1. Introduction
Eating is central to human behavior. Some evolutionary accounts suggest humans are evolutionarily optimized to eat cooked food (e.g., Carmody & Wrangham, Reference Carmody and Wrangham2009; Wrangham & Conklin-Brittain, Reference Wrangham and Conklin-Brittain2003), as indicated by the reduction of tooth and jaw size observed approximately 100,000 years ago (Brace et al., Reference Brace, Smith, Hunt, Kelley and Larsen1991), and by the fact that people cannot survive on a raw-food diet, except under unusual circumstances (e.g., extremely sedentary lifestyle; Koebnick et al., Reference Koebnick, Strassner, Hoffmann and Leitzmann1999). All human populations cook (Harris, Reference Harris, Jones, Martin and Pilbeam1992). This suggests cooking is a pan-human trait, with human biology adapted for consuming processed and cooked food.
Previous behavioral studies investigating how people conceptualize different types of foods have noted macro-distinctions (e.g., between natural vs. processed food), processes of categorization and induction (Ross & Murphy, Reference Ross and Murphy1999), and the food habits and preferences of different populations (Antmann et al., Reference Antmann, Ares, Varela, Salvador, Coste and Fiszman2011; Goldner et al., Reference Goldner, Sosa and Garitta2021; Hough & Ferraris, Reference Hough and Ferraris2010; Libertino et al., 2012). For example, Ross and Murphy (Reference Ross and Murphy1999) found knowledge of food is organized using both taxonomic categories (e.g., fruit, cereals) and what they called script categories (e.g., breakfast food, junk food), suggesting everyday interactions with food shape knowledge. Along the same lines, Hough and Ferraris (Reference Hough and Ferraris2010) asked Argentinian teenagers to perform a free-listing task for the category of fruit, and uncovered preliminary evidence that categorical knowledge of fruits differs both quantitively and qualitatively between low-income and medium-/high-income participants.
More recently, it has been proposed there is a critical distinction between “natural” (e.g., apple, carrot) and “manufactured” (e.g., cake, lasagna) foods (e.g., Capitani et al., Reference Capitani, Laiacona, Mahon and Caramazza2003; Rumiati & Foroni, Reference Rumiati and Foroni2016). Neuropsychological, neuroimaging, and behavioral evidence shows these are represented and retained in memory differently. For instance, Aiello et al. (Reference Aiello, Vignando, Foroni, Pergola, Rossi, Silveri and Rumiati2018) compared the episodic memory of people who were healthy and young, healthy and centenarians, as well as those with Alzheimer’s Disease, or who had Progressive Primary Aphasia for natural (e.g., fruits, vegetables) and transformed food (e.g., hotdogs, chocolate). They found that transformed food was remembered better by younger participants and seemed more resilient to brain damage than natural food in patients. Similarly, in a Go/No-Go association task testing the association between foods with different levels of processing and words related to safety (e.g., “pure”, “harmless”), Coricelli et al. (Reference Coricelli, Rumiati and Rioux2022) found faster response times to processed than unprocessed foods. Finally, in an EEG study, Coricelli et al. (Reference Coricelli, Toepel, Notter, Murray and Rumiati2019) recorded visual evoked potentials (VEP) of healthy participants presented with images of processed and unprocessed foods equated in caloric content. They found differences in VEPs between processed and unprocessed foods as early as 130 ms after stimulus onset, as well as distinct activation of distributed brain areas.
Taken together, these findings suggest processed food is extremely important and processed food might be especially cognitively salient. Indeed, one interpretation of these findings is that transformed food is remembered better due to its caloric content, or because it lessens the chances of being poisoned or infected, which makes it crucial from a biological-evolutionary perspective (Carmody & Wrangham, Reference Carmody and Wrangham2009; Coricelli et al., Reference Coricelli, Rumiati and Rioux2022; Rumiati et al., Reference Rumiati, Foroni, Pergola, Rossi and Silveri2016). Along these lines, it might also be hypothesized that processed foods would be lexicalized differently to natural foods, given their importance to humans. In fact, it has been suggested that language is optimized for the communicative needs of interlocutors and so things of cultural importance and salience will be more linguistically elaborated (e.g., Floyd et al., Reference Floyd, Roque and Majid2018; Kemp et al., Reference Kemp, Xu and Regier2018; Winter et al., Reference Winter, Perlman and Majid2018).
Rumiati and Foroni (Reference Rumiati and Foroni2016) propose that processed and natural food are represented as distinct categories in the semantic system, following the distinction between living and non-living entities proposed by Sensory-Functional Theory (Warrington & Shallice, Reference Warrington and Shallice1984). They suggest natural food concepts are characterized primarily by sensory information (e.g., shape, color, taste), whereas processed food concepts are characterized in terms of functional information (e.g., the occasion in which they are usually consumed, their preparation procedures). Consistent with this, an EEG study found a larger N400 for natural food after functional primes (e.g., “suitable for a wedding meal”) but a larger N400 for manufactured food after sensory primes (“tastes sweet”; Pergola et al., Reference Pergola, Foroni, Mengotti, Argiris and Rumiati2017; see also Vignando et al., Reference Vignando, Aiello, Rinaldi, Cattaruzza, Mazzon, Manganotti, Eleopra and Rumiati2019). However, recent evidence indicates that experience and familiarity may also play a role in shaping semantic memory for natural or transformed food. In a study comparing Italian healthy young–old (51–64 years), old–old (77–91 years), and centenarian (100–108 years) individuals in different semantic tasks, Vignando et al. (Reference Vignando, Aiello, Foroni, Marcon, Tettamanti and Rumiati2018) found centenarians – who report consuming processed food less frequently than natural food – were also better at naming natural food. The remaining two groups showed the opposite pattern. These results suggest food recognition, and the semantic processes that underlie it, are influenced by experience.
While food is universally salient, regional cuisines and culinary traditions differ greatly across cultures (for a review see Mintz & Du Bois, Reference Mintz and Du Bois2002). Cultures also differ in what they consider edible or inedible, following adaptive and cultural-symbolic norms (see Falk, Reference Falk1991). In fact, despite being a primary necessity, food consumption and the practices that revolve around it can be considered, at least in part, a cultural construction whose impact extends to identity processes (Appadurai, Reference Appadurai1988; Holtzman, Reference Holtzman2006). Food, culture, and language are so interwoven that structuralist anthropologist and ethnologist Lévi-Strauss (Reference Lévi-Strauss, Counihan and veal Esterile2013) proposed a model used to study languages for analyzing culinary systems. He suggested cooking is embedded in a semantic triangle composed of three extremes – raw, cooked, and rotten – representing the opposition between nature and culture (and elaborated/unelaborated), and that each term is defined differently in different societies. As an example, he mentioned the Italian notion of “raw” entailed in the consumption of crudités was far more extended than the traditional French one. Recent semiotic accounts of food also draw an analogy between the food and language in many respects (e.g., Riley & Cavanaugh, Reference Riley and Cavanaugh2017). For instance, people use food to construct and express categorical distinctions and socio-cultural identities (e.g., kosher taboos, Valentine chocolates, see also Douglas, Reference Douglas1979; Fischler, Reference Fischler1988). So, while being somehow constrained by natural and physical boundaries, the category of food seems to also be shaped by cultural and social forces (see Monaco & Bonetto, Reference Monaco and Bonetto2019).
Here, we sought to investigate the semantic knowledge – or semantic memory – of three categories of food (pasta, vegetables, and fruits), using a free-listing task with Italian and English-speaking participants. Semantic memory is broadly defined as the knowledge we possess about things in the world, and is said to be influenced by perceptual and life experiences as well as by linguistic associations (Lupyan & Lewis, Reference Lupyan and Lewis2019; Wulff et al., Reference Wulff, De Deyne, Aeschbach and Mata2022). Among the methods used to explore semantic knowledge, free-listing tasks have been extensively employed, including for the cross-cultural investigation of different conceptual domains (e.g., food, Hough & Ferraris, Reference Hough and Ferraris2010; landscape, van Putten et al., Reference van Putten, O’Meara, Wartmann, Yager, Villette, Mazzuca and Majid2020; odors, Wnuk & Majid, Reference Wnuk and Majid2014). The free-listing method is “a deceptively simple, but powerful technique” (Bernard, Reference Bernard2006, p. 301) to elicit information about the mental representation of concepts and categories, and rests upon the assumption that exemplars listed more frequently by most participants are more widely shared, familiar, and cognitively salient within a specific cultural group (Ross, Reference Ross2004; Stausberg, Reference Stausberg2021).
Free-listing data can be analyzed using a plethora of different techniques to shed light on conceptual relations within categories. Among these, semantic networks constitute an interesting option among multivariate techniques (see review by Siew et al., Reference Siew, Wulff, Beckage and Kenett2019). In network analyses, words are represented as nodes and associative relations between words (i.e., edges) are estimated based on different criteria, such as frequency or co-occurrence – hence providing a powerful means to investigate the structure of lexicalized categories (Baronchelli et al., Reference Baronchelli, Ferrer-i-Cancho, Pastor-Satorras, Chater and Christiansen2013). For instance, Krethlow et al. (Reference Krethlow, Fargier and Laganaro2020) leveraged lexical-semantic networks for the analysis of free-association data comparing six different age groups (from 10 to 80 years old), and found striking differences in the semantic associations of cohorts. The authors also showed that measures deriving from semantic networks predicted specific behavioral (linguistic) performance of each age group (see also Dubossarsky et al., Reference Dubossarsky, De Deyne and Hills2017; Mazzuca et al., Reference Mazzuca, Falcinelli, Michalland, Tummolini and Borghi2021; van Putten et al., Reference van Putten, O’Meara, Wartmann, Yager, Villette, Mazzuca and Majid2020).
With the aim of tapping into participants’ semantic knowledge of food in two cultures, we presented individuals with three target categories, including one processed food (pasta) and two natural food types (vegetables and fruits). We were primarily interested in investigating whether the representation of processed food of pasta differs between Italian and English-speaking participants since they have differential experience and cultural scripts for this food type. There are differences, for example, in the preparation and consumption of pasta at different stages of transformation: Italians make extensive use of pasta (Altamore et al., Reference Altamore, Ingrassia, Columba, Chironi and Bacarella2020), and it is associated with concepts such as “family” and “home” (Altamore et al., Reference Altamore, Ingrassia, Chironi, Columba, Sortino, Vukadin and Bacarella2018), suggesting a high degree of familiarity. Italians might therefore be considered “pasta experts” (Altamore et al., Reference Altamore, Ingrassia, Columba, Chironi and Bacarella2020), and this expertise might influence their conceptual knowledge (López et al., Reference López, Atran, Coley, Medin and Smith1997; Medin et al., Reference Medin, Lynch, Coley and Atran1997). As a comparison, we included vegetables and fruits as a benchmark for how the two groups compared for natural categories where arguably there is less cultural variation. According to national surveysFootnote 1, most Italian, American, and British participants fail to meet the daily recommended intake of vegetables and fruits (i.e., five portions per day), with 10% of Italians, around 10% of American, and 28% of British respondents meeting this goal.Footnote 2 So, overall Italian and English participants have low consumption of vegetables and fruit against recommended guidelines.
Although our study is exploratory in nature, differences in the conceptual organization of pasta between Italian and English-speaking participants can be hypothesized based on the existing literature. According to the distinction proposed by Rumiati and Foroni (Reference Rumiati and Foroni2016) we should observe different patterns for processed versus natural foods. In this case, vegetables and fruits should mostly be represented in terms of sensory properties (e.g., items with the same shape or color should cluster together), while pasta should mostly be organized in terms of functional or event-related properties (e.g., pasta types that are cooked similarly should cluster together). Pasta shapes and types are so variegated that the linguistic knowledge of different kinds of pasta has been defined “a terminological tower of Babel” (Zanini De Vita, Reference Zanini De Vita2009), with numerous attempts to draw a taxonomy (cf. Alexander, Reference Alexander2000). We expected English speakers to free list according to superficial sensory properties of pasta (e.g., shape), similar to their strategy for natural foods, but predicted Italians would draw more on functional relations given their greater expertise in the domain of pasta and to also list more exemplars in this domain.
2. Methods
2.1. Participants
A total of 203 participants took part in the experiment, 103 Italian and 100 English. Ethical approval was provided by the Psychology Research Ethics Committee of the University of York. Participants were recruited through social media. For the Italian sample, data were removed if participants did not answer demographic questions (n = 2), completed the experiment in English (n = 1), or indicated they were not Italian native speakers (n = 1). The final sample of Italian participants was therefore 99 participants, of whom 74.7% (n = 74, M age = 35.01; SD = 10.66) identified as women, 24.2% (n = 24, M age = 37.62; SD = 11.51) as men, and 1% (n = 1, age = 37) as other. All indicated Italian was their nationality. The majority of individuals were highly educated: 50% had a Master’s Degree, 19% had a PhD, 18% completed High School, and 12% had a Bachelor’s Degree. Only a small percentage of participants indicated they worked in the food industry (4.04%).
For the English sample, participants who indicated they were not native speakers or whose nationalities did not belong to the Anglosphere broadly construed were excluded (n = 9), as well as participants reporting technical problems (n = 1), resulting in a final sample of 90 participants. English-speaking participants consisted of 65.5% who identified as women (n = 59, M age = 35.49; SD = 8.85), 30% as men (n = 27, M age = 33.59; SD = 7.84), 1.1% as man-queer (n = 1, age = 48), 1.1% as queer (n = 1, age = 30), and 2.2% as woman-queer (n = 2, M age = 32.50; SD = 3.53). English-speaking participants varied in their nationalities, with a preponderance of British participants (82.2%, n = 74), followed by American (7.7%, n = 7), British-American (4.4%, n = 4), Australian (3.3%, n = 3), Irish (2.2%, n = 2). The majority of individuals were highly educated: 37% had a Master’s Degree, 29% had a PhD, 29% had a Bachelor’s Degree, and only 5% completed High School. Only a small percentage of participants indicated they worked in the food industry (8.8%).
2.2. Materials, design, and procedure
The study was implemented as an online questionnaire in Qualtrics. In the first part of the questionnaire, participants were asked to complete a free-listing task for each of the three categories: pasta, vegetables, and fruits. These were presented in English or Italian (pasta, verdura, frutta) as appropriate for participants, with the order of category presentation determined randomly across participants. For each item, participants were asked to list as many exemplars as they could in 3 minutes in their native language. In the second part of the questionnaire, participants provided demographic information such as education level, linguistic background, and gender identity. Finally, participants answered five questions related to their expertise with food and pasta. We first asked whether individuals worked in the food industry; then we assessed with open questions how many times per week they ate pasta, how many times per week they ate prepared pasta (e.g., pre-prepared pasta from supermarkets, restaurants, etc.), how many times per week they cooked pasta, and finally how many times per month they prepared pasta from scratch (e.g., making the dough; see Table 1).
Table 1. Questions related to participants’ expertise with food and pasta.
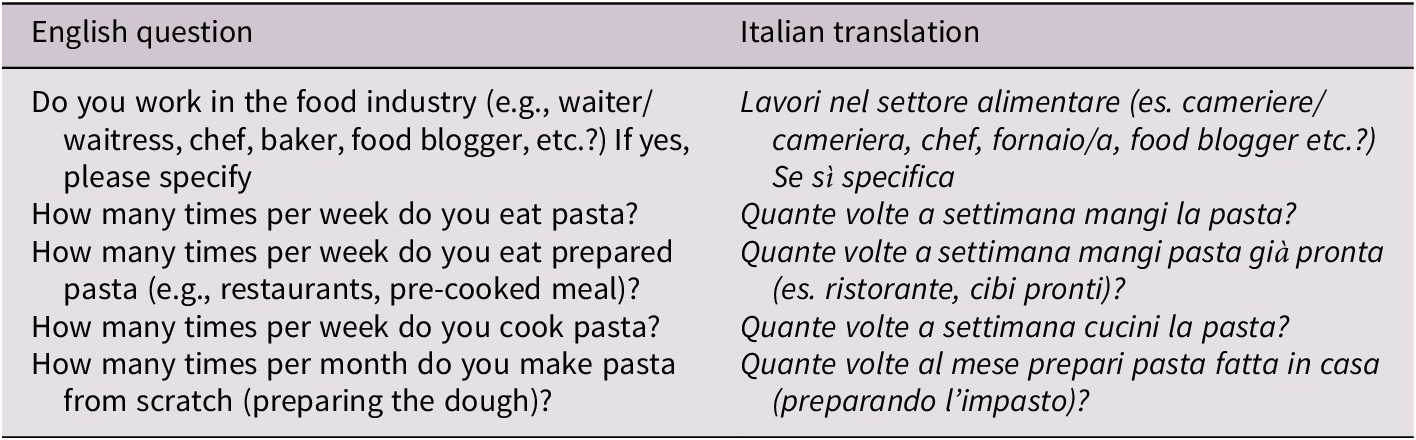
2.3. Data analysis
To prepare for data analysis, free-listing data were pre-processed by changing all upper-case letters into lower case and correcting typos and obvious misspellings. Alternative spellings of the same exemplars, regional variants, and singular and plural forms of the same word (e.g., apple, apples) were unified (i.e., apple). Open responses related to pasta habits were recoded into categorical responses. For questions about weekly consumption and preparation of pasta, we used the following coding scheme: Never = less than once a week; Rarely = between 1 and 2 times a week; Often = between 3 and 4 times a week; Very often = between 5 and 6 times a week; Always = more than 6 times a week.
All analyses were performed using R Statistical Software (v4.0.3; R Core Team, 2020). To assess whether Italian and English participants differed in their habits related to the consumption, cooking, and making of pasta we used chi-squared tests with Yates’ continuity correction implemented through the “janitor” R’s package (Firke, Reference Firke2021). To assess whether English and Italian participants differed in the number of exemplars they listed, we performed a Poisson regression using the “MASS” R’s package (Venables & Ripley, Reference Venables and Ripley2002) with the number of exemplars as the dependent variable, and Culture (English vs. Italian), Food (Pasta vs. Vegetables vs. Fruit), and their interaction as predictors. The model showed over-dispersion, with residual deviance (926.74) greater than the degrees of freedom (557), so we fit a second model using a negative binomial regression (see also Winter et al., Reference Winter, Perlman and Majid2018), that relaxes the variance from the mean (Winter, Reference Winter2019). The second model showed a better fit than the first (Model 1 AIC = 3630.8; Model 2 AIC = 3554), so we rely on the second model for interpretation. To assess the significance of predictors and interaction effects we used likelihood ratio tests on nested models. Paired comparisons were performed using Tukey’s correction’s method with “emmeans” R’s package (Lenth, Reference Lenth2021), using 95% confidence intervals. As a further control, we fit four separate models with the same structure of Model 2 and with the addition of each question related to pasta habits as a predictor and in interaction with Culture and Food. However, we focus specifically on Model 2 in the discussion of results as it features all the predictors theoretically relevant to our hypotheses while representing the simplest explanation of the dataset (Meteyard & Davies, Reference Meteyard and Davies2020).
To further investigate the categorical structure of pasta, vegetables, and fruit in the two cultures, we computed the cognitive salience index of the 10 most frequently listed exemplars and created undirected weighted semantic networks for each food category. Cognitive salience is defined as an index combining two parameters deemed as central in free-listing data: frequency and item position. Cognitive salience is thus calculated as follows: F/(N × mP) (Sutrop, Reference Sutrop2001; van Putten et al., Reference van Putten, O’Meara, Wartmann, Yager, Villette, Mazzuca and Majid2020), where F is frequency, N is the total sample of participants, and mP is the mean position of the item. The index ranges from 0 to 1; items that have higher scores are considered to be more cognitively salient.
Finally, for each language and each food category, we constructed a matrix of co-occurrences using “tidytext” (Silge & Robinson, Reference Silge and Robinson2016) R package that was used as input to construct undirected networks. Co-occurrences were calculated based on bigrams (i.e., pairs of words produced in succession by participants). We excluded pairs of exemplars that co-occurred less than two times in each dataset, so as to avoid idiosyncratic features. Undirected weighted networks were created (van Putten et al., Reference van Putten, O’Meara, Wartmann, Yager, Villette, Mazzuca and Majid2020) using “tidygraph” (Pedersen, Reference Pedersen2020) and “igraph” (Csardi & Nepusz, Reference Csardi and Nepusz2006) R’s packages. Communities detection was performed using Louvain’s algorithm, and network visualization was powered through Fruchterman-Reingold force-directed layout algorithm. We also calculated normalized betweenness centrality, which measures the number of shortest paths that go through a given node. Nodes with higher betweenness centrality are thought to be influential nodes in the network, because information flows through them. For the semantic networks, before applying any community detection algorithm we computed the modularity of the networks to verify that clustering was not random. Modularity scores higher than 0 indicate that community detection is not random. Data and scripts are available on the OSF at https://osf.io/9tkjd/.
3. Results
As our main research question concerned whether Italian and English speakers differed in their conceptual knowledge of pasta, we first describe the pasta habits of our participants before turning to the free-listing data.
3.1. Pasta habits
Italian and English participants differed in their weekly consumption of pasta: Italian participants ate more pasta on average than English participants,
$ {\chi}^2 $
(4) = 71.741, p = 0.007, with 67% of Italians reporting they often or very often ate pasta weekly, whereas 73% of English speakers said they rarely ate pasta weekly. Italians also consumed more prepared pasta (from, e.g., supermarkets and restaurants),
$ {\chi}^2 $
(4) = 13.311, p = 0.009, and cooked more pasta at home during the week,
$ {\chi}^2 $
(5) = 42.817, p = 0.001, than English participants (see Supplementary Materials for further details). However, there was no difference between Italian and English participants in relation to monthly preparation of pasta from scratch,
$ {\chi}^2 $
(2) = 1.271, p = 0.529, with most of participants (93%) indicating they never did so. Overall, then, we found that Italian participants consumed more pasta than English participants – in line with cultural based expectations – but we did not find a difference between the groups in terms of their familiarity with preparation of pasta from scratch.
3.2. The semantic representation of food: number of exemplars
We first examined the number of exemplars listed by Culture and Food. There was no main effect of Culture,
$ {\chi}^2 $
(1) = 2.323, p = 0.127, a main effect of Food,
$ {\chi}^2 $
(2) = 261.139, p < 0.001, and critically a significant interaction between Culture and Food,
$ {\chi}^2 $
(2) = 132.757, p < 0.001. Overall, Italian speakers listed more exemplars for pasta than English speakers, Z = −10.496, p <0.0001, as predicted. Both groups, in fact, listed the most exemplars for fruit (see Table 2; Fig. 1): Italian, fruit vs. pasta: Z = 5.403, p < 0.0001; fruit vs. vegetables: Z = 4.565, p = 0.0001; English, fruit vs. pasta: Z = 17.867, p < 0.0001; fruit vs. vegetables: Z = 3.369, p = 0.009. For Italians, pasta was comparable to vegetables in terms of the number of exemplars produced, but English speakers produced fewer pasta than vegetable exemplars, Z = −14.706; p < 0.0001. In addition, English speakers listed more exemplars for vegetables than Italian speakers, Z = 3.858; p = 0.001.
Table 2. Mean, standard deviation, and total of exemplars produced by Italian and English participants for the categories of pasta, vegetables, and fruit.


Fig. 1. Number of exemplars listed for pasta, vegetables, and fruit by Italian and English participants. In the boxplots, red squares represent means, black bars medians, and the dots the individual counts per participant.
3.2.1. Number of exemplars and pasta habits
In order to assess whether Italian and English participants’ experience with pasta has an impact on the number of exemplars of pasta listed by participants in each group, we fit four additional negative binomial models with the number of exemplars as dependent variable, and Culture, Food, and four different questions tackling habits related to pasta and their interaction as predictors (see Table 1). We report results from each model below.
3.2.1.1. Weekly consumption of pasta
To understand whether the weekly consumption of pasta has an impact on the number of exemplars listed by Italian and English participants we fit a negative binomial model with number of exemplars as dependent variable, Culture (Italian vs. English), Food (Pasta vs. Vegetables vs. Food) and weekly consumption of pasta (never vs. rarely vs. often vs. very often vs. always), and their interaction as predictors (AIC = 3578). There was no main effect of Culture,
$ \chi $
2(1) = 3.151, p = 0.075, nor of weekly consumption of pasta,
$ \chi $
2(4) = 3.559, p = 0.469. However, there was a main effect of Food,
$ \chi $
2(2) = 266.450, p < 0.001, and a significant interaction between Culture and Food,
$ \chi $
2(2) = 69.770, p = 0.007. No other main effect or interaction was significant, all
$ \chi $
2 < 5.976.
3.2.1.2. Weekly consumption of prepared pasta
To understand whether the weekly consumption of prepared pasta has an impact on the number of exemplars listed by Italian and English participants we fit a negative binomial model with number of exemplars as dependent variable, Culture (Italian vs. English), Food (Pasta vs. Vegetables vs. Food) and weekly consumption of prepared pasta (never vs. rarely vs. often vs. very often vs. always) and their interaction as predictors (AIC = 3516). There was no main effect of Culture,
$ \chi $
2(1) = 1.995, p = 0.157, nor of weekly consumption of prepared pasta,
$ \chi $
2(3) = 6.878, p = 0.075. Once again, there was however a main effect of Food,
$ \chi $
2(2) = 261.222, p < 0.001, and a significant interaction between Culture and Food,
$ \chi $
2(2) = 126.370, p < 0.001. No other main effect or interaction was significant, all
$ \chi $
2 < 6.878.
3.2.1.3. Weekly cooking of pasta
To understand whether the weekly cooking of pasta has an impact on the number of exemplars listed by Italian and English participants we fit a negative binomial model with number of exemplars as dependent variable, Culture (Italian vs. English), Food (Pasta vs. Vegetables vs. Food) and weekly cooking of pasta (never vs. rarely vs. often vs. very often vs. always) and their interaction as predictors (AIC = 3557). There was no main effect of Culture,
$ \chi $
2(1) = 3.736, p = 0.053, nor of weekly cooking of pasta,
$ \chi $
2(4) = 7.270, p = 0.122 There was a main effect of Food,
$ \chi $
2(2) = 276.605, p < 0.001, a significant interaction between Culture and Food,
$ \chi $
2(2) = 93.828, p < 0.001, and a significant interaction between Culture and weekly cooking of pasta,
$ \chi $
2(4) = 12.800, p = 0.012. No other main effect or interaction was significant, all
$ \chi $
2 < 7.270.
3.2.1.4. Monthly preparation of pasta from scratch
To understand whether the experience of preparing pasta from scratch (e.g., kneading the dough) has an impact on the number of exemplars listed by Italian and English participants we fit a negative binomial model with number of exemplars as dependent variable, Culture (Italian vs. English), Food (Pasta vs. Vegetables vs. Food) and monthly preparation of pasta (never vs. less than 5 vs. less than 20) and their interaction as predictors (AIC = 3565). There was no main effect of Culture,
$ \chi $
2(1) = 2.676, p = 0.101, nor of weekly cooking of pasta,
$ \chi $
2(2) = 1.908, p = 0.385. There was a main effect of Food,
$ \chi $
2(2) = 264.079, p < 0.001, and a significant interaction between Culture and Food,
$ \chi $
2(2) = 133.262, p < 0.001. No other main effect or interaction was significant, all
$ \chi $
2 < 3.832.
3.2.1.5. Summary
So, overall we found Italian and English participants differed in the number of pasta exemplars they could produce regardless of specific culinary habits related to pasta consumption and preparation. This suggests that notwithstanding distinctive food habits, being embedded in a specific cultural community with its practices and traditions influences the depth of semantic knowledge.
3.3. The semantic representation of food: cognitive salience
The cognitive salience of exemplars sheds a different light on conceptual knowledge than the previous analyses. Despite the fact that there were differences between the groups in the number of exemplars listed, the cognitive salience profiles showed some similarities (see Table 3). Out of the top 10 most salient exemplars, both groups shared five fruits (apple, banana, pear, orange, strawberry), five vegetables (aubergine, broccoli, carrot, cauliflower, courgette), and seven pasta exemplars (farfalle, fusilli, linguine, penne, ravioli, spaghetti, tagliatelle).
Table 3. Top ten exemplars listed by Italian and English participants for pasta, vegetables, and fruit, their raw frequency, and cognitive salience indices. Exemplars listed by both groups are given in bold.

3.4. The semantic representation of food: semantic networks
Colors are assigned randomly in each network based on the community detection algorithms (Fig. 2).

Fig. 2. Semantic networks for pasta (top panels), vegetables (central panels), and fruit (lower panels) from free-listing data of Italian and English.
We refer broadly to the scheme proposed in the neurocognitive literature on the representation of objects (e.g., Warrington & Shallice, Reference Warrington and Shallice1984) to interpret our results. Accordingly, object features can be distinguished into sensory properties (e.g., properties that might be perceived, such as “sharp”, “rounded”), functional properties (referred to functions, such as “used to cut”), and associative-encyclopedic features (e.g., “typical of summer”).
3.4.1. Pasta
The Italian network of pasta exemplars is composed of 44 nodes (i.e., exemplars) and 122 edges, with a modularity of 0.36 and 5 main communities in the network. The English network is composed of 22 nodes, 94 edges, and a modularity of 0.14 with 4 main communities. In both networks, we find two clearly distinct communities clustering mainly small, stuffed pasta (e.g., ravioli, tortellini), and including lasagne, as well as two communities featuring primarily long pasta (e.g., tagliatelle, pappardelle) suggesting perceptual-based distinctions.
However, we also found differences in the structure of the two networks. For instance, Italian participants seemed to distinguish long pasta according to basic ingredients (i.e., egg-based in the blue community vs. flour-and-water-based in other communities). In addition, pasta seemed to be distinguished according to procedural knowledge. There was a grouping of pasta generally used for soups (green community, e.g., ditalini, stelline, risoni), as well as a distinction between dried (red community) and fresh pasta (purple community). The English semantic network seemed more heterogeneously organized. As well as the “stuffed” and “long” pasta communities, there was a grouping of diverse types of dried pasta that can be frequently found in the supermarkets as ready meals (green community, e.g., farfalle, rigatoni, macaroni), and a more heterogeneous grouping including miscellaneous types of pasta (red community).
Overall, the semantic networks for pasta suggested different conceptual content, despite both groups relying somewhat on perceptual features of pasta. Critically, Italian participants also demonstrated reliance on functional-procedural knowledge about cooking pasta (e.g., “used in soups”), whereas it was not evident in the English participants. Instead, they relied more on generic encyclopedic knowledge (e.g., “can be found in supermarkets”).
3.4.2. Vegetables
The Italian network is composed of 46 nodes and 139 edges, with a modularity of 0.43 and 5 main communities. The English network is composed of 48 nodes and 145 edges, and has a modularity of 0.43. We identified 6 main communities in the network. In both networks, we find a community mainly featuring beans, which could be grounded in either perceptual features or encyclopedic knowledge. Perceptual features were also present in the red communities of both networks, where we found a prevalence of green vegetables. We also found two communities in both networks organized according to functional and encyclopedic knowledge: one where vegetables that go together in many preparations are grouped together (e.g., leek, onion, garlic, potato), and a second one grouping mostly summer vegetables or vegetables that can be used in salads (aubergine, pepper, courgetti, tomato, cucumber).
Even within this natural category, the two groups showed some evidence of culture-specific patterns. For instance, Italian participants frequently mentioned together a number of exemplars of the Brassicaceae family (e.g., cauliflower, broccoli, and different varieties of cabbages), which were sparser in the English network. On the other hand, English-speaking participants clearly distinguished two groups of vegetables based primarily it seems on functional properties, related perhaps to how they grow, e.g., root vegetables (blue community, e.g., turnip, swede, parsnip), and orange vegetables that are often prepared in similar ways, e.g., roasted (purple community, e.g., sweet potato, butternut squash, pumpkin).
3.4.3. Fruit
The Italian network is composed of 46 nodes and 183 edges, with a modularity of 0.39 and 6 main communities. The English network is composed of 52 nodes and 176 edges, with a modularity of 0.46. We identified 8 main communities in the network. In both fruit networks we find two communities organized according to encyclopedic knowledge, one mainly composed of exotic fruits, and a second mostly composed of berries – the latter very conspicuous in the English network. We also found in both networks a community encompassing stone fruits (e.g., plum, peach, apricot). We found some fine-grained differences in the semantic networks between the groups. For example, citrus fruits formed a distinct community in the Italian network, while in the English network, they were scattered across two communities. Interestingly, in the English network tomato and cucumber appear as fruits in the exotic fruit cluster. In addition, Italian participants grouped together nuts as a distinct category, which also happens to be described in Italian by the expression frutta secca, i.e., “dried fruit”.
4. Discussion
Influential accounts of food knowledge propose that processed food is mainly represented in terms of functional and encyclopedic properties, whereas natural food is primarily represented in terms of sensory and perceptual properties (Rumiati & Foroni, Reference Rumiati and Foroni2016). In this study, we found Italian and English-speaking participants differed both quantitatively and qualitatively in their representation of pasta. As predicted, Italian participants listed more exemplars of pasta than English-speaking participants – in line with cultural expectations (Altamore et al., Reference Altamore, Ingrassia, Columba, Chironi and Bacarella2020). In addition, Italian and English-speaking participants differed in their conceptual organization of pasta: while English-speaking participants’ seemed to focus mainly on superficial perceptual distinctions (e.g., “long” and “stuffed”) with a smattering of encyclopedic knowledge (e.g., pasta that can be found as ready meals in the supermarket), Italian responses included further distinctions (e.g., egg-based vs. flour-and-water pasta), suggesting Italians’ conceptual representations of pasta also reflect deeper ingredient-based knowledge. This was the case regardless of habits related to consumption, cooking, and preparation of pasta. So, the greater number of exemplars for pasta listed by Italian participants and the further qualitative differences do not seem to rely on specific eating, cooking, and practical experience with pasta – at least as measured within this study.
Contrary to some suggestions from the literature about the representation of food (Rumiati & Foroni, Reference Rumiati and Foroni2016), we found pasta is not only or primarily represented in terms of functional properties. Instead, both Italian and English-speaking participants distinguished pasta according to a number of different criteria. Among these, Italian responses grouped pasta along sensory properties (e.g., “stuffed”), ingredients (e.g., flour, eggs, water), macro-distinctions (dried pasta), as well as functional properties (pasta used for soups). English responses were not as elaborated, but reflected surface distinctions, e.g., stuffed versus long pasta – showing further reliance on perceptual characteristics.
Despite some global commonalities, Italians and English-speaking participants’ representation of the two natural food categories – vegetables and fruit – also showed some differences, further illustrating the role that culture-specific experiences play in shaping even basic knowledge. In both groups, we found exemplars clustered together on the basis of encyclopedic and procedural knowledge that differed between communities (e.g., vegetables typically used together in recipes). In addition, clusters emerged that specifically related to the lexicalization of certain sub-categories in each language too, as in the case of “stone fruits” for English, and “dried fruit” (i.e., nuts) for Italian.
These results shed further light on the role that experience and culture have in molding the semantic representation of food. We showed that in addition to individual differences in experience playing a role on episodic memory of food concepts (Vignando et al., Reference Vignando, Aiello, Foroni, Marcon, Tettamanti and Rumiati2018), different lifelong experiences across cultures with certain types of food also differently influence semantic representations. Other studies show culture drives sorting and categorization behaviors (e.g., López et al., Reference López, Atran, Coley, Medin and Smith1997), and these differences hold even when controlling for expertise (Medin et al., Reference Medin, Ross, Atran, Burnett and Blok2002, Reference Medin, Ross, Atran, Cox, Wakaua, Coley, Proffitt and Blok2006). As suggested by Ross and Medin (Reference Ross, Medin, Kronenfeld, Bennardo, de Munch and Fischer2011) some of the effects of culture and experience on cognition can be explained in the context of Barslaou’s (Reference Barsalou1991) formulation of goal-derived categories. More specifically, they argue cultural differences in categorization and reasoning may be explained in terms of saliency effects: in a specific cultural environment, certain features might be more or less salient and accessible to individuals, hence differently forging domain knowledge (see also Majid, Reference Majid2021; Majid & Kruspe, Reference Majid and Kruspe2018).
Cross-cultural studies targeting the representation of food mostly focus on consumers’ attitudes and perceptions toward specific foods. For example, Melendrez-Ruiz et al. (Reference Melendrez-Ruiz, Claret, Chambaron, Arvisenet and Guerrero2021), compared free associations of Spanish and French speakers to the word pulses, and found differences between the two communities: Spanish participants (who on average consume more pulses than the French) associated pulses more with cultural heritage and childhood. In a similar vein, Son et al. (Reference Son, Do, Kim, Cho, Suwonsichon and Valentin2014) assessed consumers’ perception of rice in Korea, Japan, Thailand, and France using a word-association task, and found French participants associated rice more frequently with concepts such as “exotic, culture, and travel”, whereas Asian participants associated it more with agricultural products, emotions, and necessary goods. Our study contributes to this literature by illustrating how even food types that are highly familiar to different communities, can nevertheless differ in their conceptual representations based on specific cultural knowledge and usage.
4.1. Future studies and limitations
Although exploratory in nature, this study reveals some intriguing patterns that require further systematic investigation to understand fully. First, our primary goal was to investigate the role culture played in people’s conceptualization of a processed and culturally valued entity – pasta in Italian cuisine – that is nevertheless part of the globalized food industry and so is experienced by English speakers worldwide too. Ideally, we would have had a balanced design with a culturally specific processed food for each group but the perfect Anglo counterpart to pasta eluded us. Moreover, while we focused on one processed food we tested two natural foods, fruits and vegetables. Future studies could better sample across processed and natural food types that were of more or less cultural relevance to distinct populations so as systematically measure the contribution of each factor to the conceptual representation of food.
Second, and related to the selection of food categories, previous studies have found the lexical-semantic processing of food is influenced by intrinsic properties of food, like calorie content: transformed food is perceived as more arousing and higher in calorie content (Rumiati & Foroni, Reference Rumiati and Foroni2016). High-calorie foods also responded more rapidly than low-calorie foods even when caloric information is irrelevant to the task (Harrar et al., Reference Harrar, Toepel, Murray and Spence2011). For this reason, it is important to acknowledge our comparison between pasta, vegetables, and fruit did not take this into consideration. One could speculate high-calorie food, like pasta, should correspondingly elicit more exemplars than fruits or vegetables since transformed food would be of greater significance for humans. This, however, appears not to be the case in our study but this would be an important consideration for future studies.
Third, our study revealed an unexpected difference between English and Italian speakers in the number of exemplars listed for fruits and vegetables, with English speakers listing more for both. It is unclear why this should be the case. The statistics on modern food consumption practices showed that consumption of fruits and vegetables was low across the board (see Introduction), although there was a slight tendency for more British participants to report they ingest the recommended five portions of fruit and vegetable a day, which goes against the general lore that Mediterranean people have a healthier diet overall. Our participants were also comparable in terms of educational backgrounds (i.e., university graduates) which is also known to affect semantic fluency tasks. Perhaps there are differences in the variety of natural foods sold in supermarkets across our cultures, but this is merely speculation. Future studies could investigate this and whether the difference in exemplar listing is robust by replicating the current study.
Finally, we used semantic networks to visualize the conceptual structure of food types across Italian and English participants. We interpreted the networks by relying on a distinction between sensory and functional properties proposed in previous studies (e.g., Warrington & Shallice, Reference Warrington and Shallice1984). However, analytical approaches vary, and the results can be open to varying interpretations – as is often the case for qualitative studies, as well as quantitative ones (Silberzahn et al., Reference Silberzahn, Uhlmann, Martin, Anselmi, Aust, Awtrey, Bahník, Bai, Bannard, Bonnier, Carlsson, Cheung, Christensen, Clay, Craig, Dalla Rosa, Dam, Evans, Flores Cervantes, Fong and Nosek2018). One possible way to overcome this subjectivity and potential ambiguity would be to separately conduct a study and ask a new sample of Italian and English participants to rate clusters for their sensory or functional properties, thus providing independent confirmation of the interpretation of clusters. This would be an important avenue for any future studies to consider.
5. Conclusion
Taken together, our results suggest being part of different cultural communities leads to a different conceptual representation of food. Not only did we find Italian and English conceptual representation of pasta differed quantitatively – with Italian-speaking participants listing more exemplars for the category of pasta than English-speaking participants – we also found qualitative differences in conceptual organization. In contrast with influential accounts of food concepts that would have predicted the category of pasta to be conceptually organized mainly in terms of functional properties (e.g., Rumiati & Foroni, Reference Rumiati and Foroni2016), we found both Italian and English participants included perceptually based distinctions. However, Italian participants seemed to rely on more subtle distinctions (e.g., ingredient-based distinctions) compared to English participants. This was true even accounting for differences in the level of expertise with pasta across the two groups (see also Medin et al., Reference Medin, Ross, Atran, Burnett and Blok2002, Reference Medin, Ross, Atran, Cox, Wakaua, Coley, Proffitt and Blok2006). In addition, we also found culture-specific patterns in the organization of the two natural categories driven in part by certain sub-categories being recognized in each culture (e.g., “frutta secca” in Italian and “stone fruits” in English) – further illustrating how culture and language carve up conceptual space. These findings add further nuance to the understanding of the conceptual representation of food, and in general, elucidate how semantic knowledge is forged by culture.
Supplementary material
To view supplementary materials for this article, please visit https://doi.org/10.1017/langcog.2023.4.
Data availability statement
All the data and scripts are available on the OSF at https://osf.io/9tkjd/.