Voter turnout is the modal form of political participation in established democracies, and scholars have long paid attention to who votes in elections by comparing turnout between subgroups or by predicting turnout based on background covariates (Tingsten Reference Tingsten1937; Wolfinger and Rosenstone Reference Wolfinger and Rosenstone1980; Rosenstone and Hansen Reference Rosenstone and Hansen1993). We show that different measures of turnout can lead to drastically different conclusions and methodological choices have important implications for the inferences we make about voters and nonvoters.
Broadly speaking, there are two ways to measure turnout at the individual level. Either respondents in a survey are asked to self-report whether they voted, or official voter records are used to validate who voted. A recent meta-analysis by Smets and van Ham (Reference Smets and van Ham2013, 346) shows that within the top 10 political science journals, only 11% of articles employing turnout models used validated data.Footnote 1
Despite the prevalence of surveys in the study of voter turnout, it has long been recognized that surveys overestimate the turnout rate (e.g. Clausen Reference Clausen1968; Traugott and Katosh Reference Traugott and Katosh1979; Silver, Anderson, and Abramson Reference Silver, Anderson and Abramson1986; Granberg and Holmberg Reference Granberg and Holmberg1991; Bernstein, Chadha, and Montjoy Reference Bernstein, Chadha and Montjoy2001; Karp and Brockington Reference Karp and Brockington2005; Jackman and Spahn Reference Jackman and Spahn2014). Two factors contribute to this overestimation. First, nonvoters may be less likely to participate in surveys. We will call this nonresponse bias. Second, survey participants misreport their voting behavior, typically saying that they voted when they did not. We will call this overreporting.
We contribute to the literature on voter turnout by analyzing a rich dataset of Danish voters. The dataset includes validated turnout, self-reported turnout, and background covariates for respondents, as well as validated turnout and background covariates for nonrespondents. Validated turnout and covariate information come from administrative data, and self-reported turnout comes from the Danish National Election Studies (DNES) survey.
We first demonstrate that for Danish voters both nonresponse bias and overreporting contribute to the overestimation of turnout in surveys. We then estimate three predictive models: one with all of the voters in the original sampling frame for whom we have validated turnout; one with validated turnout for only survey respondents; and one with self-reported turnout from the survey. In all three models, we use highly reliable covariates from the administrative data. We compare the models to a baseline model for the population.
The relationship between turnout and age, education, and ethnicity is weaker among the survey participants due to nonresponse bias. It becomes even weaker when we use self-reported turnout due to overreporting among respondents. When we use a self-reported measure of turnout from a survey, as most published research does, both a turnout gap of two and a half percentage points between men and women and of 25 percentage points between native Danes and non-natives disappear. That is, turnout measures that rely on self-reported data may mask important covariate relationships. We conclude that researchers should use validated turnout data when possible. If self-reported voter turnout is the only data available, researchers should use question wordings that reduce overreporting, but also be aware that both nonresponse and overreporting can bias their results.
1 Overreporting and Nonresponse Bias in Surveys
Why could overreporting and nonresponse bias in surveys affect the characterization of voters and nonvoters? First, voters may be more likely than nonvoters to participate in postelection surveys (Katosh and Traugott Reference Katosh and Traugott1981). Thus, responding to the surveys may correlate with propensity to vote. Likewise, once respondents enter surveys, overreporting is not randomly distributed among responding nonvoters. Rather, several studies have shown that overreporting is correlated with predictors of turnout (e.g. Bernstein, Chadha, and Montjoy Reference Bernstein, Chadha and Montjoy2001; Ansolabehere and Hersh Reference Ansolabehere and Hersh2012; Brenner Reference Brenner2012).
Previous research has focused on who overreports, how to reduce overreporting, and the consequences of overreporting. Studies have focused on nonvoters, to account for who is overreporting (Silver, Anderson, and Abramson Reference Silver, Anderson and Abramson1986; Granberg and Holmberg Reference Granberg and Holmberg1991; Brenner Reference Brenner2012), and techniques to reduce overreporting (Abelson, Loftus, and Greenwald Reference Abelson, Loftus, Greenwald and Tanur1992; Morin-Chassé et al. Reference Morin-Chassé, Bol, Stephenson and St-Vincent2017). For instance, Karp and Brockington (Reference Karp and Brockington2005) explore predictors of overreporting across five countries and find that overreporting is positively associated with turnout and positively associated with predictors of voting. Ansolabehere and Hersh (Reference Ansolabehere and Hersh2012) show that using validated turnout leads to different estimates for predictors of turnout compared to using self-reported turnout. Among others, Belli et al. (Reference Belli, Traugott, Young and McGonagle1999), Belli, Moore, and VanHoewyk (Reference Belli, Moore and Vanhoewyk2006), Hanmer, Banks, and White (Reference Hanmer, Banks and White2014) show that paying attention to question wording and response categories can remove some of the bias from overreporting.
Studies have also focused on nonresponse (Swaddle and Heath Reference Swaddle and Heath1989; Sciarini and Goldberg Reference Sciarini and Goldberg2016). Nonresponse typically biases the estimates of turnout, since voters are more inclined to participate in surveys. Sciarini and Goldberg (Reference Sciarini and Goldberg2016) find that nonresponse bias may also lead to misestimation of the predictors of overreporting. This highlights the need to consider not only how overreporting and nonresponse lead to turnout overestimation but also how they may bias the characterization of voters and nonvoters (Ansolabehere and Hersh Reference Ansolabehere and Hersh2012).
2 Data and Estimation Strategy
We combine the DNES from 2015 with Danish administrative data. The 2015 election took place in June and had an overall turnout rate of 85.9%. All Danes have a unique civil registration number, which is linked to administrative data containing a range of background information including age, sex, education, and ethnicity. The DNES sampling frame was drawn from the list of civil registration numbers for all Danish voters. As the civil registration numbers are unique, they provide sufficient information for uniquely matching the entire sampling frame to the administrative data.
Everyone who meets the eligibility requirements is automatically registered to vote, which guarantees that everyone sampled for the DNES was in fact eligible to vote. The voter lists for Danish elections are created based on the administrative data and can therefore also be linked directly to the civil registration number. This means that we can uniquely link turnout to the administrative data for the entire sampling frame, and to survey responses for those who actually took the survey. Because the final voter lists are created approximately one week before each election, there are close to no voters that figure on the lists even though they have died or moved. In 2015, we collaborated with 72 out of 98 municipalities to link turnout for their citizens to the administrative data (Bhatti et al. Reference Bhatti, Dahlgaard, Hansen and Hansen2016).Footnote 2 Where we linked turnout to administrative data, we have a voter record for everyone sampled for the DNES.
The DNES was carried out after the election (Hansen and Stubager Reference Hansen and Stubager2016). It consisted of a probability sample drawn from the civil registration numbers of voters less than 76 years of age with an oversample of young voters. Of the sampled subjects, 3,145 lived in one of the 72 municipalities for which we have validated turnout. The response rate in these municipalities was 52.0%, meaning that 1,635 subjects opted to participate. The DNES records turnout with a multiple-choice question for which one response option was “did not vote”. As both respondents and nonrespondents to the survey were linked to the administrative data, we have validated turnout for the sample, which is free from both nonresponse bias and overreporting. We also have validated turnout for the respondents, which suffers from nonresponse bias but not overreporting. Finally, we have self-reported turnout from the sample, which is subject to both overreporting and nonresponse bias.
First, we show that both nonresponse bias and overreporting contribute to the inflated turnout estimates. To learn about the contribution of self-selection, we compare validated turnout between the 1,510 who did not respond to the survey and the 1,635 who did. To learn about the contribution of overreporting, we compare self-reported turnout with validated turnout among those who participated in the survey.
We rely on a similar strategy to learn whether and how nonresponse bias and overreporting biases the relationship between turnout and background covariates. Based on covariates from the administrative data, we can make three predictions. First, we can predict the probability of voting in the full sample of validated voters. Second, we can predict the probability of voting in the sample of voters who participated in the DNES. Third, we can predict the probability of reporting voting in the DNES. As the first estimate will be based on the entire probability sample, it should fall within the sample variation of the population estimate and will serve as the baseline. The second estimate will suffer from nonresponse bias. Misreporting will further bias the third estimate.
3 Results
To account for the oversampling of young voters, we use inverse probability weights in all analyses for the sample; that is, we weight all observations with one divided by the probability of being sampled. Population results are unweighted. In the first column of Table 1, we show the turnout rate, 86.4%, for the population of voters younger than 76 with validated turnout; the population that the DNES with validated turnout was sampled from. The second column displays the turnout rate, 86.2%, for the entire sampling frame. In the third and fourth columns, we show validated turnout among nonrespondents and respondents. Evidently, the turnout rate is substantially higher due to nonresponse bias; 93.4% among respondents compared to 77.9% among nonrespondents. The proportion of nonvoters is roughly halved from 13.8% in the sampling frame to 6.6% in the sample. Self-reported turnout is even higher, at 96.2%, due to everreporting. Once again, the proportion of nonvoters is almost halved, from 6.6% to 3.8%.
Table 1. Validated and self-reported turnout for DNES sample frame and respondents.

Eleven respondents who answered a few questions but dropped out before the turnout question were omitted and counted as nonrespondents. Thirteen of the respondents answered “don’t know” to the turnout question. Columns 2–4 are weighted to adjust for oversampling of young voters. The population is voters younger than 76 with validated turnout.
The first set of results shows that both self-selection and overreporting among respondents drives up survey estimates of voter turnout. The next question is whether nonresponse and overreporting matter for the substantive conclusions we draw about voter turnout in a predictive model. In Figure 1, we run four models using different covariates to predict turnout. We include as background covariates age in years, education (which is coded as 1 if the respondent has four or more years of education beyond high school), the subject’s sex, and an indicator for being an immigrant or immigrant descendant.Footnote 3 We choose these covariates because are widely used in turnout studies, and we know from previous research that they predict turnout for Danish voters (Smets and van Ham Reference Smets and van Ham2013; Bhatti et al. Reference Bhatti, Dahlgaard, Hansen and Hansen2018).
As a starting point, we predict turnout for everyone for whom we have validated turnout. In the second model, we include everyone who was invited to participate in the DNES, and we estimate the relationship between validated turnout and background covariates. In the third model, we estimate the relationship between validated turnout and background covariates only among those who participated in DNES. Finally, in the fourth model, we use self-reported turnout for the DNES respondents with validated turnout while still using background covariates free of misreporting from the administrative data.Footnote 4 For each model, we estimate a logistic regression and in the figure, we present average marginal predictions.
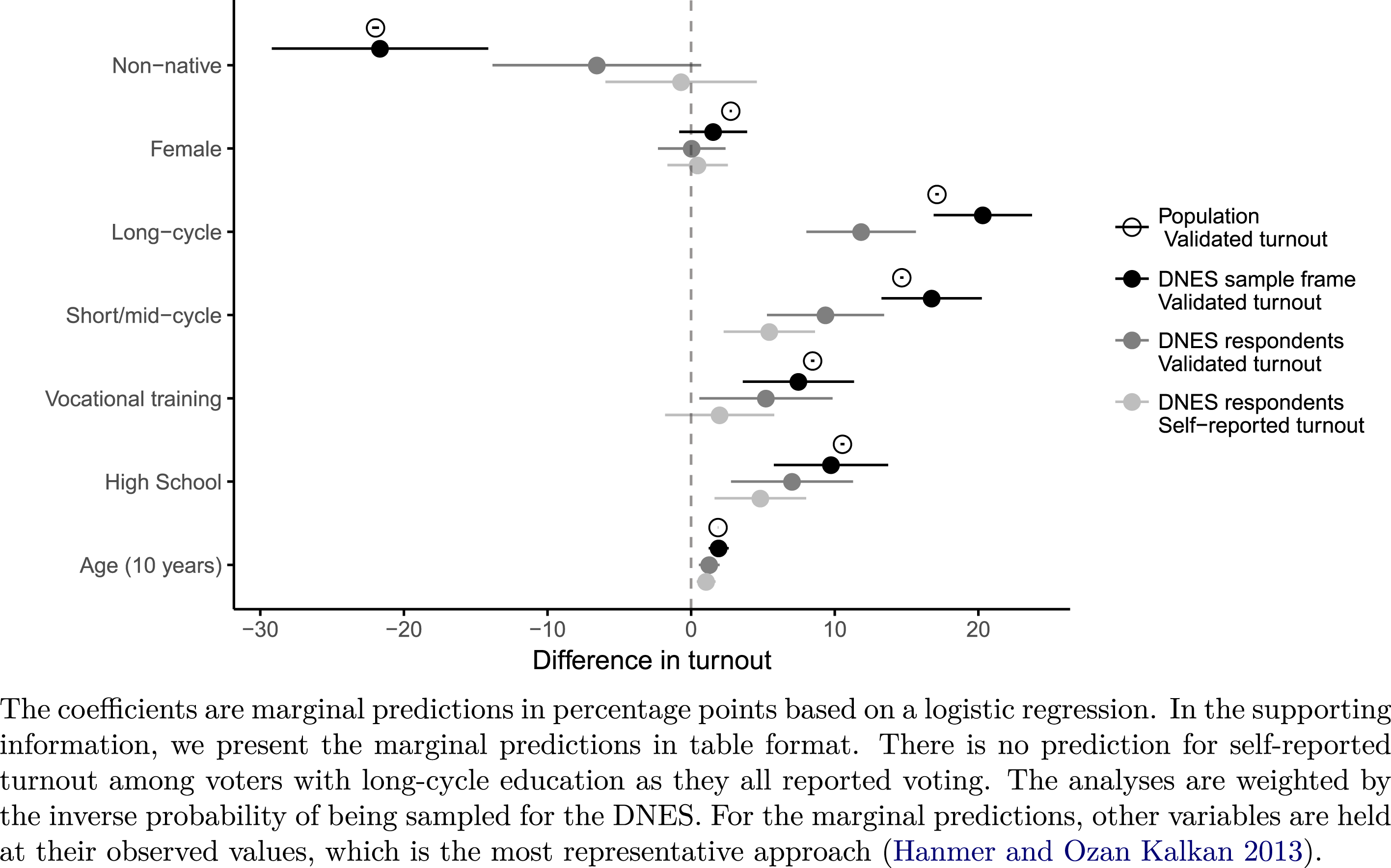
Figure 1. Predicted turnout for population, sample frame, and respondents.
When we look at the actual relationship between the covariates and turnout in 2015, we see that older and better-educated citizens, females, and native Danes were more likely to vote. These findings align with previous research (Smets and van Ham Reference Smets and van Ham2013; Bhatti et al. Reference Bhatti, Dahlgaard, Hansen and Hansen2018). As we would expect from a random sample, the point estimates in the sampling frame model are close to the population model. The population estimates are even included in all 95% confidence intervals for the sampling frame.
In model 3 (dark gray), we consider only validated turnout for the survey respondents, which tells us what happens when we introduce nonresponse bias. We would still believe that older, better educated, and native Danes were more likely to vote. However, the estimates are substantially smaller in magnitude and the difference in turnout between men and women disappears. In the fourth model (light gray), when we use the survey measure of turnout, which is subject to both overreporting and nonresponse, our conclusions become even weaker. Older and better-educated voters are still more likely to report voting, but the magnitude of the difference is now even smaller. The non-native Danes’ turnout is comparable to that of native Danes. A gender difference of more than 2 percentage points has also disappeared.
3.1 Predicting Survey Participation
To provide a clearer response as to why nonresponse biases the correlates of voting, we show in Table 2 how the covariates that we use to predict voter turnout also predict survey participation. In the table, we estimate a model with survey response as the dependent variable for both the entire sampling frame and only the part for which we have validated turnout. If voters are more prone to take the survey, we should see that what predicts turnout also predicts survey participation, effectively leading to truncation on the dependent variable. We see exactly this pattern: age and education are strong predictors of participation, just as they are of voter turnout. Older respondents and respondents with more education are more likely to take the survey. Non-natives are less likely to take the survey. It does not appear that either sex is more likely to take the survey.
We can also compare coefficients from the two models to see whether our results are biased by only some municipalities reporting turnout. The two models provide qualitatively similar results. If the results had differed, it would have caused us to worry how the results were affected by municipalities opting into the study.
Table 2. Predicted probability of survey participation.

The coefficients are marginal predictions in percentage points based on a logistic regression. Standard errors in parenthesis.
3.2 Sensitivity to Missing Turnout
It is worth taking a closer look at whether missing data could have an impact on our conclusions. In the top panel of Table 3, we show weighted descriptive statistics for the survey participants in columns 1–3. In columns 4–6, we show equivalent statistics for all Danish citizen below the age of 76. In the middle panel, we show statistics only for citizens and survey respondents without validated turnout. In the bottom panel, we show descriptive statistics for citizens and survey respondents in all municipalities where we know turnout.
We see that the survey respondents for whom we have validated turnout tend to be younger and more likely to have a high school education or a long-cycle education, while they are less likely to have received vocational training. The variations reflect differences in the populations from which the sample was drawn. When we concentrate on the populations, we see similar differences between the populations in municipalities with validated turnout and the populations in the municipalities without. Importantly, there seems to be no huge discrepancies between the populations of participating and nonparticipating municipalities.
In the supporting information, we also look at the geographic distribution of the nonparticipating municipalities. Furthermore, we run a sensitivity test where we substitute validated turnout in 2015 with validated turnout from a 2013 election where we have full population validated turnout but no polling data. If we assume that self-selection would have been the same in 2013, we can estimate the consequence of nonresponse bias for the entire population in that election and compare it to the consequence among only respondents in participating municipalities. Given this assumption, the consequence of nonresponse bias in 2013 would have been qualitatively the same for the predictive model when using full population data. Finally, we show that a model with self-reported turnout for the entire sample gives similar results as the model using only the validated turnout sample.Footnote 5
Table 3. Descriptive statistics conditional on having validated turnout.

Except for a very small proportion of disenfranchised voters, the population of voters and Danish citizen are almost identical. Only voters younger than 76 was sampled for the DNES.
4 Implications
Our findings suggest that self-reported voter turnout surveys suffer from overreporting and nonresponse, which lead to upwardly biased estimates of turnout. Further, these biases can mask relationships between turnout and key covariates. In our study, using self-reported turnout instead of validated turnout would lead to the incorrect conclusion that men are about as likely to vote as women. Similarly, using self-reported turnout data instead of validated turnout would mask a substantial gap in electoral participation between native Danes and Danes of immigrant background. It goes without saying that the two approaches to measuring carry different policy implications. Survey weights could mitigate the bias created by nonresponse. We bracket that discussion, and simply reiterate that nonresponse and overreporting bias turnout models based on self-reported voting data.
While different question wording and response categories could reduce the amount of overreporting (Belli et al. Reference Belli, Traugott, Young and McGonagle1999; Belli, Moore, and VanHoewyk Reference Belli, Moore and Vanhoewyk2006; Hanmer, Banks, and White Reference Hanmer, Banks and White2014), our paper shows that even using turnout measured with little or no overreporting, for instance validated turnout, can still lead to badly biased conclusions in descriptive turnout studies. Voter turnout is an important and widely studied topic. Our purpose is not to dissuade researchers from doing survey-based turnout studies. We simply point out that researchers should think about ways to acquire high quality data that allows for the replication of established findings that may have been based on survey data, something which is not the norm in published studies (Smets and van Ham Reference Smets and van Ham2013). In addition to thinking about how to measure turnout in a way that is void of overreporting, efforts should also include means to reduce nonresponse bias.
Just as importantly, overreporting and nonresponse bias impact not only the turnout level, but also models predicting turnout. We should not automatically accept null findings if they are based on data that may not have the necessary quality to merit the conclusions drawn. From our point of view, researchers should invest in collecting validated turnout and administrative data as a supplement to traditional survey-based studies.