as to actuators. The SSN ontology is available at http://www.w3.org/ns/ssn/ and the SOSA ontology is available at http://www.w3.org/ns/sosa/ under OCG and W3C copyrights and patent policy. GitHub (https://github.com/w3c/sdw) and mailing lists are available for issues and feedback related to the ontologies. The ontologies are live and evolved, highly reused for the past 10 years (since the initial SSN version published by the W3C Semantic Sensor Network Incubator Group and work on Sensor Web Enablement by the OGC), reusing other vocabularies/ontologies as well. It follows a custom W3C and OGC community-driven collaborative engineering approach, with GitHub and mailing lists support.
SO32. A catalogue of ontologies for the publication of homogeneous open data across cities in Spain is being developed as a community effort, led by the Ontology Engineering Group/UPM, in the context of two ongoing initiatives (the Spanish thematic network on Open Data and Smart Cities and the Ciudades Abiertas project). Approximately 100 datasets have been identified so far (2019) as potential candidate datasets to be described formally with the use of ontologies, so as to promote sharing across cities and facilitating data reuse by third parties as well as by cities themselves, and more than 20 ontologies have been made available (Espinoza-Arias et al., Reference Espinoza-Arias, Poveda-Villalón, García-Castro and Corcho2018). Most of them were developed using the Linked Open Terms methodology (lot.linkeddata.es), a tool-supported industrial version of a collaborative OE approach developed by OEG/UPM (O. E. Group, Reference Group2019). An example of such an ontology is the one to describe the census of business units in a city (Corcho & Espinoza-Arias, n.d.), available at http://vocab.ciudadesabiertas.es/def/comercio/tejido-comercial. A catalogue where all these ontologies will be made available can be reached at http://vocab.ciudadesabiertas.es/ and at GitHub: https://github.com/opencitydata and https://github.com/CiudadesAbiertas/vocab-comercio-censo-locales. The ontology examined in this paper (as well as the rest of this initiative) reuse several vocabularies (mainly related to geospatial data representation and the Schema.org) and are reused in the context of related projects. Furthermore, they are alive and continuously evolved, under CC-BY 4.0 licence.