Diet diversity scores (DDS) have been shown to offer a relatively simple and valid assessment of diet quality among various population groups( Reference Arimond and Ruel 1 ). Dietary quality indicators such as DDS can complement or even replace the calculation of nutrient intakes and probability of nutrient adequacy when evaluating food intake data. Despite their potential, the question of whether or not to apply minimum portion size for foods or food groups contributing to the DDS has not been well explored in low-income nations. The use of minimum portion sizes in defining dietary scores is a more common practice in developed nations. For instance, the food group and diet diversity components of the Healthy Eating Index (HEI) and the Diet Quality Index-Revised (DQI-R) are based on a minimum number of servings consumed by an individual and the amount of food that constitutes a single serving varies across food groups. The number of ‘food group points’ that an individual can earn in the HEI varies from 0 to 10 depending on how well his/her intake meets the recommended food group servings; while in the DQI-R, one has to consume at least one-half serving of a specific food group to be counted as a food group ‘consumer’( Reference Kennedy, Ohls and Carlson 2 , Reference Haines, Siega-Riz and Popkin 3 ). While this approach partially adjusts for differing nutrient contributions across food groups, it does not specifically consider nutrient density. Furthermore, the definition of a serving may not be appropriate in countries where serving sizes are not well defined. Despite these limitations, these dietary diversity and variety measures have been shown to have strong associations with dietary quality and individuals’ nutritional status( Reference Guthrie and Scheer 4 – Reference Murphy, Foote and Wilkens 6 ). Another index, the Healthy Diet Indicator (HDI), departs from the use of servings and assigns a specific range of intake for each food group. An individual is defined as a food group ‘consumer’ if his/her intake is within the recommended intake range. However, it does not account for all food groups( Reference Huijbregts, Feskens and Räsänen 7 ). Unlike the developed nations, only a few studies in less-developed nations have imposed a minimum food group intake amount when defining food group ‘consumers’( Reference Arimond and Ruel 1 , Reference Savy, Martin-Prével and Danel 8 – Reference Arimond, Wiesmann and Becquey 12 ). An assessment of some of these studies shows that imposing a standard 10 g or 15 g minimum intake produces a DDS that not only has a stronger association with nutrient intake but also shows a greater resilience to energy adjustment and has a better ability to differentiate children or women with different intake levels of nutrient adequacy( Reference Kennedy, Pedro and Seghieri 10 – Reference Daniels, Adair and Popkin 13 ). While the use of a fixed minimum amount across all food groups is easy to apply, such a set minimum may not consider varying contributions of food groups to nutritional needs. Accounting for these differences may require the use of varying minimum amount requirements depending on the food group’s nutrient profile. In the current analysis, we explore the use of multiple methods of calculating DDS, based on standard and variable minimum food group amounts, in predicting dietary micronutrient adequacy among schoolchildren in rural Kenya.
Background
The present analysis utilized baseline data collected as part of the Child Nutrition Project (CNP), a group randomized controlled feeding intervention study carried out in Kyeni South Division of Embu District in Eastern Province, Kenya from 1998 to 2000( Reference Neumann, Bwibo and Murphy 14 ). The study area is rural and subsistence farming is the primary occupation. The CNP baseline data were collected between June and August 1998. All children enrolled in grade 1 (median age 7·4 years) from twelve selected primary schools participated in the CNP study. Human Subjects Approval was obtained for the research study from the University of California, Los Angeles; the University of Nairobi, School of Medicine, Kenya; and the Office of the President, Government of Kenya. Local and district authorities helped facilitate implementation of the study. The community was informed in detail about the aim and procedures of the research study. Informed verbal consent from parents of study children was obtained before the study.
Experimental methods
Food intake
Dietary information consisted of up to three non-consecutive 24 h recalls for each of the schoolchildren during the baseline period. Information on daily intake for each schoolchild was obtained, with the mothers being the main respondents. A majority of the recall interviews were conducted between 08.00 and 16.00 hours when children were away at school. A relatively small but unknown number of schoolchildren were invited to assist with the interview when present and if the main respondent permitted. Previous analysis has shown that school-age children from this population consume an average of one or two foods, mostly fruits and starchy foods, outside the home and 51–84 % of children’s out-of-home foods, depending on season, were omitted from mothers’ recalls( Reference Gewa, Murphy and Neumann 15 ). Women with experience in dietary data collection methods from a previous study were re-trained on the multi-pass strategy which was used to conduct 24 h recall interviews at home( Reference Murphy, Gewa and Liang 16 ). The enumerators did not work over the weekend and so the dietary data collected reflected what was consumed on any one day from Sunday through Thursday. Procedures followed in determining dietary intake have been previously described( Reference Murphy, Gewa and Liang 16 ). In summary, mothers were asked to estimate amounts of foods consumed by schoolchildren and the ingredients used in mixed dishes prepared in the home. The CNP research team designated some of the common household measures, such as cups and tins, and defined two-dimensional food models to be used in estimating food amounts. These measures were later converted to gram weights using a database developed for this purpose( Reference Murphy, Gewa and Grillenberger 17 ). Respondents were also asked to describe foods prepared outside the home, including the primary ingredients for any out-of-home mixed dishes. A standard recipe was used when ingredients were not available for an out-of-home mixed dish( Reference Murphy, Gewa and Liang 16 , Reference Murphy, Gewa and Grillenberger 17 ). None of the participating schools provided school lunch.
Food group intakes and diet diversity scores
Schoolchildren’s intakes were categorized into nine food groups that were specific to the study population and maximized on specific nutrient contributions. For each day of dietary data, amounts of food consumed were assigned to one of nine food groups: (i) cereals and tubers; (ii) dark-green leafy vegetables (DGLV); (iii) vitamin A-rich fruits and vegetables (VAFV); (iv) vitamin C-rich fruits and vegetables (VCFV); (v) other fruits and vegetables (OFV); (vi) dairy; (vii) meat–fish–poultry (MFP); (viii) eggs; and (ix) legumes and nuts. Recipes were disaggregated into ingredients before these assignments were made. Schoolchildren’s MFP (6·18 (sd 0·98) g) and egg (0·97 (sd 0·18) g) intakes were extremely low and a decision was made to combine the two food groups into one ‘meat–fish–poultry–eggs (MFPE)’. Different scores for diet diversity were developed based on minimum intake amounts with 1 point assigned for each food group up to a maximum of 8 points. The first score, DDS-1, was based on a standard minimum intake of 1 g of food per food group. The second score, DDS-15, was based on a standard minimum intake of 15 g of food per food group. The third score, DDSRDA, used different minimum amounts for each food group. The minimum was based on the food group’s contribution to children’s RDA or Adequate Intake (AI) of a specific nutrient. The nutrient of interest for each food group was determined based on nutrients of concern within this population( Reference Murphy, Gewa and Liang 16 ) and on nutrients that are known to be rich within each food group. In the case of the cereals/tubers food group, a comparison of the performance of DDSRDA using either niacin or Fe as the group’s target nutrient showed no significant differences, and a decision was then made to retain Fe as the basic nutrient from the cereals/tubers food group. Mg was identified as the basic nutrient from DGLV, vitamin A from VAFV, vitamin C from VCFV, K from OFV, Ca from dairy and protein from MFPE and legumes/nuts food groups. We then determined the minimum amount of foods within each food group that would supply a meaningful level of the reference nutrient. Food group minimum amounts were then calculated to reflect the amount of food per food group required to meet 50 % (25 % for OFV) of the reference nutrient’s RDA or AI (Table 1). For food groups with multiple common foods like the OFV, this value was calculated as the average across the common foods within the group. Thus DDSRDA should better reflect how well the diet meets an individual’s nutrient needs. The fourth score, DDS50pct, utilized variable minimum amounts across food groups, in which minimum amounts reflected schoolchildren’s median intake level for each specific food group (Table 2). Because the median intakes for VAFV and MFPE were both at 0 g, a score of 1 was given for any intake amount above 0 g for these two food groups. For the last score, DDS90pct, minimum intake amounts were assigned to reflect schoolchildren’s 90th percentile intake level for each food group. The 90th percentile cut-off was chosen to reflect the point when intake values were greater than 1 g for all food groups among this group of children (Table 2).
Table 1 Minimum food group amounts for the DDSRDA
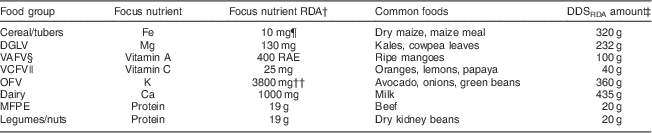
DDS, diet diversity score; DDSRDA, minimum intake based on children’s RDA; DGLV, dark-green leafy vegetables; VAFV, vitamin A-rich fruits and vegetables; VCFV, vitamin C-rich fruits and vegetables; OFV, other fruits and vegetables; MFPE, meat–fish–poultry–eggs; RAE, retinol activity equivalents; AI, Adequate Intake.
† RDA from the Institute of Medicine( 19 ).
‡ DDSRDA amounts will supply approximately 50 % of the RDA for the focus nutrients, except for the OFV amount which is based on meeting 25 % of the AI for K.
§ High carotenoids: >130 RAE/100 g.
|| High vitamin C: >18 mg/100 g.
¶ Assumes 8 % Fe availability from children’s diets( Reference Murphy, Beaton and Calloway 18 ).
†† AI for K used as the reference value.
Table 2 Daily food group intake amounts: absolute and by diet diversity scoreFootnote †

pct., percentile; DDS, diet diversity score; DDS-1, minimum intake of 1 g of food per food group; DDS-15, minimum intake of 15 g of food per food group; DDSRDA, minimum intake based on children’s RDA; DDS50pct, minimum intake based on median food group intake level; DDS90pct, minimum intake based on 90th percentile food group intake level; DGLV, dark-green leafy vegetables; VAFV, vitamin A-rich fruits and vegetables; VCFV, vitamin C-rich fruits and vegetables; OFV, other fruits and vegetables; MFPE, meat–fish–poultry–eggs.
† Intakes based on 1544 d of dietary data from 529 rural Kenyan schoolchildren, mean age 7·00 (sd 1·41) years.
‡ The median intake for VAFV and MFPE were both at 0 g. The values represent percentage of intakes above 0 g for these two food groups.
Nutrient intakes and probability of nutrient adequacy
Nutrient intakes were calculated for each individual schoolchild, for each day, using an international food composition table adapted for use in the CNP study( Reference Murphy, Gewa and Liang 16 , Reference Murphy, Gewa and Grillenberger 17 ). The database contains complete nutrient values for the foods commonly consumed in rural Kenya. Nutrient contents of less common foods were estimated from similar foods.
Intakes of available Fe and available Zn were calculated using the approach described by Murphy et al.( Reference Murphy, Beaton and Calloway 18 ), which considers the levels of dietary factors that enhance or inhibit absorption of these nutrients. The Estimated Average Requirements (EAR) for these nutrients assume 18 % Fe availability and 30 % fraction absorbability of Zn( 19 ). Thus, we adjusted the EAR values to reflect absorbed Fe and Zn requirements before estimating nutrient adequacy.
The adequacy of micronutrient intake was estimated for the following fourteen micronutrients: Fe, Zn, Ca, Mg, K, vitamin A, vitamin B6, vitamin B12, riboflavin, folate, niacin, thiamin, vitamin C and vitamin E. We did not include protein adequacy in these calculations because previous analyses had shown that protein was adequate for most schoolchildren in this region, even after adjusting for protein quality( Reference Murphy, Calloway and Beaton 20 ). Protein intakes for the current dietary data also showed mean intakes that were well above recommendations (53 g/d v. an RDA of 36 g/d). The probability of adequacy (PA) for each nutrient in each day’s diet was calculated using the approach recommended by the Institute of Medicine( 21 ). The probnorm function of SAS, based on the EAR, was used for all nutrients except Fe and K. The requirement distribution for Fe is skewed, so published PA values for specific ranges of intake were used( Reference Murphy, Calloway and Beaton 20 ). Because K does not have an EAR, the PA was defined using the AI as follows: 0 % for K intakes≤one-quarter of the AI; 25 % for one-quarter AI<K intakes≤one-half AI; 50 % for one-half AI<K intakes≤three-quarters AI; 75 % for three-quarters AI<K intakes≤AI; and 100 % for AI<K intakes. For each nutrient, the mean PA across all days of intake is approximately equal to the prevalence of days with adequate intake. However, these prevalence rates should not be interpreted as the adequacy of usual long-term intakes for the population because they are based on single days of intake data.
The mean probability of adequacy (MPA), an overall measure of micronutrient adequacy of the day’s diet, was calculated as an average of the individual nutrient PA and expressed as a percentage. Two forms of the MPA were calculated to: (i) include all fourteen nutrients of interest (MPA1); and (ii) exclude K (MPA2).
Statistical analyses
A total of 529 children with ages ranging from 4·17 to 14·50 years and a mean age of 7·00 (sd 1·41) years were included in the analysis. Of these, 486 had three days of 24 h recalls while forty-three had two days of 24 h recalls, giving a total of 1544 d of 24 h recalls. Bivariate longitudinal random-effects models were fit with REML (restricted maximum likelihood) in the SAS mixed procedure (SAS statistical software package version 9) to assess the correlation between children’s mean DDS (each of five scores) and children’s mean probability of nutrient adequacy (MPA1 and MPA2)( Reference Weiss 22 ). This model has two correlations, one correlation between the average levels of the two variables and one correlation between the day-to-day variations in the two variables. We report the correlation between average levels of the two variables in all cases. Single longitudinal random-effects models were used to assess the relationship between mean DDS (each of five scores) and MPA, with the MPA being a continuous outcome variable. These sets of analysis were all adjusted for energy intake.
Results
Daily food and nutrient intakes
Schoolchildren’s mean daily intakes of DGLV, VAFV, VCFV, OFV and MFPE were below 50 g (Table 2). Dairy and legumes/nuts mean intakes were approximately 90 g/d, while cereals/tubers mean intakes were highest at 515 g/d. MFPE and VAFV intake levels were virtually non-existent with a median intake of 0 g. The percentage of children who had consumed minimum food group amounts varied from 11 % (MFPE) to 100 % (cereals/tubers) for DDS-1, from 10 % (MFPE) to 100 % (cereals/tubers) for DDS-15 and from 1 % (DGLV) to 85 % (legumes/nuts) for DDSRDA (Table 2). Mean scores of diet diversity were highest for DDS-1 (4·71 (sd 0·04)) and lowest for DDS90pct (0·80 (sd 0·02)).
The nutrient with the lowest PA was Ca (47 %), while the nutrient with the highest PA was Fe (74 %; Table 3). When considering all nutrients, the overall MPA was 57 % (MPA1), and 56 % when K was excluded (MPA2).
Table 3 Daily nutrient intakes: nutrient amount and probability of nutrient adequacyFootnote †

RAE, retinol activity equivalents; MPA, mean probability of nutrient adequacy; MPA1, includes K; MPA2, excludes K; N/A, not applicable.
† Intakes based on 1544 d of dietary data from 529 rural Kenyan schoolchildren, mean age 7·00 (sd 1·41) years.
‡ Amount of nutrient available for utilization after accounting for inhibitory and enhancing factors( Reference Murphy, Beaton and Calloway 18 ).
Correlations between diet diversity scores and probability of nutrient adequacy
Energy intake was highly correlated with MPA1 (Table 4). All five DDS were significantly correlated with both MPA1, with correlations ranging from 0·25 for DDS-1 to 0·70 for DDSRDA. However, after adjusting for energy intake, correlation coefficient values decreased substantially and statistical significance was sustained only for the correlation between MPA1 and DDSRDA.
Table 4 Correlation between dietary diversity scores and mean probability of nutrient adequacyFootnote †

MPA, mean probability of nutrient adequacy; MPA1, includes K; MPA2, excludes K; DDS, diet diversity score; DDS-1, minimum intake of 1 g of food per food group; DDS-15, minimum intake of 15 g of food per food group; DDSRDA, minimum intake based on children’s RDA; DDS50pct, minimum intake based on median food group intake level; DDS90pct, minimum intake based on 90th percentile food group intake level; N/A, not applicable.
*Correlation coefficient is significantly different from zero, P<0·05.
† Based on 1544 d of dietary data from 529 rural Kenyan schoolchildren, mean age 7·00 (sd 1·41) years.
Again energy intake was highly correlated with MPA2. All five DDS were significantly correlated with both MPA2, with correlations ranging from 0·28 for DDS-1 to 0·70 for DDSRDA. However, after adjusting for energy intake, correlation coefficient values decreased substantially and statistical significance was sustained only for the correlations between MPA2 and DDS-15 and MPA2 and DDSRDA.
Relationship between diet diversity scores and probability of nutrient adequacy
A one-unit increase in each DDS was associated with a significant increase in MPA1 and MPA2 (data not shown). However, regression estimates decreased but remained statistically significant after adjusting for energy intake. A one-unit increase in DDS-1, DDS-15, DDSRDA, DDS50pct and DDS90pct was associated with an increase of 0·38, 0·80, 2·23, 0·84 and 1·06 units in MPA1, respectively. A one-unit increase in DDS-1, DDS-15, DDSRDA, DDS50pct and DDS90pct was associated with an increase of 0·42, 0·67, 1·72, 0·67 and 0·86 units in MPA2, respectively.
Discussion
Schoolchildren’s diets were predominantly cereal-based and lacking in fruits and vegetables, dairy foods and MFPE. Daily MFPE intake amounts were extremely low. On the other hand, legumes/nuts intake was higher with only 10 % of the legumes/nuts intake falling below 20 g/d. For the most part, schoolchildren’s micronutrient intake levels were a reflection of the lack of dietary diversity. Nutrient intake levels were low and were similar to those previously reported( Reference Murphy, Gewa and Liang 16 ). The probability of nutrient adequacy fell below 75 % for all nutrients. With or without K in the MPA measure, children’s MPA percentages showed an overall inadequate dietary nutrient intake (<60 %).
Our eight-food-group system shared a number of similarities to the recently recommended nine-food-group system also known as the Women’s Dietary Diversity Score (WDDS) and the seven-group young children’s (6 to 23 months old) DDS( Reference Arimond, Wiesmann and Becquey 12 , Reference Kennedy, Ballard and Dop 23 , 24 ). All three systems have specific food groups for cereals and tubers, dairy products and legumes and nuts. It is however important to note that our system differed from the WDDS in three specific ways: (i) we categorized VAFV and VCFV into two separate food groups; (ii) we did not categorize organ meats and flesh foods into two separate food groups; and (iii) we combined the MFP and egg categories into one food group due to the low intake values. Although Arimond et al. showed that the nine-food-group system combined better performance and ease of implementation( Reference Arimond, Wiesmann and Becquey 12 ), we selected to use eight food groups in our analysis to allow for ‘food group’ key nutrient homogeneity and practicality within our specific population.
The magnitude of the DDS examined in the current analysis was highest when the 1 g minimum food group intake cut-off was used (4·71 (sd 0·04)) and lowest when the 90th percentile of intake was used as the cut-off (0·80 (sd 0·02)). When intermediate cut-offs were chosen, as for DDSRDA and DDS50pct, mean scores were 2·22 and 3·33, respectively. The correlation coefficient between DDSRDA and MPA was the highest even after adjusting the analyses for energy intake. Previous studies have shown that imposing a minimum food group intake amount that is higher than 1 g results in stronger associations between DDS and nutrient intakes. A recent study among women in five low-income nations showed that across study sites (Burkina Faso, Mali, Mozambique, Bangladesh and the Philippines), the correlations between the 15 g minimum-diversity score and nutrient intakes were at least 27 %, 20 %, 10 %, 5 % and 21 % higher than those between the 1 g minimum-diversity score and nutrient intakes, respectively( Reference Arimond, Wiesmann and Becquey 12 ). A study among non-breast-feeding children in the Philippines showed correlation coefficients were at least 22 % higher when a 10 g minimum food group intake was imposed( Reference Kennedy, Pedro and Seghieri 10 , Reference Daniels, Adair and Popkin 13 ). Similar comparisons showed differences ranging from 2 % to 17 % among young children in Madagascar( Reference Moursi, Arimond and Dewey 11 ). The DDS-15 outperformed DDS-1 by 39–44 % in the current analysis. The largest difference was shown between DDSRDA and DDS-1 with the correlation coefficient between DDSRDA and MPA being over 150 % higher than the correlation coefficient between DDS-1 and MPA. The DDSRDA also outperformed the DDS-15 with a difference of at least 80–94 % in the correlation coefficients. These differences were heightened (95–129 %) after adjustment for energy intake. The RDA-based diversity score, DDSRDA, was shown to have a stronger and more consistent association with mean probability of nutrient adequacy than the four other DDS measures among schoolchildren in rural Kenya and thus better reflects how well the schoolchildren’s diets meet their nutrient needs.
We identified a focus nutrient for each food group and used 50 % of the RDA/AI to determine an amount of food from the group that would provide a meaningful contribution to the recommended intake of the nutrient. Such an application was not done to imply that a specific nutrient was supplied only from one food group. Rather, it allowed for ease of application and was guided by the assumption that the remainder 50 % (or 75 % in the case of K) would be provided from other food groups. However, the selection of the nutrients and the use of 50 % of the RDA were country-specific, and might not be ideal in other populations. A related concern regarding these analyses is that the minimum amounts selected for five of the eight food groups for the DDSRDA were well above amounts commonly consumed. Less than 10 % of the days of intake contained intakes at the minimum levels for DGLV, VAFV, OFV, dairy and MFPE. DDSRDA was typically composed of legumes/nuts, cereal/tubers and VCFV. Further analyses should examine the effect of lowering the minimum requirement for these food groups, to see if the associations of DDSRDA with MPA can be increased. Only seven nutrients were used to determine these minimum amounts, although we included fourteen nutrients in the MPA calculation. A more comprehensive approach that looked at food group amounts that would provide recommended levels of all nutrients might be considered in the future. For example, the type of modelling that was used to develop the US Department of Agriculture’s food patterns could be utilized( Reference Britten, Marcoe and Yamini 25 ). Although it may appear that using recommended nutrient intake to determine food group amounts and then evaluating how well the DDS predicts overall adequacy is circular, this approach is likely to result in a diversity scoring scheme that is better able to predict overall nutrient adequacy.
These results highlight the importance of accounting for food group nutrient content, in relation to recommended values, when defining DDS. To our knowledge, the present set of analyses is the first to take this approach when defining DDS. However, there is still a need for additional research to assess how well such an indicator would perform among other population groups and to define simpler ways of incorporating the variable minimum intake amounts. In this population of schoolchildren in Africa, the DDSRDA performed well in explaining nutrient intake, and the results contribute to the continued search for more valid dietary quality indicators in low-income nations.
Acknowledgements
Acknowledgements: The authors would like to thank all the participants and workers who participated in the CNP. Financial support: This work was carried out as part of the CNP project which was supported by the Global Livestock Collaborative Research Support Program (GL CRSP), University of California at Davis, through the US Agency for International Development (USAID) (grant number PCE-G-00-98-00036-00). Both GL CRSP and USAID had no role in the design, analysis or writing of this article. Conflict of interest: None. Authorship responsibilities: C.A.G. was the field nutritionist for CNP, conducted the research, performed the statistical analysis and wrote the paper. S.P.M. was Co-Investigator for CNP, assisted with the research and the statistical analysis, and edited the paper. R E.W. provided guidance in the statistical analysis used in this paper and edited numerous drafts. C.G.N. was the Principal Investigator for CNP, was responsible for the study design, oversaw overall research activities, and helped guide the writing of the manuscript. All authors read and approved the final manuscript. Ethics of human subject participation: Human Subjects Approval was obtained for the research study from the University of California, Los Angeles; the University of Nairobi, School of Medicine, Kenya; and the Office of the President, Government of Kenya.