Introduction
In 2017, global deaths attributable to cardiovascular disease and diabetes exceeded 19 million. Reference Roth, Abate and Abate1 Although age-standardised cardiovascular mortality rate has declined over the past three decades, the global prevalence of significant cardiometabolic risk factors such as hypertension, dyslipidaemia and obesity have risen dramatically during this time. Reference Zhou, Bentham and Di Cesare2–Reference Farzadfar, Finucane and Danaei4
Lifestyle factors contribute significantly to the development of these cardiometabolic risks. However, over the past three decades, the relative contributions of these behaviours to cardiovascular mortality have decreased among high income countries, particularly in relation to tobacco smoke (85 to 39 deaths per 100,000), low physical activity (15 to 12 deaths per 100,000), and imbalanced diet (139.89 to 97.06 deaths per 100,000). Reference Stanaway, Afshin and Gakidou5 Despite this, age-standardised mortality attributable to adverse cardiometabolic phenotypes continues to increase, even in populations where population level lifestyle interventions have been implemented. Reference Roth, Abate and Abate1
Suboptimal fetal growth (restricted or excessive growth) has a well-established relationship with elevated blood pressure, Reference Mu, Wang and Sheng6 obesity, Reference Schellong, Schulz, Harder and Plagemann7 and cardiovascular disease. Reference Knop, Geng and Gorny8 Animal studies suggest this relationship may be attributable to changes in the structure and function of key organs involved in cardiometabolic homeostasis. Reference Bertram and Hanson9 The hypothalamic-pituitary-adrenal axis (HPA-A) is among the most sensitive fetal organ systems vulnerable to perturbed growth, driving the hormonal response to stress through its secretion of cortisol. Reference Martin, Pennell, Wang and Reynolds10–Reference Reynolds12 Independent studies have observed positive associations between fetal growth and HPA-A function throughout the lifespan, Reference Kajantie, Feldt and Raikkonen13 as well as HPA-A function and systolic blood pressure (sBP), Reference Reynolds, Syddall, Walker, Wood and Phillips14 BMI Reference Fraser, Ingram, Anderson, Morrison, Davies and Connell15 and total cholesterol, Reference Fraser, Ingram, Anderson, Morrison, Davies and Connell15 suggesting that altered HPA-A function may be an important mediator of the relationship between fetal growth and adult cardiometabolic risk.
Alternatively, the relationship between fetal growth and cardiometabolic outcomes may vary by an individual’s HPA-A phenotype. Indeed, some studies have observed simultaneous associations between fetal growth, markers of HPA-A function and cardiometabolic outcomes in cross-sectional cohorts. Reference Phillips, Barker and Fall16,Reference Krishnaveni, Veena, Dhube, Karat, Phillips and Fall17 Unfortunately, whether the birthweight-metabolic relationship varies by HPA-A phenotype was not addressed in these studies. Given that HPA-A phenotypes are known to be influenced by genetic variation, Reference Bolton, Hayward and Direk18,Reference Anderson, Briollais and Atkinson19 it is possible that the associations between suboptimal fetal growth and cardiometabolic outcomes may also vary depending on HPA-A phenotype.
Despite substantial animal model data, the mechanisms linking fetal growth to cardiometabolic disease in adulthood remain unclear in humans. As our ability to prevent disease through lifestyle modification continues to plateau over time, understanding the mechanisms linking early life adversity to cardiometabolic disease may become increasingly important.
The aim of this study was to determine whether HPA-A function mediates or moderates the relationship between fetal growth and cardiometabolic measures in adulthood. We hypothesised that HPA-A function at age 18 would mediate the relationship between fetal growth and cardiometabolic outcomes.
Methods
Study sample
The Raine Study is a longitudinal cohort study, which recruited 2900 pregnant women between 1989 and 1991. Reference Newnham, Evans, Michael, Stanley and Landau20 Women were recruited between 16–18 weeks’ gestation from the public antenatal clinic at KEMH, as well as surrounding private clinics. Inclusion criteria included sufficient English language skills to participate in the study, an expectation to deliver at KEMH, and intention to reside in Western Australia to allow future follow-up.
Parental socio-economic, anthropometric, and medical questionnaires were administered at 18 and 34 weeks, and data regarding pregnancy, and fetal ultrasound were collected. A total of 2868 live births occurred, from 2730 mothers. Both Gen1 and Gen2 participants were contacted and invited for follow-ups at several time points. A variety of data including behavioural, environmental, genetic and anthropometric information were collected at these follow-ups. The Human Research Ethics Committees at the University of Western Australia, King Edward Memorial Hospital (KEMH) and Princess Margaret Hospital in Perth, Australia, granted ethics approval for each follow-up in the study. Parents, guardians and young adult participants provided written informed consent either before enrolment or at data collection at each follow-up. Further technical details about the Raine Study are available elsewhere. Reference Dontje, Eastwood and Straker21
The participants in this study were term singletons who participated in the Trier Social Stress Test (TSST) at age 18 and presented for routine follow-up at 20 years. Of the original Raine Study Gen2 participants (n = 2868), 17% had withdrawn from the study, died, or been lost to follow-up (n = 701). This attrition left 2167 Raine Study Gen2 participants eligible to be contacted for the 18-year review, of which 1137 agreed to participate in the TSST protocol. From the 1137 eligible participants, 220 were excluded from analysis (see Fig. 1) resulting in a TSST study population of 917. Of these participants, 851 returned for follow-up at age 20; 706 provided blood for analysis of which three were unfasted and 802 participated in the physical assessment at 20 years. Figure 1 illustrates inclusion and exclusion criteria for participants at each stage of our analysis.

Fig. 1. Inclusion/Exclusion criteria.
Early life covariates
Data regarding maternal characteristics were obtained from questionnaires at 18 and 34 weeks’ gestation. Several factors are known to affect fetal growth, HPA-A function and cardiometabolic health. Reference Martin, Pennell, Wang and Reynolds10,Reference Reynolds12,Reference Martin, Patel and Kramer22–Reference Reynolds, Gray, Li, Segovia and Vickers24 Variables in our analyses included: maternal pre-pregnancy BMI, weight gain as a percentage of pre-pregnancy weight, smoking status, cumulative antenatal exposure to stressful life events at 18 weeks’ gestation, Reference Tennant and Andrews25 and the diagnosis of hypertension at any point prior to or during pregnancy.
Data about fetal characteristics were collected at birth. In this study, we used a measure of fetal growth potential (Per cent optimal birthweight (POBW)), developed based on Raine Study Gen2 participants, as our exposure. Reference Pereira, Blair and Lawrence26,Reference Blair, Liu, de Klerk and Lawrence27 POBW is a measure of the fetus’ fulfilment of its optimal growth potential calculated using a formula adjusting for fetal sex, maternal height, maternal parity and gestational age. Reference Blair, Liu, de Klerk and Lawrence27 This was used instead of gross birthweight, because birthweight is not useful as an exposure in isolation; it requires contextual covariates such as fetal length and gestational age to make inferences about growth potential. Rather than adding these covariates and losing statistical power, we chose an exposure that intrinsically represents growth potential. POBW has been validated as a means of estimating attainment of optimal birthweight among Raine Study Gen2 participants. Reference Pereira, Blair and Lawrence26
HPA Axis measurements
HPA Axis function was assessed at age 18 using the TSST protocol, which is the validated Reference Narvaez Linares, Charron, Ouimet, Labelle and Plamondon28 gold standard for assessing the HPA Axis response to acute stress. Comprehensive details about the TSST Protocol in the Raine Study have been published elsewhere. Reference Herbison, Henley and Marsh29 In brief, the TSST is a controlled psychosocial stressor designed to elicit a cortisol stress response in participants. The TSST (∼15 min) involved 3-min preparation time in isolation, a 5-min mock job interview, and a 5-min arithmetic task, all of which occurred in front of a non-responsive group of adult assessors. In consenting participants, blood was drawn once before and at multiple time points after the TSST (16, 25, 35, 45, 60, 75 and 105 min).
Together, these measures generated cortisol and ACTH response curves spanning 105 min. From these curves, well-defined variables were created based on established definitions. Reference Pruessner, Kirschbaum, Meinlschmid and Hellhammer30 Of these, the continuous variables used in our analyses included plasma baseline cortisol concentration and adrenal sensitivity. Adrenal sensitivity was derived by dividing the cortisol area under the curve Reference Pruessner, Kirschbaum, Meinlschmid and Hellhammer30 by ACTH area under the curve. Reference Kajantie, Feldt and Raikkonen13
Several factors influence the HPA-A response to the TSST protocol. Reference Narvaez Linares, Charron, Ouimet, Labelle and Plamondon28,Reference Herbison, Henley and Marsh29 These include time of day, smoking status, sex, use of the oral contraceptive pill (OCP), phase of menstruation and adiposity. Our TSST was conducted in the early afternoon to accurately approximate baseline HPA-A function (cortisol levels peak in the morning). Among the remaining variables, only participant sex contributed to more than 1% of the variance in our final outcomes.
TSST pattern variables
In addition to continuous measures, the TSST generated characteristic stress response curves which were plotted over time and analysed according to published patterns Reference Herbison, Henley and Marsh29 : “reactive responders” (RR) who mount an appropriate cortisol rise in response to stress; “anticipatory responders” (AR) who begin the TSST with high levels of baseline cortisol in anticipation of a stressful event; and “non-responders” (NR) who do not show a change in baseline HPA activity following the TSST.
Cardiometabolic measures
Cardiometabolic measures were assessed at the 20 year follow-up of the Raine Study Gen2 participants. Our analyses included BMI (kg/m2), systolic blood pressure (sBP) (mmHg) and serum low-density lipoprotein cholesterol (LDL-C) (mmol/l). BMI was derived from weight and height measured during the physical examination. Weight was measured to the nearest 0.1 kg using a Personal Precision scales UC-321 (A&D Company) with participants dressed in light clothes. Height was measured with a wall-mounted Seca 202 hypsometer to the nearest 0.1 cm. Blood pressure was assessed using an oscillometric sphygmomanometer (Dinamap ProCare 100; Soma Technology) with an appropriately sized cuff; after 5 min of quiet rest, six recordings were automatically taken every 2 min in a supine position. After discarding the first reading, the remaining five systolic readings were then averaged to determine mean systolic blood pressure. Fasting venous blood samples were analysed for serum lipids measured in the PathWest Laboratory at Royal Perth Hospital. LDL-C was calculated using the Friedewald formula. Reference Friedewald, Levy and Fredrickson31 Blood samples from unfasted participants were not analysed (n = 3).
Statistical analysis
Variables were assessed for deviation from normality and transformed accordingly. Baseline cortisol and adrenal sensitivity were log-transformed to approximate normality. Differences in characteristics between included and excluded participants were assessed among continuous measures using independent t-tests and Chi-squared tests for categorical variables. To determine whether the HPA-A measures were correlated, Sex and OCP adjusted Pearson’s correlation coefficients were calculated.
We used a statistical approach developed by Vanderweele Reference VanderWeele32 to dissect the total effect of fetal growth on adult cardiometabolic health to determine whether HPA-A function mediated or moderated this relationship. Two multivariable linear regression models were established; the first was an outcome model which included POBW, HPA-A measure and their interaction term as predictors. The second was a mediator model which included POBW as a predictor and an HPA-A measure as the outcome. Each of these models were then adjusted by covariates that explained at least 1% of the variance in both mediator and outcome; these included maternal BMI, hypertension, smoking in pregnancy and weight gain as a percentage of pre-pregnancy body weight as well as offspring sex. BMI at age 20 (Gen2) was added as a covariate in analyses of serum LDL-C and sBP.
The total effect observed in these models was then decomposed into four components: 1) the effect due to neither mediation or interaction, that is, only POBW (controlled direct effect); 2) the effect due to an interaction between POBW and HPA-A measure (reference Interaction); 3) the effect due to mediation via HPA-A measures (pure indirect effect); and 4) the effect due to a combination of mediation and interaction (mediated interaction). Estimates for each of these decompositions was obtained for a 24% increase in POBW (two standard deviations) from the mean, while covariates were set to their mean or zero for categorical confounders. Further explanation of these definitions and their assumptions is provided in Fig. 2. Proportions of the total effect that were due to each of these four components were also calculated (see formulae in Fig. 2). Standard errors were bootstrapped using 1000 replications.

Fig. 2. Direct Acyclical Graph (DAG).
Analyses were not adjusted for multiple testing as the main objective of the study was hypothesis generation (to determine whether HPA-A function moderated or mediated the effect of fetal growth on cardiometabolic variables). We considered a two-tailed p < 0.05 to be significant. All analyses were performed using STATA v 15 software (StataCorp, College Station, TX, USA).
Role of the funding source
Study sponsors had no role in the study design, data collection, data analysis, interpretation of results or in the final decision to submit the paper for publication.
Results
Study population
On average, participants in this study had mothers who were older, less likely to have smoked during pregnancy, weighed more at birth and had longer gestations compared other Gen2 participants of the Raine Study (Supplementary Table 1). We did not observe any patterns associated with missingness. The characteristics of the participants are shown in Table 1.
Table 1. Participant characteristics

For continuous variables, data shown as means and standard deviations if normally distributed.
* Medians and interquartile ranges (IQR) if skewed.
a Data presented as raw counts and percentages for categorical variables.
b Weight gain as a percentage of pre-pregnancy body weight.
Associations between mediators
The correlation between sex-adjusted HPA-A measures are shown in Supplementary Table 2. Some HPA-A measures were correlated in our sample. Baseline cortisol explained 34% of the variance in adrenal sensitivity (p ≤ 0.05) and 19% of the probability of being an anticipatory responder (p ≤ 0.05). All other correlations explained < 1% of variance across HPA-A measures.
Regression models of outcome and mediators
The multivariate models examining the association between POBW, HPA-A measures and cardiometabolic outcomes are presented in Tables 2, 4 and Supplementary Table 3.
Table 2. Associations between per cent optimal birth weight, HPA-A function at 18 and body mass index at 20

Results are shown as Beta coefficients with standard errors in parentheses. The table describes the relationship between a 1 unit increment in either per cent optimal birthweight, HPA-A metric or the interaction between the two. The models were adjusted for Maternal BMI, smoking status, hypertension, weight gain as a percentage of pre-pregnancy body weight and offspring sex.
Natural logarithm taken because of a skewed distribution.
Table 3. Mediating and moderating effects of HPA-A Axis function at 18 on BMI at age 20

Estimates derived based on a standard deviation from mean POBW (86%–110%) when HPA-A measures were either fixed at their mean values (baseline cortisol = 5.84 nmol/L, adrenal sensitivity = 2.83 nmol/pg) or counterfactual levels (TSST patterns set to 0).
Table 4. Associations between per cent optimal birth weight, HPA-A function at 18 and systolic blood pressure at 20

Results are shown as Beta coefficients with standard errors in parentheses. The table describes the relationship between a 1 unit increment in either per cent optimal birthweight, HPA-A metric or the interaction between the two. The models were adjusted for Maternal BMI, smoking status, hypertension, weight gain as a percentage of pre-pregnancy body weight, offspring sex and BMI at age 20.
Natural logarithm taken because of a skewed distribution
Outcome models
The relationship between fetal growth, sBP and BMI was closest to their true effect estimates (Supplementary Table S5) when TSST patterns were used as interaction terms (see Tables 2 and 4). This interaction was strongest among anticipatory responders with respect to their systolic blood pressure; smaller babies with an anticipatory response phenotype had higher systolic blood pressures as adults (Interaction β = −0.17, 95% CI −0.30 to −0.04). Similar results were observed with LDL-C, in which we observed a positive quadratic relationship between fetal growth and LDL-C when TSST Patterns were set as interaction terms. (see Supplementary Table 3).
Mediator models
POBW was inversely associated with continuous measures of HPA-A function, but no associations were observed with HPA-A phenotype (Supplementary Table 4). In our analyses, a 1% increase in POBW was associated with a 0.4% decrease in both baseline cortisol and adrenal sensitivity (95% CI −0.7 to −0.06), suggesting that smaller babies had higher cortisol reactivity to acute psychosocial stress in young adulthood. Reference Martin, Wang and Lye33 Adjustment for baseline cortisol attenuated the relationship between POBW and BMI (from β = 0.054 to β = 0.051 after adjustment) while adjustment for baseline cortisol and adrenal sensitivity, respectively, attenuated the effect of POBW on sBP (baseline cortisol, from β = −0.065 to β = −0.051; adrenal sensitivity, from β = −0.065 to β = −0.055; see Supplementary Table S5). Adjusting for HPA-A function had no effect on the relationship between POBW and serum LDL-C. These findings suggested that continuous measures of HPA-A function may have been mediators of the relationship between POBW and the cardiometabolic variables examined.
Decomposition of the total effect
We decomposed the total effect of POBW on cardiometabolic outcomes into four components (see Tables 3 and 5). Effect estimates were calculated by fixing covariates and continuous HPA-A measures at their means; TSST response groups were considered as binary mediators, and set at their counterfactual outcomes (i.e. does not equal the response group being assessed). We then estimated outcomes for a two standard deviation change (86%–110%) in POBW.
Table 5. Mediating and moderating effects of HPA-A Axis function at 18 on systolic blood pressure at age 20

Estimates derived based on a standard deviation from mean POBW (86%–110%) when HPA-A measures were either fixed at their mean values (baseline cortisol = 5.84 nmol/L, adrenal sensitivity = 2.83 nmol/pg) or counterfactual levels (TSST patterns set to 0). BMI at age 20 added as a covariate.
We observed a consistent total effect of POBW on BMI throughout our four-way decomposition (β = 0.89–1.26), suggesting that a two standard deviation change in POBW increased adult BMI by up to more than one unit. The direct relationship between POBW and BMI accounted for 94%–96% of this total effect when continuous HPA-A measures were used (95% CI 69%–119% baseline cortisol; 88%–105% adrenal sensitivity). Otherwise, we found no causal evidence of mediation, moderation or mediated interaction through HPA-A function in the prediction of BMI. We observed a modest interaction approaching significance between TSST pattern and POBW in predicting BMI; the greatest effect size was seen in NR’s, in whom the interaction accounted for 0.19 units of the 0.89 unit change in BMI associated with a two standard deviation increase in POBW (95% CI −0.01 to 0.40).
Similarly, we also observed a consistent total effect of POBW on sBP, with stronger total effects when TSST Patterns were considered as mediators and moderators. In our analyses, a two standard deviation increase in POBW reduced systolic blood pressure by between 1.43 mmHg (95% CI –3.07 to 0.20) to 2.00 mmHg (95% CI –3.76 to –0.65) in young adulthood. We also observed a strong reference interaction between POBW and TSST Pattern; this was strongest in AR’s, in whom an anticipatory response accounted for –1.04 mmHg (95% CI –1.77 to –0.32) of the –1.97 mmHg (95% CI –3.46 to –0.48) decrease in sBP associated with a two standard deviation increase in POBW. This would suggest that the overall negative relationship observed between POBW and sBP in the mutlivariable linear regression models was largely driven by anticipatory responders, in whom the relationship was the strongest. Decomposition of the parabolic total effect of POBW on LDL-C was not attempted.
Controlled direct effect of per cent optimal birthweight on body mass index and systolic blood pressure
An increase of two standard deviations (86%–110%) in POBW was associated with an increase in adult BMI only in young adults above the 30th percentile of baseline cortisol (CDE = 0.90 kg/m2, 95% CI: 0.11–1.69) and 20th percentile of adrenal sensitivity (CDE = 0.98 kg/m2, 95% CI = 0.11–1.87; see Supplementary Figures 1 and 2). The controlled direct effect of POBW on adult sBP was also higher among participants with higher levels of baseline cortisol and adrenal sensitivity, though these estimates did not reach statistical significance (see Supplementary Figures 3 and 4).
Subgroup analyses
Subgroup analyses of the total effects of POBW on BMI and sBP, stratified by HPA-A responder status are shown in Figs. 3 and 4 and Tables 6 and 7. The diagrams demonstrate the different gradients between fetal growth and adult cardiometabolic measures based on HPA-A response to acute psychosocial stress. In NR, the effect of POBW on BMI increases from the overall participant gradient of 0.05 (kg/m2) per increment of POBW (95% CI = 0.02–0.08; Supplementary Table 5) to 0.08 (95% CI 0.02–0.13; Table 6). In AR, the differential effect is only present in sBP, in whom the association between POBW and sBP is stronger; more than doubling from the overall participant gradient of −0.065 (95% CI −0.13 to 0.002; Supplementary Table 5) to −0.15 (95% CI −0.29 to −0.01; Table 7).
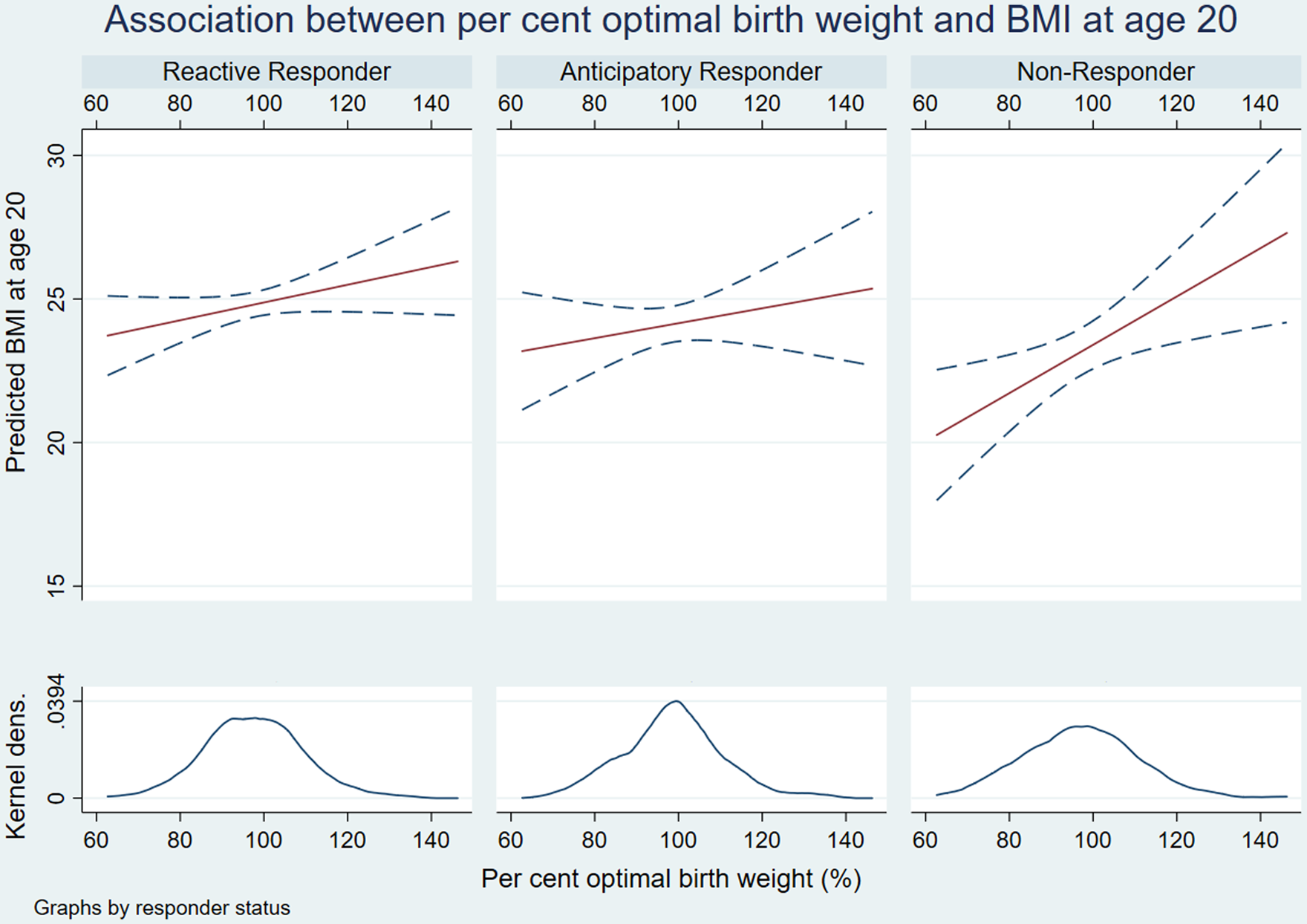
Fig. 3. Interaction plots of relationship between per cent optimal birthweight and body mass index.

Fig. 4. Interaction plots of relationship between per cent optimal birthweight and systolic blood pressure.
Table 6. Subgroup analyses demonstrating differential association between per cent optimal birth weight and body mass index at age 20

Regression table demonstrates the change in BMI for a 1% increment in per cent optimal birth weight.
Table 7. Subgroup analyses demonstrating differential association between per cent optimal birth weight and systolic blood pressure at age 20

Regression table demonstrates the change in systolic blood pressure for a 1% increment in per cent optimal birth weight.
Discussion
The purpose of this study was to determine whether HPA-A function mediates or moderates the relationship between growth restriction and cardiovascular risk. Our a priori hypothesis was that HPA-A function would mediate the relationship between fetal growth and adult cardiometabolic measures. This was based on the literature around ‘predictive adaptive responses’, Reference Hanson and Gluckman34 in which adverse early life environments generate phenotypic adaptations necessary to survive in a hostile ex-utero environment. We hypothesised that suboptimal fetal growth would drive changes to HPA-A function in our cohort, which would then manifest with altered cardiometabolic health in adulthood. Our data do not support this hypothesis.
Independent of the HPA-A, we observed a direct relationship between fetal growth and BMI, sBP and LDL-C levels at age 20. For BMI, the relationship was linear, for LDL-C it was ‘U-shaped’, and for sBP the relationship was inverse linear. These findings are similar to the existing literature for BMI Reference Zhao, Wang, Mu and Sheng35 and sBP Reference Mu, Wang and Sheng6 and novel for LDL-C at age 20. A recent meta-analysis Reference Knop, Geng and Gorny8 of these relationships in over seven million subjects has replicated the original inverse association with blood pressure, but suggested a ‘J-shaped’ relationship with the risk of developing T2DM and cardiovascular disease. Our findings add to these studies by suggesting that different mechanisms may be responsible for the increased risk of cardiometabolic disease at extremes of fetal growth. Our data suggest that in babies that fail to reach their growth potential, the increased risk of cardiovascular disease may be driven by elevated blood pressure, a relationship which is stronger in those with anticipatory HPA-A phenotypes. Conversely, the increased risk of cardiometabolic disease in babies who exceed their growth potential may be due to increased BMI.
Our data demonstrate a direct linear relationship between fetal growth and BMI in young adults. For every 10% increase in POBW, we observed a 0.5 unit increase in BMI. This observation adds to the existing literature, which suggests that the risk of overweight and obesity is higher among large for gestational age babies. Reference Zhao, Wang, Mu and Sheng35,Reference Yu, Han and Zhu36 In our study, HPA-A function did not modify this relationship. In contrast to obesity, we observed a negative linear relationship between POBW and sBP. Subgroup analyses suggested that this association was strongest among anticipatory responders. This observation was supported by repeated estimates of controlled direct effect, where effect sizes were stronger at higher levels of baseline cortisol and adrenal sensitivity, though these CDE estimates did not reach significance.
Evidence describing the relationship between fetal growth and serum LDL-C is limited. Reference Laurén, Järvelin and Elliott37 In our cohort, we observed a significant ‘U-shaped’ relationship. One key difference between our results and those previously published is age. Most of the previous studies examining the relationship between fetal growth and LDL-C have been conducted in participants of middle or advanced age. Reference Laurén, Järvelin and Elliott37 In comparison, our participants were aged 20. Measurements of cholesterol at later time points in the Raine Study will be required to see if the quadratic relationship remains consistent in this cohort.
As noted earlier, our data do not support our a priori hypotheses. There are several possible explanations for our findings. First, our participants were healthy, young adults, and may have been simply too young to manifest with clinically significant cardiometabolic changes. Reference Kajantie, Feldt and Raikkonen13,Reference Law, de Swiet and Osmond38 Our sample size was also relatively small compared to most epidemiological studies, limiting our ability to identify smaller effect sizes. Participant attrition may also have led to an analytic sample that was healthier and had more favourable antenatal exposures compared to our original cohort. This may have limited our ability to detect subtle effects across the life span.
Alternatively, the HPA-A may not be a mediating pathway through which fetal growth acts on cardiometabolic health, but rather, a genetically determined interactive factor which may enhance or attenuate the cardiometabolic impacts of suboptimal fetal growth. This is supported by a recent mendelian randomisation study of more than 200,000 participants Reference Warrington, Beaumont and Horikoshi39 which found that the inverse relationship between birthweight and adult blood pressure was primarily attributable to genetic effects, although the specific mechanisms of genetic predisposition remain unclear. Reference Manenschijn, van den Akker and Ester40 To the best of our knowledge, this is the first longitudinal study assessing the relationship between fetal growth, HPA-A function and cardiometabolic measures in a single cohort of young adults, using causal modelling in a large TSST sample. Confirming this relationship in young adults is important, as HPA-A function is known to stabilise in young adulthood Reference McCormick and Mathews41 thereby influencing an individual’s cumulative lifetime exposure to glucocorticoids. Despite this, our study has important limitations.
First, our findings may not apply to diverse populations as majority of our participants and their mothers were of Caucasian descent. POBW is also a metric derived using data from the Raine Study; this measure of fetal growth potential may not apply to other populations. Additionally, several maternal, paternal and early life exposures are known to influence the life-course relationships between fetal growth, HPA-A function and cardiometabolic health Reference Martin, Pennell, Wang and Reynolds10,Reference Warner and Ozanne42–Reference Rodriguez-Caro and Williams45 ; not all of these factors were measured in our study. Unmeasured confounding may therefore have biased our estimates toward type I error and violated the assumption of no unobserved confounding necessary for causal inference. A further limitation was that our estimates of sBP included BMI at the time of measurement as a covariate. This may have introduced collider stratification bias Reference Cole, Platt and Schisterman46 (see Supplementary Table 6) or exaggerated the POBW-sBP relationship, as part of it may have operated through BMI. Reference Linderman, Lu and Lu47 Another important weakness in our study was that our analyses of cholesterol were limited to LDL-C. Derangements in baseline levels of total cholesterol, triglycerides, high-density lipoprotein (HDL) and LDL-C are associated with later life mortality among young adults. Among these cardiometabolic risk factors, we chose LDL-C as our outcome. There are some important reasons for this. Firstly, as a limitation of our statistical methodology, it was necessary to limit our outcomes to variables that were not derived from one another; this precluded the use of total cholesterol, which is the sum total of the different serum cholesterols and triglycerides. Secondly, most interventions aimed at lowering cholesterol levels to prevent cardiovascular disease and premature mortality target LDL-C. 48 In view of this, as well as our need to minimise outcome measures, we chose to use LDL-C. Finally, participants in our study generally had a more favourable early life phenotype; they were heavier at birth, had older mothers, and were less likely to have been exposed to maternal smoking compared to Raine Study offspring who did not participate. This inadvertent selection bias may have reduce the true observed effect of certain suboptimal early life exposures on offspring outcomes.
Conclusion
Using a large longitudinal cohort of young adults and a unified approach to interaction and mediation, we were unable to show that HPA-A function in young adulthood mediates the relationship between fetal growth and cardiometabolic risk. Babies at extremes of fetal growth have an increased cardiometabolic risk in young adulthood; in smaller babies, this may be driven by elevated blood pressure, while in larger babies, this risk may manifest through increased BMI. HPA-A reactivity may interact with fetal growth to amplify these relationships.
Supplementary material
For supplementary material accompanying this paper visit https://doi.org/10.1017/S2040174422000186
Acknowledgements
We are grateful to The Raine Study participants and their families, as well as The Raine Study team for cohort co-ordination and data collection.
Financial support
We gratefully acknowledge the NHMRC for their long term funding to the study over the last 30 years and also the following institutes for providing funding for Core Management of the Raine Study: The University of Western Australia, Curtin University, Telethon Kids Institute, Women and Infants Research Foundation, Edith Cowan University, Murdoch University, The University of Notre Dame Australia, and the Raine Medical Research Foundation. The collection of the DEXA scan data for the Raine Study Gen2-20 year follow-up and Trier Social Stress Test (TSST) sub-study from the Raine Study Gen2-18 sub-study were supported by the Canadian Institutes of Health Research – CIHR (MOP-82893), while the Raine Study Gen2-20 year follow-up was supported by the National Health and Medical Research Council of Australia [grant numbers 634445, 634509, and 1021105], the Canadian Institutes of Health Research [grant number MOP-82893], Ophthalmic Research Institute of Australia (ORIA), Alcon Research Institute, Lions Eye Institute and the Australian Foundation for the Prevention of Blindness.
Conflicts of interest
The authors have none to declare.
Ethical standards
The authors assert that all procedures contributing to this work comply with the National Statement on Ethical Conduct in Human Research and with the Helsinki Declaration of 1975, as revised in 2008.