Introduction
There has been growing support for a public health approach towards minimising gambling-related harms, which means adopting a range of measures to reduce the population level of risk (Browne et al., Reference Browne, Langham, Rawat, Greer, Li, Rose, Rockloff, Donaldson, Thorne, Goodwin, Bryden and Best2016; Wardle et al., Reference Wardle, Reith, Langham and Rogers2019; Livingstone & Rintoul, Reference Livingstone and Rintoul2020; Price et al., Reference Price, Hilbrecht and Billi2021). A public health approach to gambling has been used in New Zealand for over a decade (Adams & Rossen, Reference Adams and Rossen2012) and was adopted by the UK gambling regulator in 2019 (Gambling Commission, 2019). As the UK has the world's largest regulated online gambling market (Gambling Commission, 2018), any UK policy changes can have significant immediate effects on consumer welfare and also serve as a model for policymakers in jurisdictions with less established regulated online gambling markets. Safer gambling messages can act as one of these measures by potentially shaping the intentions and behaviour of recreational and low-risk gamblers. The Gambling Commission views safer gambling messages as one of its four preventative measures that aim to reduce harm on a population level (Gambling Commission, 2019). If effective, messaging campaigns could offer a cost-effective complement to more restrictive interventions such as self-exclusion (Hayer et al., Reference Hayer, Brosowski and Meyer2020), which are most suited for those at higher risk of experiencing harm (Blank et al., Reference Blank, Baxter, Woods and Goyder2021; Regan et al., Reference Regan, Smolar, Burton, Clarke, Sharpe, Henn and Marsden2022). Nonetheless, generic messages to ‘gamble responsibly’ have been criticised for being potentially stigmatising (Livingstone et al., Reference Livingstone, Rintoul, de Lacy-Vawdon, Borland, Dietze, Jenkinson, Livingston, Room, Smith and Stoove2019) and for being easily ignored by gamblers due to their lack of actionable content and frequent repetition (Lole et al., Reference Lole, Li, Russell, Greer, Thorne and Hing2019). The evaluation of alternative safer gambling messages is therefore of interest to industry groups, policymakers, and researchers.
There are several approaches to evaluating alternative safer gambling messages, including field studies run in collaboration with the industry (Behavioural Insights Team, 2018, 2021; Auer & Griffiths, Reference Auer and Griffiths2020; Heirene & Gainsbury, Reference Heirene and Gainsbury2021), qualitative focus groups (Davies et al., Reference Davies, Collard, McNair and Leak-Smith2022; Rockloff et al., Reference Rockloff, Newall, Browne, Russell, Visintin, Hing and Thorne2022), self-report surveys (Davies et al., Reference Davies, Collard, McNair and Leak-Smith2022), and behavioural experiments (Rockloff et al., Reference Rockloff, Newall, Browne, Russell, Visintin, Hing and Thorne2022; Newall et al., Reference Newall, Weiss-Cohen, Singmann, Walasek and Ludvig2022b). Each of these methodologies probes different aspects of messaging and has different strengths and weaknesses. Field studies have the strength of measuring effects on naturalistic behaviour and have been shown to encourage the use of deposit-limit-setting tools (Behavioural Insights Team, 2018; Heirene & Gainsbury, Reference Heirene and Gainsbury2021). But field studies require the active collaboration of an industry partner, and this requirement has arguably restricted the number of messages tested via this method. By comparison, self-report surveys are a cost-effective way of evaluating both existing and potential safer gambling messages (Davies et al., Reference Davies, Collard, McNair and Leak-Smith2022), but self-report surveys can only evaluate impressions and feelings, which may not translate into real and consequential behaviour (Sheeran & Webb, Reference Sheeran and Webb2016). In contrast, experimental research, as used here, provides control over the gambling context and can go beyond self-reported impressions and feelings by measuring messaging's effect on behaviour. Experiments, however, can be limited in terms of external validity and the range of outcomes measured.
Between 2014 and 2021, and in response to the growing concerns about widespread gambling-related harm, gambling operators in the UK primarily used a ‘when the fun stops, stop’ slogan which featured prominently in most betting shop windows, on operator websites, and in TV and online advertisement campaigns (Critchlow et al., Reference Critchlow, Moodie, Stead, Morgan, Newall and Dobbie2020; van Schalkwyk et al., Reference van Schalkwyk, Maani, McKee, Thomas, Knai and Petticrew2021). An independent experimental evaluation of this message, however, found no credible protective effects on gamblers’ behaviour on sporting bets and online roulette (Newall et al., Reference Newall, Weiss-Cohen, Singmann, Walasek and Ludvig2022b), which accords with critiques of this message's lack of face validity given its emphasis on the word ‘fun’ (van Schalkwyk et al., Reference van Schalkwyk, Maani, McKee, Thomas, Knai and Petticrew2021).
As of October 2021, this message was replaced with one featuring the words ‘take time to think’ in the same colour scheme. As the new campaign's website www.taketimetothink.co.uk says, ‘All across the country, people are pausing, taking time to think … ’. In the present paper, we conduct the first independent experimental test of the newly introduced ‘take time to think’ gambling message. Following the design of Newall et al. (Reference Newall, Weiss-Cohen, Singmann, Walasek and Ludvig2022b), we created a realistic gambling scenario in which participants made decisions in an online game of roulette with real monetary consequences.
In addition to testing the new safer gambling message, the present study extends previous work in two respects. First, a gambling message may not necessarily discourage people from gambling but may rather promote other protective behaviours, such as encouraging individuals to seek help if they experience negative feelings while gambling. Given the latest message's wording, its main behavioural effect may be to promote more deliberation and slower gambling. Indeed, because impulsivity and the use of continuous-play gambling formats have been associated with gambling-related harm (Ioannidis et al., Reference Ioannidis, Hook, Wickham, Grant and Chamberlain2019; Allami et al., Reference Allami, Hodgins, Young, Brunelle, Currie, Dufour, Flores-Pajot and Nadeau2021), assessing whether the message can affect the speed of gambling is important. Second, a safer gambling message may fail to have a behavioural effect in an experiment not because of its content, but due to a lack of prominence with which it is shown to participants. In gambling research, popup messages are thought to be the most prominent type of message (Monaghan, Reference Monaghan2009) and to be the most effective at changing short-term gambling behaviours (Bjørseth et al., Reference Bjørseth, Simensen, Bjørnethun, Griffiths, Erevik, Leino and Pallesen2021).
Therefore, in the present experiment, participants were randomly allocated to either (a) a control group where no gambling message was present, (b) a message condition, in which ‘take time to think’ message was visible on the screen throughout or (c) a message+ condition with ‘take time to think’ displayed prominently as a popup prior to the game of online roulette, which had to be dismissed before the participant could continue. This popup message was in addition to the message being shown on screen throughout (as was done in the message condition). This high level of prominence and participant interaction was designed to maximise the potential effects of the message on contemporaneous behaviours.
Given the differential findings across other gambling messaging studies, we did not preregister any directional hypotheses. Instead, the experiment was designed to test the following null hypotheses, predicting that there would be no differences in behaviour between the control group and each of the two message conditions with respect to participants:
1. Proportion of available funds bet
2. Likelihood of clicking on help service information
3. Mean speed of play (total number of seconds between any two spins on the roulette wheel)
4. Total number of spins made
Methods
The preregistration document, study materials, anonymised data and analysis code are provided via the Open Science Framework repository available at: https://osf.io/k8dmg/. The study was implemented in oTree (Chen et al., Reference Chen, Schonger and Wickens2016).
This study received ethical approval from the University of Warwick Humanities and Social Sciences Research Ethics Committee.
Study design
Participants recruited for the study were paid a baseline fee of £2.50, which they all received irrespective of any roulette game outcomes (and irrespective of their performance on the initial task). The study title and instructions stated that participants could earn a larger bonus for the successful completion of an initial task and that participants would have the option to bet that bonus on a roulette game. The study title read: ‘Fill in captchas codes correctly and win a bonus that can optionally be used to bet on a roulette game’, and the rest of the participant information sheet is available via the above Open Science Framework link.
The initial task involved the typing of 10 captcha codes, which are scrambled letters similar to those used by websites to identify humans (Newall et al., Reference Newall, Weiss-Cohen, Singmann, Boyce, Walasek and Rockloff2022a, Reference Newall, Weiss-Cohen, Singmann, Walasek and Ludvig2022b). This initial task was used for two reasons. First, crowdsourced samples have been criticised for being inattentive (Pickering & Blaszczynski, Reference Pickering and Blaszczynski2021) and successful typing of captcha codes requires a non-trivial amount of focus and involvement from a participant. Second, the initial task is an example of a ‘real effort’ task used in behavioural economics to make sure that participants earn their bonus, so as to better approximate gambling with one's own money (Erkal et al., Reference Erkal, Gangadharan and Nikiforakis2011). A relatively low threshold of 2 out of 10 captchas was needed for participants to earn their bonus and proceed to the roulette (participants were not told the threshold). This threshold was implemented to screen out truly inattentive participants, while not producing a sample biased towards certain characteristics (for example, highly impulsive participants might have been screened out if all 10 captcha codes had to be successfully typed because they would be prone to completing the captchas more quickly).
Successful participants were then automatically allocated to one of the three experimental conditions randomly using simple randomisation procedures (computerised random numbers), with equal probabilities (1:1:1). Successful participants also earned a bonus of £5, which could optionally be wagered on the roulette game, and which was chosen as being representative of the median stakes wagered by UK online gamblers in casino-based games (Forrest et al., Reference Forrest, McHale, Dinos, Ashford, Wilson, Toomse-Smith and Martin2022). Participants did not have to gamble if they did not want to, and 579 participants (25.4%) took this option. These participants were paid the full £5 endowment without gambling, which along with the £2.50 baseline represented a total payment of £7.50 (see results for detailed analysis of the propensity to gamble).
The roulette game was a commercially available model programmed in Javascript (see Figure 1), which allowed participants a range of different betting options, with bets being possible in minimum increments of £0.10, up to a maximum of £2 per spin (Figure 1). Random outcomes were determined by the server after every spin. Participants were free either to proceed past the roulette game without placing any bets, or to place as many bets as they wanted and their bonus allowed, and to engage with the game in whatever way they preferred.
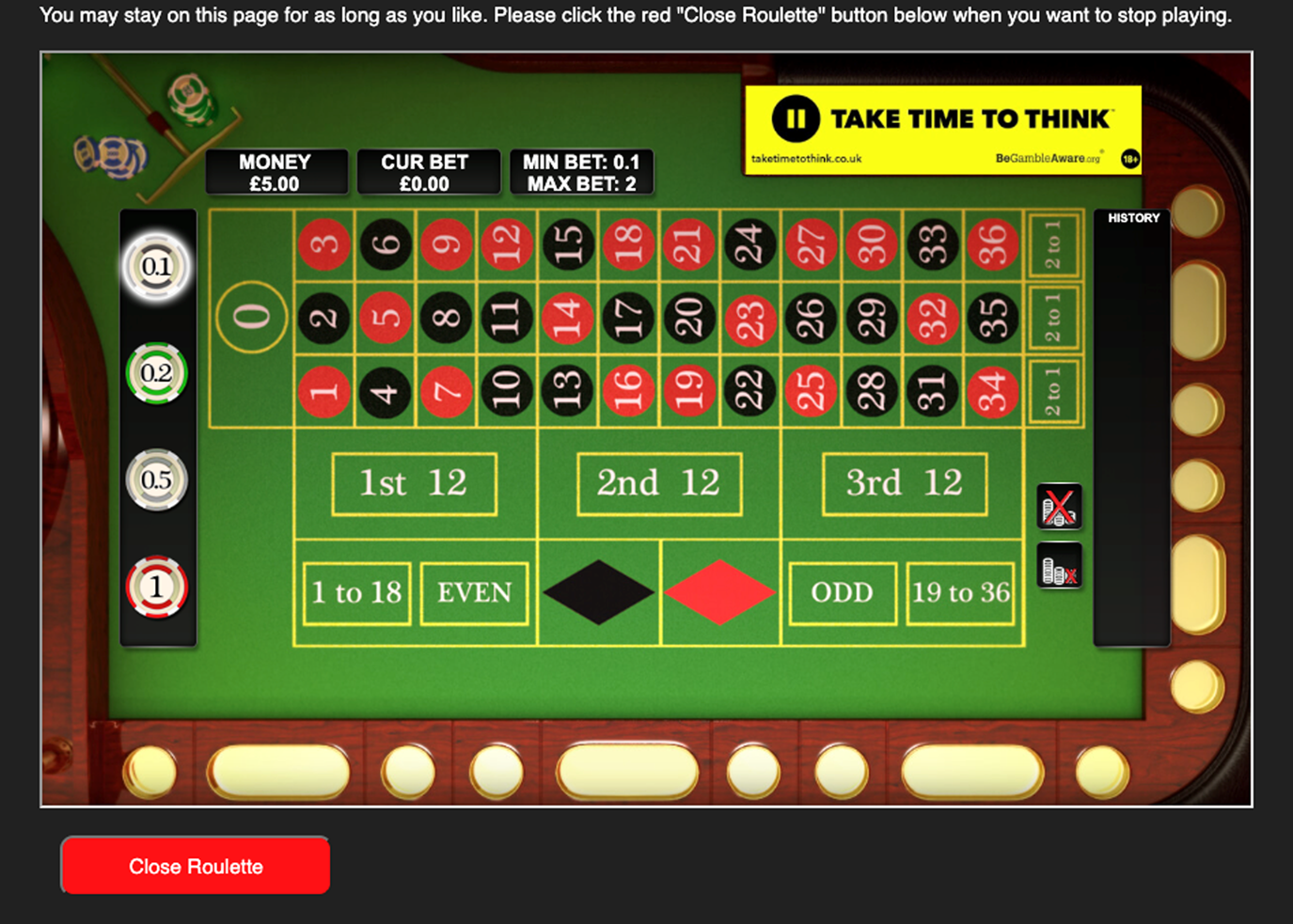
Figure 1. Screenshot of the roulette task (message condition).
Participants were randomly allocated to one of three conditions. In the no-message condition, participants completed the gambling task without seeing the ‘take time to think’ message. In the message condition, participants saw the ‘take time to think’ message while the roulette game was on screen and also while reading the roulette instructions on the preceding instruction page. Participants saw the same messages in the message+ condition, plus they were shown an identical ‘popup’ message immediately before they could interact with the roulette wheel. This ‘popup’ functioned as a modal overlay within (and completely occluding) the roulette interface, which had to be actively closed by the participant before they could proceed.
Participants
A CONSORT flowchart is shown in Figure 2. Participants were recruited via the crowdsourcing platform Prolific, which has been suggested to yield higher data quality than rival crowdsourcing platforms such as MTurk (Eyal et al., Reference Eyal, David, Andrew, Zak and Ekaterina2021). Participants were aged 18 and over, residents in the UK and, for the first five days of data collection (31 March 22 to 04 April 22), had previously reported experience in playing online roulette to Prolific (n = 1,805). In line with the preregistration, on the sixth and last day of data collection (05 April 22), eligibility was widened to include participants with experience in other online gambling games, in order to spend the remainder of the budget reserved for data collection (n = 500).

Figure 2. CONSORT flowchart.
In total, 2,305 participants took part in the initial captcha typing task, of whom 20 transcribed fewer than two captchas correctly and did not, therefore, take part in the rest of the experiment. Five participants abandoned the task part-way through, and two responses were excluded which, due to a server problem, occurred from the same participant. This resulted in a final sample size of 2,278 participants (755 [33.1%] in the no-message condition, 796 [34.9%] in the message condition and 727 [31.9%] in the message+ condition).
The average completion time was 8.5 min, and the average final bonus payout was £4.91 (range: [0, 79.4]). This bonus was added to the baseline fee of £2.50, which resulted in an equivalent pro-rata pay rate of £52 per hour. In total, 579 participants (25.4%) did not place any bets, thereby keeping their entire £5 bonus. On average, participants who gambled played for eight spins (median: 4; range: [1, 198]).
At the end of the task, participants self-reported age and gender, before completing the nine-item Problem Gambling Severity Index (PGSI; Ferris & Wynne, Reference Ferris and Wynne2001), exiting the task and receiving their debrief. Table 1 provides a breakdown of these demographic details both for the sample overall and per condition. Overall, 210 out of 2,278 (9.2%) participants had a PGSI score of 8 or higher, corresponding to the group that was referred to by the PGSI scale designers as ‘problem gamblers’. This corresponds closely to the percentage of online casino game users who are thought to have PGSI scores in this range (NHS Digital, 2019). The median age was 34 yr (mean 35.9 yr). Overall, 965 (42.4%) participants were women, and 1,289 (56.6%) participants were men (15 non-binary, 4 prefer not to say and 5 other).
Table 1. Demographic and summary data split by the condition

M, mean; SD, standard deviation; n, count.
Procedures and outcomes
Participants who successfully typed in two or more captchas were shown a page containing instructions on how the roulette game worked. Participants could remain on the roulette game's page for as long as they wanted or close it immediately. Following a previous investigation of the ‘when the fun stops, stop’ message (Newall et al., Reference Newall, Weiss-Cohen, Singmann, Walasek and Ludvig2022b), the main outcome measure was the proportion of available funds bet in this part of the experiment. This outcome varied between zero (for participants betting nothing) and one (for participants betting and losing everything, including any intermediary winnings). For participants making at least one bet but leaving with some bonus, the proportion bet was a fraction between zero and one. This approach is more sensitive than using participants’ final balance as an outcome measure, as it can differentiate between a participant who bet nothing (and received £5) and a participant who placed £10 in bets in total but broke-even overall (and therefore also received £5, which was the starting bonus). Compared to final balance as an outcome measure, the proportion bet is also less sensitive to participants’ luck as large (and random) winnings do not affect the proportion bet in an extreme manner. This outcome measure was used to investigate any message-induced effects on gambling expenditure that is as naturalistic as possible. Some gambling experiments present all participants with an identical stream of payoffs (Byrne & Russell, Reference Byrne and Russell2020), and others produce a programmed stream of losses after a certain number of bets (Rockloff et al., Reference Rockloff, Donaldson and Browne2015). Both of these approaches involve an element of deception and also limit the generalisability of any findings via the use of unnaturalistic outcomes. The study's cover sheet truthfully informed participants that all roulette outcomes were random and not predetermined in any way.
An a priori power analysis was performed for the proportion bet outcome. This analysis compared two groups each with n = 500 (the minimum preregistered sample size per condition and the actual sample size was at least n = 727 per condition). The model parameters were based on a previous study (Newall et al., Reference Newall, Weiss-Cohen, Singmann, Walasek and Ludvig2022b), and additionally manipulated the true difference in proportion bet between the two groups from 0% to 10% in increments of one percentage point. Each difference was simulated and analysed in 10,000 synthetic samples. This analysis (see also Supplementary Figure S6) showed a 79% probability of detecting a difference in proportion bet of 6 percentage points and an 88% probability of detecting a difference in proportion bet of 7 percentage points. The analysis also showed that the proportion bet model was well calibrated; in the absence of an effect, the Bayesian 95% CI excluded 0 in 4.8% (95% binomial CI [4.4–5.3%]) of cases.
The experiment also unobtrusively recorded the time spent on the roulette game and the timing of any spins made. This information was used to record the speed of play: the number of seconds between any two spins (which did not include the time taken for the roulette wheel to spin and display the bet outcome). The first spin was excluded from this measure because (a) the time taken until the first spin involved both the loading of the roulette game (which depended on participants’ individual computer set-ups), (b) participants were expected to take extra time to familiarise themselves with how the roulette game worked on the first spin and (c) the popup message created a confound between the message+ condition and other conditions for the first spin only. As a result of excluding the first spin, the data for this outcome were only available from participants engaging in at least two spins. One additional outcome, the total number of spins made, was also recorded. This additional measure allowed the evaluation of whether any potentially slower speed of play in the message conditions would also be reflected in reductions in the total number of spins made. If a slower speed of play is not also reflected in a reduction of the total number of spins made, then it may simply reflect increases in the total amount of time spent gambling (and therefore remove time for activities other than gambling).
Participants could move on to the next page of the task, beyond the roulette, whenever they wanted. In addition, the task was programmed so that participants who lost all of their bonus, or who increased their balance to £150 or more, were automatically moved on (however, no participant reached the £150 threshold at any time). This maximum-winnings threshold was not communicated to participants, so as not to potentially influence participants towards greater levels of risk-taking. The next page of the task was based closely on the current homepage for gamcare.org.uk, the UK's main provider of gambling support, and contained information on the four main Gamcare services: phone advice, live web-based chat, a group chatroom and an online forum (see Supplementary Figure S5). Participants were given the option to have a new browser tab open on the Gamcare homepage upon task completion, so as to provide participants with easy access to help resources, but without interfering with the remainder of the task. Participants indicated their preference by clicking on one of the two buttons: ‘Yes, please open a Gamcare tab’, or ‘No, please do not open a Gamcare tab’. The position of these two buttons was randomised on the screen. To see if message presence affected the rates of clicks for help service information, the proportion of participants in each condition clicking the ‘Yes, please open a Gamcare tab’ was also recorded.
Statistical analysis
All analyses were performed in a Bayesian statistical framework using R 4.1.3 (R Core Team, 2020) and the brms package (Bürkner, Reference Bürkner2017). A Bayesian framework was chosen, as Bayesian estimation is both more flexible for bespoke statistical models and is known to produce fewer convergence problems than frequentist estimation for the type of statistical model employed here (Liu & Eugenio, Reference Liu and Eugenio2018). The models used Markov Chain Monte Carlo (MCMC) sampling (Van Ravenzwaaij et al., Reference Van Ravenzwaaij, Cassey and Brown2018), which is the recommended way of estimating Bayesian models. All Bayesian analyses were based on 100,000 post-warm-up samples from four independent chains. For all models, we use the default brms priors which are also given in Supplementary Materials. To ensure model convergence, we visually checked the chains and made sure all R-hat values were approximately 1.00, which indicated that all models had successfully converged to the stationary distribution.
Each model was set up such that the no-message condition was the reference group (i.e., the intercept corresponded to the mean of the no-message condition), with different parameters estimated for each of the two message conditions. The inference criterion used was whether the 95% credibility intervals of posterior difference distributions from the no-message condition contained 0 (the a priori power analysis for proportion bet described above showed that this inference criterion was well calibrated; in the absence of an effect only 5% of the 95% credibility intervals did not contain 0).
The proportion bet analysis was performed via a zero-one-inflated beta regression (ZOIBR) model with bespoke parameterisation described in Supplementary Section 3. This model was chosen due to its ability to describe the anticipated non-normal distribution of responses, with peaks at zero (participants betting nothing), one (participants betting everything they could) and a unimodal distribution for the remaining participants, using separate distributional parameters for the location of each peak. The distributional parameters associated with the location of each peak were allowed to differ across conditions (the scale parameter for the beta-regression part was fixed across conditions). To ensure all distributional location parameters were constrained to the 0–1 range, we used a generalised linear model formulation using a probit link. To simplify the interpretation of the results from this model, we then re-combined the posterior distributions of the three distributional location parameters and created a posterior distribution of proportion bet for each message condition on which the main analyses are based.
The outcome measure of clicks for help service information via the Gamcare button was analysed via a Bernoulli model. The speed of play was analysed with a shifted log-normal model with fixed-effects for the condition for both distributional parameters, log-mean and log-SD (i.e., a heteroscedastic model allowing different log-SDs for each condition, only the shift parameter was fixed across conditions). The model also included random intercepts for participants for the log-mean. Betting times greater than 120 s were excluded from this analysis, with this preregistered threshold chosen based on an expected average betting time of 20 s (SD = 10 s) based on results from a previous study (Newall et al., Reference Newall, Weiss-Cohen, Singmann, Boyce, Walasek and Rockloff2022a), therefore corresponding to the mean plus 10 standard deviations. The total number of spin analysis was performed via a negative binomial model truncated at 1. The reason for only including participants who made at least one bet was that the outcome of participants betting nothing was already captured in the proportion bet analysis. Further details on each model (such as the choice of link functions) can be found in Supplementary Materials.
Two additional models were run for the proportion bet dependent variable. The first added participants’ PGSI scores as a main effect only, while the second also added an interaction term between the PGSI and the message condition. These analyses were done to test the robustness of the results across different levels of the PGSI score. Additional analyses exploring interactions between the PGSI and the other outcome measures were only planned in the scenario where the additional analyses for proportion bet displayed a different pattern of results with respect to the main inference criteria from the primary analysis (which they did not, as shown below).
To ensure the descriptive adequacy of the chosen models for the different outcome measures, we performed two model checks. Firstly, we visually compared the distributions of the observed data with samples from the posterior predictive distribution (i.e., synthetic data of the same size as the observed data generated from the estimated model). For each outcome measure and model, this comparison indicated a high degree of similarity between the distribution of the observed data and the distribution of the synthetic data based on the estimated model. Secondly, we compared the observed condition means with the estimated condition means. For each outcome measure and model, the observed means were very similar to the estimated means (i.e., the observed means were always within the 95% credibility of the estimated means). Taken together, these two checks indicate that the chosen models are appropriate for the different outcome measures as they can adequately recover and describe the observed data.
Results
Preregistered main analysis
The proportion bet analysis was run as preregistered, and the ZOIBR model described the data well (Supplementary Section 4). Results are shown in Figure 3: the left panel shows the posterior distribution of mean proportion bet per message condition, and the right panel shows the posterior different distributions comparing the two message conditions from the no-message condition. Descriptively, the mean proportion bet was around 2 percentage points lower in both message conditions compared to the no-message conditions (2.1 percentage points lower in the message condition and 2.0 percentage points lower in the message+ condition). As shown in the right panel of Figure 3, however, the uncertainty associated with this difference was considerably larger than the observed 2 percentage point difference (i.e., both 95% credibility intervals clearly contained 0), indicating that this did not provide credible evidence for a protective effect of the gambling messages on proportion bet.

Figure 3. ZOIBR model estimates for proportion bet across conditions. The left panel shows the posterior distributions of mean proportion bet across the three message conditions. The right panel shows mean difference posterior distributions comparing the no-message condition with the two message conditions (negative values indicate less gambling in the message conditions and positive values indicate more gambling in the message conditions). In each panel, the grey area shows the full posterior distribution in terms of a density estimate, the black dot shows the posterior mean and the horizontal black line shows the 95% credibility interval (CI). The 95% difference CI excluding 0 (the vertical grey line) would represent evidence for a credible difference between two conditions.
The proportion bet can also be decomposed into the three-individual distributional ZOIBR parameters (Supplementary Section 3). This exploratory analysis suggested no credible effect from the messages on the three sub-components of proportion bet (Supplementary Section 5). For example, the percentages of participants betting nothing at all were: 24.6% [95% credibility interval (21.6–27.7%)] in the control condition, 26.6% [23.6–29.7%] in the message condition and 24.9% [21.9–28.1%] in the message+ condition.
To ensure the proportion bet results were robust to potential differences in participants’ self-reported gambling behaviour, two preregistered ZOIBR analyses were run including participants’ PGSI scores. The first model included PGSI scores as a main effect for each distributional parameter, and the second model additionally included them as an interaction with the message condition effect. In both of these models, the null effect of the gambling message was replicated: the 95% CIs of the difference distributions from the no-message condition for proportion bet all included 0 (Supplementary Section 6).
The additional ZOIBR models revealed that PGSI was positively related with the proportion bet, indicating that participants with higher PGSI scores were betting more of their available funds than participants with lower PGSI scores. When the PGSI was included only as a main effect, it showed a statistically credible positive relationship with all three distributional location parameters. Participants with higher PGSI scores were more likely to gamble at all (b = 0.07, [95% CI: 0.04, 0.10]), were more likely to gamble everything (b = 0.09, [0.06, 0.12]) and had a higher mean proportion bet in the beta-regression part of the model (b = 0.02, [0.01, 0.03]). When also including interactions of PGSI with the message condition effects, we found a similar pattern (i.e., overall positive relationships for all distributional parameters) but also some evidence for a differential pattern. In particular, the interaction between the PGSI and the message+ condition was statistically credible for the mean proportion bet parameter of the beta-regression part (b = 0.05, [0.02, 0.08]). For all five remaining interaction parameters (two for the message+ condition and three for the message condition), the 95% credibility intervals contained 0. This interaction for the beta-regression component of the model can be interpreted in two equivalent ways: high PGSI scores in the message+ condition could be associated with a backfire effect (i.e., more gambling) compared to the control condition or the positive relationship between the PGSI and proportion bet was particularly strong in the message+ condition. The effect, however, was only credible for one out of six interactions, suggesting that the evidence for a differential pattern is not overly strong.
Null results showing no credible protective effect of the ‘take time to think’ gambling messages were also observed for the secondary outcome measures. Results for the preregistered Bernoulli model analysing the rate of clicks for help service information – the probability with which participants opened the Gamcare link – are shown in Figure 4 (the model provided an excellent account of the data, Supplementary Section 7). Across all message conditions, the rate of clicks was low with posterior means between 2.5% (in the message+ condition) and 2.9% (in the message condition). Furthermore, the difference between the no-message and the message conditions was near zero with difference distributions centred almost perfectly on zero.

Figure 4. Bernoulli model estimates for the rates of clicks for help service information across conditions. The left panel shows the posterior distribution of the probability with which participants open the Gamcare link per condition, and the right panel shows the difference posterior distributions for the differences from the no-message condition. See Figure 3 for more details.
There were 13,590 spins made (11,891 from the second spin onwards which were used for the following analysis), providing a large sample size for the speed of play analysis. Overall, 29 bets (0.24%) took longer than 120 s and were removed from the analysis (following our preregistration plan). These 29 bets were distributed as follows: no-message (8), message (12), message+ (9). As a robustness check, we ran a version of the below analysis that included these 29 bets, which produced the same qualitative and very similar quantitative results. As expected, the distribution of times was strongly right-skewed, with an observed mean betting time of 9.7 s (median = 5.9 s; SD = 11.2 s). We used a shifted log-normal mixed-effects model which adequately described the betting time distribution (Supplementary Section 8). The model results are shown in Figure 5. Descriptively, betting times in the message+ condition were around 0.5 s slower compared to the no-message condition but very similar between the message and no-message conditions. As shown in the right panel of Figure 5, the uncertainties of the different distributions were again considerably larger than the estimated differences with all 95% CIs clearly including 0. Thus, the ‘take time to think’ message did not credibly induce participants to take more time to place their bets.

Figure 5. Shifted log-normal model estimates for participants’ betting times (excluding the very first bet) across conditions. The left panel shows the posterior distribution of the estimated mean betting times, and the right panel shows the mean difference posterior distributions for the differences from the no-message condition. See Figure 3 for more details.
The final analysis concerned participants’ number of spins. Figure 6 shows the results from the preregistered truncated negative binomial model for the data after removing participants with zero spins. This model also provided an adequate account of the observed data (Supplementary Section 9). The estimated mean number of spins was very similar across message conditions ranging between posterior means of 7.6 (message condition) and 8.3 (no-message condition). As for the other outcome measures, the uncertainties of the different distributions shown in the right panel of Figure 6 clearly exceeded the estimated differences between conditions; both 95% CIs clearly include 0. Thus, there was no evidence for a credible difference in the mean number of spins between gambling message conditions.

Figure 6. Truncated negative binomial model estimates for the number of spins (after excluding participants with 0 spins). The left panel shows the posterior distribution of the estimated mean number of spins, and the right panel shows the mean difference posterior distributions for the differences from the no-message condition. See Figure 3 for more details.
Discussion
‘Take time to think’ is a safer gambling message, which at the time of writing is used by the majority of the UK gambling industry. The UK regulator, the Gambling Commission, views safer gambling messages as one of its four preventative measures that aim to reduce harms on a population level (Gambling Commission, 2019). Furthermore, the Gambling Commission is aware that any intervention may not always work as intended, so welcomes the evaluation of interventions, particularly independent evaluations such as the present research (Gambling Commission, 2019). The present research tested whether the prominent display of this message would have any effect on contemporaneous gambling behaviours in an online roulette game. Overall, the results showed no credible effects comparing the two treatment conditions with the control condition across the four dependent measures. These null effects were observed despite the message being shown more prominently before and during gambling than it is typically used on, for example, gambling operators’ websites. The lack of a credible effect on the speed of play was perhaps especially striking, as the message explicitly reminds gamblers to ‘take time to think’. Furthermore, this message was shown as a popup message immediately before the gambling opportunity in the message+ condition, which should be optimal conditions for a message to affect behaviour (Bjørseth et al., Reference Bjørseth, Simensen, Bjørnethun, Griffiths, Erevik, Leino and Pallesen2021).
Whereas the effect was not statistically credible, it is notable that the proportion bet outcome was around 2 percentage points lower in each of the two message conditions. If statistically credible, this decrease would correspond to a reduction of £0.1 in median stake, assuming a median stake of £5 for online casino-based games (Forrest et al., Reference Forrest, McHale, Dinos, Ashford, Wilson, Toomse-Smith and Martin2022). A 2-percentage point difference, however, was below our detection threshold of around 5–6 percentage points, so the current result cannot be reliably distinguished from a null effect. A study with the goal of reliably detecting such a small effect would likely need a considerably larger sample size than the current one (or an outcome with considerably less variability). Whether an effect of such a small potential magnitude justifies such an effort should be carefully considered before running a corresponding study.
We also found no evidence of any protective effect across the two treatment conditions for the other three outcome variables. There was very little difference between the three conditions for the clicks for help service information outcome, although the low base-rate of clicks may have contributed to this finding. For the speed-of-play outcome, there was a small and not-credible effect such that participants took additional 0.5 s in the message+ condition, with very little difference between the control and message conditions. For the total number-of-spins outcome, the results were also small and not statistically credible, as participants made 0.7 fewer spins in the message condition, with very little difference between the control and message+ conditions. Overall, these results cast doubt on the possibility that the collection of a larger sample size will lead to consistent protective effects across the four gambling behaviours studied here.
Nonetheless, the present results do provide some optimism for the potential of further improvements in safer gambling message content to affect behaviour. A previous study on ‘when the fun stops, stop’ showed an overall pattern of null results but with a descriptive pattern that instead pointed towards a potential backfire effect (Newall et al., Reference Newall, Weiss-Cohen, Singmann, Walasek and Ludvig2022b). In line with the improved face validity of ‘take time to think’ as a safer gambling message, the descriptive patterns in the present study were more encouraging, even if very small in magnitude. This suggests that other messages may be able to display credible (but likely small) protective effects. For example, messages that have been previously proposed in the literature include messages that, similar to the health warnings on tobacco products, highlight the harms associated with gambling, e.g., ‘gambling is associated with significant harms including increased risks of physical and mental health problems, separation, divorce, financial difficulties and bankruptcy, intimate partner violence and fraud’ (Livingstone et al., Reference Livingstone, Rintoul, de Lacy-Vawdon, Borland, Dietze, Jenkinson, Livingston, Room, Smith and Stoove2019: 10). Another recently proposed class of messages focuses on leveraging positive emotions to prevent heavy gambling, e.g., ‘save the rest of your money for that family trip next month’ (Harris et al., Reference Harris, Parke and Griffiths2018: 271). Positive emotional messages have received some circumstantial support in a different experimental paradigm using self-reported gambling intentions and expenditure (Rockloff et al., Reference Rockloff, Newall, Browne, Russell, Visintin, Hing and Thorne2022), which should be further investigated in an experiment using direct measurements of gambling behaviour, as done here.
This conclusion is subject to various limitations, which create alternative explanations for the present results. Firstly, multiple exposures of the message may be necessary, or gamblers may need to interact with other materials run alongside the message itself, such as accompanying TV commercials. Secondly, the message may be better at affecting distal gambling behaviours, instead of the contemporaneous behaviours measured here. Thirdly, the campaign may have already attained its maximum effectiveness through prior exposure through these various channels, such that the additional exposure to the message provided within the experiment did not have any further effect (however, these data were collected less than seven months from the campaign's launch). A longitudinal study could best test this first explanation, field data from online operators could best test this second explanation, while a replication study using participants from another jurisdiction could test this third explanation. Furthermore, the low base-rate of clicks on the Gamcare tab may have made it harder to detect any potential credible effects on this outcome measure. Another limitation inherent to gambling experiments is that participants gambled with a bonus given to them, which is psychologically different from gambling with their own money (Gainsbury & Blaszczynski, Reference Gainsbury and Blaszczynski2011).
One further possibility is that the observed null results occur because our experimental design is insufficient for capturing the effect of safer gambling interventions on gambling behaviour. However, two independent pieces of evidence speak against this possibility. Firstly, previous work using an online roulette game did find a protective effect of a speed-of-play restriction on two outcomes which overlap with the present research. Specifically, a minimum interval of 60 s between any two spins in the roulette game led to a credible decrease in proportion bet of around 4.6 percentage points and a credible reduction of 1.6 mean total numbers of spins among those who gambled (n = 1,002; Newall et al., Reference Newall, Weiss-Cohen, Singmann, Boyce, Walasek and Rockloff2022a). This demonstrates that participants’ behaviour in online roulette gambling tasks can be influenced by experimental interventions and that interventions stronger than messaging alone may be necessary to see meaningful behavioural change. Furthermore, there was a positive relationship between participants’ PGSI scores and gambling behaviour, such that participants with higher PGSI scores gambled more of their money in the experiment. This indicates that participants’ behaviour in the online roulette gambling task is similar to their actual gambling behaviour and affected by similar factors as their actual gambling behaviour.
Conclusion
From a policy perspective, this work allows for two conclusions. First, it shows how the effectiveness of any new messaging campaign can be tested experimentally across multiple dimensions in a timely manner, in a way that recreates several aspects of naturalistic online gambling. It can be difficult to test messaging campaigns via field studies, as these designs require the active collaboration of an industry partner, be it privately- (Heirene & Gainsbury, Reference Heirene and Gainsbury2021) or state-owned (Jonsson et al., Reference Jonsson, Hodgins, Munck and Carlbring2020). However, any optimism around novel types of messages should be informed by the results of previous field studies, which have often shown either null or small effects from messaging on gambling behaviour (Behavioural Insights Team, 2018, 2021; Heirene & Gainsbury, Reference Heirene and Gainsbury2021). Second, and relatedly, messaging alone may simply be insufficient to reduce gambling-related harm. Indeed, even the prominent display of the ‘take time to think message’ message is unlikely to affect a range of contemporaneous gambling behaviours. We conclude that messaging alone can only make up a small part of a public health approach to reducing gambling-related harms (Browne et al., Reference Browne, Langham, Rawat, Greer, Li, Rose, Rockloff, Donaldson, Thorne, Goodwin, Bryden and Best2016; Wardle et al., Reference Wardle, Reith, Langham and Rogers2019; Livingstone & Rintoul, Reference Livingstone and Rintoul2020; Price et al., Reference Price, Hilbrecht and Billi2021), which should also use a range of complementary interventions (Blank et al., Reference Blank, Baxter, Woods and Goyder2021; Regan et al., Reference Regan, Smolar, Burton, Clarke, Sharpe, Henn and Marsden2022). Indeed, messaging should perhaps be principally used to increase the effectiveness of other interventions, such as support services, changes to the structural characteristics of harmful gambling products, or a potential universal pre-commitment system (Livingstone et al., Reference Livingstone, Rintoul, de Lacy-Vawdon, Borland, Dietze, Jenkinson, Livingston, Room, Smith and Stoove2019). Furthermore, personalised messages that accurately convey information about a gambler's own situation are likely more effective than the repetitive slogans in current use (Auer & Griffiths, Reference Auer and Griffiths2020; Rintoul, Reference Rintoul2022).
Supplementary material
To view supplementary material for this article, please visit https://doi.org/10.1017/bpp.2023.2.
Funding statement
This research was funded by a Research Development Fund Strategic Award grant awarded to Lukasz Walasek, Elliot Ludvig and Ty Hayes from the University of Warwick. The funders played no role in the design, execution, analysis and interpretation of data, or writing of the study.
Competing interests
P.N. is a member of the Advisory Board for Safer Gambling – an advisory group of the Gambling Commission in Great Britain – and in 2020 was a special advisor to the House of Lords Select Committee Enquiry on the Social and Economic Impact of the Gambling Industry. In the last three years, P.N. has contributed to research projects funded by the Academic Forum for the Study of Gambling, Clean Up Gambling, Gambling Research Australia, NSW Responsible Gambling Fund, and the Victorian Responsible Gambling Foundation. P.N. has received open access fee grant income from Gambling Research Exchange Ontario. T.H. declares no conflicts. H.S. has received open access fee grant income from Gambling Research Exchange Ontario. L.W.-C. has received open access fee grant income from Gambling Research Exchange Ontario. E.L. has received open access fee grant income from Gambling Research Exchange Ontario and is also the Research Co-Chair for the Academic Forum for the Study of Gambling. L.W. has received open access fee grant income from Gambling Research Exchange Ontario.