Estimation of RMR using prediction equations is a fundamental part of clinical dietetic practice and is the basis for estimating daily energy requirements using the factorial method(1). Despite known limitations, prediction equations offer a practical alternative to measuring RMR by indirect calorimetry since energy requirements can be calculated using routinely available measures such as weight, age, sex and height. Expense of indirect calorimetry equipment, requirement for trained personnel, and the time-consuming nature of RMR measurement prevent indirect calorimetry being routinely available in dietetic settings(Reference Frankenfield, Roth-Yousey and Compher2), though it is frequently used in research studies.
RMR accounts for 60–70 % of total daily energy expenditure and fat-free mass (FFM) is the primary determinant of energy expended at rest(Reference Ravussin, Lillioja and Anderson3). FFM consists of multiple organs and tissues with varying metabolic rate(Reference Javed, He and Davidson4). Despite comprising <6 % of total body weight, organs are the major drivers of RMR, with the brain, heart, kidneys and liver collectively accounting for 60–80 % and although skeletal muscle represents a greater proportion of FFM, its metabolic activity is lower(Reference Javed, He and Davidson4,Reference Muller, Bosy-Westphal and Kutzner5) . Since accurate measurement of FFM is not routinely available, RMR equations usually rely on body weight as the dominant predictor, and also consider age, sex and sometimes height(Reference Frankenfield, Roth-Yousey and Compher2). There are many factors influencing RMR and there is considerable individual variation even when adjusting for FFM, age and sex(Reference Ravussin, Lillioja and Anderson3).
Numerous published prediction equations are available to estimate RMR, with 248 different formulas being identified within the literature(Reference Sabounchi, Rahmandad and Ammerman6). The equations of Schofield(Reference Schofield7), Henry(Reference Henry8), Mifflin–St Jeor(Reference Mifflin, St Jeor and Hill9), Harris–Benedict(Reference Harris and Benedict10) and Owen(Reference Owen, Kavle and Owen11,Reference Owen, Holup and Dalessio12) are all widely used. There have been several studies evaluating prediction equation accuracy over the years, including investigations in European(Reference Weijs, Kruizenga and van Dijk13–Reference Ruiz, Ortega and Rodriguez17) and US populations(Reference Weijs, Kruizenga and van Dijk13,Reference Flack, Siders and Johnson18–Reference Frankenfield20) , but regular revalidation and updating of recommendations remain important due to changing demographics of modern populations, as well as changes in body composition. More specifically, in recent years the European Food Safety Authority recommended the Henry equation for predicting RMR in all European Union countries(21), and it is recommended for use by UK dietitians(Reference Todorovic and Micklewright22), yet its predictive accuracy still needs to be established within the current population(23), particularly within studies across BMI categories. The purpose of the present study was to evaluate the validity of several RMR prediction equations, by comparing RMR measured by indirect calorimetry with RMR predicted by equation, in a group of healthy women with varying BMI.
Methods
Participants
The present study is a secondary analysis of data collected from healthy adult women across energy balance research studies performed in the Human Nutrition Unit at the University of Glasgow between 2008 and 2017(Reference Alfheeaid, Gerasimidis and Nastase24–Reference Thom, Dombrowski and Brosnahan29). Participants (n 125; aged 20–57 years; BMI 17–44 kg/m2) were sedentary, non-smokers, not pregnant, not taking medication which could affect RMR and had maintained stable body weight for at least 2 months prior to study enrolment. They were asked to avoid exercise in the 24 h prior to testing. Only the baseline RMR values measured prior to participation in the interventions were considered for the present study. Four studies were approved by the College of Medical, Veterinary and Life Sciences of the University of Glasgow Ethics Committee(Reference Alfheeaid, Gerasimidis and Nastase24–Reference Barwell, Malkova and Leggate28), and the other by the West of Scotland NHS Ethics Committee(Reference Thom, Dombrowski and Brosnahan29). Studies were conducted in accordance with the Declaration of Helsinki, and all participants recruited provided written informed consent.
Data collection
Participants reported to the metabolic investigation unit following a 12 h overnight fast. Height was measured to the nearest 0⋅1 cm using a stadiometer (Seca). Body weight was determined by bioelectrical impedance scales (TBF-300; Tanita), and values obtained were incorporated within prediction equations for estimation of RMR. Participants rested for 20 min prior to RMR measurement, which took place in a thermoneutral environment between 07.00 and 10.00 hours to ensure that fasting periods were not extended for too long during daytime hours. Indirect calorimetry was conducted using a calibrated open-circuit ventilated hood system, Oxycon Pro (Jaeger GmbH) or Deltatrac Metabolic Monitor (Datex Engstrom). RMR was obtained in sixty-six subjects using Deltatrac and fifty-nine subjects using Oxycon Pro. Both devices were calibrated prior to the measurements and alcohol-burning validation tests were conducted weekly on Oxycon Pro during testing periods. The measurements involved recording the rate of oxygen consumption (V˙O2) and carbon dioxide production (V˙CO2) every 30 s for the duration of the 20-min test period with participants lying in a supine position whilst fully awake. To ensure that steady-state conditions were obtained(Reference Compher, Frankenfield and Keim30), data from the first 5 min were discarded and V˙O2 and V˙CO2 measured during the following 15-min period were required to have a CV ≤10 % to be accepted for the calculation of RMR, which was achieved using the following indirect calorimetry equations, with reference to Frayn(Reference Frayn31):
Rate of fat oxidation (g/min) = (VO2, litres/min – VCO2, litres/min)/0⋅57
Rate of carbohydrate oxidation (g/min) = (1⋅4 × VCO2, litres/min – VO2, litres/min)/0⋅3
RMR (kcal/min) = (rate of fat oxidation × 9 kcal) + (rate of carbohydrate oxidation × 4 kcal)
RMR (kcal/24 h) = RMR (kcal/min) × 1440 (min)
(To convert kcal to kJ, multiply by 4·184.)
Prediction equations
Of the many prediction equations that have been published in the literature, those of Harris–Benedict, Henry, Mifflin–St Jeor, Owen and Schofield are amongst the most widely used, either currently or in the recent past. Therefore, these equations were used in the present study to predict RMR and compared against measured RMR by indirect calorimetry. The RMR equations, and the populations from which they were derived are described in Table 1.
Statistical and data analysis
Accuracy of the five prediction equations was assessed at both group and individual levels. Results are presented as mean values and standard deviations. At group level, bias (predicted – measured RMR) was assessed by one-sample t test. The 95 % limits of agreement were calculated as the mean of the two values, ± 1⋅96 sd. Root-mean-square error was calculated to show the sample standard deviation of differences between predicted and measured RMR. Agreement between predicted and measured values was assessed by Bland–Altman analyses(Reference Bland and Altman32). Slopes and intercepts of bias as a function of average RMR (mean of predicted and measured RMR) were calculated by regression analyses. At the individual level, RMR prediction was considered accurate if it was within ± 10 % of measured RMR, which is a standard approach for investigating accuracy of RMR prediction equations(Reference Frankenfield, Roth-Yousey and Compher2) and in line with recommendations for conducting this type of research(Reference Madden, Mulrooney and Shah33). When predicted RMR was <90 and >110 % of measured RMR, it was considered as an underestimation and an overestimation, respectively. Accuracy was calculated for the whole group and separately by BMI category: underweight, healthy weight, overweight and obesity. Predictors (age, height and BMI) of the bias were investigated using univariate and multivariate regression analysis. Results were considered statistically significant if P < 0⋅05. Data were analysed in Minitab 17 and MedCalc 17.2.
Results
Descriptive data of the study participants are shown in Table 2. Of the 125 participants included in the analysis, mean age was 30⋅7 (sd 8⋅8) years (range 20–57 years), body weight 70⋅8 (range 41⋅0–120⋅9) kg and BMI 26⋅1(sd 6⋅6) kg/m2 (range 16⋅8–44⋅4 kg/m2). Combined overweight and obesity prevalence was 58 %.
Table 2. Participant characteristics
(Mean values and standard deviations)

Comparison of overall performance of the prediction equations is summarised in Table 3. At group level, the difference between predicted and measured RMR (bias) was not significant for the Mifflin–St Jeor and Henry equations, but for the Harris–Benedict, Schofield and Owen equations, predicted RMR values were significantly different from measured. The Mifflin–St Jeor and Henry equations were most accurate on an individual basis, and predicted RMR was within 10 % of measured values in 71 and 66 % of the participants, respectively. Accuracy for the Schofield, Harris–Benedict and Owen equations was 63, 61 and 59 %, respectively. Where loss of accuracy was observed, underpredictions were most common for the Owen equation, whereas overpredictions occurred more frequently for the other equations. Root-mean-square error data provided additional evidence of greater individual level accuracy for both the Mifflin–St Jeor and Henry equations.
Table 3. Evaluation of prediction equation accuracy in comparison with RMR measured by indirect calorimetry†
(Mean values and standard deviations; limits of agreement (LOA); percentages)

REE, resting energy expenditure; P-M, predicted RMR minus measured RMR; RMSE, root-mean-square error calculated as √(Σ(actual RMR – predicted RMR)2)/n.
* Mean value was significantly different from zero (P < 0⋅05).
† To convert kcal to kJ, multiply by 4·184.
‡ Percentage of participants with predicted RMR within 5 % of measured RMR.
§ Percentage of participants with predicted RMR within 10 % of measured RMR.
ǁ Percentage of participants with predicted RMR being more than 10 % below measured RMR.
¶ Percentage of participants with predicted RMR being more than 10 % above measured RMR.
Fig. 1 shows the Bland–Altman plots which display differences in RMR measured by indirect calorimetry and predicted using the five equations. Limits of agreement were wide for all equations, ranging from an upper limit of +384 kcal/d (+1607 kJ/d) to a lower limit of −443 kcal/d (–1854 kJ/d) for the Harris–Benedict and Owen equations, respectively. For all Bland–Altman plots, slopes and intercepts, calculated from regression analyses between average RMR (mean of predicted and measured RMR) and the bias, were significantly different from zero. The slopes ranged between −6⋅8 (Owen) and −0⋅2 (Henry) and were negative for the equations.

Fig. 1. Bland–Altman plots of differences in RMR measured by indirect calorimetry and predicted using five different equations in 125 adult women. The solid line represents the mean difference (predicted – measured RMR). Upper and lower dashed lines represent the 95 % limits of agreement (±2 sd). The regression line indicates the difference between predicted and measured RMR, plotted against the mean. REE, resting energy expenditure. * To convert kcal to kJ, multiply by 4·184.
For each equation, the percentage of accurate RMR predictions was assessed for each BMI category separately and results are presented in Fig. 2. In women who were underweight, estimation accuracy was lowest with ≤55 % of predictions being within 10 % of measured RMR. In women who were of healthy weight, prediction accuracy was better for the Schofield, Mifflin–St Jeor, Henry and Owen equations, with all equations achieving approximately 73 % accuracy, but the Harris–Benedict equation predicted RMR accurately in only 59 %. Prediction accuracy was higher for all equations in women who were overweight, ranging from 69 to 76 %. In women with obesity, the Mifflin–St Jeor equation was highly accurate and predicted RMR within 10 % of measured RMR in 80 % of cases. Accuracy rate was lower for all other equations, ranging from 40 to 63 %.

Fig. 2. Percentage of adult women for whom RMR predicted by Schofield (■), Owen (□), Mifflin–St Jeor (), Henry (
) and Harris–Benedict (
) equations was within ± 10 % of RMR measured by indirect calorimetry, according to BMI category (underweight, BMI <18 kg/m2; healthy weight, BMI ≥18⋅5–24⋅9 kg/m2; overweight, BMI ≥25–29⋅9 kg/m2, and obesity BMI ≥30 kg/m2).
Table 4 shows predictors of the bias between estimated and measured RMR, based on multivariate linear regression analysis. Increasing age, height and BMI were significant predictors of RMR under- or overestimation, but this effect varied with all equations and collectively explained only a small proportion of the variance in estimation bias (maximum adjusted R 2 15 %). Thus, at least 85 % of the estimation bias was explained by other factors. In additional regression analyses, we found no difference in absolute and percentage prediction error between the Oxycon Pro and Deltatrac metabolic carts.
Table 4. Predictors of the difference between estimated and measured RMR based on univariate and multivariate linear regression†
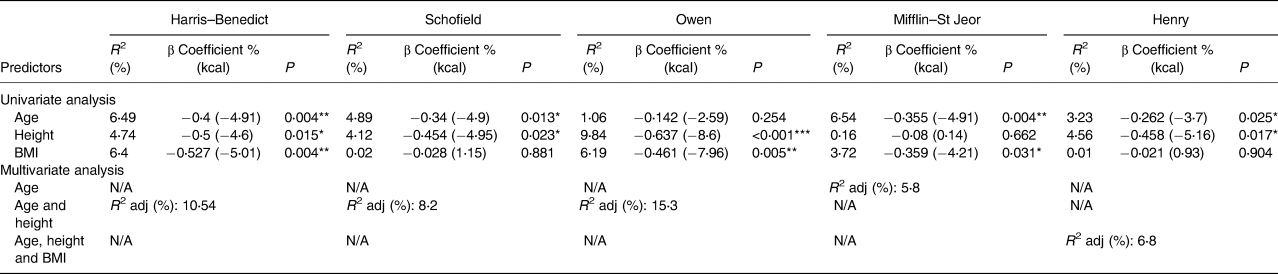
N/A, not applicable; adj, adjusted.
* P < 0⋅05, ** P < 0⋅01, *** P < 0⋅001.
† To convert kcal to kJ, multiply by 4·184.
Discussion
Different methods for determining RMR are not likely to agree exactly, but whether differences in accuracy are likely to affect patient management is an important clinical consideration. In the present study conducted on healthy females with varying BMI, there was considerable room for improvement in the performance of each of the prediction equations at individual-level assessment, though good agreement between measured and predicted RMR was observed at group level using the Mifflin–St Jeor and Henry equations. Although our data suggest that the most accurate prediction of RMR can be expected from using the Mifflin–St Jeor equation, we note that in nearly one-third of participants predicted RMR was outwith 10 % of measured RMR. The wide limits of agreement and slopes and intercepts being different from zero in the regression from Bland–Altman analysis for Mifflin–St Jeor, and indeed for all equations, suggest that there was an asymmetrical relationship in the bias and that RMR prediction at individual level should be interpreted with caution and considered only as a starting point for planning dietary interventions. The findings of the present study also imply that in healthy women at the extremes of BMI (underweight/obesity), and therefore with relatively low or high measured RMR, the validity of prediction equations in general is particularly poor, with the one exception being when applying the Mifflin–St Jeor equation to women with obesity. This is of concern given that underweight and obesity are clinical situations where equations are frequently used in practice. Despite relatively poor accuracy at the individual level, the equations investigated in the present study can be expected to operate well at population level to assist governments in planning and monitoring nutrition programmes.
In this dataset, the Mifflin–St Jeor equation performed best in women with healthy weight, overweight and obesity, predicting RMR accurately in 73, 76 and 80 % of the participants, respectively. Our findings that Mifflin–St Jeor is the most accurate prediction equation, especially for women with obesity, is in line with evidence from systematic reviews which recommend the Mifflin–St Jeor equation for use in individuals with overweight and obesity, and also report a similar number of inaccurate predictions(Reference Frankenfield, Roth-Yousey and Compher2,Reference Madden, Mulrooney and Shah33) . These findings imply that the Mifflin–St Jeor equation should not necessarily be restricted to women with obesity, since it was also as accurate as other equations for estimating RMR in underweight, healthy-weight and overweight women. It is perhaps not surprising that the Mifflin–St Jeor equation provided the most consistent accuracy across BMI categories since it was developed in a large sample of individuals with healthy weight, overweight and obesity(Reference Mifflin, St Jeor and Hill9). Although predicted RMR values do in part reflect the characteristics of the population they were derived from, and thus can lack specificity when applied to individuals different from the original population(Reference Carpenter, Pencharz and Mouzaki34,Reference Miller, Milliron and Woolf35) , our data suggest that population-specific factors play a relatively minor role in influencing prediction of RMR, and the Mifflin–St Jeor equation has demonstrated the best accuracy in several other studies using differing populations(Reference Weijs, Kruizenga and van Dijk13,Reference Frankenfield20,Reference Dobratz, Sibley and Beckman36) . The ± 10 % ‘rule’ for defining prediction accuracy is a pragmatic criterion which facilitates comparison between studies and is clinically relevant since chronic energy imbalances are likely to contribute to cases of malnutrition, or, conversely, to overweight and obesity. Due to the large number of confounding factors that exist, it is difficult to show clear links between adverse clinical outcomes and the selected cut-offs for under- and overfeeding (<90 or >110 % of energy requirements); however, respiratory and liver dysfunction, poor wound healing and infections, azotemia and renal failure, and total complication rate have been associated with under- and/or overfeeding in critically ill patients(Reference Berger and Pichard37–Reference Dvir, Cohen and Singer42). Furthermore, prescription of higher (or lower) than intended energy deficits to people seeking to correct or ameliorate overweight and obesity-related complications are likely to result in poorer adherence and weight-loss outcomes(Reference Frost, Masters and King43).
The work of Schofield and colleagues(Reference Schofield7) was influential in re-establishing the use of RMR rather than reported food consumption as the basis for estimating energy requirements(Reference Henry8), and was used to inform the development of dietary reference values for children and adults(44). The Schofield equations were widely used by dietitians in clinical practice(Reference Judges, Knight and Graham45) until relatively recently, but key advisory groups now recommend using the Henry equation, as the older Schofield equation overestimates RMR(21–23). The newer Henry equation addressed some of the limitations of the Schofield equation by including data collected on greater numbers of people from tropical climates, and a large cohort of young, physically active Italian subjects were excluded who were found to have higher BMR per kg/body weight than other Caucasian groups(Reference Henry8). We found no difference between RMR measured by indirect calorimetry and predicted RMR using the Henry equation at group level and 66 % of predictions were within 10 % of measured RMR. Thus, the Henry equation predicts RMR more accurately than the Schofield, Harris–Benedict and Owen equations at group and individual levels, though overall the Mifflin–St Jeor equation is marginally more reliable than the Henry equation for prediction of RMR at the individual level. This is approximately agreeable with a study involving 239 US adults, which showed that the Henry equation predicted RMR accurately in 74 % of individuals, whilst the Mifflin–St Jeor equation provided accurate RMR predictions in 79 % of individuals; however, both equations performed poorer in a Dutch cohort(Reference Weijs, Kruizenga and van Dijk13).
In the present analysis, all equations except Schofield demonstrated a tendency to systematically over- or underestimate RMR in individuals with relatively higher or lower RMR, which is in line with findings reported elsewhere(Reference Jesus, Achamrah and Grigioni46). This has implications for the lightest- and heaviest-weighing subjects in this sample, and therefore, is likely to affect those with low and high BMI. The number of underweight participants in this analysis was modest though prediction accuracy was low in underweight women for all equations, with the Harris–Benedict equation being particularly susceptible to error. Systematic overestimation of RMR was found in women with low RMR and thus most likely in those with low BMI, which would suggest that overestimation rather than underestimation of RMR can expected in underweight women. Although our findings should be interpreted with caution given the small numbers of underweight subjects, a study containing a larger underweight population showed even poorer prediction accuracy than in our dataset(Reference Rising, Keys and Ravussin47). This might be because fewer underweight individuals participated in the original development and validation of equations, or that anthropometric measurements and demographic characteristics are unable to predict the majority of variance in RMR measurements in this group.
In the regression analyses, very little of the prediction error was explained by age, height or BMI. This may partly be explained by factors not investigated within this analysis which influence RMR, including body temperature(Reference Rising, Keys and Ravussin47), sympathetic nervous system activity(Reference Spraul, Ravussin and Fontvieille48), circadian and menstrual rhythms(Reference Weekes49), thyroid function(Reference Muller, Bosy-Westphal and Kutzner5), ethnicity(Reference Sharp, Bell and Grunwald50) and genetic factors(Reference Konarzewski and Ksiazek51,Reference Bogardus, Lillioja and Ravussin52) . These factors are also unable to be factored into prediction equations, and this underlies their inaccuracy at the individual level for a proportion of individuals.
A strength of the present study was that we had data available for large numbers of females across BMI categories, meaning we generalise findings to women though we make no assumptions regarding accuracy of these equations in males. Although all prediction equations have been derived from healthy populations, they are commonly applied in hospital settings where energy requirements of patients are altered by physiological stress imposed by disease state and factors such as surgery, infection, inflammation and changes in body composition(Reference Todorovic and Micklewright22,Reference Weekes49) . However, it should be appreciated that this evaluation was conducted in healthy women and overgeneralising to other populations should be avoided. Predictions of RMR and energy requirements of individuals incur errors using all equations, so careful monitoring of body-weight changes remains paramount to assess whether nutritional targets are being met(Reference Weekes49). It has been reported that validity of RMR prediction equations can be influenced by the accuracy of the reference method (indirect calorimetry), with some metabolic carts having insufficient accuracy(Reference Galgani, Castro-Sepulveda and Perez-Luco53) and that RMR is not necessarily concordant between instruments(Reference Schadewaldt, Nowotny and Strassburger54). However, in the present study weekly alcohol burning tests, conducted on Oxycon Pro produced CV of ≤1⋅7 and Deltatrac is considered to exhibit the greatest accuracy of all metabolic carts. Furthermore, in regression analyses, accounting for the confounding effect of BMI and age, no difference was found in absolute or percentage prediction error between the two devices for obtaining RMR.
There are some limitations to this analysis. For reasons of feasibility and generalisability, we evaluated RMR prediction equations which used only routine anthropometric variables. Aggregate predictions of RMR, incorporating numerous equations, have been shown to significantly improve accuracy and reduce potential for selecting the worst-performing equation for an individual(Reference Wells, Williams and Haroun55). A further alternative might be to develop BMI-specific prediction equations, which were superior when compared with generic equations in a large German population, particularly in underweight individuals, with no additional benefit of including FFM in equation design(Reference Mueller, Bosy-Westphal and Klaus14). Incorporating additional, simple anthropometric variables (for example, waist and hip circumference) within prediction equations may also improve accuracy(Reference Hedayati and Dittmar56). More affordable hand-held calorimeters are becoming available and may provide a more accurate alternative to prediction equations(Reference Hipskind, Glass and Charlton57), though others have reported that these machines have limited utility and traditional metabolic carts should remain the preferred method for estimating nutritional requirements where feasible(Reference Anderson, Sylvia and Lynch58).
In conclusion, the equation of Mifflin–St Jeor is the most reliable for predicting RMR, closely followed by the Henry equation, though clinically relevant errors can still be expected in about one-third of individuals, most commonly in individuals with obesity, or who are underweight. Although there is room for improvement, prediction equations do continue to provide convenient estimations of RMR and a starting point for estimating daily energy requirements and developing dietary interventions.
Acknowledgements
We would like to thank all of our volunteers who took part in the research studies contributing to this paper.
The present study received no financial support from any organisation.
G. T., D. M. and K. G. conceived the study. G. T. and D. M. drafted the manuscript. K. G. and G. T. performed the statistical analyses and data interpretation. G. T., E. R., H. A., E. M., S. F. and N. B. recruited study participants and contributed to data collection. All authors critically reviewed and contributed to the final version of the manuscript.
There are no conflicts of interest.