Introduction
In December 2019, an outbreak of viral pneumonia in China’s Hubei province led to the identification of a novel strain of coronavirus. A small number of cases were soon detected in Thailand, Japan, South Korea, and the United States. In the United States, the accompanying illness, COVID-19, was initially confined to those who had recently traveled to or were in close proximity to those who had traveled through Wuhan, China. Yet attempts to contain the virus over the interceding months failed with cases swelling to the millions and over 100,000 fatalities (Dong and Gardner Reference Dong, Du and Gardner2020).
As cases surged, often sluggish governments transitioned from strategies of containment to mitigation with uncharacteristic haste. Public health officials worried that without considerable intervention, healthcare systems would be overwhelmed by patients. Multiple articles were published in preeminent medical journals discussing triage strategies for allocating limited medical resources, particularly ventilators (Emanuel et al. Reference Emanuel, Persad, Upshur, Thome, Parker, Glickman, Zhang, Boyle, Smith and Phillips2020; Ranney, Griffeth, and Jha Reference Ranney, Griffeth and Jha2020; Truog, Mitchell, and Daley Reference Truog, Mitchell and Daley2020). Hospitals converted parking garages into overflow facilities (Tamburin Reference Tamburin2020), field hospitals were set up in the parks of major cities (Jeffery Reference Jeffery2020), and medical professionals were recruited out of retirement to treat the influx of COVID-19 patients (Einhorn Reference Einhorn2020). Success would no longer be measured in terms of prevention, but in “flattening the curve” to avoid the more severe scenarios for the healthcare system.
At the mass-level, these strategies involved various forms of “social distancing,” a set of nonpharmaceutical interventions (NPIs) aimed at minimizing interpersonal interactions as a means of slowing the spread of infection. To promote social distancing, all 50 states implemented a combination of policies that included limiting the size of social gatherings, enforcing social buffer zones, mandating school closures, reducing retail capacity, closing nonessential business, and requiring facial coverings in public (Fullman et al. Reference Fullman, Bang-Jensen, Amano, Adolph and Wilkerson2020). The most restrictive of these policies, “stay-at-home” or “shelter-in-place” ordinances, aimed to minimize social interactions by ordering residents to stay in their home whenever possible.
Like many aspects of contemporary public life, adoption of these policies took on a noticeably partisan hue. In this paper, I demonstrate how partisan diffusion of state and local government policy responses to the COVID-19 pandemic undermined long-term public health efforts. To support this claim, I highlight three patterns in the adoption of stay-at-home orders. First, using data on state and local COVID-19 responses, I show that states with Democratic governors were more likely to adopt stay-at-home orders independent of how severe the outbreak had become. At the county level, I find similar results after controlling for a battery of county-level demographics, including the prevalence of COVID-19 co-morbidities.
Next, using aggregate data from mobile device geo-tracking, I show that stay-at-home orders caused individuals to reduce their daily average distance traveled, number of visits to retailers, number of encounters with non-household individuals, and increased the their time in residence. Most dramatically, stay-at-home orders reduced the rate at which individuals encountered people outside of their home by 25%. In other words, while social distancing behaviors increased nationwide, stay-at-home orders caused people to stay at home and stay apart in greater numbers. These results run counter to Republican governors’ argument that “constituents didn’t need a mandate to…practice social distancing” (Adler Reference Adler2020).
Finally, I highlight the implications these findings have for COVID-19 public health outcomes. I provide initial evidence demonstrating that more ardent adherence to social distancing behaviors reduced case growth rates at the county level early in the pandemic. Specifically, a one standard deviation increase in social distancing behavior in March reduced COVID-19 growth rates by 86 percentage points in the first 2 weeks of April. These results conform to public health simulations from standard Susceptible-Exposed-Infectious-Recovered (SEIR) epidemiological models, studies of previous viral outbreaks, and contemporary examinations of COVID-19 responses, reinforcing the importance of social distancing.
Together these findings suggest that party politics drove divergent responses to the COVID-19 pandemic and that those different approaches have led and will continue to lead to divergent public health outcomes. In the broadest sense, these findings highlight a critical weakness in the mechanics of responding to a crisis in a federal system and preface the contours of future public health debates. More specifically, these results remind us of the difficulties surrounding policymaking in a hyper-partisan era.
Party Politics and Stay-at-Home Order Diffusion
On March 19, 2020, California Governor Gavin Newsom issued the nation’s first “stay-at-home” order. In order to protect public health, he directed the Public Health Officer to order “all individuals living in the State of California to stay home or at their place of residence” (Newsom Reference Newsom2020). Over the next 2 weeks, 37 states followed suit with four more states issuing strong advisories to the same intent. Arkansas, Iowa, Nebraska, North Dakota, Oklahoma, South Dakota, Utah, and Wyoming never adopted a stay-at-home order.
National public health officials were uniform in their support for a national stay-at-home order (Moreno Reference Moreno2020). As The National Institute of Allergy and Infectious Diseases Director Anthony Fauci responded: “the tension between federally-mandated versus states rights to do what they want is something I don’t want to get into, but if you look at what’s going on in this country I just don’t understand why we’re not [issuing a national stay-at-home order]—we really should be” (Forgey Reference Forgey2020). While other officials were less willing to directly recommend particular policies, all reiterated the importance of staying home and minimizing social interactions.
Yet not all states followed these recommendations. The eight states lacking stay-a-home orders had at least one point in common: Republican governors. Gov. Ricketts (R-NE) called on “people to exercise their freedom to do the right thing, that individual responsibility, that civic duty, to do the right thing” (Adler Reference Adler2020). Some pointed to the redundancy of such orders in the face of massive voluntary social distancing, as Gov. Reynolds (R-IA) did when she noted that “it would be irresponsible for me to just do a statewide [order], when according to Dr. Fauci many of the mitigation efforts that I have put in place are aligned with the results that they’re trying to get” (Nguyen Reference Nguyen2020).
While many aspects of the COVID-19 pandemic are unprecedented, these partisan trends mirror many recent changes in state policy. For example, Callaghan and Jacobs (Reference Callaghan and Jacobs2017) find that states’ early decisions in implementing the Affordable Care Act (ACA) were largely driven by party control. Similar partisan effects are found in state lottery adoptions (Berry and Berry Reference Berry and Berry1990), policy changes to the Children’s Health Insurance Program (Volden Reference Volden2006), youth anti-smoking policies (Shipan and Volden Reference Shipan and Volden2014), and voter ID laws (Hicks et al. Reference Hicks, McKee, Sellers and Smith2015). Despite many unique dynamics at play within each policy, party politics serves as the most consistent and consequential driver of policy adoption.
Two common mechanisms through which policies spread through governments—learning and imitation—are further limited by partisan politics. States are less likely to learn from policy innovations adopted by ideologically or politically dissimilar governments (Grossback, Nicholson-Crotty, and Peterson Reference Grossback, Nicholson-Crotty and Peterson2004). Even when presented with evidence of effectiveness, policymakers are still less likely to seek out information concerning policies adopted by those across the aisle (Butler et al. Reference Butler, Volden, Dynes and Shor2017). While partisanship may generate diverse policy solutions, it may also lead to the dismissal of options due to partisan biases.
Given the unusual nature of a global pandemic and the accelerated time tables under which policies were considered, it is likely that policy imitation influenced gubernatorial support for stay-at-home orders as the effects of these unprecedented policies were largely untested prior to adoption. Here, rather than adopt successful policies observed elsewhere, governments copy policies out of a desire to remain in-sync with other actors. Yet in these polarized times, policymakers are “no longer…looking to do what is popular or widely accepted. Now they are looking to do what is widely accepted within their…ideological community” (Volden Reference Volden2017). These difficulties are highlighted by Grogan, Singer, and Jones (Reference Grogan, Singer and Jones2017) who describe the challenges conservative officials faced when expanding Medicaid under the ACA.
These partisan trends can be directed through elite signaling. With “unrivaled public attention…the president can cue Americans as they assess new policy initiatives” (Callaghan and Jacobs Reference Callaghan and Jacobs2017). For example, Barber and Pope (Reference Barber and Pope2019) find that low-knowledge respondents, strong Republicans, Trump approving respondents, and self-described conservatives were likely to accept cues from President Trump whether the signal was in support of a liberal or conservative policy. At the state level, Governors are able to influence legislators of their own party to help sustain legislative vetoes regardless of the legislator’s ideology (Wilkins and Young Reference Wilkins and Young2002). Some governors made this explicit, as Gov. DeSantis (R-FL) noted he took the “signal” from President Trump on issuing a stay-at-home order (Dartunorro Reference Dartunorro2020). In sum, elite cues can signal to the public and other elected officials whether to support particular policies.
The variation in state policy responses to COVID-19 can be understood in this light. As Democratic governors in Washington and California were forced to respond early in the pandemic, Republican governor’s internalized many of these initial policies as unacceptable Democratic overreach. This dynamic was likely exacerbated by the short time horizons under which governor’s were forced to make decisions, preventing them from considering the policy’s success against its partisan association (Butler et al. Reference Butler, Volden, Dynes and Shor2017). Add the Trump administration’s aversion to a coordinated, national policy response (Samuels Reference Samuels2020) and one is left with a recipe for Republican hesitation.
As the two panels of Fig. 1 demonstrate (Patterson Reference Patterson2021)Footnote 1 , Democratic governors were faster and more likely to adopt stay-at-home mandates compared to their Republican counterparts. But as Gov. Noem noted, “South Dakota is not New York City” (Adler Reference Adler2020). The divergent responses may have been a product of different underlying conditions. To account for the different political and public health environments governors faced, I model the time until governors adopted the policy in relation to state-level characteristics.
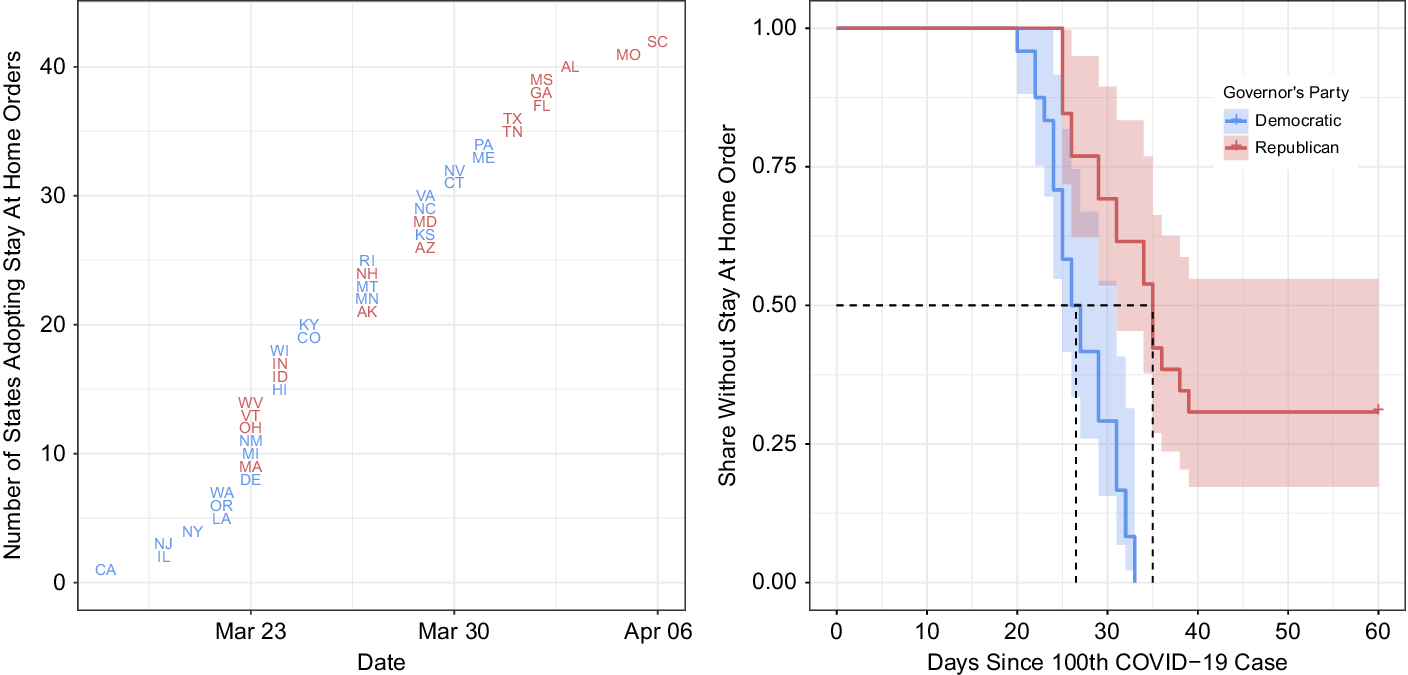
Figure 1. Partisan trends in stay-at-home order adoptions—state level.
Cox proportional-hazards (CPH) models are useful for estimating this relationship as the results account not only for the total number of events, but also the timing of those events. I use a CPH model to estimate how the long governors “survived” pressures to institute stay-at-home orders, measured in days from the first U.S. fatality, February 28th. The models also control for the total number of cases (log scale) on March 28th—the median day on which state’s implemented stay-at-home orders and 1 month since the first fatality—to account for the virus’s trajectory.Footnote 2
To account for both the susceptibility of the population to an outbreak and the demand constituents may place on policymakers, I control for a battery of covariates. First, locations that are more heavily and densely populated with a larger population of seniors are more susceptible to the disease and may adopt stricter regulations sooner. Second, citizens might vary in their support of stay-at-home orders, with the demands for less regulation originating from the bottom-up (e.g., see Pacheco Reference Pacheco2012). Those with college education are more likely to work in industries amenable to working from home, and therefore may be more encouraging of stay-at-home orders. As COVID-19 has disproportionately impacted communities of color (Oppel et al. Reference Oppel, Robert, Rebcca, Will and Smith2020), predominantly white and Republican areas may see less reason for government action. Similarly, those areas with greater unemployment may be less willing to accept the economic hardships stay-at-home orders would require.
The results from this model can be found in Table 1. While states with higher Republican vote share and unemployment were slower to adopt stay-at-home orders, there remains an independent effect for Republican governors across all specifications. In the third specification, I further add regional fixed effects to account for potential spillover effects from neighboring states. Here too the main result is unchanged—Democratic governors were faster and more likely to adopt stay-at-home orders.
Table 1. Governors “surviving” pressure to issue stay at home orders

* p < 0.05.
** p < 0.01.
*** p < 0.001.
But not all stay-at-home orders were issued statewide. For example, while Gov. Tom Wolf (D-PA) held off issuing a statewide order until April 1st, more populous counties like Philadelphia were closed on March 23rd. More commonly, local authorities implemented stay-at-home orders before statewide orders. For example, Blaine County, Idaho sent residents home a week before Gov. Little (R-ID) issued a statewide order. Similarly, while Gov. Lee (R-TN) issued a stay-at-home order on April 2nd, Nashville’s Mayor Cooper issued a stay-at-home order for Davidson County 10 days prior.Footnote 3
Table 2 provides a similar analysis to that in Table 1 estimated at the county-level.Footnote 4 The county-level variation also allows for a more granular measure of mass viral susceptibility. I control for COVID-19 co-morbidities, such as the prevalence of diabetes, lung and heart diseases, and immunological issues.Footnote 5 If policymakers are responding to constituent demands, one would assume that those representing more vulnerable populations would take more aggressive actions. The third specification includes state-level fixed effects. Even allowing for within state variation, counties represented by Republican governors were significantly less likely to have stay-at-home orders.Footnote 6
Table 2. Partisan trends in stay-at-home order adoptions—county level

* p < 0.05.
*** p < 0.001.
Taken together, these results strongly suggest that Democratic governors were faster and more likely to adopt stay-at-home orders and localities in states with Democratic governor’s were similarly more aggressive in adopting these ordinances. However, the question remains whether these ordinances were necessary. In the next section, I test whether stay-at-home orders affected mass behavior.
Stay-At-Home Orders and Social Distancing
Republican governors resisting the calls for stay-at-home orders often portrayed them as unnecessary. As Gov. Parson (R-MO) noted, no “piece of paper is going to tell Missourians exactly what to do…they got enough common sense to realize this is a serious enough thing” (Hancock Reference Hancock2020). In other words, citizens, understanding the severity of the situation, would self-impose social distance and not require a state mandate. This raises the question: Do stay-at-home orders actually affect mass behavior?
Little academic work speaks directly to this question. While stay-at-home or shelter-in-place orders have been previously issued to handle protests, riots, and natural disasters, there are few parallels for such policies aimed at mitigating the transmission of communicable diseases. While a considerable body of research (discussed in “Social Distancing and COVID-19 Outcomes”) estimates the effects of particular social distancing measures on public health outcomes, little work directly address the effect of state mandates on NPI compliance.
However, parallels can be drawn from the literature on vaccination mandates. Both stay-at-home orders and mandatory vaccinations can be seen as government mandates affecting individual compliance with public health measures. And while both usually experience significant levels of voluntary consent, public health benefits are only achieved with near universal compliance (see Du et al. Reference Du, Wang, Pasco, Petty, Fox and Meyers2020; Khabbaz et al. Reference Khabbaz, Robin, Riley, Alexandra and Bell2014). Most U.S. states require a schedule of immunizations to enroll in school and numerous studies have shown that these requirements increase immunization rates (Abrevaya and Mulligan Reference Abrevaya and Mulligan2011; Davis and Gaglia Reference Davis and Gaglia2005; Orenstein and Hinman Reference Orenstein and Hinman1999). Moreover, there is a strong association between the prevalence of vaccine exemptions and resurgences of diseases like measles and pertussis (Constable, Nina, and Caplan Reference Constable, Nina and Caplan2014). These trends are notable given how rarely sanctions are used to enforce these mandates (Weithorn and Reiss Reference Weithorn and Reiss2018). In sum, public health mandates can increase mass compliance, even when individuals stand little legal risk from noncompliance.
Current research suggests that stay-at-home orders have affected individual-level behavior. Alexander and Karger (Reference Alexander and Karger2020) find that stay-at-home orders caused reductions in consumer spending in business sectors associated with mobility, namely small businesses and large retain stores. In a related study, Painter and Qiu (Reference Painter and Qiu2020) find that residents in counties that voted for Hillary Clinton (and thus more likely to be under a stay-at-home order) were more likely to increase social distancing and to transition spending toward more remote services. Both findings suggest that stay-at-home orders have influenced individual behavior.
To address the question of whether stay-at-home orders affected individual propensities to stay at home directly, I rely on geo-location data from individuals’ mobile devices to summarize changes in behavior during the COVID-19 crisis. These data, usually too costly for academics, have been aggregated and made generously available to researchers investigating the pandemic from a variety of private sector sources (as detailed in Appendix A).
The first panel in Fig. 2 shows the relative change in distance traveled by individuals across the 50 states. The second shows the relative change in visits to nonessential businesses. Both figures compare behavior to the average behavior observed in February before community spread was detected in the United States and present the trends for individual states as well as the average of states with Democratic and Republican governors. The third panel shows the relative change in visits to nine different industries as compared to January, whereas the fourth figure shows the relative reduction in visits to these locations in states with Democratic governors compared to those with Republican governors. While dramatic reductions can be seen across the country, more stringent adherence to social distancing can be seen in states with Democratic governors than those with Republican governors.

Figure 2. Partisan trends in social distancing.
While these trends suggest that residents in states with Democratic governors stayed home more so than their counterparts with Republican governors, it is not evidence that the stay-at-home orders themselves are driving this relationship. To test this directly, I estimate the effect of stay-at-home orders on aggregate adherence to social distancing behaviors at the county-level before and after orders were issued.
Conventional analysis of time-series cross-sectional data has relied on two-way fixed-effects models. However, recent methodological advances suggest the interpretation of these coefficients is incorrect, and does not “represent a single estimate of X on Y while accounting for unit-level heterogeneity and time shocks” (Kropko and Kubinec Reference Kropko and Kubinec2020). Therefore, to directly determine if stay-at-home orders influenced staying at home, I rely on the generalized synthetic control (GSC) method developed by Xu (Reference Xu2017), which builds upon the synthetic control approach described by Abadie, Diamond, and Hainmueller (Reference Abadie, Diamond and Hainmueller2010). Unlike the related difference-in-difference analysis, synthetic control methods do not assume parallel trends. The GSC method also has the advantage of estimating a single model with an estimate for the average treatment effect on the treated (ATT) across all the treated units while allowing for differential treatment onset. Finally, it produces traditional inferential statistics for determining uncertainty. This method has been used to assess the impact of election day registration on voter turnout (Xu Reference Xu2017) and the effect of Citizens United on state-level public policy (Gilens, Patterson, and Haines Reference Gilens, Patterson and Haines2020).
The generalized synthetic control approach estimates the impact of a treatment by constructing, for each treated unit (in this case, each county under a stay-at-home order), a synthetic control unit consisting of a weighted average of the control units (i.e., counties without stay-at-home orders). The synthetic units are constructed such that each synthetic control matches as closely as possible the variation over time in the treated unit during the pretreatment period. In this analysis, the synthetic controls are constructed with pretreatment social distancing, COVID-19 cases, and fatalities. The quantity of interest, then, is the difference between the observed outcomes in the treated units during the treatment period (i.e., post-stay-at-home) and the counter-factual predicted values. Specifically, GSCs “imputes counterfactuals for each treated unit using control group information based on a linear interactive fixed effects model that incorporates unit-specific intercepts interacted with time-varying coefficients” (Xu Reference Xu2017, p. 57).
Table 3 presents the results of the GSC analysis for the effect of county-level stay-at-home orders on six social distance outcomes: the relative change in the maximum distance traveled from home (Warren and Skillman Reference Warren and Skillman2020), the relative change in average distance traveled, the relative change in number of nonessential retail services, the rate of unique encounters with nonhousehold members (Unacast 2020), nonessential retail visits (Google 2020), and time spent at home (SafeGraph 2020). All six measures are derived from the geo-location reports from mobile devices aggregated to the county-level. I drop from the analysis any county that lacked seven pretreatment observations, as these observations lack sufficient pretreatment information to construct viable synthetic controls (Xu Reference Xu2017).
Table 3. Effect of stay-at-home orders on social distancing behaviors

* p < 0.05.
** p < 0.01.
*** p < 0.001.
It is important to note that the treatment—the issuing of a stay-at-home order—is but one policy option deployed by state governments during the pandemic. States also implemented a combination of large gathering prohibitions, mandated school closures, restaurant and bar restrictions, and nonessential business closures. For example, every state had effective closed primary and secondary schools prior to adopting stay-at-home orders. Yet these orders were the most restrictive option in the policy toolbox. Therefore, while other less restrictive policies may contribute to the effect, these results can be conservatively viewed as the effect of adopting the most restrictive social distancing regime.
Across all six metrics, stay-at-home orders have caused an independent increase in social distancing behavior. People stayed closer to home, interacted with fewer people outside of their home, visited nonessential businesses less often, and spent more time inside their home. These findings suggest that Republican governors’ assertions that residents did not need a stay-at-home order to make the socially responsible decision was incomplete. Instead, they suggest that stay-at-home orders can increase adherence to social distancing behavior beyond the sizable voluntary shift in mass behavior.
Thus far, I have demonstrated that Democratic governors were more likely to adopt stay-at-home orders and that these orders had an independent effect on mass behavior. The final question then is whether adherence to social distancing policies improves public health outcomes. In other words, does staying apart help slow the spread of COVID-19?
Social Distancing and COVID-19 Outcomes
Epidemiologists and public health officials appear in uniform agreement that failing to maintain social distancing policies will lead to significantly more COVID-19 cases and deaths. A research team at the University of Texas reports that social distance compliance rates as high as 75% may still not be sufficient to prevent some Texas cities from experiencing unmanageable surges in COVID-19 hospitalizations (Du et al. Reference Du, Wang, Pasco, Petty, Fox and Meyers2020). The Institute for Health Metrics and Evaluation (IHME) model, frequently cited by the White House, has updated their projections to include greater fatalities because of “premature relaxing of social distancing” policies (Miranda-Ollstein and Oprysko Reference Miranda-Ollstein and Oprysko2020). The COVID-19 simulator, developed by researchers at Massachusetts General Hospital and Georgia Tech, shows total cumulative cases nearly doubling if social distancing policies are relaxed in the short term (Morrison Reference Morrison2020). Governors attempting to re-open their states understand the politics behind these findings. Gov. Ducey (R-AZ) ordered his health department to “shut down the work of academic experts predicting the peak of the state’s coronavirus outbreak was still about two weeks away” (Stanley-Becker and Weiner Reference Stanley-Becker and Weiner2020) as he re-opened nonessential businesses.
Results from history also highlight the importance of social distancing in dealing with viral outbreaks. Simulations based off of the spread of H5N1 influenza through Western Australia similarly stress the importance of social distancing (Kelso, Milne, and Kelly Reference Kelso, Milne and Kelly2009). Glass et al. (Reference Glass, Glass, Beyeler and Min2006) find that school closures and work reductions decreased the attack rate of the 1957–1958 Asian flu by greater than 90%. Much of the research on the 1918 Flu Pandemic suggests that nonpharmaceutial interventions helped reduce peak mortality rates—flattening the curve—and cumulative mortality rates (Bootsma and Ferguson Reference Bootsma and Ferguson2007; Markel et al. Reference Markel, Lipman, Navarro, Sloan, Michaelsen, Stern and Cetron2007; Yu et al. Reference Yu, Lin, Chiu and He2017). A working paper from the Federal Reserve studying the effects of nonpharmaceutical interventions (NPIs) on the economic recovery of cities following the 1918 Flu Pandemic shows “that NPIs not only lower mortality; they also mitigate the adverse economic consequences of a pandemic” (Correia, Luck, and Verner Reference Correia, Luck and Verner2020).
By reducing interpersonal interactions, social distancing policies should improve public health outcomes. To demonstrate this relationship, I estimate the relationship between increasing social distancing behaviors on county-level growth rates in COVID-19 cases and fatalities. To measure compliance with social distancing, I use the overall social distancing scores provided by Unacast (2020), an index of distance traveled, nonessential retail visits, and close encounters with members outside of the household. This score ranges from 1 to 5, with 5 representing a greater overall adherence to social distancing. In practice, no county experienced perfect compliance, with this measure ranging from 1.08 to 4.3, with a standard deviation of 0.76. This score is provided each day for each county. I average the score across the month of March to determine a county’s overall social distance compliance.
The main dependent variables are the percent change in total positive COVID-19 cases between April 1st and April 14th and the change in total fatalities between April 1st and April 28th.Footnote 7 I use a 2-week window for cases as this provides sufficient time to determine who has contracted the disease and will become contagious to other people (Locker-Maragakis Reference Locker-Maragakis2020). Using observed cases as a dependent variable may raise some concerns as to the testing capacity of these localities. As most testing regimes are run by state agencies, the state-level fixed effects should account for these limitations. I estimate the effect of social distancing behavior on the percent change in total fatalities, as fatality data, while likely still under-reported (Weinberger et al. Reference Weinberger, Jenny, Ted, Forrest, Farzad, Don, Virginia, Reich Nicholas, Marcus, Lone, Anne and Viboud2020), is less subject to the variability in testing ability. I use a 4-week window for fatalities as case-fatality studies suggest a 2-week median from diagnosis to death (Wilson et al. Reference Wilson, Amanda, Lucy and Baker2020).
The results of this analysis are presented in Table 4 and show a nearly 86 percentage point reduction in the growth rate for a one standard deviation (0.74) increase in social distancing compliance, which is a considerable effect considering the average growth rate was approximately 225%. Both specifications control for the log of total cases on April 1st. The second specification also includes the battery of covariates from Table 2. Similarly, after 1 month, counties that practiced greater social distancing in March saw a similar reduction in the growth of fatalities over the month of April.
Table 4. Social distancing and COVID-19 outcomes

Standard errors clustered by state.
*** p < 0.001.
While confined to the early stages of the pandemic, these preliminary results match results from other scholars. Thakkar et al. (Reference Thakkar, Burnstein, Hu, Selvaraj and Klein2020) found that in King County, Washington, the effective reproduction number of new infections—an important metric in epidemiology—decreased from about 2.7 in late February to roughly 1.4 in mid-March as a result of social distancing. San Francisco, an early adopter of stay-at-home policies, had a significantly “flatter” curve than other comparable metropolitan areas (Hwang and Massa Reference Hwang and Massa2020). Research from the Center for Disease Control and Prevention (CDC) has found that where “social distancing interventions were in place, 20% of new cases and most hospitalizations and deaths were adverted, even with modest reductions in contact among adults” (Matrajt and Leung Reference Matrajt and Leung2020). In a working paper, Fowler et al. (Reference Fowler, Hill, Levin and Obradovich2020) also find a direct effect of stay-at-home orders on reductions in county-level COVID-19 growth rates. These results, combined with the initial results I present here, provide strong evidence that adherence to NPIs improves public health outcomes in a pandemic.
Discussion
In this paper, I have shown that Democratic governors were faster to adopt stay-at-home orders. This is important because stay-at-home orders cause residents to adhere more strictly to social distancing, behaviors that in turn help mitigate the spread and severity of COVID-19. In this section, I discuss the implications of these findings in terms of future policymaking in a hyper-partisan federal system.
Federalism in a Crisis
The variation in state responses to COVID-19 serves as a reminder of federalism’s double-edged nature. On the one hand, the discretion to act independently allowed states and localities to serve as policy laboratories where competing versions of public policy could be compared and a diverse array of solutions tested over equally diverse areas. A one-size-fits-all policy may not be the best strategy to mitigate COVID-19 in a nation as vast and diverse as the United States, especially when one considers economic impact of many of these policies.Footnote 8
Federalism also provides a backstop against an inability or unwillingness to act at the federal level. Partisan divisions have driven legislative productivity to historic lows. Even when policy changes come from Washington, the gears of power are notoriously slow. For example, nearly 20 million Americans were still waiting for emergency relief payments, the most straightforward implementation in the federal COVID-19 response bill, 2 months after becoming law (Picchi Reference Picchi2020). And given the Trump administration’s expressed hostility toward and desire to quickly “liberate” states from stay-at-home orders, the discretion to act independently allowed localities to overcome federal resistance and place over 90% of the population under stay-at-home orders during peak implementation (Silverstein Reference Silverstein2020).
On the other hand, the lack of a national, coordinated strategy likely resulted in a less efficient, less effective response effort. For example, in responding to shortages in medical supplies, the state-by-state strategy has lead to inter-state competition which has dramatically driven up prices through a bidding war (Estes Reference Estes2020). In response to Gov. Cuomo’s (D-NY) plan to construct a “nationwide buying consortium,” some pejoratively and satirically suggested that perhaps the 50 governors should form a country (Borowitz Reference Borowitz2020). In terms of stay-at-home orders, (Fowler et al. Reference Fowler, Hill, Levin and Obradovich2020) estimate that a national directive “might have reduced by hundreds of thousands the current number of infections and by tens of thousands the total number of deaths from COVID-19.” National coordination could have helped overcome many of the collective action limitations inherent in pandemic response, limited the growth in cases, and most importantly, saved lives.
As governors begin reopening state economies, the state-level discretion sets up the potential for spillover effects across porous state borders. If two neighboring states adopt different mitigation strategies and these interventions cause variation in individual compliance (as shown above), noncomplying residents may simply cross state lines to avoid regulation. The potential for “cross-contamination” along state borders could induce a race to the bottom, where individual states would find little reward to the high costs of restrictive policies.
For example, Gov. Beshear (D-KY) asked Kentuckians living on the border to “not go to Tennessee for anything other than work or helping a loved one or maybe the grocery…if you ultimately go down over that border and go to a restaurant or something that’s not open in Kentucky, what you do is you bring the coronavirus back here in Kentucky” (Tobin Reference Tobin2020). Gov. Raimondo (D-RI) went one step further, requiring all out-of-state visitors to stop at information centers and detail their plans to self-quarantine if staying in Rhode Island (Cuoco Reference Cuoco2020). To help reduce the uncertainty, regional collectives of governors have sought to coordinate strategies for reopening the economy, but they have proven difficult to enforce when preferences diverge (Gronewold Reference Gronewold2020).
In sum, the diverse state and local government responses to the COVID-19 pandemic highlight the strengths and weaknesses of responding to a crisis in a federal system. With the discretion to act on their own, states and counties used a combination of business and school closures, gathering limitations, travel restrictions, quarantine procedures, and emergency medical contingencies to mitigate the virus in their jurisdiction. It is unlikely that any such efforts would have emerged from a resistant Trump administration, and as demonstrated above, these policies helped slow the spread of the virus and save lives. But lacking a coordinated national response, states created a patchwork of inconsistent policies undermined by variation across state lines.
Parties and Pandemics
Why did Democratic and Republican officials respond differently to the COVID-19 pandemic? At first glance, the question may appear naive: In an era of deep polarization and visceral partisan conflict, nearly all public discourse is divided along party lines. From this view, it should come as no surprise that wearing masks in public (Roberts Reference Roberts2020), staying home (Beauchamp and Animashaun Reference Beauchamp and Animashaun2020), and faith in the CDC (Feldman Reference Feldman2020) are becoming new fronts in an evolving culture war.
While these findings paint a very partisan picture, it may be that Republican governors simply represented populations who were less supportive of stay-at-home orders and were merely following public opinion. As Fig. 3, demonstrates, however, this simply was not the case in the initial stages of the pandemic. The COVID-19 Consortium for Understanding the Public’s Policy Preferences Across States (Lazer et al. Reference Lazer, Baum, Ognyanova and Volpe2020) conducted a survey to gauge public preferences on COVID-19 responses. The survey sampled 22,912 respondents across all 50 states from an online, nonprobability sample, with state-level representative quotas for race, ethnicity, age, and gender. The first panel reports the share of respondents reporting that their state government was not taking the outbreak seriously enough in relation to their approval of the governor’s handling of COVID-19. In comparison, panel 2 plots the share feeling that their state government was overreacting to the crisis. The pattern is stark. The public is generally more supportive of governors seen as actively addressing the pandemic (see Clement and Balz Reference Clement and Balz2020).

Figure 3. Public reaction to COVID-19 responses.
Note. Survey was conducted by The COVID-19 Consortium for Understanding the Public’s Policy Preferences Across States (Lazer et al. Reference Lazer, Baum, Ognyanova and Volpe2020) between April 17 and 26, 2020.
What was even more stark was the widespread support for government interventions to slow the spread of COVID-19. Nationally 94% of respondents reported somewhat or strongly approving of governments asking residents to stay at home, and in no state did fewer than 80% agree. In fact, of the eight policiesFootnote 9 under consideration, only tracking cellphones lacked greater than 60% approval across any of the 50 states. Another poll conducted in mid-April similarly found that 89% of Democrats and 72% of Republicans agree that strong social distancing should continue, and large majorities of both groups say it is more important to stop the virus than to resume economic activity (Shepard Reference Shepard2020). While Republicans have become less supportive of social distancing policies, majorities in both political parties responded in support continued state NPIs.
In sum, Republican governors’ reticence to adopt stay-at-home orders is likely driven not by public opinion, but by a top-down, elite driven process. As seen in Fig. 4, when President Trump dismissed the virus as a “hoax,” Republicans dismissed the risks surrounding COVID-19. When he declared a national emergency, signed a 2 trillion dollar stimulus bill, and predicted over 100,000 fatalities, the threat became significantly more concerning for his co-partisans. When it became apparent that a quick return to normalcy was unlikely, Trump began touting “miracle cures” and ignoring CDC guidelines, which in turn reassured the party faithful. While by no means definitive, taken together with the surveys above and the significant work on public response to the pandemic (Allcott et al. Reference Allcott, Levi, Jacob, Matthew, Michael and Yang2020; Gadarian Reference Gadarian2020), it is at least suggestive that Republican voters are following their leaders on this crisis.

Figure 4. Republican perceptions of COVID-19 pandemic.
Note. Survey was conducted daily by Civiqs (2020) between February 25 and July 1, 2020.
Why Democratic and Republican officials then pursued different strategies is beyond the scope of this paper. However, the evidence at least suggests that these divisions were driven by elite preferences, and led and will continue to lead to divergent public health outcomes. Whether policymakers are considering public face coverings, or perhaps mandating vaccination, partisanship will continue to shape the diffusion of mitigation strategies. While this is likely unsurprising to even the most cursory observer of American politics, it serves as an important reminder that while partisanship may be a “helluva drug” (Reference KleinKlein 2016), like most addictions, it too can be fatal.
Supplementary Materials
To view supplementary material for this article, please visit http://dx.doi.org/10.1017/spq.2020.5.
Data Availability Statement
Replication materials are available on SPPQ Dataverse at https://doi.org/10.15139/S3/4QUN5B.
Acknowledgments
The author would like to thank Josh Clinton, Spencer Hall, Eunji Kim, Sara Kirshbaum, Alex Lawhorne, Meredith McLain, Mary Catherine Sullivan, and CSDI’s American Politics reading group for feedback and encouragement. Special thanks go to Mellissa Meisels who read and revised multiple drafts.
Funding Statement
The authors received no financial support for the research, authorship, and/or publication of this article.
Conflict of Interest
The authors declared no potential conflicts of interest with respect to the research, authorship, and/or publication of this article.
Appendix A: Data sources
The large table below provides the sources of all data used in this paper’s analyses. Almost all variables in the above analyses come directly from these sources, but four I construct myself. Their calculations are described below.
-
• COVID-19 comorbidity: While a definitive understanding of COVID-19 is still a work-in-progress, older patients suffering from cancer, chronic lung diseases, diabetes, liver failure, and asthma experience worse clinical outcomes. Geographically, the presence of these covariates is highly correlated. My measure of susceptibility for COVID-19 is the first principal component of county-level co-morbidity prevalence (rates of cancer, heart disease, and chronic lung disease morality; smoking, diabetes, alcoholism, and sedentary lifestyle prevalence; average number of sick days; share of residents reporting “poor health”). This variable explains over 60% of the variance, compared to the second which explains less than 10%. Age appears orthogonal to health metrics, and is therefore included as a separate variable.
-
• Relative visits by industry: SafeGraph’s Weekly Places provides the total number of visitors to particular points of interests on a daily basis. To describe the state-level trends in Fig. 3, I aggregate the individual location data to the state-NAICS-day-level for all of 2020. NAICS codes provide a five-digit categorization of industry for particular businesses and points of interest. I then divide each date’s total number of visits to a particular industry by the average number of visits to that industry in January to highlight relative changes in behavior.
-
• COVID-19 case growth rate: The growth rate over the course of the first 2 weeks of April was measured as:

-
• COVID-19 fatality growth rate: The growth rate over the course of the first 4 weeks of April was measured as:

-
• Social distance grade: The social distance grade is the average Unacast social distance score over the month of March. Some smaller counties have some missing days of observations because of insufficient sample size. In these instances it is the average of the available observations in March.

Appendix B: Specification chart
To alleviate concerns that the results presented in Table 2 are driven by a particular model specification, below I present an iterative specification chart. The main model presented in Table 2 is highlighted in red. Each additional point is the estimated effect of a Republican governor under a model specification including the gray highlighted variables. The only specification decision that significantly affects the results is the inclusion of state-level fixed effects. However, under all specifications the effect is statistically and substantively significant at any convention.

Figure B1. Specification chart for Table 2.
Appendix C: Alternative measure of case prevalence
As an alternative measure of case prevalence, Appendix C uses the number of days between a counties first and fiftieth case to account for speed and trajectory of the local outbreak. Specifications (2) and (3) control for all of the covariates from Table 2, and the specification (3) includes state-level fixed effects. While the effect in the full specification is substantially smaller than other specifications, the direction of the effect remains consistent.
Table C1. Partisan trends in stay-at-home order adoptions—county level
