Introduction
The COVID-19 pandemic has not only dramatically changed the world we are living in but also exacerbated health inequality among different groups of people that has been covered up by the economic prosperity that we have achieved in the past several decades (Takian et al., Reference Takian, Kiani and Khanjankhani2020). Under such circumstances, the development of telehealth, eHealth or mHealth, which, simply speaking, refers to ‘health care carried out at a distance’ with the help of technologies, especially the internet (Wootton, Reference Wootton1995), is believed to be an important tool to provide solutions to the health inequality that is partially caused by the unevenly distributed resources of health care (Hussain et al., Reference Hussain, Wenbi, da Silva, Nadher and Mudhish2015; Vancea and Solé-Casals, Reference Vancea and Solé-Casals2016).
The recent development of medical applications best exemplifies this. With the worldwide proliferation of smartphones, there has been a dramatic increase in medical and health-care mobile applications. According to a commercial report, there were more than 165,000 apps developed by 45,000 app publishers in 2015, the total market value of which was estimated to be US $10.2 billion (Research2Guidance, 2015: 3). However, it is noteworthy that, despite a steady increase in internet use among older people, the digital gap remains between older adults and other age groups in the world, especially in non-Western countries (Cole et al., 2017: 23, 2018: 19).
This perfectly demonstrates the importance of technology in the so-called Fourth Industrial Revolution gradually taking shape in the past two decades. This emerging trend is characterised by a fusion of technologies blurring the lines between the physical, digital and biological spheres (Schwab, Reference Schwab2016: 12). A combination of the internet and traditional industries constitutes one of the main features of the Fourth Industrial Revolution, under which the internet is growing rapidly as an industry and reshaping all the traditional industries (Shao, Reference Shao2015: 225–256). This trend has been further intensified under the COVID-19 pandemic. However, the advancements of the internet and its incorporation into the traditional industries do not necessarily challenge the multiple forms of digital inequalities. Instead, people with limited knowledge of and/or access to the internet will be further marginalised in the new age. Among these different groups, older people deserve our special attention.
Ageing is a problem faced by almost every country in the world, which, for developed and developing countries alike, is believed to have profound influences on and bring tremendous challenges for a country's economic progress, social welfare system and medical system (Díaz-Giménezab and Díaz-Saavedrac, Reference Díaz-Giménezab and Díaz-Saavedrac2009; Manson and Lee, Reference Manson, Lee, Lee and Manson2011; Rudnicka et al., Reference Rudnicka, Napierala, Podfigurna, Meczekalski, Smolarczyk and Grymowicz2020; Nagarajan et al., Reference Nagarajan, Teixeira and Silva2021). However, the older people with the greatest health needs also tend to be those with the least access to the resources that might help them, which has posed great challenges for policy makers and service providers (World Health Organization, 2017: 3). This is especially true in the context of the COVID-19 pandemic. While existing literature illustrates how the pandemic motivates different countries to focus on the potential of telehealth (Fisk et al., Reference Fisk, Livingstone and Pit2020), we still know little about the willingness of older adults to use medical apps during the COVID-19 pandemic.
For several reasons, an investigation of the Chinese case provides fertile ground for studying older people's intention to use medical apps during the COVID-19 pandemic. First, according to the World Health Organization, China is ageing much faster than many other high-income or low- and middle- income countries. The official statistics show that there were 253.8 million people aged 60 years or over (18.1% of the whole population) in 2019, which is expected to increase to 402 million (28% of the entire population) in 2040. One major concern about ageing is the associated increase in the burden of chronic disease. In 2013, of the 202 million older people aged 60 or over in China, more than 100 million had at least one chronic non-communicable disease. By 2030, there will be three times more people living with at least one chronic disease (World Health Organization, 2015: 1–4). As one of the fastest ageing countries with one of the largest aged populations in the world, China constitutes a good starting point to examine technology use among the older population in an ageing context.
Second, the rise of an ageing society has emerged concurrently with the popularity of internet use in China (Guo et al., Reference Guo, Sun, Wang, Peng and Yan2019). The number of Chinese netizens reached 989 million in December 2020, with a penetration rate of 70.4 per cent. If it keeps growing at this rate, the number of Chinese netizens will exceed 1 billion in the first half of 2021, accounting for 69.4 per cent of the total population (Beijing Youth Daily, 2021). Although senior citizens have become an important part of the internet consumer population in China, only 20 per cent of them have access to the internet. Basic operations, such as downloading and using apps, are still one of the biggest obstacles to using mobile devices for them (Tencent, 2018). When encountering difficulties in online shopping, 50 per cent of them will give up (People's Daily Online, 2020). For most of them, the internet is a new thing that only appeared when they entered middle or old age. Therefore, learning to use the internet is challenging for them (Chen, Reference Chen2017), especially when seeking online medical services (Zhou et al., Reference Zhou, Liang and Ma2021). The tension between China's fast-ageing population and the reluctance of the Chinese older population to use the internet for health services makes China an ideal case to investigate older people's willingness to use medical apps in a context where the population is rapidly ageing.
Third, the pandemic outbreak has increased older people's exposure to the internet and their demand for it. Statistics have shown that the proportion of older users who registered and used the food-ordering website Elema increased significantly. The number of older adults who began to use the internet during this period also far exceeded the number in other age groups (People's Daily Online, 2020). Moreover, the pandemic encouraged them to use medical apps instead of in-person services (Zhou et al., Reference Zhou, Liang and Ma2021), especially during and after the Chinese Spring Festival (from late January to February 2020), when people were forcibly quarantined at home. As such, an examination of Chinese older people's intention to use medical apps during this period serves our research purpose perfectly.
Building on the research background presented above, this research demonstrates older people's willingness to use medical apps during the COVID-19 pandemic in China. Following an analysis of 1,318 questionnaires distributed online in the early stages of the pandemic (February 2020), we combined the Technology of Acceptance Model (TAM; Davis, Reference Davis1989) and the Unified Theory of Acceptance and Use of Technology model (UTAUT model; Venkatesh et al., Reference Venkatesh, Morris, Davis and Davis2003) in our analysis to better capture the participants' intention to use medical apps with the users' attitudes as the important mediator. We then tested this model during the COVID-19 pandemic. We believe our findings also have implications for meeting older people's needs in the Fourth Industrial Revolution, partially characterised by the incorporating information technology into the traditional industries (Schwab, Reference Schwab2016).
Literature review and hypotheses development
Usage of medical apps among older people
Previous studies employed multiple research methods in investigating senior citizens' intention to use medical apps. Some studies adopted qualitative methods, such as focus group discussions (Parker et al., Reference Parker, Jessel, Richardson and Reid2013) or semi-structured interviews (Byambasuren et al., Reference Byambasuren, Beller, Hoffmann and Glasziou2020) to understand the older people's willingness to use medical apps. For example, Parker et al. (Reference Parker, Jessel, Richardson and Reid2013) found that older people in New York City showed great interest in using medical apps. In this research, facilitators include professional training before using devices and tailoring services to the needs of older adults, and the barriers are the cost and a lack of familiarity with the devices. Similarly, Byambasuren et al. (Reference Byambasuren, Beller, Hoffmann and Glasziou2020) found that, despite the ubiquity of electronic devices and doctors' recommendations of apps, older adults were still concerned about the usability of medical apps.
Others studies employed quantitative methods, such as structural equation models (Guo et al., Reference Guo, Keane, Ellis and Xu2013; Hoque and Sorwar, Reference Hoque and Sorwar2017; Lan et al., Reference Lan, Liu and Yang2020) and regressions (Askari et al., Reference Askari, Klaver, Van Gestel and Van de Klundert2020) to identify the factors that may influence older people's choices. Based on different theories and models (e.g. UTAUT or UTAUT2 and trust transfer models), factors such as ease of use, usefulness, social influence, facilitating condition, social trust and technological anxiety have been tested. However, there is also inconsistency in the research findings. For example, in the research of Askari et al. (Reference Askari, Klaver, Van Gestel and Van de Klundert2020), facilitating conditions significantly impacted the older people's adoption intentions, but not others (Hoque and Sorwar, Reference Hoque and Sorwar2017; Lan et al., Reference Lan, Liu and Yang2020).
Despite the contributions these studies have made to our understanding of older people's needs in the development of eHealth, our knowledge about the potential mechanism contributing to their adoption intention is still in its infancy. Further, we still know little about how these identified factors work during irregular circumstances like the COVID-19 outbreak, which juxtaposes a forced spatial restriction and a relative lack of medical resources. This article fills this gap by investigating the situation in China during the pandemic.
Theoretical framework
We developed our theoretical framework based on the TAM (Davis, Reference Davis1989) and UTAUT model (Venkatesh et al., Reference Venkatesh, Morris, Davis and Davis2003). The UTAUT model is an integrated model consisting of eight theories commonly utilised in behavioural research and user technological acceptance research. It has been widely applied in various cultural settings, such as North America (Serbern, Reference Serbern2014), South America (Thomas et al., Reference Thomas, Thakur, Jackman, Thomas, Gajraj and Allen2014), Europe (Nuq and Aubert, Reference Nuq and Aubert2013), Asia (Isaac et al., Reference Isaac, Abdullah, Aldholay and Ameen2019), sub-Saharan Africa (Karuri et al., Reference Karuri, Waiganjo and Manya2013), and the Middle East and North Africa (Nassuora, Reference Nassuora2013), and in diverse technological contexts, such as mobile payment (Khalilzadeh et al., Reference Khalilzadeh, Ozturk and Bilgihan2017), mobile learning (Ye et al., Reference Ye, Zheng and Yi2020), social media (He et al., Reference He, Zhang and Zeng2020) and electronic medical applications (Hwang et al., Reference Hwang, Dutta and Chang2019) since its release.
Although the UTAUT model excels at explaining users' intention to use information technology, it has been criticised for its lack of attention to users' attitudes to the technology, such as customer satisfaction (Isaac et al., Reference Isaac, Abdullah, Aldholay and Ameen2019). As Montesdioca and Macada (Reference Montesdioca and Macada2015), among others, have argued, users' satisfaction, or the so-called performance effect, has played a significant role in the success of the Information System. Following this, this research aims to add users' satisfaction to the original UTAUT model to analyse the older adults' intention to use medical apps during the COVID-19 pandemic in China, which, as can be seen from the following discussion, requires a combination of the UTAUT model and the TAM.
TAM postulates that (a) people's intention to use new technology is dependent upon their attitudes and (b) their attitudes towards new technology are influenced by technology's perceived usefulness and perceived ease of use (Davis, Reference Davis1989). Users' attitudes towards certain technology (e.g. satisfaction) refers to the psychological state of users' emotional responses, such as favourable or unfavourable responses, when their previous expectations towards certain technology are confirmed or not (Davis, Reference Davis1989). Behaviour intention refers to the belief that an individual will perform a certain behaviour in reality (Ajzen and Fishbein, Reference Ajzen, Fishbein, Albarracin, Johnson and Zanna2005). Perceived usefulness refers to the extent to which users find a particular technology helpful in improving their work and life (Davis, Reference Davis1989). Perceived ease of use refers to the extent to which users believe that adopting a particular technology is effortless (Davis, Reference Davis1989). According to TAM, we proposed the following three hypotheses:
-
H1: Attitudes towards use are positively related to intention to use.
-
H2: Perceived usefulness is positively related to attitudes to using.
-
H3: Perceived ease of use is positively related to attitudes to using.
The variables shown in the original TAM model mainly focus on the features of the technology itself (Davis, Reference Davis1989). Recent efforts have also been made to investigate variables on social (e.g. subjective norm; SN) and organisational (e.g. facilitating conditions; FC) (Agustina and Indriati, Reference Agustina and Indriati2018) levels to explain user satisfaction with certain technology. SN refers to ‘the extent to which an individual perceives that significant others believe he or she should use the new system’ (Venkatesh et al., Reference Venkatesh, Morris, Davis and Davis2003: 450). According to the Expectation-confirmation Theory, people tend to form a positive attitude if significant others, such as family members (Cheng et al., Reference Cheng2008), friends (Lian, Reference Lian2015), co-workers (Brown et al., Reference Brown, Venkatesh and Hoehle2015) and experts (Cheng, Reference Cheng, Liu, Qian and Song2011), also have similar feelings (Agustina and Indriati, Reference Agustina and Indriati2018; Ma et al., Reference Ma, Ruangkanjanases and Chen2019).
FC refers to ‘the degree to which an individual believes that an organisation and technical infrastructure exist to support the use of the system’ (Venkatesh et al., Reference Venkatesh, Morris, Davis and Davis2003: 453). Facilitators include the resources (hardware, software and training knowledge) (Lian, Reference Lian2015), technical assistance (Nistor et al., Reference Nistor, Lerche, Weinberger, Ceobanu and Heymann2014) and compatibility with other technologies (Nistor et al., Reference Nistor, Lerche, Weinberger, Ceobanu and Heymann2014). People with these facilitators tend to be satisfied with a certain product (Agustina and Indriati, Reference Agustina and Indriati2018). Following this logic, we postulated the following hypotheses:
-
H4: The subjective norm is positively related to attitudes to using.
-
H5: Facilitating conditions are positively related to attitudes to using.
There are four antecedents of the UTAUT model: performance expectancy (e.g. perceived usefulness), effort expectancy (e.g. perceived ease of use), social influences (e.g. subjective norm) and facilitating conditions. During this epidemic, even the older people who, more often than not, suffer from chronic diseases are not allowed to leave home to see a doctor as usual. Thus, in this case, the older adults' intention to use online medical services is dependent on the app's usefulness, ease of use (de Veer et al., Reference de Veer, Peeters, Brabers, Schellevis, Rademakers and Francke2015; Hoque and Sorwar, Reference Hoque and Sorwar2017) and how significant others (Alam et al., Reference Alam, Hoque, Hu and Barua2020), such as doctors, peers, particularly those who suffer from similar diseases and family members (Or and Karsh, Reference Or and Karsh2009), influence them, and whether they have better technological knowledge and skills, and devices that promote their use (Yuan et al., Reference Yuan, Ma, Kanthawala and Peng2015). Thus, based on the UTAUT model, the following hypotheses were proposed:
-
H6: Perceived usefulness is positively related to intention to use.
-
H7: Perceived ease of use is positively related to intention to use.
-
H8: The subjective norm is positively related to intention to use.
-
H9: Facilitating conditions are positively related to intention to use.
Based on the discussions above, we proposed the research framework shown in Figure 1.
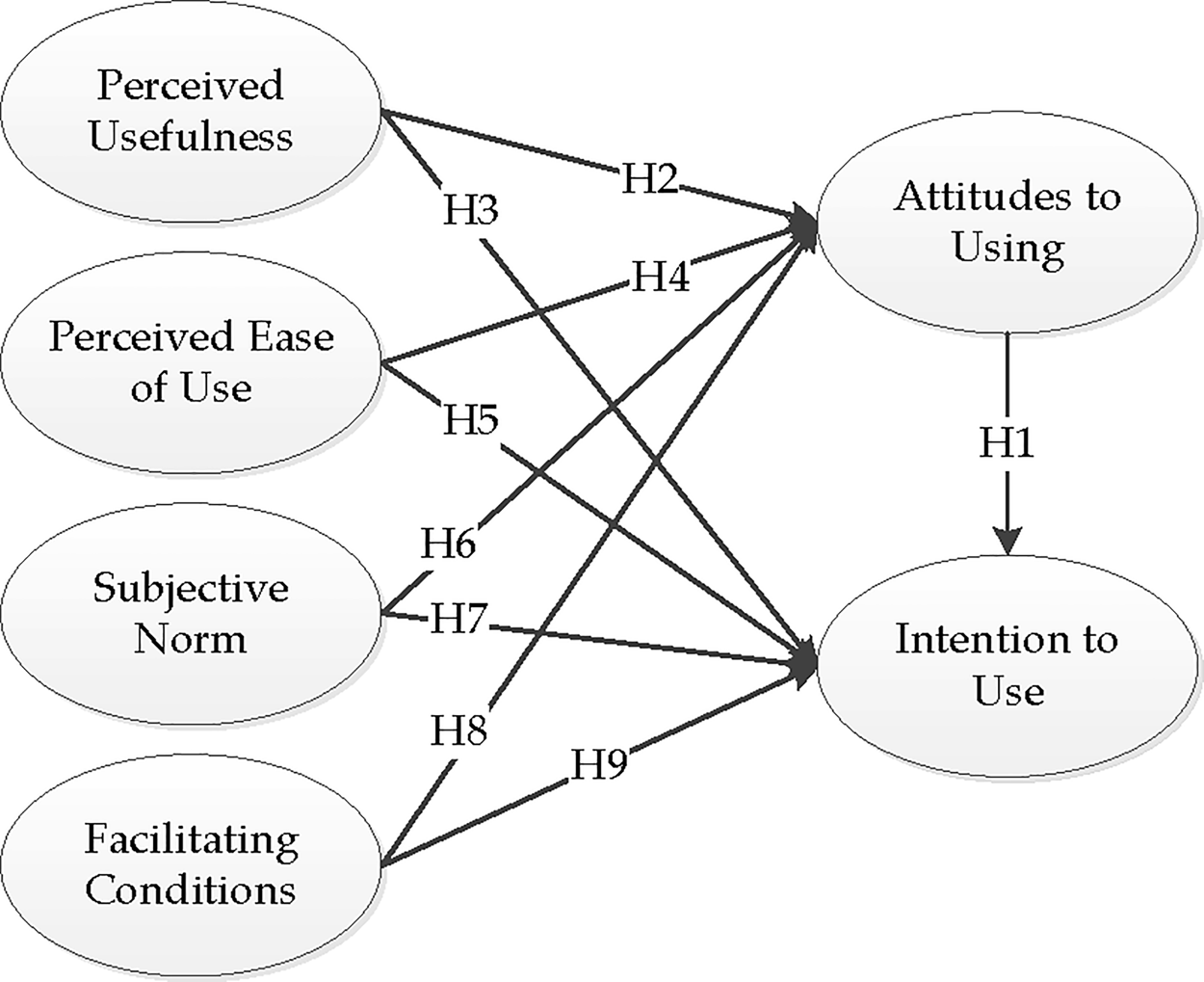
Figure 1. Research framework.
Note: H: hypothesis.
Methodology
Sample
China closed off the city of Wuhan on 23 January 2020, to stop the spread of the deadly virus due to the impact of the COVID-19 pandemic. Other areas of China subsequently started the first-level response to address this public health emergency. Several measures, such as grid management, were implemented immediately to minimise contacts among people, and all outdoor activities were called off. People were called on to stay at home for at least 14 days.
Our data were collected during this period, from 26 January to the end of February 2020. We commissioned a local professional data survey company to help us collect the data. They first approached community workers, told them the research purpose and gave them online questionnaire, which could be accessed by scanning a QR code. In other words, the community was the intermediary institution, helping us to distribute research information to the older people aged 60 or above (Han et al., Reference Han, He, Lyu, Yu, Bian and Lee2020). It took participants approximately 30 minutes to finish the online survey. Those who completed the survey and successfully submitted it were rewarded with CNY20 (approximately US $3). The survey was completed by 1,318 participants and their data were used for further analysis. All respondents were informed in advance that the information would be confidential and used for research purposes only. They were also told that they could withdraw from the study at any time without giving a reason.
At the beginning of the questionnaire, we described the following scenario to them: an increasing number of health apps, such as Chunyu Yisheng (Dr Chun Yu), Xunyi Wenyao (seeking medical advice), Dingxiang Yisheng (Dr Clove), appear. There are thousands of certified doctors on these medical apps and they can provide one-to-one online consulting services to the public. At the same time, potential patients can also check others' consultation experiences and obtain relevant medical knowledge. All in all, people can consult professional doctors without leaving home. This is of great significance when all outdoor activities are cancelled. Thus, we seek information about the informants' willingness to turn to these medical apps for professional medical services under these circumstances.
Among the 1,318 participants, 71.5 per cent were men and 28.5 per cent were women; 73.4 per cent of the respondents were from urban China and 26.6 per cent came from rural China. The average age of our respondents is 63.98 years (range = 60–75; standard deviation = 3.243). It should be noted that the technical knowledge of our respondents may be better than the average level among older adults in China because of the electronic survey we employed. Although older people are important online consumers in China, only 20 per cent of them can surf the internet, and basic operations such as downloading and using applications are still their biggest obstacles (Tencent, 2018). It is one of our limitations.
Measures
All variables were measured with a seven-point Likert scale, from 1 = strongly disagree to 7 = strongly agree.
Perceived usefulness
Perceived usefulness was measured with five items from previous studies (Adams et al., Reference Adams, Nelson and Todd1992; Straub et al., Reference Straub, Keil and Brenner1997; Moon and Kim, Reference Moon and Kim2001; van der Heijden, Reference van der Heijden2003). The sample item for the scale is ‘I find it convenient to use medical apps’; reliability is 0.906.
Perceived ease of use
Perceived ease of use was measured with four items from the previous work (Adams et al., Reference Adams, Nelson and Todd1992; Straub et al., Reference Straub, Keil and Brenner1997; Moon and Kim, Reference Moon and Kim2001; van der Heijden, Reference van der Heijden2003). The sample item for this construct is ‘I think it easy to use medical apps’; Cronbach's α is 0.9.
The subjective norm
Five items from previous work (Davis, Reference Davis1989; Moore and Benbasat, Reference Moore and Benbasat1991; Venkatesh et al., Reference Venkatesh, Morris, Davis and Davis2003) were employed to measure the subjective norm. The sample item for this scale is ‘Basically, people around me want me to use medical apps' services’; reliability is 0.914.
Facilitating conditions
Four items from previous studies (Ajzen, Reference Ajzen1991; Compeau et al., Reference Compeau, Higgins and Huff1999) were utilised to measure the facilitating conditions. The sample item is ‘I have the necessary resources, such as mobile phones, to use medical apps’; reliability is 0.862.
Attitudes to using
Four items from previous studies (Adams et al., Reference Adams, Nelson and Todd1992; Straub et al., Reference Straub, Keil and Brenner1997; Moon and Kim, Reference Moon and Kim2001; van der Heijden, Reference van der Heijden2003) were employed to measure this construct. The sample item for this scale is ‘I like to use medical apps’; reliability is 0.913.
Intention to use
Four items from previous work (Adams et al., Reference Adams, Nelson and Todd1992; Straub et al., Reference Straub, Keil and Brenner1997; Moon and Kim, Reference Moon and Kim2001; van der Heijden, Reference van der Heijden2003) were utilised to measure intention to use. The sample item for this dimension is ‘I would like to recommend other older people to use medical apps’; Cronbach's α is 0.918.
Data analysis
Structural equation modelling (SEM) allows a detailed analysis of the complex interrelationships between observed and latent variables. Partial least squares SEM (PLS-SEM) is a particularly well-known method used to estimate these relations, which has been widely used in informational sciences (Hair et al., Reference Hair, Hult, Ringle and Sarstedt2017a), psychology and business (Sarstedt et al., Reference Sarstedt, Ringle, Cheah, Ting, Moisescu and Radomir2020), etc. Compared to covariance-based SEM (CB-SEM), in PLS-SEM, the variance-based technique relaxes some research assumptions. It enables researchers to estimate complex models with many observed variables, latent variables and structural paths without imposing distributional assumptions on the data (Hair et al., Reference Hair, Risher, Sarstedt and Ringle2019a). More importantly, it emphasises the predictive function of the model, the basis for proposing managerial implications, rather than the explanatory function that is usually emphasised in previous studies (Hair et al., Reference Hair, Sarstedt and Ringle2019b). When the analysis involves testing a theoretical framework from the predictive perspective, PLS-SEM is highly recommended (Hair et al., Reference Hair, Risher, Sarstedt and Ringle2019a). Based on the UTAUT and TAM theory, our research is very interested in the predictive power of the integrated model, that is, whether the older adults had the intention to employ medical apps during the epidemic. Therefore, we performed the PLS-SEM analysis with the SmartPLS (v.3.2.9) software (Ringle et al., Reference Ringle, Wende and Becker2015).
Ethical concerns
Despite the absence of official ethical review institutions in social sciences in most universities in mainland China, we take research ethics very seriously in our research process. First, we used an anonymous online survey and deliberately avoided asking any questions that included identifiable information to protect our participants' privacy. Second, we had a consent letter at the beginning of our questionnaire that clearly stated the information about this research project, the researchers and their rights as research participants. In this letter, we specifically mentioned that they had the right to stop participating at any time and that there would be no any adverse consequences if they chose to do so. Finally, all the data collected are kept and used only by the researchers and for academic purposes.
Results
The two-step approach recommended by scholars was employed to perform the analysis (Anderson and Gerbing, Reference Anderson and Gerbing1988). First, the measurement model was estimated, which involved the examination of indicator loadings, internal consistency reliability, convergent validity and discriminant validity (Hair et al., Reference Hair, Risher, Sarstedt and Ringle2019a). After the measurement models met the criteria requirements, the structural model was evaluated (Hair et al., Reference Hair, Ringle and Sarstedt2017b), which included the assessment of the coefficient of determination (R 2), the blindfolding-based cross-validated redundancy (Q 2) and the path coefficients and significance test. The study also performed a bootstrapping mediation evaluation to understand the mediation effect of attitudes to using apps.
Measurement model
The standard for the item reliability was met if the factor loadings were above 0.708 (Hair et al., Reference Hair, Risher, Sarstedt and Ringle2019a). Composite reliability was used to assess internal consistency reliability, and values more than 0.95 were undesirable (Hair et al., Reference Hair, Risher, Sarstedt and Ringle2019a). The convergent validity was evaluated by the average variance extracted (AVE). The minimum acceptable value of AVE was 0.50, which meant that the construct could explain more than 50 per cent of the items' variance (Hair et al., Reference Hair, Risher, Sarstedt and Ringle2019a). Finally, the discriminant validity was assessed (Fornell and Larcker, Reference Fornell and Larcker1981). According to Fornell and Larcker (Reference Fornell and Larcker1981), if the square root of AVE was more than the correlations of the constructs, the discriminant standards could be met.
In our data, the composite reliability was less than 0.95, and AVE was above 0.50 (see Table 1), indicating the standards for internal consistency reliability and convergent validity could be met. The minimum square root of the AVE was 0.884, which was larger than the maximum correlation of 0.827 (see Table 2), indicating that the discriminant validity met the requirements.
Table 1. Reliability and average variance extracted (AVE) of the measurement model
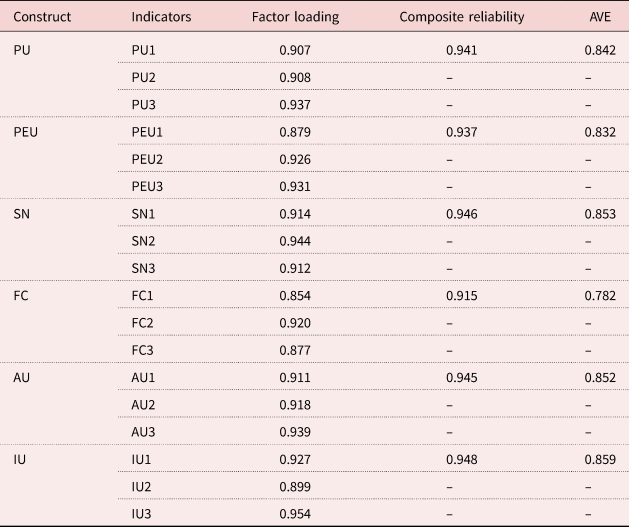
Notes: PU: perceived usefulness. PEU: perceived ease of use. SN: subjective norm. FC: facilitating conditions. AU: attitudes to using. IU: intention to use.
Table 2. Fornell–Larcker criterion for discriminant validity
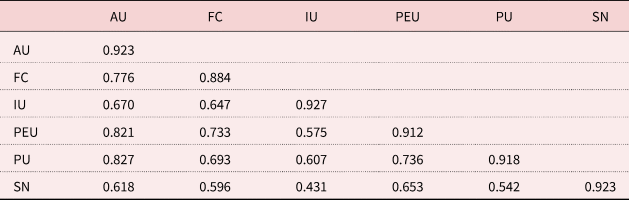
Notes: Diagonals (bold) represent the square root of the average variance extracted while the other entries represent the correlations. PU: perceived usefulness. PEU: perceived ease of use. SN: subjective norm. FC: facilitating conditions. AU: attitudes to using. IU: intention to use.
Structural model
The structural model was evaluated by R 2, Q 2, path coefficients and a significance test. R 2 showed the percentage of explanatory power for the endogenous constructs, and it represented the predictive power of the model (see Figure 2). R 2 thresholds of 0.75, 0.50 and 0.25 were seen as substantial, moderate and weak (Hair et al., Reference Hair, Hollingsworth, Randolph and Chong2011). In our data, the predictive power of the perceived usefulness, perceived ease of use, the subjective norm and the facilitating conditions of the attitudes towards using apps was substantial (0.807 > 0.75), and the predictive power of the intention to use apps was weak to moderate (0.492).
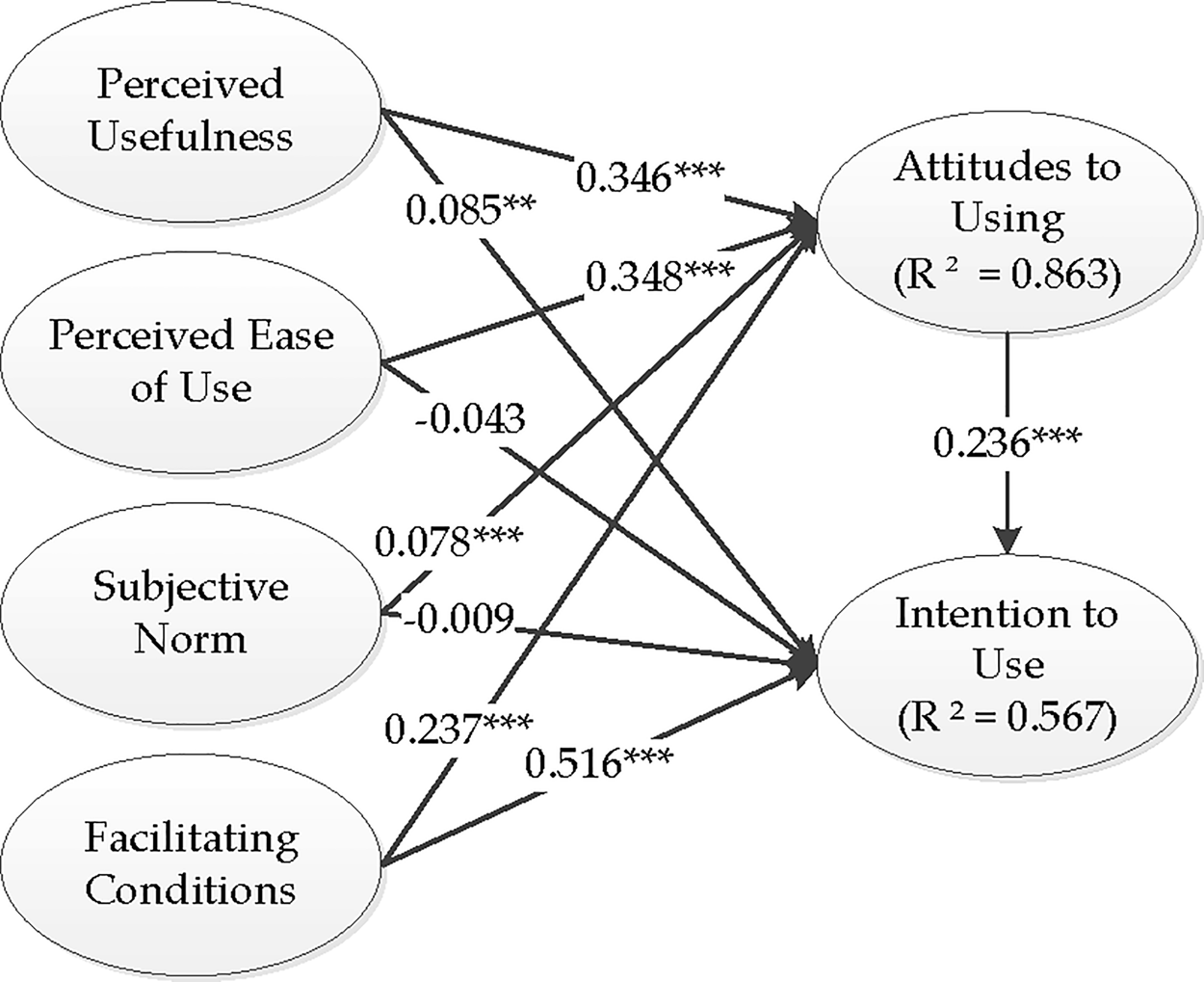
Figure 2. Path coefficients and R 2-values of the structural model.
Significance levels: ** p < 0.01, *** p < 0.001.
A Q 2-value larger than zero for a specific endogenous construct indicated the predictive accuracy of the structural model for that construct. According to Hair et al. (Reference Hair, Risher, Sarstedt and Ringle2019a), Q 2 larger than 0, 0.25 and 0.50 indicated the small, medium and large predictive relevance of the model. In our data, the Q 2 of attitudes towards using apps was 0.683, indicating the large predictive power of the model. The Q 2 of intention to use apps was 0.416, indicating the medium to large predictive power of the model.
Path coefficients showed the significance and directions of the relations proposed in the model. Bootstrapping was employed to evaluate the path's significance (Hair et al., Reference Hair, Hollingsworth, Randolph and Chong2011). Figure 2 and Table 3 summarise the results of the significance test. Two hypotheses were not supported: H7 (PEU → IU) and H8 (SN → IU). H7 showed that perceived ease of use could not predict older people's intention to use apps (β = −0.025, t = 0.518), and H8 demonstrated that the subjective norm was not significantly related to the intention to use apps (β = −0.033, t = 0.929).
Table 3. Summary of structural model results

Notes: Number of bootstrap samples = 10,000. PU: perceived usefulness. PEU: perceived ease of use. SN: subjective norm. FC: facilitating conditions. AU: attitudes to using. IU: intention to use.
Significance levels: * p < 0.05, ** p < 0.01, *** p < 0.001.
The rest of the hypotheses were supported. H1 showed that attitudes towards using apps positively and significantly predicted the intention to use apps (β = 0.360, t = 6.794), which meant that those satisfied with the medical apps intended to use them. H2 demonstrated that perceived usefulness was positively and significantly associated with attitudes towards using apps (β = 0.402, t = 15.390), showing that people would be satisfied with the medical apps if they thought they were useful. H3 showed that perceived ease of use was positively and significantly related to attitudes towards using apps (β = 0.323, t = 10.690), indicating that people would be pleased with medical apps if they thought apps were easy to use. The subjective norm had a positive and significant association with attitudes to using; thus, H4 was supported (β = 0.053, t = 2.543). It suggested that others easily influenced older people, and because of this, they tended to use medical apps if other people spoke highly of these apps.
H5 showed that facilitating conditions significantly impacted the attitudes to using apps in a positive manner (β = 0.229, t = 9.002). It showed that people would be pleased with medical apps if they had access to related devices, such as the internet and mobile phones. Perceived usefulness positively impacted the intention to use medical apps (β = 0.124, t = 3.441), supporting H6. It meant that people tended to ask for help with medical apps if they thought these apps were useful to them. H9 illustrated that facilitating conditions positively and significantly impacted intention to use medical apps (β = 0.319, t = 7.631). It showed that senior citizens were likely to use medical apps if they had related facilities, such as mobile phones.
Mediation analysis
A bootstrapped 95 per cent confidence interval was generated to examine the mediation effects. The literature (MacKinnon et al., Reference MacKinnon, Lockwood and Williams2004) indicates that indirect effects can be confirmed if zero is not included in the confidence interval. Table 4 shows the results, including the original sample, the sample mean, the standard deviation, and the t-statistics and p-values. The findings illustrated that the attitudes towards using medical apps mediated all the paths, which identified satisfaction as the important mechanism, linking perceived usefulness, perceived ease of use, subjective norm and facilitating conditions with people's intention to use medical apps. The current study's findings suggest that senior citizens' intention to use medical apps is conditioned by their attitudes even if the attributes of apps are excellent (e.g. useful and easy to use), and the surrounding environments (e.g. internet and other people's influence) are well prepared. In other words, senior citizens' ‘attitudes’ to medical technologies is of great importance in the apps utilisation process.
Table 4. Mediation effect

Notes: Number of bootstrap samples = 10,000. PU: perceived usefulness. PEU: perceived ease of use. SN: subjective norm. FC: facilitating conditions. AU: attitudes to using. IU: intention to use.
Significance levels: * p < 0.05, *** p < 0.001.
Conclusion and discussion
We constructed a research model based on TAM and UTAUT models and used SEM to examine older people's intention to use medical apps for information and services during the COVID-19 pandemic in China. In line with previous studies, our empirical findings provide insight into the UTAUT constructs, such as how the perceived usefulness and facilitating conditions influence the adoption of medical apps (Hoque and Sorwar, Reference Hoque and Sorwar2017; Byambasuren et al., Reference Byambasuren, Beller, Hoffmann and Glasziou2020; Lan et al., Reference Lan, Liu and Yang2020). Also consistent with previous work is the finding that attitudes towards medical apps are also a significant antecedent of behaviour intention (Askari et al., Reference Askari, Klaver, Van Gestel and Van de Klundert2020; Byambasuren et al., Reference Byambasuren, Beller, Hoffmann and Glasziou2020).
However, the remaining two factors in the original UTAUT model, perceived ease of use and subjective norm, did not predict users' intentions. Past research has shown that when a system is mandatory, perceived ease of use is not significantly related to intention to use (Venkatesh et al., Reference Venkatesh, Morris, Davis and Davis2003; Holtz and Krein, Reference Holtz and Krein2011), which also explains our case. Our data were collected from 26 January to the end of February 2020, when China implemented the first-level response (i.e. grid management and all outdoor activities cancelled) to prevent the spread of the deadly virus, and almost all the resources were amassed and sent to Wuhan to ensure their usage (China Development, 2020). Hospital appointments for chronic diseases were not guaranteed in this context; thus, increasingly, the use of medical apps has become mandatory for older adults. Accordingly, perceived ease of use is not significantly related to their intention to use medical apps.
Therefore, the subjective norm measure in our research may not be as important as once assumed (Venkatesh et al., Reference Venkatesh, Morris, Davis and Davis2003). Although comments from significant others seem like an important factor in determining what people use, the UTAUT model uses subjective norms as an antecedent (i.e. if people suppose using a particular technology is expected by those who consider it significant, they would use it too). However, the lack of significance seen in our research could indicate descriptive norms, where their app usage might depend on what an individual perceives to be the actual norm (Cialdini et al., Reference Cialdini, Kallgren and Reno1991). In other words, the older people in our sample might not be easily influenced by others and have their own opinions on whether to use medical apps.
Besides factors that are directly related to the older adults' intention to use medical apps, we also find that the older people's attitude to using these apps can serve as an important channel, through which the measures of perceived usefulness, perceived ease of use, subjective norm and facilitating conditions can have a significant impact on their intention to use apps. Older people lag behind in technology use compared to the rest of the population (Huxhold et al., Reference Huxhold, Hees and Webster2020), particularly in the context of today's rapidly changing technology (Vicente, Reference Vicente2021). To our relief, older people worldwide have already realised the importance of this change, and have gradually begun to use electronic products (Li et al., Reference Li, Wang and Wang2017; Mohlman and Basch, Reference Mohlman and Basch2021). This positive change in the attitude of older adults towards using technology can inspire future marketing campaigns. Providers of medical apps need to extend their focus from the usefulness and ease of use of their products to building a positive image of their products or their companies among their potential clients. They could also receive regular feedback from older people about their products to improve updated versions.
We believe that the factors and mechanism identified in our research are crucial to implementing eHealth services in the Fourth Industrial Revolution. Big data and cloud storage could be used to collect and address common problems of older people to enable those with similar diseases to search for and check their symptoms (Yang, Reference Yang2019). We also believe it is very likely that using medical apps to access professional help will become the norm in a post-pandemic era. When we wrote this article, the COVID-19 variants were only Alpha and Beta. Since then, mutations including Gamma, Delta and Lambda have emerged, and we are now experiencing Omicron, whose overall global risk is rated as ‘very high’ by the World Health Organization. Many countries (e.g. Japan) have even closed their borders. Whether we like it or not, Omicron will not be the last variant, and China's case has lessons for other countries.
However, our findings are not without limitations. The sample component is one issue. We delivered our questionnaires through the internet, which means that older people who do not use the internet were excluded from the sampling process. This digital inequality among different groups further highlights the importance of paying special attention to the older adults in the Fourth Industrial Revolution. Future research should continue our efforts in two ways. Attention should be paid to those who choose not to use the internet by investigating their main reasons for rejecting it (e.g. Weaver et al., Reference Weaver, Zorn and Richardson2010), and possible strategies to encourage them to use the internet and medical apps should be developed. Researchers also need to focus on older people who cannot use the internet for socio-economic reasons and take these people into account in providing health care in the Fourth Industrial Revolution.
Our methodology also contains limitations. First, we employed a cross-sectional research design, thus, the variable relations cannot be interpreted as causality. Second, we have only included those who completed the survey in our total number of respondents (1,318); in other words, those who rejected the survey invitation and those who did not finish the survey were not included. Therefore, the return rate of this article cannot be calculated. Last but not least, future studies should pay attention to the courtesy bias, where respondents answer the questions in a way they expect researchers to potentially want them to, especially when they will be compensated for their participation. Although we used reversed code items and participants were not told explicitly about how much money they could obtain from this survey, the courtesy bias still cannot be fully addressed.
Acknowledgements
We want to thank the anonymous reviewers and the editor of Ageing & Society for their very careful reading of the previous submissions of this article and their valuable comments.
Financial support
This work was supported by the Fundamental Research Funds for the Central Universities (21lzujbkyjh011).
Conflict of interest
The authors declare no conflicts of interest.