12.1 Introduction
Last mile delivery is the movement of products from a warehouse or store to their final delivery points, most commonly residential locations. One of the largest and growing components of last mile delivery operations is parcel delivery. The total volume of the parcel delivery market in the United States was 14.8 billion packages in 2018 [1], with the United Parcel Service (UPS) having the largest market share at 5.2 billion shipped packages [Reference Del Rey2].
E-commerce is a major contributor to the global parcel delivery market and is one of the fastest growing business sectors in the world. Retail e-commerce revenue is expected to rise to $6.5 trillion in the world and to $565 billion in the United States by 2023 [3, 4]. The number of packages shipped increases along with revenue growth. Amazon alone, which accounts for about 49 percent of all e-commerce in the United States [Reference Lunden5], shipped more than 5 billion items to its Prime customers in 2017 [6] and delivered more than 3.5 billion packages through its own delivery network in 2019, almost half of its total number of shipped packages [Reference Del Rey2]. The size of the last mile delivery market increases rapidly as the share of e-commerce in the whole retail industry continues to grow and new contributors to last mile logistics activities, such as food and grocery deliveries, that rely on the same last mile infrastructure emerge. It is thus a vital matter for many businesses to manage last mile operations efficiently.
Last mile delivery related costs are estimated to reach up to 53 percent of total delivery costs [Reference Dolan7]. There are several factors that make last mile delivery operations challenging and more expensive than the other parts of the delivery journey. One factor is that last mile delivery generally takes place within urban areas where the speed limit is lower and there is more traffic congestion. Hence more vehicles are required to deliver parcels by their due time. Furthermore, while multiple and cheaper transportation modes, such as those using railroads and waterways, are usually available for intercity logistics, mostly the only available mode for last mile delivery in an urban area is road transportation.
Meanwhile, the size and number of urban areas also keep growing. By 2030 the number of megacities, which are cities with a population of 10 million or more, is expected to rise to 41 [8]. High population density in cities leads to increased transportation activity and high emissions, as more people and packages travel. In 2017, the transportation sector was the largest contributor to greenhouse gas emissions production by generating 28.9 percent of greenhouse gas emissions in the United States [9]; Figure 12.1 compares sectors with high emissions.
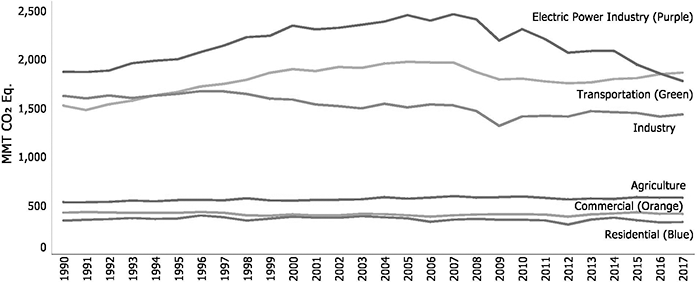
Figure 12.1 U.S. greenhouse gas emissions allocated to economic sectors (MMT CO2 Eq.) [9].
In general, objectives regarding increasing efficiency and reducing negative externalities may contradict each other. Furthermore, unexpected externalities, such as due to changing consumer behavior, may result from policy and/or operational changes leading to outcomes that are the exact opposites of the originally intended ones. For example, traffic congestion and emissions, two negative externalities of last mile delivery, are among the major concerns of city administrations, because they reduce residents’ quality of life. Shared last mile delivery attempts to make the logistics operations in urban areas more efficient and also is thought to have the potential to reduce negative externalities such as emissions by reducing the number of vehicles in traffic and by converting deliveries performed by trucks to crowdsourced cars since cars produce less emissions than trucks. However detailed analysis of some of these sharing-based last mile logistics systems have shown that opposite impacts might occur under certain city and service system characteristics. It is observed that in a subset of last mile logistics systems using crowdsourced drivers increases congestion and emissions because the small carriage capacity of cars ends up increasing the number of vehicles required and the deadheaded distance to deliver all packages [Reference Qi, Li, Liu and Shen10]. Therefore, these systems and the characteristics of the environment they exist in must be analyzed comprehensively and designed and operationalized in a way that creates a win-win-win outcome for businesses, customers, and the society.
There are different applications of crowdsourcing and sharing economy practices in last mile delivery. Since they differ from conventional business models they introduce new system characteristics to be considered in both practice and research. In this chapter, we briefly introduce the existing and potential sharing economy business models in last mile delivery, and discuss the aspects that distinguish them and make their operations more complex to manage.
12.1.1 History of E-Commerce
Home shopping existed before the Internet was widely used by the public. People could buy products via phone calls. They could choose a product they saw in catalogs or TV or newspaper advertisements and order it by calling the number given. In 1994, around 98 million consumers made $60 billion worth of home shopping purchases, and almost all of the purchases was ordered through phone calls [Reference Tuttle11].
The history of e-commerce transactions and online bookstores goes back to as early as 1991 and 1992 when Computer Literacy Bookstores and Book Stacks Unlimited started selling books online through email and their websites [Reference Van Schewick12]. The first item sold online through a secure and encrypted system using a credit card was made possible by Dan Kohn, a 21-year-old entrepreneur. It was the compact disc “Ten Summoners’ Tales” by the rock musician Sting and was sold through the website of Net Market Company of Nashua [Reference Arcand13]. The transaction took place on August 11, 1994, and it was important enough to be featured in the New York Times the next day [Reference Lewis14]. In 1995, Amazon started as an online bookstore [15] and eBay (starting as AuctionWeb) was established as an online auction and shopping website [16].
Since 1995, many online shopping websites have opened. The revenue from e-commerce continues to grow as the number of people who use the Internet grows as shown in Figures 12.2(a) and 12.2(b). The only period e-commerce retail revenue did not grow significantly was 2008–2009 due to the 2008 financial crisis (see the GDP growth in Figure 12.2(c)). It is clear, by comparison of Figures 12.2(a) and 12.2(c), that even a recession could not bring a sustained halt to the growth of e-commerce sales; in fact, in terms of percentage of total US retail, online retail grew during the recession [17].

Figure 12.2 (a) E-commerce retail sales in the United States between 2002 and 2018 [17], (b) percentage of adults who use the Internet in the United States between 2000 and 2018 [18], (c) GDP growth in the United States between 2000 and 2018 [19], and (d) the number of mobile device owners in the United States between 2012 and 2018 [20] (d)
12.1.2 History of Algorithmic Research for Optimizing Last Mile Delivery Operations
One of the earliest challenges of last mile delivery operations was faced by traveling salesmen, as they had to determine the order of visitation of potential customers’ homes or towns, often taking into account the products that they carried and the expected demand at each location. Peddlers have existed for more than 2000 years [Reference Friedman21]. After loading products, such as toiletries, clothing, and accessories, on their pack animals, or in vehicles in recent times, they travelled in one trip to several towns and villages to sell them. Both for the peddlers and the modern travelling salesman, efficiency often is equal to minimizing the cost of travel, which usually incorporates multiple components, such as energy and vehicle depreciation costs that are all a function of the total distance travelled.
The famous Traveling Salesman Problem (TSP) aims to optimize a traveling salesman’s route. The objective of TSP is to find the sequence of customers or towns to be visited which has the minimum travel cost. The sequence must begin and end at the same location and each location must be visited exactly once. The earliest known mention of the problem is in a handbook titled “The Traveling Salesman – how he should be and what he has to do to get orders and be sure of happy success in his business” published in 1832 [Reference Voigt22]. The first known work on the problem by a mathematician goes back to Menger’s work in 1930 [Reference Menger23], after which the attention of many mathematicians was drawn to the problem [Reference Schrijver24], especially after the 1954 seminal work of Dantzig, Fulkerson, and Johnson [Reference Dantzig, Fulkerson and Johnson25], as discussed in [Reference Little, Murty, Sweeney and Karel26–Reference Naddef and Rinaldi31]. Many variations of the problem have also been developed [Reference Gutin and Punnen32]. Nowadays, TSP is a well-studied problem among researchers and continues to attract the energies of both mathematicians and practitioners alike due to its complex and interesting theoretical nature and its applicability in a wide variety of real-world problems. The methods developed to solve TSP are the fundamental methods for solving many combinatorial optimization problems that aim to find the optimal solution in a countable set of solutions.
TSP was later generalized into the Vehicle Routing Problem (VRP), which first appeared in a paper by Dantzig and Ramser in 1959 [Reference Dantzig and Ramser33] and is the most common form of modeling last mile delivery optimization problems. The objective of the VRP is to find the minimum cost assignment of orders to a fleet of homogeneous vehicles and delivery routes for each vehicle starting from the depot and ending at the depot. Since 1959, an immensely rich literature has been established for VRP models and the development of exact, approximation, and heuristic algorithms to solve them (see [Reference Balinski and Quandt34–Reference Solomon41] for some of the early major works).
Many variants of VRP have been introduced over the years (see [Reference Laporte42] for a detailed history) to reflect the changing and complex characteristics of real-world delivery operations. The most important VRP variants are obtained by changing or relaxing some of the assumptions such as considering multiple depots, a heterogeneous fleet of vehicles, allowing both pickup and delivery at the destination locations, adding time-dependent costs, or removing the requirement for a vehicle to return to the depot. Furthermore, new constraints such as delivery (or pickup) time windows and load-balancing for all vehicles can be added to the problem. Finally, one can use different objective functions, such as minimizing fuel costs, minimizing emissions, and a combination of different goals to produce variants of the VRP. Mostly, these new variants of the VRP are proposed and then studied by researchers as a result of novel needs emerging from the markets by the development of new technologies or changing customer requests. For example, time windows, which are the time frame when a pickup or delivery operation must take place, are important in same day delivery; in crowdsourced delivery models, heterogenous vehicles must be considered, as drivers may have different cars; and time-dependent costs are common in urban mobility where the time to travel from one point to another point may drastically change depending on the time of day.
12.2 Sharing Economy Models in Last Mile Delivery
Digital platforms enable the interaction among different participants who supply or demand a set of services or products. Generally, sharing platforms themselves do not offer the services or products, but only match the available supply and demand. Matching as a service has been around for more than three millennia [Reference Evans and Schmalensee43]. However, large scale and rapid matching of supply and demand became available with the pervasiveness of the Internet and smartphones. The Internet enabled the exponential growth of digital platform economy businesses, since it lowered the entry barrier by not requiring a large-scale initial investment in hard assets. Especially after the 2008 financial crisis, a wide variety of online platforms started services such as DoorDash, Uber, TaskRabbit, and AirBnB. The growth of online platforms was also accelerated as smartphones became widespread and the development of mobile technologies and applications accelerated (Figure 12.2d).
Currently in shared last mile delivery, many businesses operate with different sharing models. In the following, we categorize different business models that currently exist or are being discussed as options for the near future.
12.2.1 Crowdsourced Delivery
Crowdsourced delivery platforms do not perform shipments with their own dedicated vehicle fleets or professional drivers. Individual drivers join these platforms as independent contractors, commonly after a security background check, and deliver the packages to customers using their own vehicles. In many platforms, crowdsourced drivers have different levels of flexibility in choosing when to start and stop working.
The landscape of last mile delivery platforms evolved over time. Different platforms, past and present, such as Deliv, GoShare, Instacart, GrubHub, and Postmates, provide last mile delivery using crowdsourced drivers for different types of products such as parcels, groceries, and meals. Furthermore, while some platforms focus on delivery of one type of product, others deliver multiple types. The type of product delivered impacts the constraints of service. For example, some groceries must be carried in cold boxes to prevent spoilage and must be delivered rapidly, while parcel delivery may have a longer delivery time period and can be heavier or bulkier.
Sharing economy delivery platforms also differ in terms of how they match delivery orders and drivers. Some platforms post the delivery orders with origin, destination, and earnings information. Drivers login to these platforms, check the posted orders, and can select the orders that they would like to complete. Other platforms perform the matching themselves given the availability of the drivers and time windows of the orders. They assign the orders to drivers so as to maximize the platform’s profit. Afterwards, drivers can choose to accept the order or refuse it. Furthermore, some of these platforms only make the assignment and leave the delivery sequence up to drivers, while others generate efficient delivery routes and ask drivers to follow these routes. All of these supply–demand matching strategies, complemented with different pricing mechanisms, create digital marketplaces with different characteristics and externalities.
It is commonly believed that crowdsourced drivers provide greater flexibility for delivery companies compared to a dedicated fleet of vehicles, because the driver pool can be adjusted to meet service demand dynamically and with lower costs. However, since crowdsourced drivers are currently not full-time employees, they usually are less stable and predictable, which introduces a high level of uncertainty in workforce and capacity planning. Additionally, crowdsourced driver performance is generally observed to be worse compared to delivery employees of the platform, as the latter have more experience with the business and its customers.Footnote 1 ReputationFootnote 2 mechanisms and other incentives are designed to encourage crowdsourced drivers to display more stable behavior and deliver services with an eye towards higher customer satisfaction.
From an optimization perspective, the crowdsourced delivery problem builds upon the rich body of VRP literature; see [Reference Berbeglia, Cordeau, Gribkovskaia and Laporte44] for an overview. In addition, recent studies have focused on the optimization of crowdsourced delivery systems, the challenge of finding the right balance with respect to the above-mentioned criteria, and the design of appropriate incentives [Reference Arslan, Agatz, Kroon and Zuidwijk45, Reference Archetti, Savelsbergh and Grazia Speranza46–Reference Torres, Gendreau and Rei48]. However, many questions related to market design and comprehensive optimization of crowdsourced delivery platforms, including externalities, still remain open.
12.2.2 Shared Urban Distribution Centers
Urban distribution centers are facilities that are designed and built close to city centers to efficiently handle warehousing operations, such as loading and unloading trucks and sorting packages, as well as cross-docking operations. Their objective is to reduce last mile delivery mileage and fuel consumption and benefit from economies of scale by pooling deliveries into larger vehicles at the urban distribution centers. While it is generally believed that delivery operations using urban distribution centers benefit both delivery companies by reducing their costs and the general public by reducing pollution and traffic congestion, comprehensive analyses of these systems sometimes point to a net negative impact depending on the characteristics of the demand and the urban area.Footnote 3
In operational models that use a shared urban distribution center, larger trucks bring packages from different companies’ warehouses to an urban distribution center. After sorting the packages according to their destinations, packages are loaded to usually smaller vehicles and delivered to customers. At this stage, as an additional benefit of shared distribution centers, orders from multiple companies can be delivered by the same vehicle. Urban distribution centers have multiple docks to load and unload the delivery trucks which reduces the truck queue as the operations can be completed in parallel. Also, these docks complete loading and unloading operations faster than most brick-and-mortar stores, as they are designed for this purpose.
Furthermore, sharing might occur at different levels among companies in the urban distribution centers. They can share only the floor of the building which would increase the space utilization and could reduce the energy consumption, as heating, air conditioning, and lighting could be achieved more efficiently. The participating companies can also share some or all of the operations mentioned earlier. This would further increase the benefits, as the idleness of the shared machinery and equipment would be reduced. Additionally, a collaboration could be formed among the delivery companies, so that the deliveries of different companies could be pooled together, and better routes could be formed in terms of cost efficiency, traffic congestion, and emission production.
On the other hand, convincing e-commerce and/or delivery companies to participate in an urban distribution center is a challenge. The competition among these companies may prevent them from joining a collaborative system. They must be assured that benefits will be greater than costs. Several related problems regarding stable sharing of distribution centers, such as costing, space allocation, and delivery scheduling are discussed in [Reference Quak, Tavasszy, Nunen, Huijbregts and Rietveld49–Reference Cattaruzza, Absi, Feillet and González-Feliu51].
12.2.3 Pickup Lockers
Pickup lockers are secure delivery locations similar to post office (PO) boxes. Generally, however PO boxes are rented for a long time period, whereas lockers are assigned to deliveries for a short time, mostly up to a few days. For this reason, lockers are typically shared by customers. Companies, such as GoLocker, Neopost, and Amazon Locker, currently utilize pickup or product return lockers in their last mile delivery operations.
To use locker delivery service, a customer would choose a pickup location as the delivery address. The delivery company ships the package to this pickup location. When the package is placed into a locker, the customer receives a one-time passcode that is used to open the locker which contains the order. After the customer picks up the packages, the locker is assigned to other deliveries.
Pickup lockers provide benefits for both delivery companies and customers. For customers, a locker station is a secure place to receive their packages in case they do not have a safe area, such as a lobby, in their building. Also, customers do not have to wait at home for delivery since they can pick up their packages from the locker anytime they want. For delivery companies, the locker delivery may reduce costs, since multiple deliveries are expected to be consolidated if their destination is the same locker location. Additionally, drivers avoid delivery problems, such as a wrong address, since the lockers are at known locations.
Pickup locker stations must be designed so that they are an attractive option for consumers. For example, the station must be user friendly and close to areas that customers visit frequently. Additionally, incentivizing customers, for example by providing discounts to encourage consumers to use the service, may increase both the cost and environmental benefits. Designing incentive schemes that consider several factors, such as demand and geographical area characteristics, for each customer dynamically may amplify these benefits. Hence system-wide analysis of all benefits and costs for these shared locker model must be made carefully to inform its design and operations. Comparatively, this area in last mile delivery systems has been studied less completely (see, for example, [Reference Punakivi and Tanskanen52–Reference Ulmer and Streng54]).
12.2.4 Autonomous Vehicles
Autonomous vehicles (AVs) including self-driving cars, automated guided vehicles (AGVs), and unmanned aerial vehicles (drones) may release a big potential in last mile delivery operations. There are several AV brands that are working to produce autonomous vehicles that can follow a given route without human input along the way or be guided by an operator in a semi-automated manner. Currently no AVs are fully autonomous, because such vehicles still require human supervision to prevent accidents when unexpected events occur.
Development and commercial availability of fully autonomous vehicles in the future will open up the possibility for owners of AVs both to make personal use of them and share them with delivery companies. A future scenario could include people going to work with their AVs and then, while they are at work, allowing those AVs to be used by last mile delivery platforms. In a more advanced scenario, AVs could communicate and choose their paths so that traffic congestion could be reduced. Such future sharing of AVs can significantly reduce the idle hours of vehicles sitting in parking lots, while also benefiting the public and environment, as fewer vehicles would be required to achieve both urban mobility and freight transport.
Similar to AV sharing, and in the much nearer future, drones could be shared between their owners and delivery companies. When owners are not using their drones, they can lend them to make deliveries. Currently, one of the limitations of personal drones is their small size, which constrains delivery capacity and allows only small parcels to delivered. Also, personal drones currently have batteries that are much smaller compared to commercial drones, thus reducing their delivery radius. This poses a significant problem especially in cities with tall buildings, where drones cannot fly in a straight line.
Autonomous vehicles can transport packages between locations, but a system to load and unload packages to and from AVs is also required in a last mile logistics operation. An automated system to handle loading to different brands of vehicles, as might be the case in a shared AV system, might be hard to develop in the near future. Additionally, customers might need to be present for delivery, as there will be no one to pick up a package from the AV and deliver it to them. A customer picking up a wrong package can cause significant problems. Therefore, the safest approach currently could be distributing packages one at a time on an AV, which would increase inefficiency and potentially the resulting energy use and pollution of the operations. Incorporating both system optimality and energy usage in operational models may help mitigate the side effects of using autonomous vehicles (see [Reference Levin55].
12.2.5 Public Transportation for Package Delivery
In most cities public transportation network capacities are designed to handle a significant portion of the rush hour commuter needs. However, this causes the public transportation network to have a high idle capacity during long stretches of the day. This idle capacity during low volume hours could be used for last mile delivery, which in turn would reduce the number of vehicles in traffic, especially in the most congested parts of the cities. For example, instead of sending additional vehicles to downtown areas to pick up or deliver packages, last mile delivery operations could utilize the existing public transportation networks in similar ways as described in several studies [Reference Trentini and Mahléné56–Reference Mourad, Puchinger and Van Woensel59]. Synergistic usage of public transport for package delivery could also produce extra revenue for the public transportation authority of an urban area, which in turn can be invested in infrastructure, benefitting the general population.
Public transportation vehicles, such as buses, trams and subways, are designed to carry people and not packages. To use them to deliver packages, operational strategies must be developed and required tools must be deployed at the stops for loading and unloading operations. This would require a high initial installation cost. Furthermore, loading and unloading operations would increase time spent at stops, thereby extending travel times. A small tail car designated for carrying packages that can be automatically and quickly removed and replaced at specific stations is a possibility for carrying packages in and out of highly congested areas using public transportation. Additionally, not all customers might be willing to come to pick up their orders. Therefore, a system to distribute packages from the stops to customers’ houses could complement such a system. An alternative approach, that may be ideal for certain types of customers and packages, could be to combine public transport of packages with delivery lockers at metro stations. This way customers could pick up their packages at their local stations, perhaps at the end of the day on their way home.
12.3 Sharing Economy Platforms vs. Conventional Business Models
Sharing platforms and conventional last mile delivery businesses differ in several ways, including workforce, competition, structure of their corresponding markets, and the externalities that may arise as a result of their operations. In this section we discuss these differences.
12.3.1 Workforce
Conventional delivery companies have dedicated and professional drivers to deliver packages. Since these drivers are traditionally also full-time employees, they have certain benefits which may include health insurance, paid sick leave, and retirement plans. In crowdsourced delivery businesses, generally drivers do not have such benefits, as they are categorized as independent contractors and not as employees, which continues to be a subject of many heated debates and regulatory initiatives from a labor perspective [Reference Conger and Scheiber60].Footnote 4
From an operational perspective, although professional drivers have responsibilities regarding the use of the instruments and vehicles given to them, their employers take care of the cost and planning of insurance and maintenance of these instruments and vehicles. However, crowdsourced drivers have to manage these issues because they use their own equipment and vehicles.
Furthermore, traditional delivery companies have well-established procedures for making deliveries. The decisions on package sorting, loading, and delivery routing are mostly made by specialized professionals or software packages following established procedures. In these traditional business models, drivers have little freedom or flexibility on how to perform these operational processes. Their work shifts may also not be as flexible. In contrast, crowdsourced drivers working as independent contractors are thought to have more freedom of choice on these operational issues. Depending on the platform, they can decide when to work, which deliveries to accept, and how to route their journey. While there appears to be more freedom for an independent contractor, in many situations these drivers’ actions are also restricted through rules or incentive mechanisms. For example, drivers might be held responsible for late or missed deliveries if they occur because of drivers’ actions. In some cases, the flexibilities given to crowdsourced drivers are increasing as platform companies are reacting to recent regulatory efforts and trying to fortify the often-blurred boundary between the definition of a worker and an independent contractor [Reference Paul61].
In traditional delivery companies, the fluctuation in the workforce on a given day is very limited, as drivers must inform their employers in advance if they cannot work on a day and this does not occur frequently. As a result, the delivery capacity on a given day is known in advance, which allows for better operational planning of deliveries. However, crowdsourced drivers may choose not to work and do not even have to inform the company in advance. These conditions create a high degree of uncertainty, which leads to higher operational costs.
12.3.2 Competition
Traditional businesses mostly compete with others in the same business sector. For example, parcel delivery companies compete with other parcel delivery businesses and not with taxi companies. However, crowdsourced last mile delivery companies must compete with crowdsourced urban mobility companies as well as other crowdsourced delivery companies, because drivers can choose to work for any of these businesses as independent contractors. Drivers can check multiple platforms at the same time while sitting in their cars and pick the offer with the best payment and/or work conditions from all the platforms they have joined. Drivers can simply switch from a parcel delivery platform to a ride-sharing platform as soon as they complete an order.
Although payment is an important factor for drivers in choosing among platforms, there are other factors to be considered. A driver might be indifferent to small changes in earnings depending on other features of the platforms. For example, if a driver prefers to socialize while driving, it is more likely that they will choose a mobility platform even though a delivery platform might be offering higher earnings. Level of freedom of choice (orders being assigned by the platform vs ability to choose orders), pressure from the platform (receiving frequent calls/texts to induce fast delivery), and incentives offered by the platform are among possible factors that might impact drivers’ decisions.
The factors that impact drivers’ choice of platform and their performance must be explored. These factors are important as they impact the loyalty of a driver to a platform and their dedication to providing quality service to the customers of the platform. Additionally, incentive schemes for the drivers can be developed. The incentives can be customized for each driver according to their individual preferences. Individualized incentives can improve drivers’ job performance and happiness.
Most platforms do not offer employment benefits to crowdsourced drivers. However, recently Deliv started to offer full time benefits, such as retirement plans and health coverage, to its drivers in California [Reference Said62]. While this decision by Deliv was taken partly in anticipation of CA AB5 regulation [Reference Conger and Scheiber60], Deliv also believed that converting to a full-time employee model might improve service quality and reduce operational costs as well as attract and keep high-performing drivers since offering these benefits provides a bargaining leverage for drivers against other platforms. In a similar recent move, Uber announced that it will consider paying healthcare benefits to workers proportionate to the number of hours they work [Reference Sonnemaker63].
Alternatively, multiple platforms might collaborate instead of competing with each other. For example, a mobility and a delivery platform can collaborate to share their driver pool, and the platform with low demand can transfer some of its drivers to the other one with high demand. However, formation of such collaborations must be regulated and watched carefully in order to prevent the emergence of monopolies or trusts and to ensure drivers are not harmed as a result.
12.3.3 Market Structure
In order to survive in the harshly competitive environment, platforms need to have a certain level of demand and supply in their systems and a continuous dynamic balance between the two must be kept. Dynamic matching of supply and demand in sharing platforms has been a very active research area in recent years [Reference Arslan, Agatz, Kroon and Zuidwijk45, Reference Agatz, Alan, Savelsbergh and Wang64–Reference Masoud and Jayakrishnan66]. For crowdsourced delivery platforms, if there are not enough drivers at any given time, the orders in the systems will end up not being delivered on time or get canceled, increasing the risk of customers switching to another platform. On the other hand, if there are not enough orders in the system, drivers will not have enough work to do resulting in low earnings that incentivize them to switch to another platform.
Combined with the dynamic competition for labor, having to match supply to demand dynamically leads platforms to develop complex pricing policies. Reducing service prices is an effective method for competing for demand. However, this contributes to the major problem of unprofitability experienced by most platform-based businesses [Reference Morozov67, Reference Conger and Griffith68]. Moreover, if the platform lowers prices too much, its drivers might switch to other platforms. This might in turn result in unsatisfied customers who might also leave. On the other hand, surge pricing, in which prices are increased at times of high demand and low supply, could instigate gaming behavior by the drivers (such as turning their apps off to generate “low supply”). Setting the prices to achieve an equilibrium for both supply and demand is a very challenging problem. While the gold standard in two-sided markets is to develop a dynamic pricing policy that balances supply and demand and drives desired customer and driver behaviors, the adaptive behavior of stakeholders and the continuously changing environment make this a moving target. Additionally, in principle, establishing good and clear communication with both sides of the market about the nature of the pricing policies might provide further benefits, as behaviors of customers and drivers can be shifted to a desired setting.
12.3.4 Social and Economic Externalities
Shared delivery platforms have the potential to reduce negative externalities, such as pollution and congestion. They can reduce the number of vehicles operating or the distance travelled. For example, the use of urban distribution centers can reduce distance travelled by increasing truck capacity utilization and integrating public transportation. Similarly, freight transportation networks can reduce the number of vehicles in traffic, especially in congested areas of the city.
However, simple implementations that do not consider all impacts and that lack necessary adjustments to the local needs of urban areas have led shared delivery systems to produce negative externalities in recent years [Reference Ranieri, Digiesi, Silvestri and Roccotelli69]. For example, as discussed previously, crowdsourced delivery cars could be expected to reduce pollution and congestion because they produce less emissions than delivery trucks and delivery trucks are required to return to company parking lots, thereby increasing the distances driven. However, cars are smaller and cannot deliver as many items as trucks. Therefore, more cars are required to complete all deliveries than trucks, and this in turn may produce just as much or more congestion and pollution.
Innovative solutions and strategies are required to reduce the potential negative externalities of shared delivery systems (see [Reference Ranieri, Digiesi, Silvestri and Roccotelli69, Reference Digiesi, Fanti, Mummolo and Silvestri70] for the literature on such strategies). For instance, self-driving cars (that might also be electric) and drones can be used for deliveries (or mobility) when they are not used by their owners. This might reduce the number of vehicles in a city which can lighten the traffic congestion and pollution. However, some people might buy autonomous cars only to produce revenue for themselves. Such a pattern may increase both congestion and pollution in a city.Footnote 5
12.4 Conclusion
Crowdsourcing and shared delivery platforms are among possible solutions which might help to reduce the externalities. They can reduce traffic congestion and pollution by decreasing miles travelled and the number of vehicles in the traffic. Moreover, they can provide additional income opportunities and improve the sense of community. On the other hand, large-scale sharing platforms are relatively new, growing with the speed of internet and mobile technologies. They have many differences with classical business models because they require a level of collaboration of participants to provide benefits for all involved parties. As a result, many questions arise on how to implement and operate these platforms.
To achieve significant benefits from the shared last mile delivery applications for the society, environment, and participating companies, each business model must be analyzed as a system, considering all of its unique set of challenges and impacts. The analyses should take all stakeholders, such as employees, city administrations, and residents, and the interactions among them into account. Additionally, multiple business models should be assessed together. For example, public transportation can be used to bring packages to urban distribution centers from a company’s warehouse, and after the sorting operations, they can be delivered to customers by crowdsourced drivers. Although creating and maintaining such a large collaboration among multiple companies would be very complex, this kind of synergy can amplify the overall benefits in the last mile delivery ecosystem.