INTRODUCTION
With the significant growth in the population aged 65 years and older, the number of Americans with Alzheimer’s disease (AD) and other dementias is projected to increase from 58 million in 2018 to 88 million by 2050 (Alzheimer’s Association, 2018). As these figures grow, disparities have emerged in the prevalence of AD. As compared to non-Hispanic Whites, Black adults have higher rates of dementia per capita and are approximately twice as likely to have AD or other dementias (Gurland et al., Reference Gurland, Wilder, Lantigua, Stern, Chen, Killeffer and Mayeux1999; Potter et al., Reference Potter, Plassman, Burke, Kabeto, Langa, Llewellyn and Steffens2009). There are also considerable barriers to treatment; Black adults are diagnosed later in the disease (Chin, Negash, & Hamilton, Reference Chin, Negash and Hamilton2011) and are less likely to receive antidementia medications (Zuckerman et al., Reference Zuckerman, Ryder, Simoni-Wastila, Shaffer, Sato, Zhao and Stuart2008) as compared to non-Hispanic Whites. This evident disparity that places Black adults at higher risk for developing AD and barriers to treatment has been attributed to social and behavioral determinants of health: perceived discrimination and environmental stress (Zahodne, Sol, & Kraal, Reference Zahodne, Sol and Kraal2019); a higher incidence of comorbid modifiable health risks (i.e., hypertension, cardiovascular disease, diabetes; Brancati et al., Reference Brancati, Kao, Folsom, Watson and Szklo2000; Sundquist, Winkleby, & Pudaric, Reference Sundquist, Winkleby and Pudaric2001; Cushman et al., Reference Cushman, Cantrell, McClure, Howard, Prineas, Moy, Temple and Howard2008); and insensitive assessment related to years of education, educational quality, and literacy (Manly, Schupf, Tang, & Stern, Reference Manly, Schupf, Tang and Stern2005; Manly, Touradji, Tang, & Stern, Reference Manly, Touradji, Tang and Stern2003).
Due to the increased prevalence of dementia and importance of early detection for possible intervention, there remains a critical need for assessments that are sensitive to preclinical signs of AD, especially among persons at high risk. Towards this goal, assessments that are sensitive to identify mild cognitive impairment (MCI) may be useful for clinical diagnosis and research in the development of interventions to promote cognitive resiliency. MCI is often a preclinical stage of AD, and individuals with MCI are more likely to develop AD or other dementias (Petersen et al., Reference Petersen, Lopez, Armstrong, Getchius, Ganguli, Gloss and Rae-Grant2018). MCI clinical diagnostic criteria include cognitive impairments that are not normal for the individual’s age, but not yet severe enough to cause significant impairment in instrumental activities of daily living (Winblad et al., Reference Winblad, Palmer, Kivipelto, Jelic, Fratiglioni, Wahlund and Petersen2004). MCI has been divided into subtypes based on the number of affected cognitive domains and whether memory is affected, that is, single or multidomain amnestic (aMCI) and nonamnestic (naMCI) subtypes. An estimated 15.8% of adults aged 60 and older who are residing in the USA are diagnosed with some subtype of MCI (Petersen et al., Reference Petersen, Lopez, Armstrong, Getchius, Ganguli, Gloss and Rae-Grant2018), and there is evidence suggesting a higher prevalence of naMCI among Black adults as compared to non-Hispanic Whites (Lopez, Jagust, DeKosky et al., Reference Lopez, Jagust, DeKosky, Becker, Fitzpatrick, Dulberg and Kuller2003; Katz et al., Reference Katz, Lipton, Hall, Zimmerman, Sanders, Verghese, Dickson and Derby2012).
Despite the increased risk posed to Black adults for developing AD, Black adults are largely underrepresented in the research seeking to understand or treat these conditions. Out of 10 of the most prominent biomarker studies on AD in the USA, half did not achieve adequate samples of Black adults required to determine racial/ethnic differences effectively (Shin & Doraiswamy, Reference Shin and Doraiswamy2016). It has been proposed that community-based approaches to recruitment which focus on building trust in the research process involve, for example, researchers of the same ethnicity as the participants and use visit locations and timing that are convenient for the participants to reduce the burden of participation (Gilmore-Bykovskyi et al., Reference Gilmore-Bykovskyi, Jin, Gleason, Flowers-Benton, Block, Dilworth-Anderson and Zuelsdorff2019). These methods have been proposed as ways to help reduce both selection and sampling bias when attempting to evaluate differing rates of disease progression across racial/ethnic groups in large AD center datasets (Gleason et al., Reference Gleason, Norton, Zuelsdorff, Benton, Wyman, Nystrom and Asthana2019). Community-based assessment can also be facilitated by computer administration of cognitive tests due to the ease of accessibility, transportability, and the reduced amount of time and effort required to complete such assessments (Hinton et al., Reference Hinton, Carter, Reed, Beckett, Lara, DeCarli and Mungas2010). Limited research suggests that older Black adults are more highly satisfied with computerized neuropsychological assessment than paper–pencil methods and that individual levels of familiarity and comfort with computers do not affect performance on assessments of cognition (Gamaldo et al., Reference Gamaldo, Tan, Sardina, Henzi, Guest, Ross and Andel2018). While this highlights the strengths of a computerized assessment that can be used in the community, the validity and sensitivity of these methods to identify individuals with MCI- or AD-related cognitive symptoms, specifically among Black adults, have not been thoroughly evaluated.
Two computerized cognitive assessment batteries that are increasingly being used in clinical research are the Cogstate Brief Battery (CBB; Hammers et al., Reference Hammers, Spurgeon, Ryan, Persad, Barbas, Heidebrink and Giordani2012; Hammers et al., Reference Hammers, Spurgeon, Ryan, Persad, Heidebrink, Barbas and Giordani2011; Maruff et al., Reference Maruff, Lim, Darby, Ellis, Pietrzak, Snyder and Masters2013) and the NIH Toolbox-Cognition Battery (NIHTB-CB; Heaton et al., Reference Heaton, Akshoomoff, Tulsky, Mungas, Weintraub, Dikmen and Gershon2014; Weintraub et al., Reference Weintraub, Dikmen, Heaton, Tulsky, Zelazo, Bauer and Gershon2013). These measures have demonstrated acceptable reliability and construct validity as compared to traditional paper–pencil methods (Casaletto et al., Reference Casaletto, Umlauf, Beaumont, Gershon, Slotkin, Akshoomoff and Heaton2015; Heaton et al., Reference Heaton, Akshoomoff, Tulsky, Mungas, Weintraub, Dikmen and Gershon2014) but lack clear support as a replacement for gold-standard neuropsychological assessment (Scott, Sorrell, & Benitez, Reference Scott, Sorrell and Benitez2019). Although there has been significant effort put forth to develop representative normative samples for these measures, there continues to be a lack of knowledge around the performance on these two cognitive batteries by individuals from underrepresented populations. For example, two of the largest studies on the psychometric properties of the CBB make no mention of the race/ethnicity of their samples (Maruff et al., Reference Maruff, Lim, Darby, Ellis, Pietrzak, Snyder and Masters2013; Mielke et al., Reference Mielke, Machulda, Hagen, Edwards, Roberts, Pankratz and Petersen2015), pointing to a lack of information regarding the use of these measures in ethnically diverse populations. With regard to the NIHTB-CB, the original normative data were unadjusted for demographic factors, with subsequent normative samples providing corrections for race/ethnicity (Casaletto et al., Reference Casaletto, Umlauf, Beaumont, Gershon, Slotkin, Akshoomoff and Heaton2015); however, it has been found that the NIHTB-CB may overestimate such individuals’ deficits (Scott et al., Reference Scott, Sorrell and Benitez2019) and more research is needed to validate the clinical utility of this measure. Overall, there remains a fundamental gap in our understanding of the utility of these computerized cognitive batteries for use in clinical research trials including Black adults.
The present study was designed to evaluate the convergent validity of the CBB and NIHTB-CB with the current gold-standard consensus diagnosis process from the National Alzheimer’s Coordinating Center (NACC) and the Uniform Data Set (UDS). The aim of the study was to determine which individual subtests from these computerized cognitive measures would reflect the greatest difference between MCI and healthy controls (HC) in a sample of community-dwelling Black adults. Based on typical cognitive symptoms of AD and related dementia, we hypothesized that measures of fluid ability (i.e., declarative memory, executive functioning, attention, and processing speed) from the CBB and NIHTB-CB would most strongly differentiate between diagnosis groups as compared to measures of crystallized ability (e.g., vocabulary).
METHOD
Participants
All participants consented to participate in the research study, and procedures were approved by the University of Michigan Medical School Review Board (IRBMED) and/or Wayne State University Research Subjects Review Board. Participants were 113 community-dwelling Black adults aged 56–90 years with self-reported cognitive complaints and no previous neurocognitive diagnosis. Participants were enrolled based on their responses to a question asking if they had experienced “a change in memory or other cognitive areas over the past year, but not so severe as to interfere with ability to complete daily activities.” Recruitment was conducted through the Wayne State University, Michigan Center for Urban African American Aging Research—Healthier Black Elders Center, and the Michigan Alzheimer’s Disease Research Center (Michigan ADRC). All participants completed the NACC-UDS evaluation, a multidomain medical, neurological, social, and neuropsychological evaluation used as the basis for consensus conference diagnostic procedures (Rahman-Filipiak, Giordani, Heidebrink, Bhaumik, & Hampstead, Reference Rahman-Filipiak, Giordani, Heidebrink, Bhaumik and Hampstead2018; Weintraub et al., Reference Weintraub, Besser, Dodge, Teylan, Ferris, Goldstein and Morris2018; Weintraub et al., Reference Weintraub, Salmon, Mercaldo, Ferris, Graff-Radford, Chui and Morris2009). Participants also completed the NIHTB-CB and CBB within 3 months of their NACC-UDS assessment, the results of which were not used as part of the consensus conference diagnostic process. Patients were excluded if they suffered from a significant psychiatric, medical, or neurological deficit other than AD that may impair cognitive ability. Those with physical limitations which precluded completion of neuropsychological measures were excluded. Nine participants were excluded from the analyses as they did not meet MCI diagnostic criteria based on NACC-UDS criteria but were judged to be cognitively impaired (impaired but not MCI). These criteria parallel the MCI criteria published by the 2011 National Institute of Health and Alzheimer’s Association workgroup on MCI and AD (Albert et al., Reference Albert, DeKosky, Dickson, Dubois, Feldman, Fox and Phelps2011). The final sample for analysis included 61 HC and 43 MCI patients (n = 29 aMCI and n = 14 naMCI), which were majority multiple domains (Table 1).
Assessment Measures
NACC-UDS Neuropsychological Battery
The NACC-UDS neuropsychological battery was initially developed in 2005 and recently underwent a third revision (Weintraub et al., Reference Weintraub, Besser, Dodge, Teylan, Ferris, Goldstein and Morris2018). The NACC-UDS has been implemented by the National Institute on Aging Alzheimer Disease Centers nationally since its inception, and has been described extensively before (Weintraub et al., Reference Weintraub, Salmon, Mercaldo, Ferris, Graff-Radford, Chui and Morris2009). Briefly, the neuropsychological battery, which is used for case-consensus diagnosis, includes measures of dementia severity, learning and memory, vocabulary and reading skills, verbal fluency, processing speed, executive functioning, and visuospatial perception and memory. In the present study, we included data from the NACC-UDS version two, with supplemental data including the Wide Range Achievement Test 4th edition, reading subtest (WRAT-IV; Wilkinson & Robertson, Reference Wilkinson and Robertson2006) and the Wisconsin Card Sorting Test (WCST; Heaton, Reference Heaton1993).
NIH Toolbox-Cognition Battery
The NIHTB-CB is a multidimensional measure comprised of seven subtests and provides individual subtest performances as well as composite summary scores of crystallized cognitive abilities, fluid cognition, and total cognition. The crystallized cognition composite includes the Oral Reading Recognition (ORR) and Picture Vocabulary (PV) subtests. Measures of fluid abilities include the Dimensional Change Card Sort (DCCS) task, Flanker Inhibitory Control and Attention (FICA), List Sorting Working Memory (LSWM), Pattern Comparison Processing Speed (PCPS), and Picture Sequence Memory (PSM) subtests. The specific test details, procedures, and extensive psychometric evaluation are available elsewhere (Weintraub et al., Reference Weintraub, Dikmen, Heaton, Tulsky, Zelazo, Bauer and Gershon2013). The DCCS test assesses set shifting and requires participants to match a target stimulus to one of two choice stimuli according to shape and color. The FICA task tests an individual’s ability to inhibit visual attention to task-irrelevant dimensions. The LSWM test involves individual presentation of a series of stimuli and requires the participant to repeat them in order from smallest to biggest by category and size. The PSM test is a measure of episodic memory based on ordering pictures in sequence. The ORR test measures participant’s ability to pronounce single printed words out loud, and recognize letters. The PV test assesses language by asking participants to pick a picture that matches a spoken word. Finally, the PCPS test requires participants to identify whether two visual patterns are the same or different. In our dataset, all resultant subtest scores were fully adjusted for age, sex, and education. Summary scores were not included in the analyses.
Cogstate Brief Battery
The CBB is a computerized cognitive assessment that provides measures of four different cognitive domains using playing card paradigms: visual learning, working memory, processing speed, and attention. These separate tests and their psychometric properties have been described previously (Falleti, Maruff, Collie, & Darby, Reference Falleti, Maruff, Collie and Darby2006; Lim et al., Reference Lim, Jaeger, Harrington, Ashwood, Ellis, Stöffler and Maruff2013; Maruff et al., Reference Maruff, Lim, Darby, Ellis, Pietrzak, Snyder and Masters2013). Briefly, the core tests include the Detection Task (DET), a simple reaction time task, the Identification Task (IDN) which is a choice reaction time test of visual attention, the One Card Learning Task (OCL), a continuous visual recognition learning task, and the One Back Task (ONB), a test of working memory. We included data on both accuracy (correct vs. incorrect responses) and reaction time (in milliseconds) in our analyses.
Computer Anxiety
Computer anxiety was measured using the Wild et al. (Reference Wild, Mattek, Maxwell, Dodge, Jimison and Kaye2012) Computer Anxiety Survey, a 16-item measure on which participants rate their level of anxiety when using computers (e.g., “I feel relaxed when I am working on a computer”). Responses are rated on a five-point, Likert-type scale and range from “Strongly Disagree” to “Strongly Agree.” Total scores range from 16 to 80, with higher scores indicating greater levels of computer anxiety. Computer anxiety summary scores were derived by totaling rating for each item (Wild et al., Reference Wild, Mattek, Maxwell, Dodge, Jimison and Kaye2012).
Statistical Analysis
Hypotheses were tested with a discriminant function analysis (DFA). DFA is a data-driven approach that benefits from a priori selection of variables to describe multivariate differences between groups, but is agnostic to the relative importance of each variable. A discriminant function is a composite that describes observed between-group variance by a set of predictor variables. The procedure estimates a number of discriminant functions equal to the minimum degrees of freedom; combined, the functions account for all of the between-group variance and the first function accounts for the largest proportion. In analysis of two groups, a single function accounts for all of the between-group variance. A significant model indicates a reliable difference between groups, and the proportional variance explained by each function is further evaluated. Each predictor variable has a standardized loading in the structure matrix, that, is its unique contribution to the discriminant function; absolute values at least 0.30 are considered meaningful by convention (i.e., at least 9% commonality with the other variables to differentiate the groups). Separation of the group centroids on a discriminant function is consistent with between-group differences in that multivariate combination of data. Applying cross-validation procedures, group membership can be predicted from the loadings of each variable across functions, which is used to assess model accuracy. We apply this technique to describe the differences between HC and MCI in terms of performance on NIHTB-CB and CBB tests and to evaluate the unique contribution of each measure in rank order.
Prior to analysis, all measures were screened for univariate and multivariate outliers, and multivariate normality was assessed with visual inspection of Q–Q plots. One case was identified as a statistical outlier on DCCS and DET tasks and no other univariate outliers were observed; as there was no evidence of multivariate outliers and the assumption of normality was reasonably met, this case was included in analysis. In each DFA analysis, all variables were entered in a single step and included control variables: age, sex, years of education, and computer anxiety summary score. Assumed prior probabilities for group classification were computed from frequency of diagnosis observed in the sample. Of the eligible sample, nine participants were missing data from one or more subtests (e.g. computer malfunction) and eight participants fell below recommended CBB minimum accuracy percentage on one subtest (Cogstate, 2020). Data were missing at random (Little’s χ2 (730, N = 104) = 257.18, p = .99); those missing data values were replaced by the sample mean in the DFA procedure.
A preliminary analysis applied DFA to confirm diagnosis that was made by consensus conference of the NACC-UDS test battery. This analysis serves as a demonstration of the method and to validate the approach to test between-group differences with the exploratory technique. In our primary hypothesis test, measures of the NIHTB-CB and CBB were tested as predictors in the DFA that were expected to replicate the pattern of results with the NACC-UDS in the preliminary analysis. Namely, performance on tests of fluid cognitive ability would identify participants with MCI apart from HC. Although combined in the primary analysis, aMCI and naMCI are prodromal stages of different dementias, and therefore we also conducted a supplementary DFA analysis to determine if performances may depend on MCI subtype. Models were assessed for significance of the discriminant function and accuracy of classification with cross-validation. Unique contributions of each variable, controlling for all other variables in the model, were interpreted from rank-order structure matrix coefficients.
RESULTS
Participants diagnosed with aMCI (n = 29) performed equivalent to those with naMCI (n = 14) on all CBB (t = −1.27–1.38, all p’s ≥ .10) and NIHTB-CB tests (t = −1.91–2.97, all p’s ≥ .06), except the Flanker subtest (t (39) = 2.97, p = .01). Due to the low incidence of naMCI observed in this sample and the statistical equivalence to aMCI, primary analyses tested hypotheses with a combined group of aMCI and naMCI, described hereafter as the MCI group, as compared to HC counterparts.
Confirmation of MCI Diagnosis from the UDS
A preliminary analysis applied the DFA method to confirm the diagnosis made by consensus conference when reviewing performance on the NACC-UDS (version 2) neuropsychological battery and ancillary tests (WRAT-IV and WCST). The collective performance on the test battery and control variables significantly described differences between MCI and HC participants: Wilk’s λ = 0.27, χ 2 (27, N = 104) = 55.17, p = .001. Reviewing the structure matrix, discriminating variables included delayed story recall (0.52), immediate story recall (0.40), digit-symbol coding, a measure of processing speed (0.35), and a test of oral word reading (WRAT-IV; 0.30). All other variables made a small independent contribution that fell below the 0.30 threshold. The collection of variables correctly classified 78.8% (63.5% cross-validated) of participants, and cross-validated accuracy was similar for HC (63.9%) and MCI (62.8%). Taken together, performance on declarative memory and executive function tasks in the NACC-UDS, controlling for all other variables in the model, most strongly distinguished MCI apart from HC. Notably, low WRAT-IV reading scores differentiated MCI participants independent of age and education, but it was of lower rank order importance as compared to the other cognitive measures.
Evaluation of Performance on NIHTB-CB and CBB
Repeating the analysis with the NIHTB-CB and CBB batteries, which were not used to make diagnoses, revealed a different pattern of group differences in performance. The model significantly differentiated between groups: Wilk’s λ = 0.56, χ 2 (19, N = 104) = 43.28, p = .001. In rank order, the strongest discriminating variables were NIHTB-CB Oral Reading (0.55) and NIHTB-CB PV (0.51). Accounting for these effects, several measures of declarative memory, learning, and executive function further differentiated groups: NIHTB-CB List Sorting (0.41), CBB One Card Learning accuracy (0.41), NIHTB-CB DCCS (0.38), NIHTB-CB Pattern Comparison (0.36), NIHTB-CB PSM (0.34), CBB One Card Back accuracy (0.31), and reaction time (−0.30). The collective performance on these tests indicated participants with MCI performed worse than HC (Figure 1). All other performance measures and control variables contributed small independent effects that fell below threshold (Table 2). The model correctly classified 77.9% (66.3% cross-validated) of cases. The cross-validated accuracy was lower for MCI classification (55.8%) as compared to HC (73.8%).
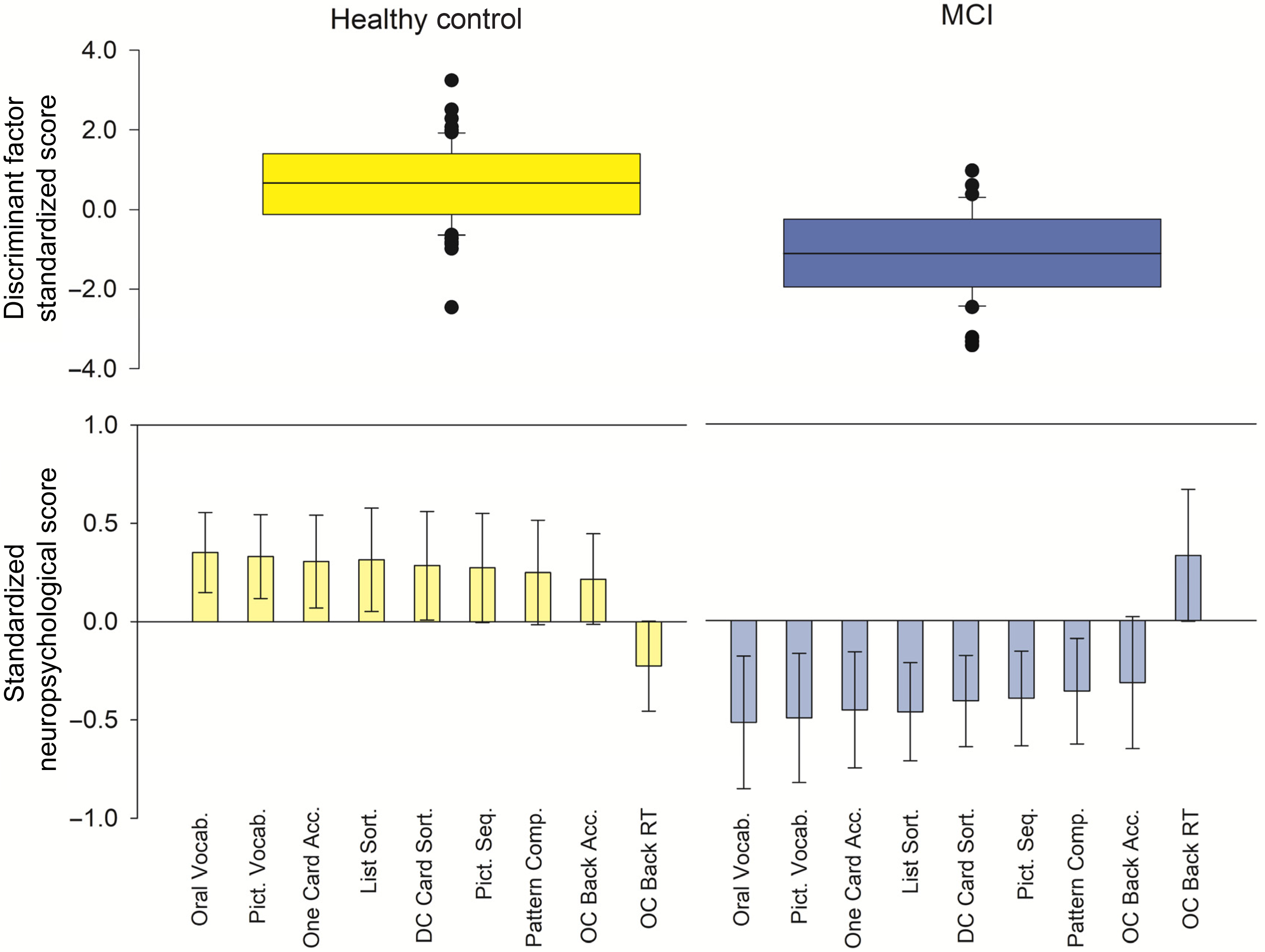
Fig. 1. Multivariate description of performance differences between MCI and HC. Top panel: The discriminant function factor score includes all variables submitted to the DFA. The distribution of scores is shown for HC (yellow) and MCI (blue) groups, including group means (line) and distribution. The model significantly differentiated between groups: Wilk’s λ = 0.56, χ 2 (19, N = 104) = 43.28, p = .001. Bottom panel: The variables that made the strongest, unique contribution to the discriminant function (structure matrix loading > |0.30|), are displayed for each group, including error bars that represent 2 standard errors of the group mean. For the purpose of data visualization, scores were standardized to the sample mean. In rank order, NIHTB-CB Oral Vocabulary/Reading and Picture Vocabulary subtests most strongly differentiated between groups, followed by several tests of declarative memory, learning and executive function. Oral Vocab = NIHTB-CB Oral Reading Recognition score; Pict. Vocab. = NIHTB-CB Picture Vocabulary; One Card Acc. = CBB one card learning accuracy; DC Card Sort = NIHTB-CB dimensional change card sort; Pict. Seq. = NIHTB-CB picture sequence; Pattern Comp. = NIHTB-CB pattern comparison processing; OC Back Acc. = CBB one card learning back accuracy; OC Back RT = CBB one card learning back reaction time. NIHTB-CB scores were fully adjusted for demographic characteristics.
Table 1. Sample description

HC = healthy controls; MCI = combined group amnestic and nonamnestic mild cognitive impairment.
Note: Descriptive statistics are reported for each group as M (SD) and percentage of participants who self-identify as female. Statistical comparisons between groups are reported at t-tests or chi-square statistics, with significance testing * p < .05.
Table 2. Variables that significantly differentiated between MCI and healthy controls

Note: All variables contributed to the significant model. Coefficients are standardized structure matrix scores that identified the discriminant function. Coefficients with an absolute value of at least 0.30 were interpreted; positive coefficients indicate cognitive typical adults scored higher (e.g., greater accuracy) as compared to combined aMCI/naMCI, and negative coefficients indicate lower values (e.g., shorter reaction time). * = scores were fully adjusted for age, sex, and education. NIHTB-CB = NIH Toolbox Cognition Battery; CBB = Cogstate Brief Battery; RT = Reaction time (in milliseconds).
Our supplementary analysis of performances on the NIHTB-CB and CBB with three groups (aMCI, naMCI, and HC) largely replicated the result pattern. With three groups, two discriminant functions were estimated (Wilk’s λ = 0.39, χ 2 (38, N = 104) = 70.36, p = .001), although the second function was not significant beyond the first (Wilk’s λ = 0.72, χ 2 (18, N = 104) = 24.38, p = 0.14). Function 1 was determined by NIHTB-CB PV (0.54), NIHTB-CB Oral Reading (0.46), CBB One Card Learning accuracy (0.41), NIHTB-CB List Sorting (0.37), NIHTB-CB PSM (0.37), and NIHTB-CB DCCS (0.35), and all other variables fell below threshold. The cross-validated classification accuracy was low (53.8%) and poorly identified aMCI (31.0%) and naMCI (14.3%). The pattern of results underscores that NIHTB-CB and CBB tests of declarative memory and executive function differentiated Black adults with MCI as compared to HC, but regardless if amnestic or nonamnestic, NIHTB-CB PV and Oral Reading tests were the strongest unique predictors of MCI.
DISCUSSION
The present evidence indicates that, even when controlling for education and other demographic factors, computerized measures of crystallized intelligence, namely NIHTB-CB measures of Oral Reading and PV skills, were most sensitive to identifying MCI apart from HC in this sample of Black adults as compared to other NIHTB-CB and CBB tasks. Second to these unique effects, other deficits in memory and executive function were predictive of MCI, which was consistent with the expected preclinical symptoms; but, surprisingly, these were not the strongest descriptors of group differences. The NACC-UDS outcomes that were used for clinical diagnosis via case consensus produced the expected pattern of differences between the groups, foremost in memory and executive functioning deficits in individuals with MCI. Therefore, the results from the CBB and NIHTB-CB assessments appear to reflect differences in the cognitive domain assessments when administered via laptop computer. In sum, while reading and vocabulary scores across assessment tools were lower in Black adults with MCI as compared to HC, the computerized assessments produced a pattern of results that was not fully consistent with what is typically expected for diagnosis of MCI and did not fit with the pattern of results observed on the NACC-UDS measures.
Differences in measures of reading ability and vocabulary skills have been shown between individuals with MCI and HC previously. For example, individuals with MCI perform worse than HC on the Peabody Picture Vocabulary Test, a paper–pencil test which is similar to the PV task administered in the NIHTB-CB (Jokel, Seixas Lima, Fernandez, & Murphy, Reference Jokel, Seixas Lima, Fernandez and Murphy2019). Although such vocabulary skills are thought to generally remain stable across the lifespan and represent estimated premorbid cognitive functioning (i.e., crystallized abilities), there is also evidence that performance on the Peabody Picture Vocabulary Test declines with increasing cognitive deficit, such as when comparing individuals with MCI to those with AD (Snitz, Bieliauskas, Crossland, Basso, & Roper, Reference Snitz, Bieliauskas, Crossland, Basso and Roper2000) and could reflect changes in language functioning. The relation of reading ability and literacy to cognitive performance is more complex. Low reading ability may present a bias in verbal assessments of fluid cognitive abilities, for example, NIHTB-CB scores are adjusted for education level. However, it is unclear if a single adjustment can be applied to all persons, regardless of race and ethnicity. In contrast, high educational attainment and literacy may resemble a form of “cognitive reserve”—protective factors which appear to stave off eventual cognitive decline—which may be more predictive of subsequent declines in memory, executive functioning, and language skills than years of education alone (Manly et al., Reference Manly, Schupf, Tang and Stern2005; Manly et al., Reference Manly, Touradji, Tang and Stern2003; Stern, Reference Stern2002; Stern, Reference Stern2006).
The pattern of results we report here suggests that crystallized abilities measured by the NIHTB-CB and CBB are relatively more sensitive than NIHTB-CB and CBB tasks of memory and executive functioning as predictors of MCI in this sample of Black adults. Notably, our sample consisted of individuals with an average of 14 years of education, and all analyses identified years of education as having a negligible, independent effect. In the preliminary analysis of the NACC-UDS supplemental data, WRAT-IV reading scores contributed to differentiating between MCI and HC, which suggests that differences in literacy and educational quality (Dotson, Kitner-Triolo, Evans, & Zonderman, Reference Dotson, Kitner-Triolo, Evans and Zonderman2009) may be relevant when identifying MCI among Black adults, harkening to previous discussions of educational inequality in the USA (Baker, Johnson, Velli, & Wiley, Reference Baker, Johnson, Velli and Wiley1996). However, this alone cannot account for the unexpected pattern of results observed in the computerized test batteries. First, the rank order importance of WRAT-IV reading scores was lower than performance on declarative memory and executive function, as would be expected for identifying persons with MCI. Second, the equivalent measures in the NIHTB-CB (Oral Reading and PV) were found to be the strongest unique effects to identify MCI apart from HC, even after scores were adjusted for education and race/ethnicity following standardized procedures. One plausible interpretation of this result is the examined tests are relatively more sensitive to reading ability, which may present as a source of bias when assessing MCI. Alternatively, it may be the case that these computerized measures are relatively less sensitive to memory ability than the NACC-UDS measures, leading to the observed pattern of findings on those measures. These unexpected differences should be evaluated in future studies.
Another interpretation is the possibility that computerized tests are not wholly equivalent to paper–pencil assessments that were the original basis of diagnostic criteria, perhaps due to differences in difficulty level and additional sources of performance error related to computer use. This issue has been raised by Loring et al., (Reference Loring, Bowden, Staikova, Bishop, Drane and Goldstein2019), who found differential predictive ability of the NIHTB-CB PSMT and the Rey Auditory Verbal Learning Test (AVLT), with the PSMT accurately predicting impaired AVLT performance, but poorly predicting combined borderline/impaired performance (Loring et al., Reference Loring, Bowden, Staikova, Bishop, Drane and Goldstein2019). This raises the possibility that there is something inherently different about verbal learning tests administered via paper and those administered on a computer, and the same may hold for tests of crystallized ability. Additionally, the NIHTB-CB and the CBB have now been reformatted for iPad administration and although the iPad NIHTB-CB and computer-based formats have been found to be more or less equivalent in non-Hispanic White samples (Mielke et al., Reference Mielke, Machulda, Hagen, Edwards, Roberts, Pankratz and Petersen2015), further comparisons are needed to determine if there is a difference between computer and iPad administrations in Black adult samples. Such a comparison is warranted given the evidence that the CBB iPad version yielded slower performance then computer-based administrations (Stricker et al., Reference Stricker, Lundt, Edwards, Machulda, Kremers, Roberts and Mielke2019). We are currently conducting this analysis at the Michigan Alzheimer’s Disease Research Center, but more work including comparisons across different racial/ethnic groups is needed to determine the sensitivity and validity of these assessments in characterizing MCI and AD.
Beyond our finding of group discrimination based foremost on crystallized abilities, computerized measures of declarative memory, learning, and executive functioning also significantly contributed to the group discrimination model, albeit to a relatively lesser extent. While memory impairment is emphasized in studies of preclinical AD, evidence suggests that impairment in cognitive domains other than memory, such as executive functioning and language, may be more accurate predictors of early cognitive decline than memory functioning, particularly in Black adults who are living independently in urban settings (Gamaldo, Allaire, Sims, & Whitfield, Reference Gamaldo, Allaire, Sims and Whitfield2010). This raises the possibility that the preclinical/MCI symptom profile in Black adults may be different than non-Hispanic White adults, but this has yet to be fully elucidated given little demographic diversity in many clinical trials of MCI, AD, and other dementias. Further, participants in the MCI group more closely represented a multidomain MCI profile, rather than a single-domain aMCI, which was consistent with the NACC-UDS consensus diagnoses. Using the computerized batteries and developing cognitive profiles using a data-driven approach is an important step in determining which diagnostic features appear to be universal and which may differ in Black adults as compared to non-Hispanic Whites. Overall, while these computerized assessments appear to be relatively more sensitive to differences in crystallized ability than memory and executive functioning, the extent to which these measures can be used to identify multidomain MCI in Black adults indicates that computerized measures may be suitable for this type of community-based research, as long as relevant control variables are considered. As of now, these computerized measures do not appear to be a suitable substitute for gold-standard neuropsychological assessment and clinical diagnosis.
This study is not without limitations. First, we did not have an adequate sample size within our groups to compare aMCI and naMCI participants; instead we combined these samples into a single MCI group. This was decided based on our preliminary analysis showing no significant differences on cognitive test performances between those groups, except on the NIHTB-CB Flanker subtest. We verified this by showing that the three-group model also yielded a similar pattern of discriminant tests. Further, it is important to emphasize that our MCI participants were, for the most part, multidomain MCI which helps to explain the presence of tasks of executive functioning, learning, and declarative memory as also contributing to our DFA model. Future research will seek to expand on these findings and include a wider range of cognitive-associated diagnoses (e.g., multi- and single-domain naMCI, aMCI, and AD samples). Secondly, our sample was based on volunteers from the community and was predominantly female, which may limit the generalizability of these findings to broader populations outside of an AD research center setting. Importantly, Sundermann et al. (Reference Sundermann, Maki, Rubin, Lipton, Landau and Biegon2016) found that females performed better than males on a test of verbal memory, despite comparable brain hypometabolism, and the relationship between hypometabolism and memory performance was stratified such that females were most different from males at a later disease state (i.e., increased hypometabolism) and differences between sexes were minimal at the mild end of the disease state. Therefore, the authors argue that females may have a “cognitive reserve” advantage over males in the domain of verbal memory which is protective against memory decline later in the disease state. Future research should include more males to further explore that hypothesis. We report a cross-sectional analysis that cannot evaluate the sensitivity of the cognitive assessment to decline and transition to disease; however, the between-group comparison and validation against the NACC-UDS identified critical directions for future research on computerized assessments. Computer-related anxiety was included as covariate in all analyses, but there also remains a question as to whether computerized assessments may differentially bias performance across cognitive domains, especially assessments of crystallized functions (Scott et al., Reference Scott, Sorrell and Benitez2019). A more thorough understanding of how this may contribute to differences in sensitivity to detect MCI among Black adults is warranted.
To our knowledge, this is the first report to use both the NIHTB-CB and the CBB in a sample of community-dwelling Black adults in the assessment of MCI. This research expands our understanding of the use of computerized cognitive batteries and hopefully highlights important areas of needed work in utilizing and interpreting findings from these measures. One conclusion and area of further work is the need for more sensitive and specific methods for evaluating ethnic minority populations. Including aspects such as depression and perceived discrimination as it relates to cognitive decline, as others have (Zahodne et al., Reference Zahodne, Sol and Kraal2019), will also help us better elucidate our findings. As we continue the important work of seeking novel ways to enhance our understanding of MCI in underrepresented populations and improve recruitment and retention strategies, computerized cognitive assessments will likely play an important role in this endeavor. Therefore, we must ensure that the data we are obtaining are applicable to these populations of interest, are culturally and racially agnostic, and yield useful clinical research outcomes across diverse samples which can serve as comparable outcomes to more formalized assessment batteries such as the NACC-UDS. We will extend on this work by seeking to determine if these results are replicable and through observing the longitudinal trajectories of these individuals to inform our cross-sectional findings.
SUPPLEMENTARY MATERIAL
To view supplementary material for this article, please visit https://doi.org/10.1017/S135561772100028X
ACKNOWLEGMENTS
The authors would like to thank the research participants for taking part in this study and our research staff for their concerted effort in recruitment and data collection.
FINANCIAL SUPPORT
This research was partially supported by funding from NIH/NIA grant P30 AG053760 to the Michigan Alzheimer’s Disease Research Center (MADRC; PI, H. Paulson) and from NIA/NIH, R21 AG046637-01A1, R01 AG 054484 (PI, V. Kavcic).
CONFLICTS OF INTEREST
None.