America’s legal technology boom presents a puzzle. On the one hand, America’s market for legal services is among the most tightly regulated in the world, suggesting infertile ground for a legal technology revolution. America has the world’s most robustly protected lawyer monopoly. Its version of the prohibition against the unauthorized practice of law (UPL) is among the broadest and most aggressive in the world because it theoretically bans all non-lawyers from giving any “legal advice” in addition to the more typical bar against filing papers or appearing in court. Likewise, America’s barriers to entering the legal profession are exceedingly high: generally speaking, an undergraduate degree, another three years of law school, a challenging bar exam, an intrusive character and fitness process, and so forth. Between these two barriers, the American lawyer’s monopoly has historically been capacious and jealously guarded. Given this regulatory environment, one would expect America to have a small and beleaguered legal technology sector. Surely wealthy and politically connected bar associations would nip any potential competitors in the bud.
Actually, not so much. The other side of this puzzle is America’s advanced and free-wheeling market for legal tech, which is probably the most robust in the world, as the Sako and Armour chapter herein establishes. The present chapter seeks to explain this seeming puzzle, and then uses that explanation to make some predictions about where legal technology will continue to flourish in America. The explanation is that both America’s seemingly monolithic market for legal services and its uniform regulation of lawyers are, in fact, quite segmented. In some parts of the market, lawyer regulation is almost non-existent (or at least very rarely enforced) – typically at the high end in so-called BigLaw and at the low end in non-profits and legal aid offices serving the indigent. In so-called PeopleLaw, where lawyers serve middle-class Americans and small businesses, regulation plays a significant role and has hampered technological advancement, especially in areas like providing legal advice. This chapter demonstrates how these different markets operate, how they are regulated, and how that explains the current explosive growth in legal tech in some areas, but not others.
This insight also raises a note of caution as we celebrate current efforts at “re-regulating” the market for legal services in states like Utah, Arizona, and others. These jurisdictions have rightfully (so far) been praised for allowing non-lawyer and technological innovations into a very constricted market. And yet any lawyer-led regulatory effort, no matter how well-meaning, could easily slide into lawyer self-protection, as many (most?) prior regulatory efforts have. The status quo favors innovation in at least the top and the bottom of the market, but we need to push regulators to loosen barriers in the PeopleLaw space, rather than surreptitiously raising barriers where innovation is currently blossoming.
1.1 An Extra-brief Overview of America’s Unusual Lawyer Regulation
The first oddity is that America’s legal profession is regulated primarily by its judicial branch, rather than its legislative or executive branches. In America, state supreme courts control lawyer regulation in the first instance. This is unusual domestically – every other American occupation, from cosmetologists to doctors, is governed by legislatures – and internationally.Footnote 1
Unsurprisingly, given that the judiciary is staffed by former elite lawyers, the resulting regulation has turned out to be pretty lawyer-friendly.Footnote 2 The upshot is a uniquely well-defined and defended lawyer monopoly. Requiring seven years of higher education followed by notoriously difficult licensing exams makes it very expensive in time and money to become an American lawyer.Footnote 3
Regulators also fight to keep non-lawyers out of the market. That’s where the uniquely American version of UPL comes in. UPL is prohibited in all fifty states.Footnote 4 The definition of the “practice of law” and the levels of enforcement differ from state to state, but at a minimum in no state may a non-lawyer appear in court on behalf of another party or give “legal advice.”Footnote 5 For decades the combination of UPL and high barriers to entry left the American legal profession uniquely powerful and protected, and through at least the 1990s likely increased lawyer earnings.Footnote 6
Given a monolithic regulatory structure, run largely by friendly actors, one would expect American lawyer regulation to favor lawyers. That has indeed been the case historically. If past were prologue, then we would expect that non-lawyer-driven legal tech would either be barred altogether or severely stunted in this country. After all, consider the lengthy battle by some lawyer regulators to hang on to real estate closings as “legal work” protected by UPL.Footnote 7
1.1.1 Legal Tech Thrives Regardless: Why?
Yet, in this regard history has proven a poor guide. Legal tech has actually flowered in the United States. There are three primary reasons. The first is that the American lawyer’s monopoly has been taking on water since the most anticompetitive regulation came under attack in the 1970s. Initially the Supreme Court held bar association mandatory fee schedules and some advertising bans were unlawful.Footnote 8 In the 1990s the Justice Department investigated whether the ABA’s role in regulating law schools violated antitrust law. The investigation resulted in a pretty far-reaching settlement and much looser regulation of law schools.Footnote 9
Most recently, in 2015, the Supreme Court held that the enforcement of the dental equivalent of UPL against non-dentist teeth whiteners violated antitrust law in North Carolina Board of Dental Examiners v. Federal Trade Commission.Footnote 10 As of yet no court has extended this case to lawyers, but even the chance that one might has chilled the ardor of bar association UPL enforcement.Footnote 11
Some states and localities have launched pretty aggressive reforms. Famously, Utah has created a new “regulatory sandbox” that allows for non-lawyers and new technologies to apply for approval to offer some legal services.Footnote 12 Oregon is considering adding two new ways to join the profession in addition to three years of law school and a bar examination: an “experience-based learning pathway” and a “supervised practice pathway.”Footnote 13 Arizona has allowed non-lawyer ownership of law firms under a new “alternative business structures,” or ABS, model, and it also created a class of “legal paraprofessionals” who are allowed to perform some activities previously limited to lawyers.Footnote 14 There are working groups considering similar changes in California, Illinois, New York, Michigan, and other states.Footnote 15
Taken together, the bar’s ability (and desire) to maintain tight control over its monopoly has lessened quite a bit over recent decades. This partially explains why the market in PeopleLaw is so competitive and why lawyer earnings in that market have shrunk since the 1970s.Footnote 16
The second reason is America’s ever-deepening access-to-justice crisis. Characteristically, Deborah Rhode led the way in this area with her 2004(!) book Access to Justice, which explained that poor and middle-class Americans struggle to afford lawyers or to address serious legal problems.Footnote 17 The most recent and comprehensive study is the 2020 World Justice Project (WJP) Rule of Law Index, which ranks the United States thirty-sixth in the world in terms of the fairness of its civil justice system, placed snugly between Malaysia and Jordan.Footnote 18 By one estimate, four-fifths of the legal needs of poor Americans remain unmet.Footnote 19
Gillian Hadfield has repeatedly demonstrated that the problem affects more than just the poor. Middle-class Americans also cannot afford legal help, despite living in a “law thick” society where legal help is often desperately needed.Footnote 20 As demonstrated below, lawyers have, so far, been relatively uninterested in meeting these acute needs, mostly because their business model requires bespoke work and hourly fees and most Americans can’t afford to pay for that sort of help. Nevertheless, nature abhors a vacuum, so legal tech has bloomed in this space, despite regulatory concerns.
A final point – and it holds the key to understanding the past, present, and future of legal tech – is that, while America’s regulation is monolithic, its market for legal services is anything but. So, regulations that nominally apply to every lawyer and provider of legal services operate quite differently depending on the market segment.
1.2 Four Broad Categories of American Civil Law Lawyers
America’s market for legal services is also unique. Start with a number count of lawyers. In 2021, America claimed 1,327,910 licensed lawyers.Footnote 21 This was actually a slight decline from the number of American lawyers in 2019 and 2020, interrupting a 105-year streak where the profession grew every year. Nineteen-fifteen is the last time the profession shrank. America may have the most lawyers in the world, depending on how you count India’s.Footnote 22 America is second in lawyers-per-capita at one lawyer for every 249 Americans, behind only Israel.Footnote 23 By any measure, America has a lot of lawyers.
America’s legal services market is the largest in the world, with total revenues of $314 billion in 2018, accounting for a staggering 47 percent of global revenue in that sector by one estimate.Footnote 24 The United States runs a substantial trade surplus in law and is the world’s largest exporter of legal services.Footnote 25 America’s corporate law firms are the largest and most profitable in the world.Footnote 26
For our purposes we can divide America’s lawyers into four super-broad categories: (1) corporate lawyers, including so-called BigLaw firms and in-house lawyers; (2) “main street lawyers” or “PeopleLaw,” private-sector lawyers that represent small businesses and less wealthy individuals ranging from the working poor up to the upper-middle class; (3) government-supplied civil legal services for the poor (legal aid); and (4) plaintiff’s side lawyers that make a living suing large corporations or wealthy individuals.
I am well aware that, like my aggressively brisk overview of lawyer regulation, these categories may cause some consternation and that exceptions abound. The point of this very rough taxonomy is to think about how regulation and legal tech work in these broad areas, not to perfectly describe the market.
1.2.1 BigLaw/In-house Counsel
BigLaw looms large in the public imagination and in the popular press, but it has never represented even a plurality of the profession. For example, in 2019 the National Law Journal estimated that 169,477 lawyers worked at America’s 500 biggest law firms.Footnote 27 This works out to roughly 13 percent of the American lawyers that year. There are even fewer in-house counsel. In 2005 the American Bar Foundation estimated that 8 percent of licensed lawyers worked in “private industry,” that is, as corporate or in-house counsel to large businesses.Footnote 28 The numbers of in-house counsel have reportedly grown since 2005, but still these lawyers represent a relatively small portion of the market. In terms of revenue, however, BigLaw does dominate. In 2019 Statista estimated that just the hundred largest law firms earned roughly a third of the total industry revenue for legal services in America.Footnote 29
It may seem strange to lump these two very different types of lawyers together. BigLaw lawyers are ever hustling to find as many wealthy clients as they can to pay their high rates. In-house counsel naturally work for just one organization and seek to cut costs on legal matters. BigLaw lawyers are encouraged to specialize and generally work within defined practice areas. Many in-house counsel are by nature and requirement generalists.
Yet, the similarities outpace the differences, and for regulatory purposes these groups of lawyers operate in similar ways. First, the bulk of in-house counsel, and especially for larger and wealthier companies, come from BigLaw originally.Footnote 30 Second, the clients are the same. BigLaw’s revenue is driven by providing services to the largest and wealthiest businesses and individuals. Smaller or poorer clients cannot afford BigLaw. One sure sign that a company is big enough to afford BigLaw is hiring their own BigLaw lawyer as in-house counsel.
The last similarity is how lawyer regulation affects these lawyers. Historically these lawyers have been virtually unregulated. This may strike some readers as too strong, because if you have worked in BigLaw or a top corporate legal department, you know that they generally take the Rules of Professional Conduct and other ethical mandates very seriously.
Nevertheless, it is fair to consider these compliance efforts largely voluntary because bar regulators almost never investigate, let alone censure or punish, lawyers in BigLaw or in-house counsel.Footnote 31 This surely reflects the fact that most in-house and BigLaw lawyers are excellent, ethical practitioners. It also reflects that few if any of the corporations that hire in-house counsel or BigLaw are going to complain to bar regulators about their lawyers, especially when any alleged ethical violation or UPL is to the benefit of all parties concerned. When discussing legal tech and UPL, it is always worth asking who is harmed and who might complain about it. Here UPL in particular is a victimless crime. Other lawyers don’t complain. The clients don’t complain. In fact, they often request it.
For decades BigLaw has operated as if UPL is a flexible standard and have probably never fully followed the requirement that only lawyers do “legal work.” Consider the great Tom Morgan’s thoughts:
Law firms have long used paralegal and other support personnel nominally working under the lawyer supervision that ethical standards require. In addition, corporations now use non-lawyers to help deliver the total package of services they need done. Negotiating contracts, troubleshooting discrimination claims, even writing court documents can all be done by non-lawyers within an organization receiving a level of lawyer supervision and training to which unauthorized practice rules cannot effectively speak.Footnote 32
Technology has further pushed the boundaries. Consider JP Morgan’s computer program snappily entitled “Contract Intelligence or COIN.”Footnote 33 JP Morgan claims this program reviews approximately 12,000 new wholesale contracts per year and replaced “360,000 hours” of staff time between lawyers and loan officers.Footnote 34 This news was met by some fear for the future earnings of lawyers – a Harvard Business School blog warned COIN “spells disruption for the legal industry”Footnote 35 – but nary a peep from lawyer regulators or UPL enforcement. In a separate context, in an FLSA overtime suit by a contract lawyer, the Second Circuit held that “tasks that could otherwise be performed entirely by a machine” are not the “practice of law.”Footnote 36
Contract review is just the tip of the iceberg. Outsourcing to India, outsourcing legal work from one state to another, or using computer programs to perform discovery tasks all fall into at best a gray area for UPL. A BigLaw or in-house counsel would argue that all of these tasks are “supervised” by a lawyer, and thus not UPL, regardless of how much actual supervision occurs.
1.2.2 Legal Aid/Pro Bono Programs for the Poor
Ironically, there is another group of lawyers who operate with great impunity under an otherwise strict regulatory regime: legal aid and other lawyers that primarily serve the very poor. The irony here is that legal aid lawyers offer civil legal assistance to the exact opposite end of the spectrum from BigLaw: the poorest Americans, typically households earning 125 percent of the poverty line or less.Footnote 37 These lawyers do yeoman’s duty for low pay. The need radically outstrips the supply and has basically since at least the 1980s.Footnote 38
In 2016 the Justice Index estimated that there were 6,953 legal aid lawyers, or 0.64 legal aid lawyers for every 10,000 eligible clients.Footnote 39 These lawyers and other volunteers far outpunch their numbers and help a massive number of people. The 2019 Legal Services Corporation report claims that “LSC grantees closed over 743,000 cases, including more than 231,000 Family cases and more than 214,000 Housing cases. In addition, LSC grantees helped more than 1.5 million people with legal education and information at court help desks and in workshops and clinics.”Footnote 40
How did approximately 7,000 lawyers handle so much work? They didn’t. They had a lot of assistance, often from non-lawyers, technology, or pro bono lawyers working around the edges of their license. LSC’s report itself baldly states: “Private attorneys, law students and graduates, paralegals, and other legal professionals were involved in closing 80,592 cases.”Footnote 41 There is roughly one paralegal for every three legal aid lawyers, and, given the workflow and a tsunami of client need, these paralegals naturally handle some (or a lot) of what might otherwise be termed “legal work,” from drafting documents to giving advice to prepping clients for court.Footnote 42
Nor has legal aid shied away from using technology to forward its mission. In 2013, LSC called a “Summit on the Use of Technology to Expand Access to Justice” that announced an aggressive new stance toward computerized solutions.Footnote 43 LSC created a technology grant program that has funded some of the most innovative work on providing basic legal services to those in need, as detailed below.
Legal aid is ground zero for the access-to-justice crisis, and they have, appropriately, tried to meet their statutory mandate to serve the legal needs of the poor by hook or by crook, which often means by bending UPL in ways that weirdly resemble BigLaw’s behavior. Non-lawyers do a lot of work. Volunteers, whether lawyers or not, do a lot. More recently, technology has come center stage and lets legal aid expand their reach exponentially. Once the time and money are spent to routinize a legal task and place it online, additional users are essentially free. Rather than trying to solve the access-to-justice crisis one case at a time with bespoke work, legal aid is trying to automate simpler matters to then focus their time and energy on areas of high impact or the greatest individual need. For decades, the brilliant legal futurist Richard Susskind has predicted this model of providing computerized legal services.Footnote 44 In the US the clearest examples tend to occur at the high end and the low end.
All of this is happening with little to no input from lawyer regulators, again for reasons that mirror BigLaw. Few to none of legal aid’s desperate clients are going to complain to bar regulators about tech solutions. Anecdotally, these clients are actually thrilled with these DIY-type services. Likewise, lawyers in private practice are not threatened because legal aid serves only the indigent, which is hardly a client base for main street lawyers.
To offer a personal example of this phenomenon, I run two programs here at the University of Tennessee that may edge toward UPL in spots: the A2J Lab and the Homeless Legal Advocacy Project. In the A2J Lab, I teach a class that tasks student teams with creating online guided interviews that result in desperately needed legal documents for poor Tennesseans. In the Homeless Project I bring groups of students down to a local homeless shelter every other week and we meet with the homeless, try to answer their questions, and if there’s a local legal case we can actually handle, we try to take it.
Sometimes when I describe this work to our alums or other groups of lawyers, I get some pushback as to whether either of these projects might run afoul of UPL. I point out to them that I’m a licensed lawyer and “oversee” this work (echoing the BigLaw/in-house/legal aid excuse), but I also say that as a law professor and gadfly in this area I’d be happy to defend this work against a UPL claim or any other regulatory complaint. This may explain why none has been forthcoming.
1.2.3 PeopleLaw
While UPL barely touches BigLaw and legal aid, the story is very different for what I call PeopleLaw – the small firm and solo practice lawyers that work for small businesses and individuals. The most recent American Bar Foundation survey (2005) estimated that around 75 percent of American lawyers worked in private practice.Footnote 45 Of those lawyers, a little fewer than half worked as solo practitioners, making solo practice the plurality status within the profession. If you add in the lawyers that worked in “law firms” of two-to-ten lawyers, you get closer to a full majority of lawyers. While not all of these lawyers work in PeopleLaw, it is fair to conclude that the biggest share of American lawyers work in this space, serving individuals and smaller businesses.
Most solo and small firm lawyers operate in a fashion that is largely unchanged from their predecessors from hundreds of years ago. Admittedly, some small firms and solos behave more like BigLaw (e.g., small boutique patent firms) or more like legal aid (a small firm that does only disability work or consumer bankruptcy work actually runs more like a legal aid office than a traditional solo practitioner’s office), but the bulk of the lawyers in this category are generalists, hustling to make it.
This market has been flooded with lawyers for decades and is highly competitive, resulting in shrinking earnings and an even clearer demarcation between “two legal professions.” In 1976, John Heinz and Edward Laumann conducted a groundbreaking study of the Chicago Bar Association and came to the then-surprising conclusion that there were essentially two separate legal professions: lawyers who represent individuals and made a decent living and lawyers who represent corporations and earned much more.Footnote 46 Heinz and his co-authors repeated the Chicago survey in 1995.Footnote 47 The distance between the two hemispheres had grown dramatically, with tremendous growth in the incomes and numbers of corporate lawyers, while lawyers in small firms, government, and public-interest practices constituted a declining proportion of the profession, and had lower incomes.Footnote 48 Many of the lawyers in the latter group experienced a loss in real income over the period.Footnote 49
IRS data shows the same. Every year from 1967 forward the Internal Revenue Service has gathered, anonymized, and released two different sets of tax returns from people who provide legal services: solo practitioners and partners.Footnote 50 The data show two clear trends. First, the gap between partners and solos has grown a lot over the last sixty years. In 1967 law firm partners earned about 2.5 times more than solos. In 2014 it was roughly 7 times as much. If you use the much smaller list of AmLaw100 partners, those partners earned 16 times as much as the average solo practitioner in 2014. Second, the gap between partners and solos reflects rising partner earnings, but also a collapse in solo practitioner earnings. Between 1967 and 2016 solo practitioners’ real income shrunk by a third.
If the regulatory structure and UPL are designed to prop up lawyer incomes, why have these lawyers struggled? Part of the answer is law school avarice and law grad overproduction. More law grads have crowded into this market and driven down earnings, particularly since the 1990s. Part of the answer is the more recent arrival of competition from tech providers like LegalZoom and Rocket Lawyer, who explicitly target this market and peel away at least some potential customers.Footnote 51
Part of the answer is the business model itself. In a market that has too many providers, one would expect prices to decline to match what demand exists. As the access-to-justice crisis establishes, there is hardly a lack of demand for legal services from the poor or middle class. To the contrary, the need is acute and growing. If there is copious need and there is market over-supply in lawyers, surely prices will fall to meet demand?
Sadly, no. This is because the vast majority of these lawyers are still practicing law in the old-fashioned, bespoke manner they learned in law school and from their predecessors: providing individualized services for a fee to individual customers. Given that IRS data suggests the average solo practitioner is earning less than $60,000, there just is not much room to lower prices, and in fact the average hourly rate for lawyers in this space remains stubbornly high. In 2016 Clio, a leading practice management software provider to small firms and solo practitioners, estimated that the average hourly rate for solo practitioners/small firm lawyers is $232 an hour.Footnote 52
If $232 is the average hourly rate, how are these lawyers possibly struggling? If they collected that rate on 20 hours of work a week, and worked 48 weeks a year, they should be grossing $222,720 annually.Footnote 53 Unfortunately, this is not the case. The same Clio report showed that in practice these lawyers actually are only able to collect on about 1.5 hours of billable work a day.Footnote 54 These lawyers are hardly lazy though. They bill more than they can collect from their clients and then spend the rest of their time hustling to find paying customers and running their small businesses.Footnote 55
Unlike BigLaw or legal aid lawyers, main street lawyers are actually pretty heavily regulated. These lawyers are the most likely to face client complaints, bar investigations, and the more serious punishments of public censure, suspension, or disbarment.Footnote 56 Critics of bar enforcement note that even these results are too infrequent to protect the public at large, but insofar as the regulatory system has teeth at all, it is in this market.
Humorously, this market is also the source of the great bulk of UPL complaints and prosecutions, almost always generating from the lawyers themselves rather than aggrieved members of the public.Footnote 57 There are, of course, unfortunate victims of scammers who claim to be lawyers and are not and then do little or no work. There has been a spate of these cases where “notarios” claim to be qualified to represent immigration clients and then steal their money.Footnote 58 Nevertheless, the bulk of the cases involve aggrieved lawyers complaining about UPL, reflecting that UPL has always been more focused on protecting lawyers than the public. As Deborah Rhode put it, “bar leaders and case doctrine insist that broad prohibitions on unauthorized practice serve the public, [but] support for that claim is notable for its absence.”Footnote 59
1.2.4 One Last Hybrid Category – Plaintiff’s Side Firms and Settlement Mills
The plaintiff’s side tort and class-action bar is sort of a hybrid of the other categories but is unique enough for some separate thoughts. First, unlike a more traditional generalist practice, plaintiff’s side firms tend to specialize more, and sometimes quite narrowly.Footnote 60 Second, the business model and client base is completely different, since plaintiff’s side firms almost always work on a contingency basis rather than charging by the hour or per activity. This means the client base spans from the very poor upward. In fact, these firms are more easily grouped by whom they sue (typically the deep-pocketed, whether wealthy individuals, insured individuals, or large businesses) than whom they represent. Third, the regulation hits quite differently here, as much of the most anti-competitive regulations, like limits on advertising or in-person solicitation, are squarely aimed at these lawyers.
Like other lawyers, it is quite typical for these lawyers to stretch the limits of UPL in the name of profit and efficiency. The best example is the settlement mill, which has a few titular lawyers at the top and is otherwise completely staffed and operated by lower paid non-lawyers. Nora Freeman Engstrom has pioneered the study of these firms.Footnote 61 Settlement mills run high-volume personal injury practices where their lawyers carry as many as 200–300 cases at a time.Footnote 62 How is this possible? Because non-lawyers actually do all of the work. Settlement mills almost never take a case to trial and actually try to avoid litigation altogether; they let non-lawyers handle intake and negotiating with insurance companies.Footnote 63 The titular lawyers “oversee” this work. UPL is again not typically strongly enforced in this market (although other regulatory and client complaints are quite common).
1.3 How Regulation, Culture, and Markets Have Shaped American Legal Tech
This section describes the current state of American legal tech from areas that are booming to areas that are in a slow burn. Unsurprisingly, the parts of the market that operate with less regulation are seeing explosive growth; the rest of the market, less so.
1.3.1 Penthouse to Outhouse – Boomtown USA
In America the very top of the market and the very bottom are leading the way in legal tech/justice innovations. The strangeness of this result should jump off the page. In what other market is the very bottom and very top the most forward- looking and aggressive technologically?
1.3.2 BigLaw
Large corporate legal departments are leading the way on legal tech, and BigLaw is following, sometimes reluctantly and sometimes aggressively, depending on the firm. Some readers will immediately object here. The individual lawyers that run BigLaw are by reputation notoriously tech-ignorant and change-averse. Above the Law summarizes: “Law firms are notoriously averse to change and, relatedly, technology. I worked with partners who responded to email with handwritten notes circulated via intra-office mail. I knew another who dictated responses to his secretary rather than draft an actual response.”Footnote 64 BigLaw’s internal systems are often outmoded. Their tech security lags. Some of the senior lawyers have declined to adopt email, let alone Slack.Footnote 65 True.
Bill Henderson has noted that trying to sell innovative technologies directly to law firms has often been a losing game, even when those technologies would increase firm-wide efficiency.Footnote 66 Why? Because law firms are risk-averse by nature and because in some ways law firms get paid for their inefficiencies. Telling a business that primarily runs on hourly fees that you can make them more efficient is not necessarily a winning strategy.
But don’t mistake the employee’s reticence for the employer’s desires. If there is a single story of corporate legal work over the last decades, it is cutting costs, increasing efficiency, and using technology to replace humans wherever possible.Footnote 67 Some press reports on legal tech seem to suggest that this is a new process, but it has been going on for years. It started with “in-sourcing.”Footnote 68 In-house legal departments have grown relentlessly and are incentivized to seek cheaper solutions. Simple work is sloughed off to non-lawyers. More complicated work is offshored to cheaper jurisdictions like India.Footnote 69 AI (like the COIN program described earlier) can handle mundane contract or litigation related tasks.Footnote 70 eDiscovery is a prime example.Footnote 71 In-house counsel break legal work into constituent parts and only hire expensive outside counsel for sui generis or critically important areas.Footnote 72 Everything else can be handled in the most efficient and cheapest manner.Footnote 73
The year 2020 was the biggest yet for investment in legal tech startups. The programs covered a bevy of corporate legal needs, including contract analytics and contract review, automated legal spend management, eDiscovery companies, and knowledge-integration platforms.Footnote 74 I have two reactions to the explosion of investment and activity in this area. On the one hand: WOW. The full span of the work is incredible, and after a slow start the market for these sorts of disruptions seems to be really humming. As late as 2011, Gillian Hadfield was asking “where are the ‘garage guys’ in law?”Footnote 75 This question has been answered emphatically.
On the other hand, these innovations often reflect what machines are currently excellent at and what humans sometimes struggle to accomplish: using repeatable and clear rules to wrangle a large quantity of data or documents into a useful format for humans. Computers are already excellent at rules-based legal work. Interactive document production programs from LegalZoom, Rocket Lawyer, or Community Lawyer are other examples. Humans can get tired or bored with these tasks and make mistakes, or they can be too expensive. Once a task is automated, computers are essentially free and never tire.
But current computer programs still struggle with more human tasks that require more nuance and judgment.Footnote 76 In contrast to eDiscovery, due diligence document review has been harder to mechanize, because rather than asking a machine to find a mass of documents with common features (emails about an accident, or featuring legal advice, for example), due diligence before an acquisition often searches a company’s data for weird or non-compliant documents that signal the existence of something undisclosed and potentially harmful (like a future legal liability or some kind of underlying fraud).Footnote 77 Again, computers have improved at this task, and not all due diligence requires a search for anomalies. Still, as you might guess, telling an algorithm to search for things “that might signal a future legal liability” is trickier than an eDiscovery search for all the emails about a particular deal.
1.3.3 Access-to-Justice Work for the Poor
Legal Aid and others are aggressively pursuing tech solutions to legal problems through grant programs and working with community partners.Footnote 78 As an overview, consider the 2019 LSC-funded projects, which are aggressive, creative, and if you care about access to justice, heartening.Footnote 79 The projects can be divvied into two baskets: automating legal processes or sharing legal information with the poor.
Here’s a sampling of the processes LSC is seeking to automate: (1) filing for disability benefits in Alaska; (2) filing for consumer debt relief in California; (3) intake for Spanish-speaking clients in Atlanta; (4) making fair housing complaints in Michigan; (5) creating an automated legal help chatbot in Michigan; (6) establishing legal self-help document assembly software in New Hampshire for housing and consumer law problems; (7) online intake and referral in Pennsylvania; (8) filing for consumer bankruptcy (with an assist from consumer bankruptcy tech pioneer Upsolve).
Legal Aid also supported the following new sources of legal information: (1) online tutorials in landlord-tenant law in Georgia; (2) online “classrooms” covering six different areas of poverty law in South Carolina; (3) self-help videos covering evictions and orders of protection in Vermont; (4) redesigns of websites to include much more free information and forms in Kentucky and Minnesota.
This is just a snapshot of the projects in one year! And note that because of the nature of technology, every one of these projects makes the next project easier. Local law and information are not always transferable (although federal law like bankruptcy is easier), but the technology platforms and processes are absolutely transferable, especially here in a non-profit setting where all the players are on the same team and happy to share.
Further, Legal Aid is hardly fighting this battle alone. Felicity Conrad, one of the founders of the pro bono tech platform Paladin, recently launched a database that lists “justice tech” startups.Footnote 80 Conrad describes the “justice tech” sector as “a new breed of legaltech – instead of focusing on modernizing the existing legal services market (contracts, practice management, legal research, etc.), their goal is to leverage technology to directly scale legal services to the billions of people underserved by the existing market.”Footnote 81 I will not endeavor to describe all twenty-seven listed entities (as of January 2021), but if you want to feel like technology might actually be doing some good in the world, I strongly encourage you to go to the link and read the spreadsheet. It covers areas from reporting police misconduct, to recording police interactions, to the “do not pay app” for challenging corporate fees and parking tickets, to landlord-tenant, divorce, and immigration services.
There are also a handful of law schools working in this space. Stanford’s Center on the Legal Profession, built by and around Deborah Rhode and her decades of leadership in legal ethics, feminism, and access to justice, has been a world leader. Margaret Hagan’s Legal Design Lab was originally housed within the Center and focuses on what she calls “human facing” technology, creating apps and programs that help ordinary folks navigate complicated court processes and legal problems.Footnote 82 Suffolk Law School has a Legal Innovation & Technology Lab and Chicago–Kent a Law Lab, among others.
My A2J Lab at the University of Tennessee is a more modest example. We use the excellent Community Lawyer platform to create the interviews and have automated processes to help with immigration forms, orders of protection, convictions expungement, and debt relief. On the one hand, the class is embarrassingly easy to teach, and the platform and our non-profit and legal aid clients do all of the really hard work. On the other hand, I’ve taught the class for three years, and we’ve had a pretty big impact in Tennessee! This area is packed with low-hanging fruit.
Some courts are also leading the way, especially those courts on the front lines of the pro se crisis. Courts started to complain about a rise in pro se litigation as far back as the 1980s, but this century the problem has ripened into a true crisis. Courts’ and clerks’ offices found themselves buried under miserable and angry pro se litigants and could not help but notice that case results, and thus justice, were heavily affected. Encouragingly, many judges and court administrators have gone to work, often using technology, process reforms, or a combination. The pandemic has likewise helped, as online court went from a theoretical idea to a necessity. The trend toward online dispute resolution (ODR) has likewise accelerated, as the chapters by Spalding and Prescott establish. ODR is often pitched as an efficiency measure, but when used in pro se courts, it is also an access-to-justice effort, because these programs are often designed to be used by ordinary people and not lawyers. Like David Engstrom and colleagues’ work on AI in government, courts present different, and in some ways more promising, use cases than legal work for individuals.Footnote 83
These projects are blossoming despite potential regulatory and lawyer opposition because few want to push back against large government entities like courts or legal aid, but also because these projects to do not typically compete against flesh-and-blood lawyers, who barely try to serve the middle class, let alone the poor. Some of these projects, especially those aimed at areas where lawyers wish to remain dominant and at least somewhat expensive, like consumer bankruptcy or divorce, have faced some pushback, of course. But overall the A2J tech revolution continues apace.
1.3.4 Seas Were Rougher, Clear Sailing Now? – Interactive Forms
The provision of legal forms to the middle class and small businesses initially faced tougher sledding. The public face of American legal tech are LegalZoom and Rocket Lawyer. Computerized legal services (online or via cellphone apps) for the consumer market are expanding at a dizzying rate and in direct competition to main street lawyers. If you are a typical lawyer, you may have some idea what these sites offer, but probably have not taken a spin through them recently. I strongly recommend you do, just so you understand the competition.
Both LegalZoom and Rocket Lawyer offer guided interviews and document assembly (or more basic forms) for virtually any kind of written legal document that is not meant to be filed directly in court, and some that are meant for court. They offer everything from LLC formation to wills to contracts to internet terms of service. You name a legal document, they offer it, and often at a radical discount to what a human lawyer would charge. Have you heard of the basic $500 will offered by your local solo practitioner? LegalZoom’s basic will starts at $89.Footnote 84 LLC University estimates that a lawyer will charge between $1,000–1,500 to form a basic LLC.Footnote 85 LegalZoom’s cost? Seventy-nine dollars plus filing fees.Footnote 86
Given that these are bread-and-butter matters for main street lawyers, it is stunning that LegalZoom and Rocket Lawyer survived UPL. How did the lawyer regulators fail? Partially due to Silicon Valley chutzpah, partially due to poor timing, and partially due to the shifting sands of antitrust and public opinion. But currently there can be little doubt that the horse is out of the barn, and these forms providers seem destined to remain stiff competition for lawyers.
First, consider the gall of LegalZoom. Its founders were surely aware that providing forms and interactive document creation online could violate UPL or skate close to the line. Yet, they went forward guns blazing. LegalZoom was launched in 2001 and it was hardly a sneak attack. It recruited former O. J. Simpson lawyer Robert Shapiro as a co-founder and launched an all-out PR blitz.Footnote 87 This is, of course, a well-known Silicon Valley strategy, most famously pursued by companies like Uber or Airbnb. Plow into a legal gray area and assume/hope that you will be able to grow fast enough to eventually beat back any regulatory resistance.Footnote 88
Second, these companies had some great good luck in terms of initial regulatory disinterest. LegalZoom launched in 2001 but did not face any serious UPL investigations and prosecutions until 2008.Footnote 89 Why? Because of the brokenness of the market for legal services in the United States. Most main street lawyers still focus on very expensive, bespoke, hourly services. Most middle-class customers and small businesses cannot afford these services. This means that solo practitioners (or their receptionists) spend a lot of time explaining to potential customers that they cannot afford to hire a lawyer. These non-clients were the first adopters of LegalZoom and Rocket Lawyer. Fans of Clayton Christensen’s Innovator’s Dilemma will recognize this exact process, as the innovative upstart focuses on the lowest-end, least-valuable work, before moving up the ladder to more profitable areas.Footnote 90
When LegalZoom started, its products were not as polished as they are now, so the first wave of LegalZoom customers were not really a loss to lawyers at all. These folks likely couldn’t afford a lawyer’s services. Over time, of course, LegalZoom got better, and lawyers started to notice a pinch on their business. But by then years had passed and LegalZoom was pretty well established.
That said, the first UPL cases were still somewhat ominous. In Washington State in 2011, LegalZoom settled a UPL investigation by paying $20,000 in costs.Footnote 91 That same year in Missouri it paid the state’s attorney’s fees and changed some parts of its site for Missouri customers.Footnote 92 The Missouri case was particularly worrisome because the district court there concluded in dicta that LegalZoom’s interactive forms were very similar to the provision of legal services by a lawyer and thus were likely unlawful as UPL.Footnote 93
At this point LegalZoom decided to take the fight to the regulators, responding to a UPL sanction by the State Bar of North Carolina with a lawsuit alleging antitrust and constitutional violations.Footnote 94 When the Supreme Court decided that North Carolina’s own dental board had violated antitrust law in 2015, the State Bar quickly settled, and on very favorable terms to LegalZoom.Footnote 95 Not coincidentally, this launched a series of LegalZoom wins on UPL, including in South Carolina and Arkansas.Footnote 96 These decisions have put LegalZoom in a good position and plowed the road for Rocket Lawyer and others.
Last, LegalZoom could count on public opinion. Just as Uber has leveraged public distaste for overweening regulators (overweening according to Uber at least),Footnote 97 LegalZoom could count on some political pressure if it was fully driven from any given state. For example, in the late 1990s the Texas Bar Association attempted to ban a program called “Quicken Family Lawyer” as UPL, only to be overruled by the Texas legislature.Footnote 98
Future signs are mixed. On the one hand, recent UPL developments look good, and the decisions by Utah, Arizona, and others to allow for more provision of legal services by non-lawyers and technology certainly suggests future growth.Footnote 99 On the other hand, today’s announced loosening sometimes leads to tomorrow’s tightening. The 2016 ABA Report on the Future of Legal Services’ seemingly innocuous suggestion that legal tech should be regulated to protect client interests is worrisome given the history of lawyer regulation that allegedly protects the public but does more to protect lawyers.Footnote 100
1.3.5 Controls Are Tighter, but Technology Is Still Coming: Legal Advice
If you are wondering whether the market for interactive forms alone is enough to meet the unmet demand from the middle class and small businesses, consider that both LegalZoom and Rocket Lawyer have legal advice subscription offerings staffed by licensed lawyers. Both sites try to sell these plans along with their basic forms.Footnote 101 For a monthly fee subscribers get a set amount of “free” legal advice from licensed lawyers. These plans skirt the regulations that make fee-splitting with non-lawyers illegal because the lawyers themselves are not paid for any individual consultation. Instead, they get a “small cut” of the aggregated monthly fees.Footnote 102 Why would lawyers give what essentially amounts to free legal consultations that mostly serve to enrich their online competitors? Business development. Some of these advice clients may eventually have a “real” case – that is, a case where they will pay a lawyer an hourly rate for more valuable legal work. Yes, the market is so tight for these lawyers that it’s worth offering “free” consultations just for the hope of generating more useful business.
Other sites like Avvo offer searchable, free legal advice from lawyers.Footnote 103 Potential clients ask a short question, and lawyers volunteer to answer.Footnote 104 The answers are publicly available and searchable. Lawyers do this in the hope that some of the answers will lead to actual paying clients and also because Avvo is a lawyer rating service (like TripAdvisor or Yelp for lawyers) and answering questions can help their ratings.
Avvo used to offer a legal services plan that directly connected consumers and lawyers, but bar regulators closed it down for fee-splitting and other alleged violations.Footnote 105 If you are wondering whether bar regulators still have teeth, the answer is yes when it comes to direct provision of legal advice or services for a fee.
1.3.6 Little Immediate Help: Filing Papers or Appearing in Court
Historically, prohibitions on UPL have been at their strongest in courthouses. Non-lawyers are rarely allowed to represent others in court proceedings or to sign and file papers on their behalf. This is less true in administrative hearings, but generally the more formal the procedural setting, the less the tolerance for non-lawyer assistance.
The actual drafting of legal documents is, of course, subject to encroachment by computerization. The chapter by Nyarko and Frankenreiter establishes that we may still be some ways away from any really high-level work in that regard.Footnote 106 As such, the actual filing of papers and appearing in court will remain a human’s only proposition for at least as long as judges prefer talking to humans than robots.
1.4 So What Does the Future Hold?
America will continue to be a world leader in legal tech, regardless of its regulatory structure. The current American demand for legal services is currently grossly underserved by lawyers and their focus on selling bespoke services. There is a massive amount of low-hanging fruit, and the lure of disruption will continue to draw innovators and investors.
Law tech in America will continue to thrive most in the areas that are regulated the least, at the highest and lowest ends of the market. We will continue to see rapid automation of legal processes for the poor and corporate clients, bounded mostly by technological barriers rather than regulatory barriers.
Wealthy corporations will naturally get first access to innovations like eDiscovery or the use of AI to predict legal outcomes. These technologies involve large startup costs, so will be expensive and exclusive at first. Nevertheless, computer processes are hard to pen in once created and mastered, since the marginal cost of adding new customers can run as low as zero. We can expect at least some leakage downstream, and over time these tech innovations should make law cheaper and better even for main street lawyers and the public at large.
The poor will have some advantages! Regulators have shown remarkable tolerance of tech solutions offered by legal aid or non-profits. Likewise, all the most exciting courtroom innovations, such as ODR, will continue to arrive first in small claims or other pro se courts. In my book Glass Half Full I talk about these innovations offering the possibility of a truly bizarre science fiction experiment: a world where courts for the poor are fairer and more efficient than courts for the rich.Footnote 107
Further, and this is not necessarily a good thing, legal work for the poor does not need to be perfect, just better than nothing. Corporate clients will only accept automated processes that work perfectly or near perfectly. Technology aimed at the poor, like interactive order-of-protection forms, face a lower bar. Let me be absolutely clear, there are significant reasons to feel uncomfortable with this fact of American life, but still, it is what it is. The bar for an interactive form for the poor is often “Is it better than nothing?” This allows for more aggressive experimentation.
That said, making the perfect the enemy of the good is part of the reason we are mired in the current access-to justice crisis. We cannot afford to offer BigLaw style services to the poor for free, and of course the middle class could never afford such services. Rather than pretending that is our goal, realistic attempts to do our best should be encouraged and accepted. The status quo is so bad that experimentation and triage are not only recommended; they are desperately needed.
As Engstrom and Gelbach aptly note, technical challenges in natural language processing will make advances in automated legal advice or reasoning slower and on a different time horizon.Footnote 108 Note that even in looser regulatory environments, such as England or Australia, we have not seen anything resembling a for-profit “robot lawyer,” at least partially due to the current limitations on natural language processing. Chatbots that handle intake or more basic questions exist now and will, of course, improve and expand over time. For now, at least, most of these applications will require human lawyer inputs, so they will exist “under a lawyer’s supervision.” As long as a lawyer is even nominally involved, regulators are likely to be copacetic. In-person work, especially in court, stays the province of lawyers for the longest time.
The current regulatory atmosphere, where the top and the bottom of the market are essentially unregulated, is, of course, not set in stone. In fact, seemingly salutary efforts like those in Utah, Arizona, and other states could turn sour quite easily. Historically, most lawyer-driven consumer protection efforts like UPL or the bar exam eventually tend to favor lawyers over the public.Footnote 109 Reform advocates have taken to calling the Utah approach “re-regulation,” to avoid the implication of willy-nilly deregulation. But these reforms might turn out to be “re-regulation” in the strong sense of bringing regulation (via a sandbox entry process or other routes) that currently doesn’t exist for much of the market, especially at the high and low ends of the market. We must be vigilant to avoid the “we’re from the Bar Association and we’re here to help” type of regulation.Footnote 110
In my preferred version of the future, lawyers of all stripes leverage technology to work more efficiently and to focus on the work that humans are especially excellent at: talking to other humans, high-level reasoning, and strategic thinking. There is a version of the future where technology solves the access-to-justice and related pro se crisis and makes an immeasurable difference in the lives of the poor and middle class in America and all over the world. Done properly, technology could even improve the lives of lawyers, as they spend more of their time doing the activities that likely drew them to the profession in the first place and less time on repeatable grunt work. I have, admittedly, been called a Pollyanna on this front, with Deborah Rhode’s reactions alternating between “good luck with that” to the more generous “from your lips to God’s ears.” For the millions of Americans with unmet civil justice needs, we can all hope the latter ends up the more apt of the two.
The legal services market is commonly thought of as divided into two “hemispheres” – PeopleLaw and BigLaw.Footnote 1 These segments represent, respectively, individuals and corporate clients. The last few decades have seen an increasing concentration of resources in the legal market toward serving corporate clients, to the alleged detriment of consumer clients. At the same time, the costs of accessing legal representation exceed the financial resources of many ordinary citizens and small businesses, compromising their access to the legal system.Footnote 2
We ask: will the adoption of legal tech – new digital technologies in legal markets – lead to a leveling of the playing field between the PeopleLaw and BigLaw sectors? By “leveling,” we mean convergence of business modelsFootnote 3 in the two sectors so as to deliver a more equal opportunity to access legal services. Convergence in business models would enable legal service providers to exploit opportunities for scaling and cost reduction, and to meet more of the unmet legal needs of clients.Footnote 4 We focus, not on what legal tech in theory may be capable of doing, but on which use cases are likely to emerge in practice and take root. In order to do so, we use a causal framework that takes account of organizational complementsFootnote 5 and regulatory constraints to the adoption of emergent business models.
By leveraging the regulatory differences between the UK and the US, we aim to gauge the relative importance of regulatory shifts in meeting unmet legal needs.Footnote 6 In 2007, the UK enacted a bold set of reforms.Footnote 7 By reducing the substantive domain over which lawyers have exclusive service rights, and by permitting alternative business structures (ABS), the intent was to stimulate competition and thereby more cost-effective legal services to meet latent demand.Footnote 8 Similar proposals have long been debated in the US,Footnote 9 and some states have taken tentative first steps.Footnote 10 Our comparison of secular trends in the UK (England and Wales, strictly speaking) and the US demonstrates that regulatory reforms may be necessary but not sufficient to bring about desirable changes in meeting latent legal demand.
This chapter proceeds as follows. Section 2.1 sets the scene with an overview of the development of the PeopleLaw and BigLaw sectors in Britain and the United States in the last few decades. Section 2.2 examines the adoption of legal tech in the BigLaw sector, to identify specific use cases and complementary changes taking place (or not taking place) to adopt emergent business models. Section 2.3 conducts a similar exercise for the PeopleLaw sector. Section 2.4 then provides an explicit comparison of the two sectors to address the question of whether or not legal tech will enable a convergence in the extent to which consumer or client needs are met. Our conclusion is that while legal tech and data aggregation have enormous potential to meet unmet legal needs, different constraints continue to hold back the realization of such potential. Major barriers are human capital and data aggregation in BigLaw, and financial capital and the technological limits to automating human lawyers in PeopleLaw. We discuss some regulatory policy options that might promote greater convergence between the two sectors.
2.1 Overview of PeopleLaw and BigLaw Sectors
This section provides a macro-level overview of the state of PeopleLaw and BigLaw sectors.Footnote 11
Differences in data availability mean the contours of these sectors can be outlined in sharper focus for the US than the UK. But in both countries, there is evidence that the share of PeopleLaw activities relative to BigLaw has been in secular decline over the past few decades. The similarity in this secular trend is striking, given that reforms have been under way for over a decade in the UK, whereas they have only just begun in some US states.
In the US, lawyers have long been aware of a distinction made between the part of the legal market that provides services to sizable corporate clients and the part that does not. This divide was brought to prominence in the seminal work Chicago Lawyers: The Social Structure of the Bar.Footnote 12 The study found that Chicago lawyers in 1975 devoted 45 percent of their total effort to services for individual or small business clients, unions, environmental plaintiffs, and state administrative agencies or municipalities. The same survey repeated in 1995 showed that this PeopleLaw proportion had declined to 35 percent of lawyers’ total effort.Footnote 13
A similar story emerges from US Economic Census Data reporting spending on services provided by lawyers employed in private practice. In 2005, 39 percent of this was attributable to individual clients.Footnote 14 By 2007, the share of PeopleLaw in total revenue was 29 percent; by 2012 it had declined to 24 percent, while the share of BigLaw grew over the same period from 68 percent to 73 percent.
Henderson also highlights striking differences between the economics of PeopleLaw and BigLaw. In PeopleLaw, lawyers (typically sole practitioners) charged an average $260 per hour (data source: Clio), but billed for only 1.6 hours per day, amounting to $422 a day, or $105,000 in gross receipts over a fifty-week year. In BigLaw by contrast, Am Law 100 total gross revenue in 2012 was $71 billion, with a total lawyer headcount of 86,272. So, average gross revenue per lawyer was $822,978, while average profit per partner was $1.48 million.Footnote 15
The problem of access to justice for consumers led a handful of state bars to address this issue in recent years.Footnote 16 Regulatory reforms are intended to permit new providers including human non-lawyers and non-human non-lawyers (i.e., software) to operate in legal markets. It is too early to tell how these reforms would begin to meet the latent demand of consumers. For now, we know much more about cases of unauthorized practice of law by technology providers.Footnote 17
The UK’s legal services market, with a total turnover of £24 billion in 2017, is about a sixth (17 percent) of the size of the US market.Footnote 18 Unfortunately, UK national statistics do not shed light directly on the relative size of PeopleLaw and BigLaw sectors. Instead, we present two alternative approaches to gauging trends in two spheres of legal markets.
One approach is to break down law firm revenues into practice areas that predominantly serve individuals (B2C) and others that predominantly serve corporate clients (B2B). A recent study by KPMG reports that 60 percent of law firm turnover in England and Wales is in B2B and 20 percent in B2C.Footnote 19 This 60 percent does not take account of growth in in-house lawyers, rising from 16 percent of all solicitors in 2004 to 23 percent by 2019.Footnote 20 Because this growth is directed at corporate work, it is strongly suggestive of a corresponding decline of PeopleLaw’s relative share in the overall legal services market.
Another approach draws on UK survey evidence investigating latent consumer demand for legal services. While differences in survey methodology over time impede identifying secular trends, the data clearly suggests that the legal services market is not meeting the needs of consumers. Adults based in England and Wales were asked about the legal issues they experienced in the four years prior to the survey and the help they sought in order to resolve them. In 2019,Footnote 21 the majority of respondents’ legal needs remained unmet in all of the most commonly encountered types of legal issue, from family issues and property matters to labor and employment and personal injury.Footnote 22 While there are numerous reasons legal needs may be unmet, a significant component appears to be the perceived inaccessibility of the civil justice system.Footnote 23
Policy concern over the unmet legal needs of consumers informed a major overhaul of UK legal services regulation in 2007.Footnote 24 This permitted for the first time ownership of law firms by non-lawyers through so-called Alternative Business Structures (ABS).Footnote 25 It is striking that the UK’s PeopleLaw sector has continued to shrink relative to BigLaw, and that high levels of unmet consumer legal needs have persisted, despite these wide-ranging reforms.
To summarize, the PeopleLaw sector represents only a small fraction – estimated to be about a fifth to a quarter – of the overall legal services market in both the US and the UK. The evidence suggests that this fraction has been in secular decline in both countries for the past few decades. Moreover, UK citizens’ unmet legal needs are not adequately addressed despite the major overhaul in legal services regulation a decade ago. We now turn to consider how technology has been impacting the provision of legal services in each of the two hemispheres, BigLaw and PeopleLaw, respectively.
2.2 Digital Technology in the BigLaw Sector
This section analyzes how digital technology is influencing the work of lawyers and emergent business models adopted by law firms and other providers in the BigLaw sector. A “business model” is a focused way of understanding how client needs are met, in ways that translate into sustainable profit-making for providers.Footnote 26 We first briefly survey digital technologies being deployed in BigLaw, focusing in particular on AI, and distinguish these from earlier waves of automation. We conclude that despite the availability of new business models, significant constraints remain in the form of human capital rather than financial capital, preventing BigLaw law firms from deploying technology more effectively.
2.2.1 Technology Use Cases in BigLaw
“Legal tech” may be defined as technology that supports or enables the provision of legal services. It is a broad category, encompassing the use of interactive websites, electronic documents, and elements of artificial intelligence (AI) to automate the review and prediction from text, and automation of workflow and matter management. In the BigLaw context, users distinguish between technologies supporting the “practice of law” – that is, supporting the delivery of legal services themselves – and those supporting the “business of law” – that is, supporting the management of client relationships and the allocation of human resource internally.
In the practice of law, a core system for most law firms and corporate in-house teams is document management, which provides digital indexing for legal services work product. Closely related to this, but more varied in their implementation, are knowledge management systems, which seek to aggregate content, including prior work done by the firm, in ways that are relevant and accessible for busy professionals. Increasingly, firms and corporate in-house teams are also making use of workflow automation platforms applying what in other industries might be referred to as robotic process automation (RPA) – that is, the automation of scalable and repetitive tasks. Increasingly common too are the use of extranets or digital deal-rooms that provide secure repositories of data that are accessible by the lawyers and clients or others outside their team. In large-scale litigation, or corporate transactions, there are typically huge volumes of digital documents that are provided by outside parties and need to be reviewable by a range of personnel across organizational boundaries.Footnote 27
The deployment of AI differs from earlier generations of automation in that it requires training to parameterize a model that best classifies items of a particular category.Footnote 28 Training is done using a set of data labeled according to the variable of interest. This training requires data – the more the better – that is relevant and accurately labeled. In the litigation context, AI is now commonly used to identify potentially relevant documents in a pre-trial discovery exercise.Footnote 29 This necessitates the training of new models for each suit, based on aspects identified as “relevant” by expert human reviewers. In the transactional context, AI is increasingly deployed to review contracts. In-house teams train AI systems to review their company’s everyday or “business as usual” contracts; BigLaw firms by contrast train AI systems to do due diligence, reviewing a large corpus of an M&A target’s contracts to identify clauses that may pose problems for buyers (such as change-of-control clauses).Footnote 30 In each case, the training requires legal expertise.Footnote 31 Moreover, increasing numbers of legal practitioners are making use of AI in support of their legal research, training tools that complement the offerings of the big legal data providers.Footnote 32
In the “business of law,” technology is widely used to support accounts and time recording. BigLaw firms are beginning to deploy AI-based systems both to enhance and leverage the data from these earlier systems. An appropriately trained model can both help to fill gaps in time recordings and predict the likely time budget for new instructions. This opens up the possibility of output-based pricing, as opposed to the traditional input-based model of the billable hour. Similarly, lawyers are increasingly turning to customer relationship management (CRM) systems to support marketing and client relationships. These systems themselves increasingly make use of AI.Footnote 33
2.2.2 Augmented Lawyering and Business Models in BigLaw
Focusing on the adoption of AI, survey and interview evidence suggests that it has multiple impacts on lawyers’ work.Footnote 34 The most well-understood effect is substitution – that is, AI systems replacing humans for repetitive, scalable tasks. At the same time, lawyers’ work in giving bespoke advice is augmented by the use of AI, freeing up their capacity to deliver judgment-oriented tasks for which humans remain uniquely capable. Here, lawyers are consumers of AI systems’ outputs, which augment the quality of their advisory work. Third, the deployment of AI creates new tasks for humans, necessitating a multi-disciplinary mix of skills and expertise – not just legal but also data science, information security, process and project management, and user experience. Where lawyers work in such multi-disciplinary teams (MDTs), they are helping to produce AI-enabled legal services.
Having characterized these three distinctive ways in which AI affects lawyers’ work, we need to determine where in the BigLaw ecosystem we can observe these in practice. The “ecosystem” consists of corporate clients as the ultimate customer, serviced by law firms – the traditional “outside counsel” – and by a rapidly growing congeries of other providers including law companies and legal tech providers (see Figure 2.1). In prior work, we sought to organize these relationships by reference to business models, which clarify how value is created for clients.Footnote 35 We distinguish between a traditional “Legal Advisory” business model, which entails the provision of customized advice or analysis, a “Legal Operations” business model that enhances efficiencies in legal workflows, and a “Legal Technology” business model that focuses on the development of technological systems for legal services.Footnote 36

Figure 2.1 The BigLaw ecosystem
The Legal Advisory model focuses on work that requires skills that for the foreseeable future remain distinctively human; lawyers rely on AI predictions to augment their work. By contrast, in both the Legal Operations and Legal Technology business models, technology substitutes for humans in certain tasks. Technology in these business models also engenders new tasks, most obviously in young legal tech ventures, but also in large law firms and in-house corporate legal departments.
We characterize these business models as ideal types; in practice, experimentation in combining different business models is rife. For instance, some law firms have developed internal legal operations expertise and/or have an in-house legal tech capability either via organic growth or acquisition. In the US, Wilson Sonsini has a wholly owned subsidiary in the form of SixFifty, and in the UK, Simmons & Simmons acquired Wavelength Law, a legal tech provider. At the same time, notable law companies have used legal operations excellence as a launchpad to move into legal advisory work. Elevate has its in-house law firm, Elevate Next, and UnitedLex created a subsidiary law firm, Marshall Denning. In the meantime, law companies and legal tech providers are partnering with law firms to access premier corporate clients.Footnote 37
Combining all three business models – Legal Advisory, Legal Operations, and Legal Technology – under one roof in an integrated legal management company might be desirable from the point of view of providing a one-stop shop for corporate clients. However, such integration creates tensions in strategic focus and reputational capital. In particular, employing both lawyers-as-consumers of AI and lawyers-as-producers of AI under one roof is challenging, not least due to the need to establish career paths to integrate, or segregate, the two types of lawyers. As it stands, these career paths are yet to be clarified. And some firms are implicitly signaling which type of lawyer – lawyer-as-consumer or lawyer-as-producer of AI – they prioritize as their core human capital. Thus, human capital challenges, rather than the challenge of accessing external finance, are more central to the difficulty that law firm partnerships face in sustaining effective deployment of digital technology, including AI.Footnote 38
2.3 Digital Technology in the PeopleLaw Sector
Legal tech also has potential to unlock capacity to address consumers’ legal needs in PeopleLaw. Just as in BigLaw, technology promises to lower costs of delivery for PeopleLaw through exploiting economies of scale. However, there are differences in the way legal tech systems add value in the two contexts. For BigLaw, as we have seen, this is through a mixture of substitution by automated systems for some human tasks (lowering costs) and augmentation of high-value human work on bespoke tasks (enhancing productivity). In PeopleLaw, the tasks are in general more routine in nature, meaning there is relatively more potential for value to be created by legal tech through lower-cost substitution. As we shall see, technology adoption is as yet more limited in the PeopleLaw than the BigLaw context. We argue that this is determined by financial and technological constraints that currently limit opportunities for scaling legal tech to substitute for human lawyers.
We identify two significant constraints on the adoption of legal tech in PeopleLaw in contrast to BigLaw. First, PeopleLaw firms are generally much smaller than their BigLaw counterparts, limiting their ability to invest fixed costs necessary to deliver automation. Second, the fact that PeopleLaw clients are not usually versed in the law (unlike the in-house counsel who typically purchase BigLaw services) means that technical solutions substituting for lawyers must also perform a lay-to-legal (and vice versa) translation function. We develop these points below.
We can usefully distinguish barriers to technology adoption in PeopleLaw that stem from the demand and the supply sides of the market, respectively. The demand side, discussed in Section 2.1’s consideration of the BigLaw and PeopleLaw segments of legal services markets, is well understood. To recap, there are persistently high levels of unmet legal needs in many issue areas (see Figure 2.1), owing to individual (that is, non-corporate) consumers’ inability to identify their problems as legal in natureFootnote 39 and, for problems recognized as legal, the perceived inaccessibility of the justice system and the costs of accessing lawyers. Survey evidence suggests consumers’ budget constraints are binding.Footnote 40 Many consumers are unable to afford a lawyer to advise them whenever they have a problem at home (divorce, child custody, debt collection, etc.), at work (employment dispute), or when moving home (immigration, conveyancing, etc.). The adoption of legal tech could help resolve currently unmet legal needs by lowering the unit cost of legal service delivery, particularly in settings where such legal services can be productized.Footnote 41 In transactional contexts, this can take the form of providing standardized document templates or transaction-processing pipelines.Footnote 42 For contentious matters, this could involve technologically enabled dispute resolution mechanisms (commonly referred to as online dispute resolution, or ODR) that are quicker and simpler to execute than traditional court proceedings.Footnote 43
Despite the clear potential for technology to meet latent demand, adoption of technology by solo practitioners and small law firms – the sort that traditionally service individual clients – remains lower than in larger firms. Several recent surveys in both the UK and US have asked firms about deployment of emerging technologies (see Table 2.1), and have consistently found that small firms are less likely to have done so.Footnote 44 Why does this pattern emerge so consistently in both the UK and US, notwithstanding the considerable unmet legal needs in both countries, and the UK’s reforms designed to liberalize legal services for the benefit of consumers?
Table 2.1 Use of digital technology by type in the UK in 2021Footnote 45
Which of the following legal technologies are you currently using, or planning to use, in your firm? N = 891 | Currently using | Planning to use | Not planning to use | |||
---|---|---|---|---|---|---|
N | Row % | N | Row % | N | Row % | |
Videconferencing with clients | 770 | 86.4 | 48 | 5.4 | 73 | 8.2 |
Model documents/templates on our website | 217 | 24.4 | 149 | 16.7 | 525 | 58.9 |
Interactive website to generate legal documents in response to client input | 88 | 9.9 | 173 | 19.4 | 630 | 70.7 |
Chatbots or virtual assistants | 55 | 6.2 | 125 | 14.0 | 711 | 79.8 |
Online portals for matter status updates | 137 | 15.4 | 189 | 21.2 | 565 | 63.4 |
E-verification/electronic signatures | 332 | 37.3 | 226 | 25.4 | 333 | 37.3 |
Storing data in the cloud | 587 | 65.9 | 102 | 11.5 | 202 | 22.6 |
Practice management software | 550 | 61.7 | 87 | 9.8 | 254 | 28.5 |
Legal research software | 449 | 50.4 | 90 | 10.1 | 352 | 39.5 |
Contract review software | 65 | 7.3 | 120 | 13.5 | 706 | 79.2 |
Blockchain/distributed ledger | 16 | 1.8 | 74 | 8.3 | 801 | 89.9 |
Data analytics with AI | 45 | 5.1 | 92 | 10.3 | 754 | 84.6 |
Two complementary factors seem relevant. The first relates to fixed costs associated with deployment of automated systems. Small firms, such as those involved in PeopleLaw, have more limited capacity to bear fixed costs than do larger firms.Footnote 46 This means that technology is likely to penetrate first into BigLaw firms. It also implies that deployment in PeopleLaw is likely to be preceded by consolidation of service providers, and that constraints on external finance are likely to be more of a barrier to legal tech deployment for PeopleLaw than BigLaw.Footnote 47
Second is the challenge of translation between how lay clients speak about their problems and the way in which the legal system frames these same issues. This translation exercise is a core part of a human lawyer’s “client skills.” Social intelligence – including the ability to empathize and communicate with a with range of backgrounds – remains particularly elusive for AI systems.Footnote 48 In the BigLaw context, the users of technical systems are typically themselves lawyers, who are able to provide such translation for their ultimate clients, and the costs of having human lawyers provide this are typically small relative to the value of the service in question. For PeopleLaw, the cost of having a human lawyer remain in the loop may be prohibitive. This suggests that at least part of the unmet legal needs may be beyond the current technical possibility frontier.
2.3.1 Use Cases in PeopleLaw
For the reasons described above, deployment of technology in the PeopleLaw context remains relatively modest. One key use case is automated document assembly – that is, the production of customized legal documentation using an automated system. In particular, it is the only technology of the ten considered in the Legal Services Board’s 2018 survey for which law firm respondents serving individuals were more likely to report adoption than those serving large businesses (25 percent v. 11 percent).Footnote 49 Transaction management tools are also increasingly widely deployed to assist in residential real estate and personal finance,Footnote 50 which have large throughputs of transactions for which individuals need legal services.
These tools are in many cases deployed in conjunction with transactional platforms that facilitate the connection of users to relevant human lawyers. They are typically fronted by a portal offering users simple Q&A on basic legal issues relating to their concerns, accompanied with document templates – perhaps automatically generated – and referrals to human lawyers as necessary. Each platform retains a network of lawyers whose work is ranked by users and to whom referrals are made.
Chatbots may seem a promising solution to the problem of engaging with lay users, but they need to be supported by systems capable of dealing with a sufficiently wide range of user inputs. Expert system approaches are constrained by the need to hard-code the relevant knowledge frameworks, creating limitations where user queries go outside this. Machine learning approaches trained on legal materials must not only be able to dispense and classify legal advice – beyond the capabilities of current systemsFootnote 51 – but also to be able to translate this into how laypersons understand legal issues. There is evidently a serious gap between ordinary parlance used by laypersons and the specialized terminology of legal discourse. This gap tests the frontier of applying natural language processing (NLP) to use laypersons’ statements or queries as data for prediction.Footnote 52 Making progress with these technological challenges will permit chatbots and virtual assistants to give wider-ranging advice to consumers.Footnote 53 This current technological bottleneck may explain the relatively low rate of use of chatbots and virtual assistants (see Table 2.1). These technological constraints limit the extent to which legal tech systems can substitute completely for human lawyers.
2.3.2 Augmented Lawyering and Business Models in PeopleLaw
Legal tech is engendering new business models in the PeopleLaw context, just as for BigLaw. The PeopleLaw ecosystem, however, is somewhat different in both the stakeholders and the emergent business models (see Figure 2.2). In the PeopleLaw ecosystem, clients are individual consumers and small businesses, rather than large businesses. Thus, all the lawyers in the ecosystem are on the supply side, offering advice directly to lay consumers. In contrast, for the BigLaw ecosystem, the demand side is driven by in-house lawyers.
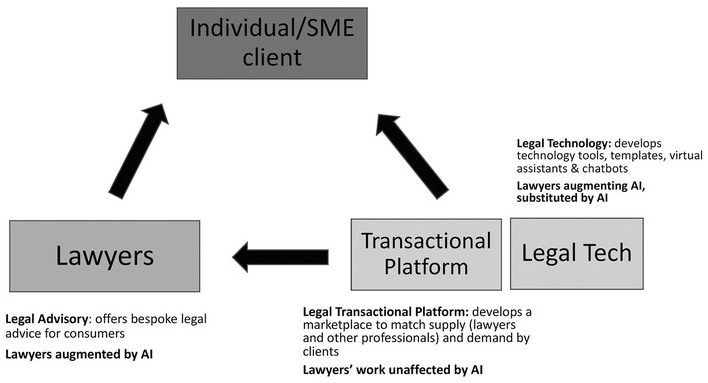
Figure 2.2 The PeopleLaw ecosystem
In both settings we characterize a new legal tech business model, developing technological systems for legal services. The design and implementation of such systems engenders new tasks for persons with legal expertise, working together as part of multi-disciplinary teams. Lawyers working in such teams are producers of digital legal services.
The limits of existing technology to provide legal services directly to consumers mean that human lawyers remain in the loop in most PeopleLaw contexts. This means that, at present, there is less opportunity than in BigLaw for what we term the Legal Operations business model – leveraging technology to substitute for humans in the delivery of legal services.Footnote 54 Instead, much of the current deployment of legal tech in PeopleLaw has the lesser ambition of simply lowering search costs to match human lawyers to clients, just as e-Bay provides a marketplace for buyers and sellers. Thus, a legal tech firm offering such a marketplace acts as a two-sided platformFootnote 55 to lower costs of matching lawyers to consumer needs. Such platforms do not substitute for human lawyers; rather, their value lies in augmentation of human lawyers’ productivity. We characterize the provision of such platforms as a “Transactional Platform” business model.
Some providers seek to capture further economies of scale by defining consumer needs more broadly than simply “legal” needs, offering a conveniently integrated package of legal and other services. For example, Farewill offers “death” services, combining will writing and funeral services;Footnote 56 other providers may offer a service in “moving home,” combining conveyancing and mortgage brokerage, or in “injury” combining advice on personal injury law with insurance services.
The constraints we have identified mean that the full promise of legal tech to unlock value for PeopleLaw consumers has not yet been met. As of the early 2020s, legal tech is not yet capable of substituting effectively for human lawyers except in very simple tasks, such as generating standardized documents for wills or small business incorporation. The value created by legal tech in the PeopleLaw setting appears so far to be limited to augmenting human lawyers and lowering the search cost for end-users. While augmentation brings the overall costs of legal services down by increasing productivity, there is still a need for human lawyer input in many cases.Footnote 57 Paradoxically, the scope of potential gains from augmentation are likely smaller in PeopleLaw than BigLaw, because of the more routinized nature of the legal work. This helps explain the more limited deployment of legal tech in the PeopleLaw setting. In the future, if and when technology can substitute for human lawyers more comprehensively, there remains significant further scope for PeopleLaw providers to meet remaining latent demand for legal and associated services (see Figure 2.2).
2.4 Implications for Convergence: Leveling the Playing Field?
We are now in a position to return to our central question: Will the adoption of legal tech level the playing field through convergence in the PeopleLaw and BigLaw sectors’ relative capacity to meet legal needs? To do so, we employ a causal framework based on the following elements (see Figure 2.3). First, we summarize the emergent business models that are theoretically possible given the nature of legal tech and other constraints. Second, we examine factors that encourage or discourage the adoption of these business models, including regulation and access to financial capital and relevant human capital, as mediated by organizational governance of law firms and other providers. Third, to the extent that it is possible, we draw implications for the market size and industry structure (the degree of concentration or fragmentation) of PeopleLaw and BigLaw markets, and their relative capacity to meet latent demand for legal services.

Figure 2.3 Causal framework for convergence-divergence
2.4.1 Convergence in Meeting Client Needs?
We begin with a high-level consideration of the possibility of convergence and divergence using an economic lens. This focuses on the black boxes in Figure 2.3 imputing causal links between legal tech and data on the one hand, and market size and industry structure on the other, while assuming that latent demand is more or less fixed.
Digital technology is a double-edged sword when it comes to leveling the playing field with respect to meeting client or consumer needs. To begin, the possibility for convergence relative to the past lies in technology’s ability to reduce costs of delivery, expanding the “legal production possibility frontier” given user budget constraints.Footnote 58 The reduction in cost per unit of legal service delivery derives from both supply-side economies of scale, with technology facilitating automation (substituting human lawyers) and better workflows, and from demand-side economies of scale, the so-called network effects.
Other factors, however, suggest we may still be a long way from absolute convergence, and the two sectors may continue to diverge in meeting latent legal needs.Footnote 59 Convergence would require the demand curve to remain fixed, which may not be the case. For example, in the BigLaw context, while technology assisted review (TAR) lowers the unit cost of document review, its availability may simultaneously increase the level of effective demand (that is, the number of documents sought to be reviewed), thus raising the capacity needed to meet the overall demand. Thus, while the per-unit cost is lowered by technology, the equilibrium price might increase due to an increase in the size of the pie. (This assumes a relatively high price elasticity of demand or an outward shift in the demand curve.) This type of effect requires users to have significant financial resources, more readily available in BigLaw than in PeopleLaw.Footnote 60 In PeopleLaw, notwithstanding consumers’ meager financial resources, inability (yet) to automate interfaces with end-users due to the translation challenge (from lay language to legal framing as discussed in Section 2.3) means that unmet legal needs are likely to remain significantly high.Footnote 61 In short, this is a scenario in which PeopleLaw will be left behind in the artificial intelligence revolution, while BigLaw leapfrogs in the scale and scope of AI adoption.
Thus, predictions of progressive convergence versus continued divergence entail assuming different conditions for each side of the market. On the supply side, the case for convergence is based on technology’s capacity to reduce the cost of service delivery, but this may operate asymmetrically between the sectors – implying continued divergence – because of the uncertainty around technological capacity to translate lay language into legal framing. On the demand side, convergence would come about if latent legal demand is more or less fixed. By contrast, the divergence perspective is grounded in a view that unmet legal needs are a movable feast, with latent demand turning into effective demand not only through a change in the price but also through outward shifts in the demand curve arising from societal and commercial forces.
Below, we start by comparing emergent business models and their complements in PeopleLaw and BigLaw, to unravel some of these differing conditions.
2.4.2 Technological Possibilities, Business Models, and Data: BigLaw and PeopleLaw Compared
Our first task is to compare the technological possibility offered by legal tech and data to develop new business models in the two segments of the legal services market. In both the BigLaw and PeopleLaw sectors, the current phase of legal tech is based not only on rule-based expert systems to generate documents based on templates, but also machine learning that enables the generation of prediction. We have characterized four distinct business models (see Sections 2.2 and 2.3), which exist in both sectors but with some variations in relative importance (see Table 2.2). In particular, traditional Legal Advisory delivered by human lawyers remains significant in both sectors. However, the cost of such advice likely remains unaffordable for many PeopleLaw consumers. Legal tech solutions are now available in both sectors as inputs to Legal Advisory, augmenting the productivity of human lawyers-as-consumers of the technology. To date, however, the impact of this appears to have been more pronounced for BigLaw than PeopleLaw. The differing trajectories of the two sectors are due in part because the gains from augmentation are greater where the legal problems are more complex (typically the case in BigLaw), and in part because the delivery of these gains to end-users requires them to be able to afford the cost of the human legal adviser who intermediates them (again, more likely the case in BigLaw).
Table 2.2 Business models in BigLaw and PeopleLaw compared
Business model | BigLaw | PeopleLaw |
---|---|---|
(1) Legal Advisory | Bespoke legal advice by lawyers for corporate clients; augmented by use of services/products from (2) and/or (3) as inputs. | Bespoke legal advice by lawyers, individual customers. Likely unaffordable for many consumers. |
(2) Legal Operations | Improve workflow of legal service delivery at law firms and corporations by automation. May use products from (3) as inputs. | [Less important, as neither consumers nor PeopleLaw providers have large organizations.] |
(3) Legal Technology | Develop software tools to automate processes and practices in BigLaw legal services. | Develop and provide tools to automate processes and practices in PeopleLaw legal services. |
(4) Transactional Platform | Lawyers-on-demand for corporate clients wanting services on a project-by-project basis. | Marketplace to lower search costs for consumers to find lawyers with relevant experience. |
What has not yet happened on a large scale is the delivery of legal tech solutions direct to end-users without human lawyer intermediation. We view this as primarily a function of technological constraints. Given this, the future application of legal tech to bypass lawyers by using chatbots and virtual assistants has high latent demand in PeopleLaw.
In making a distinction between legal advice (more bespoke) and legal services (subject to repeated and scalable delivery), the Legal Operations business model has wider application in BigLaw than in PeopleLaw. This is because large law firms and corporations in BigLaw would wish to exploit workflow efficiency and automation within their organizations, while solo practitioners and law firms in PeopleLaw have less need or opportunity due to their small scale. In other words, opportunities to seek efficiency and lower costs exist due to both supply-side and demand-side reasons, but BigLaw is in a position to benefit more from supply-side economies of scale than PeopleLaw.
With respect to demand-side economies,Footnote 62 network effects could be leveraged in both sectors by using the Transactional Platform business model. Not only do such marketplaces lower search costs, the possibilities of finding appropriate transactional partners rise exponentially with more users of the platform.
Last and not least, the central importance of training data in artificial intelligence is likely to give advantages to providers that can scale in both BigLaw and PeopleLaw.Footnote 63 First-mover advantage may accrue to data aggregators that have a head start in training their AI models using data. Both sectors face challenges in turning unstructured data into machine-readable structured data, while also developing NLP methods to analyze less structured data. However, there are granular differences in the dynamics. In PeopleLaw, data aggregation between users may be relatively straightforward in the marketplace, although this may recede with growing background constitutional data protection.Footnote 64 In BigLaw, between-user data aggregation requires careful negotiation that takes account of commercial sensitivity. For now, much of the data aggregation taking place in BigLaw is within-user – for example, for a specific corporate client, be it a bank or an insurance company.Footnote 65
Another possible route to scaling up, leading to convergence, is the emergence of providers that serve both BigLaw and PeopleLaw clients. If a machine learning algorithm can be used for contract analytics in BigLaw, why not deploy the same algorithm for tenancy agreements, employment contracts, and other documents in PeopleLaw? In reality, providers serving both market segments are not a common trend, reasons for which may include the vastly different price points to generate demand in the two market segments, and the importance of cultivating a client base as a market entry barrier.
2.4.3 Access to Finance including External Capital
We now shift our analysis to the boxes labeled “financial constraint” and “human capital constraint” in Figure 2.3 representing distinct constraints on the effective deployment of new business models that arise from organizational governance of law firms and other entities providing legal services in BigLaw and PeopleLaw. Our analysis suggests that the financial capital constraint is not an issue in BigLaw in the way that it might be in PeopleLaw, whereas the human capital constraint may be more of a problem in BigLaw than in PeopleLaw.
The inability of traditional law firm partnerships to raise external capital was considered a major challenge preventing law firms from adopting technology.Footnote 66 Our research suggests otherwise: The main challenge for law firms in the BigLaw sector is in human capital, and in recruiting and motivating non-lawyers working in multi-disciplinary teams to deploy digital technology for legal service delivery.Footnote 67 In PeopleLaw, by contrast, sole practitioners and small firms likely suffer from financial constraints, if they wish to access legal tech.
Given the absence of publicly available information on spending on digital technology by law firms and corporate legal departments, it is difficult to compare aggregate investments made in the PeopleLaw and BigLaw sectors. We focus instead on a subset of investment activity for which data are available: the amount of external funds that have been invested in legal tech start-ups. We here present insights from an analysis of legal tech start-ups for which investment data were available in the Crunchbase Pro database in January 2021.Footnote 68 In total, legal tech start-ups in the UK raised $853 million, compared to $5.98 billion by legal tech start-ups in the US.Footnote 69 This divergence between investment in UK and US lawtech start-ups tracks differences in the overall levels of venture capital investment more generally, for which the US historically greatly exceeds the UK.Footnote 70
Start-ups and their venture capital financiers tend to operate in geographically concentrated clusters, owing to the importance of regional networks and in particular the hands-on nature of the financing relationship.Footnote 71 We focus, in our analysis, on 129 legal tech start-ups with headquarters clustered in London (45), New York (37), and the San Francisco Bay Area (47).Footnote 72 By reading company descriptions in Crunchbase, LinkedIn, and company websites, we manually classified start-ups according to whether they primarily served the PeopleLaw (41) or BigLaw (61) sectors.Footnote 73 These start-ups target a wide range of legal work. In BigLaw, start-ups in all three locations were in contract analytics, knowledge management, practice management, or lawyers-on-demand marketplaces. In PeopleLaw, tech start-ups existed typically in will writing, residential conveyancing, and simplifying the process of setting up a new business for start-up founders (see Figure 2.4).Footnote 74

Figure 2.4 Investment raised by legal tech start-ups in three locations
In terms of money raised (including angel and venture capital financing) over the lifetime of all start-ups in our sample, BigLaw has raised significantly more overall than PeopleLaw. However, this is driven entirely by London; in the two US clusters, the levels of investment in legal tech firms are approximately equal across these sectors (see Figure 2.4). Total funds raised in San Francisco exceed those raised in each of the other two clusters.Footnote 75
However, fundraising by start-ups in BigLaw captures only a portion of the total investment in technology for this sector. Not only do BigLaw start-ups attract venture capital funding, they also receive complementary investment by law firm and corporate clients to co-create new technology and share data, and the latter also make their own proprietary investments in technology. For example, according to the annual financial statement submitted to Companies House, the English magic circle firm Allen & Overy LLP invested approximately $27 million in internally generated software in 2018/19. This investment by a single large firm is equivalent to 15 percent of the total amount raised ($175 million) by BigLaw legal tech start-ups in London, as shown in Figure 2.4. While in BigLaw, the start-up fundraising figures understate the total investment in technology, in PeopleLaw there is no corresponding investment by individual consumers.
To summarize, financial capital appears not to be a binding constraint for the BigLaw sector, given that law firms organized as partnerships are able to invest more in technology than the fundraising by start-ups. In PeopleLaw, because law firms serving the sector tend to be smaller and have fewer financial resources, outside capital is plausibly more important to enable more legal tech start-ups to emerge in this market segment.
2.4.4 Access to Multidisciplinary Human Capital
We argued that an effective use of multidisciplinary teams (MDTs) in which lawyers-as-producers-of-AI work alongside non-legal professionals was essential for the effective deployment of legal tech. Here, we argue also that the problem of accessing multidisciplinary human capital to enable MDTs is more pertinent in BigLaw than in PeopleLaw.
In BigLaw, clients are large law firms as lawyer-only partnerships and corporate legal departments. Within law firms, human lawyers are required for bespoke work, and these lawyers-as-consumers-of-AI do very different work from lawyers-as-producers-of-AI working in MDTs. Other non-legal professionals are also not given opportunities for promotion to top management, making it challenging to recruit and retain the best talent in data science, management, and other disciplines.Footnote 76 Thus, there is likely to be a bifurcation in legal service delivery, between law firm partnerships whose business model (Legal Advisory) continues to center on human capital, and corporations that are aligned better to implement MDTs, pursuing Legal Tech or Legal Operations business models.Footnote 77
In PeopleLaw, the transformative impact of legal tech is likely to come about through legal tech start-ups that employ lawyers-as-producers-of-AI. There are of course law firm partnerships in the PeopleLaw sector, but the absence of career paths for multi-disciplinary non-legal professionals does not hit small law firms and sole-practice lawyers as much as large law firms, which are more prevalent in BigLaw. PeopleLaw may also be delivered by professionals other than lawyers – for example, experts in tax, insurance, real estate, and human resources.
In short, BigLaw faces a greater human capital challenge than PeopleLaw. BigLaw law firms’ challenge lies in aligning its human capital investment as a complement to their newly adopted business models other than the Legal Advisory model. PeopleLaw lawyers can also take advantage of the Transactional Platform, which enhances individual lawyers’ reputational transparency for consumers, thus reducing the significance of reputation pooling at the firm level.
2.4.5 Regulation
From the foregoing discussion, the financial constraint appeared to be more binding, and the human capital constraint less binding, in PeopleLaw than BigLaw. This suggests that the UK’s relaxation of rules that prohibited ownership of law firms by non-lawyers – which would facilitate the raising of outside capital – should have had more of an impact in the PeopleLaw than the BigLaw sector.
There are now over 1,000 licensed ABSs in the UK, as against a total population of over 10,000 law firms. For England and Wales, the Solicitors Regulation Authority (SRA) approved 1,089 ABSs by December 2020. Of these, 73 percent are limited companies, and 22 percent are limited liability partnerships.Footnote 78 About half of these ABSs have transformed from law firm partnerships,Footnote 79 and a sizable number have consequently changed the way in which they raise finance, to invest more in technology and innovation.Footnote 80 Consistently with the foregoing account, the vast majority of these law-firm-to-ABS moves have been very small firms whose clients are individuals rather than businesses.Footnote 81
This seems to suggest the UK’s regulatory reforms had an impact on the PeopleLaw sector. In particular, we might expect to observe more capital being raised by the UK than the US legal tech start-ups in our sample. Figure 2.4, however, does not support this prediction. While supply-side considerations may explain the greater levels of investment in San Francisco, this evidence does tend to suggest that the impact of the UK’s deregulation has been less than transformative. Put another way, access to financial capital may be necessary but not sufficient to transform PeopleLaw. Other regulatory reforms, not just those that aim to unlock capital flows, may be necessary – hence, UK policy initiatives such as the SRA’s Legal Access ChallengeFootnote 82 and the LawTech UK’s sandbox hosted by TechNation.Footnote 83 These sandboxes are intended to not only give providers better access to granular regulatory expertise to test new service offerings, but also to enhance legitimacy and consumer confidence in the robustness of the underlying legal tech. This is consistent with the conclusion by BartonFootnote 84 that in the US, the speed of legal tech adoption, ironically led by providers serving the poor and corporate clients, is bounded by the technological barriers rather than the regulatory barriers alone.
2.4.6 Future of Market Size and Industry Structure
Pulling these various strands together, we want to know what the likely future for PeopleLaw and BigLaw is in terms of their relative market size and industry structure. We attempt to address this question in the context of no change in current regulation, and first identify the scale-up possibilities and advantage of each identified business model. Simply put, the Legal Advisory model does not scale. By contrast, the Legal Operations and Transactional Platform models are subject to supply-side and demand-side economies respectively. Legal technology that has a platform characteristic has the potential to scale and dominate,Footnote 85 while other technologies such as software tools may remain “point solutions” that do not scale without a platform.
In BigLaw, technology solutions that are specific to the legal industry are already wired into cross-sector technology solutions – for example DocuSign with its e-signature, Salesforce, and contract analytics tools that use the Microsoft Office platform. Moreover, data providers such as Thomson Reuters and LexisNexis are vying to become technology platform leaders via the acquisition of legal tech providers. One possibility is that legal tech for BigLaw will become more and more subject to the platform logic, leading to greater market concentration of technology providers, many of which hail from outside the legal industry. If that is the case, the UK Competition and Markets Authority’s recent recommendation for a unified register of legal tech providers may be necessary but not sufficient as effective public policy.Footnote 86 At a minimum, what is a legal tech provider, as opposed to simply a tech provider, needs to be defined.Footnote 87 Also, market concentration is more likely if governments do not implement policies to restrain anti-competitive behavior of big tech companies.
In PeopleLaw, market growth (and concentration) can accelerate if two things happen: first, the growth of Transactional Platforms, and second, technological solutions to the lay-to-legal-framing translation problem. While platforms may take off to provide a launching pad for scaling up, one side of the marketplace will remain human lawyers, rather than chatbots or virtual assistants, until this translation problem is addressed and resolved by data scientists and linguists. In the meantime, blurring the boundary between BigLaw and PeopleLaw market segments would not happen for some time to come in spite of the theoretical possibility of sharing the same platform and the same algorithms across segments. The reasons stated earlier include the difficulty of aggregating across-user data, the vastly different price points to solicit demand, and client base cultivation as a market entry barrier. Moreover, convergence is more likely to come about via “trickling up” rather than “trickling down,” consistent with Christensen’s idea of disruptive innovation.Footnote 88 That is, it is more likely for legal service innovation that starts by addressing the low end of the market whose needs are currently not met by incumbents to move up the value chain, and less likely that expensive high-end solutions will be adapted for low-end markets by stripping down functionality to achieve lower costs.
2.5 Conclusion
This chapter addressed a question of central importance to public policy, namely, whether or not the adoption of legal technology will level the playing field between two hemispheres of the legal services sector – PeopleLaw and BigLaw. In the late 2010s, PeopleLaw constituted only a fifth to a quarter of the total revenues in legal services markets in the US and UK. We argue in this chapter that, in order to level the playing field and to make PeopleLaw thrive relative to BigLaw, the use of legal tech is necessary but not sufficient.
Legal tech, together with the aggregation of data, has enormous potential to transform the way legal services and legal advice are delivered in both hemispheres. Repetitive and scalable tasks can be automated, substituting technology for human lawyers and lowering unit costs. Tasks requiring extensive customization or social intelligence remain in the exclusive competence of human lawyers, but their capacity is augmented by the deployment of technology for repetitive tasks. Through these channels, technology offers the potential to lower the costs of legal service delivery and thus reach consumers and clients whose needs had gone unmet. This would most obviously play out through the adoption of new business models (such as legal operations, transactional platforms, and legal tech) that focus on capturing economies of scale. In turn, these economies of scale would drive market concentration, with emerging winners likely being those who can best combine network externalities associated with both usage and data aggregation. This is a dynamic increasingly familiar from tech firms in other sectors. The change would be most obvious in PeopleLaw, which traditionally operates at a much smaller scale, but the underlying dynamic would be similar, and the process would lead to convergence in meeting client needs, business model adoption, and market structure.
However, the reality is far more complex, because – at least for now – various constraints create obstacles to market participants’ ability to leverage technology through the adoption of new business models. In BigLaw, key barriers lie in the human capital constraints associated with mixing Legal Advisory with other business models. Legal Advisory is, by definition, focused on work that is human-centric, and so organizational and management structures that appeal to the humans with the relevant capital will be crucial for competitive advantage. However, these institutions correspondingly constrain the deployment of Legal Operations and Legal Tech business models, creating a constraint on concentration. Alongside this, users’ hesitancy about data aggregation, at least for now, constrains the extent to which legal tech platforms are able to achieve concentration.
In PeopleLaw, the process of concentration appears to be well under way, with transactional platforms and integrated service delivery offerings capturing economies of scale and scope. However, there remains a seemingly significant obstacle to meeting latent demand, through the fact that the “client-facing” aspect of service delivery still eludes complete automation. Because human lawyers have high costs, this translates into high prices that raise the bar on the extent to which demand remains latent. There is some evidence that financial constraints are also an obstacle, but this is challenged by the greater levels of legal tech investment in the US (where law firms are not permitted to raise outside equity) than in the UK (where they have been able to do so for a decade under the Alternative Business Structure model). This suggests that the stakes in the US regulatory debate may be lower than participants imagine. At the same time, these constraints are unlikely to be eased by a policy focus on price transparency and comparison shopping emphasized by the UK’s Competition and Markets Authority.Footnote 89 Legal services, however productized, are after all credence goods, and consumers and clients who purchase them must overcome information asymmetry and/or behavioral biases.
While the work of lawyers long appeared to be beyond the reach of automation, the “legal tech” revolution now seems to be in full swing. Of particular importance is the emergence of a new generation of legal tech applications that utilizes artificial intelligence (AI) and machine learning (ML). Their underlying technologies have started to profoundly change the work of various professionals, including bankersFootnote 1 and physicians.Footnote 2 And so it comes as no surprise that the rise of AI is also predicted to allow for the automation of some of the core tasks performed by lawyers.Footnote 3 Others go further, arguing that AI will ultimately allow computers to replace attorneysFootnote 4 and judgesFootnote 5 in many scenarios, with profound changes for the functioning of the legal system.Footnote 6
In reality, however, the extent to which AI can contribute to automation of the legal industry will depend on several factors, including regulation,Footnote 7 culture,Footnote 8 and technology.Footnote 9 In this chapter, we focus on the last factor, the current state and likely future trajectory of technological progress. Among the different technological requirements, our focus is Natural Language Processing (NLP), a key component of many current and envisioned legal tech tools. Understanding the inherent constraints of current NLP models will be crucial in determining the extent to which legal tech applications will succeed in their quest to revolutionize the market for legal services. Although it cannot be ruled out that “robo-judges” and “robo-lawyers” will one day dominate the reality of legal engagement, our analysis suggests that recent developments in the relevant fields provide no basis for the prediction that such comprehensive legal automation is right around the corner.
The legal system trades in words, and NLP promises to automate an activity that lies at the heart of many tasks performed by lawyers: the extraction and processing of information from unstructured text. Lawyers routinely encounter unstructured text in their daily work routine, be it in the form of judicial opinions, statutes, legal briefs, written agreements, or witness testimony. Understanding and processing the information from this text is essential for them to be effective. For example, without reading prior case law, lawyers will generally be unable to determine whether a case at hand has a chance of succeeding in court. Consequently, many legal tech applications, and particularly those seeking to automate the tasks lying at the heart of what it means to “be a lawyer,” depend on NLP to process such information in a meaningful way.
We provide readers with an overview of the current state of NLP techniques, focusing on their promise and potential pitfalls in the context of legal tech applications. Like many other fields of AI, NLP has seen some drastic improvements in recent decades. Among other things, these improvements have contributed to some of the most talked-about success stories in legal tech, including the development of novel tools that promise to facilitate often mundane and repetitive tasks like document review. Against this background, one might conclude that advances in NLP are poised to similarly benefit the development of other legal tech tools. Yet, while there certainly is ample room for optimism, a realistic outlook must simultaneously recognize that NLP suffers inherent and important constraints, limiting its utility for legal tech applications in significant ways.
We explain why NLP-powered legal tech can be expected to outperform humans in specific categories of tasks that play to the strengths of current ML techniques. In particular, NLP-assisted applications perform well in prediction or classification tasks in which large amounts of pre-labeled data is available or can be generated to train an algorithm. The availability of suitable training data enables an algorithm to detect even subtle patterns in text or other data that predict the label a human would have attached to the document or other item.Footnote 10 At the same time, the chapter explores and highlights some of the central obstacles to deploying these tools in other contexts, most importantly in tasks that require the equivalent of legal reasoning. This includes, for instance, applications seeking to assess the likely outcome of legal disputes.
To be successful in this latter context, legal tech applications would need to automatically extract from relevant texts a structured representation of legal concepts and their interconnections (what we refer to as a “legal ontology”). However, despite recent progress in developing tools that appear – at least on a superficial level – capable of extracting meaning and knowledge from text, attempts to derive legal ontologies have so far been largely unsuccessful. On the contrary, recent studies suggest that current advancements in NLP, for the most part, do not meaningfully increase the performance of algorithms in tasks requiring legal reasoning. This, in turn, suggests that many legal tech applications may not benefit significantly from improvements made in general language processing and general language understanding. Instead, in order to make significant progress, a concentrated and domain-specific effort may be required that is specifically designed to promote the capabilities of language models to engage in forms of legal reasoning.
3.1 NLP as Part of the Broader Legal Tech Landscape
3.1.1 The Rise of ML-Powered Legal Tech
Changes to the work of lawyers brought about by technology are not a new phenomenon. Over the last decades, the introduction of technologies such as email and word processing and the availability of computer-based legal databases have profoundly changed lawyers’ daily routines. With the advent of ever-more-powerful computers, the Internet, and recent developments in AI and ML, this development appears to have accelerated. The emerging field of “legal tech” promises to equip lawyers with tools capable of automatically generating substantive content descriptions of contract clauses,Footnote 11 analyzing legal briefs,Footnote 12 and performing data-driven venue analysis.Footnote 13 In addition, because of its potential to scale, technology is often seen as a way to make legal services available to those who cannot afford a (human) lawyer.Footnote 14
Many commentators writing about legal tech adopt a broad definition that goes well beyond applications seeking to automate the activities at the core of a lawyer’s work. As an example, consider a recent article by David Engstrom and Jonah Gelbach. Their definition of legal tech encompasses applications ranging from outcome prediction to online marketplaces for lawyers.Footnote 15 Notably, as the example of online marketplaces for lawyers illustrates, not all of these applications relate to tasks that lawyers routinely perform as part of their work. And even within the set of applications that substitute for work usually performed by lawyers, the technical sophistication required to automate the task varies substantially.Footnote 16
Despite this broad definition of legal tech, many observers are particularly interested in legal tech applications made possible by applying ML and similar techniques to the legal field.Footnote 17 While legal tech includes applications powered by technology that has been around for decades (in one form or another), many tasks performed by lawyers and other knowledge workers long seemed beyond the reach of automation. Recent advances in ML, however, have enabled significant technological progress in many areas that were commonly considered the sole domain of humans, including the driving of cars,Footnote 18 translation,Footnote 19 and the writing of human-readable textFootnote 20 and music.Footnote 21 Not surprisingly, these developments have also spurred discussion about novel legal tech applications that automate the work of lawyers to a hitherto unknown extent.Footnote 22
Many techniques in ML are designed to generate predictions from example data.Footnote 23 Consequently, its potential is greatest for tasks that can comfortably be viewed as prediction exercises. To illustrate, consider reviewing a large number of documents with the aim to identify privileged information before the documents are shared with the opposing party in litigation.Footnote 24 This task can comfortably be characterized as a prediction exercise: The goal is to determine whether a document should receive one of two labels, “privileged” or “non-privileged.” ML techniques can facilitate this process. In particular, through supervised classification, an ML algorithm can be trained to “learn” the difference between privileged and non-privileged documents using a small, human-labeled sample. Once trained, the algorithm can then create predictions for the entire, unlabeled corpus of documents. In this way, the ML algorithm “predicts” the label that is most likely to be assigned to a document by a human coder, based on the features of the labeled documents.Footnote 25
Another task that is often discussed as an important use case for ML-assisted legal tech is outcome prediction. Potential litigants deciding whether to file a case – and attorneys deciding whether to represent them – will usually attempt to form an expectation about the chances of succeeding at trial. At least in principle, this is a straightforward prediction task: If the right data were available, ML algorithms should be able to determine whether a plaintiff is likely to win by comparing the features of the present case with the features of past cases.Footnote 26
Of course, the examples above do not constitute an exhaustive list of potential applications. Instead, they simply serve as an illustration for the broad range of tasks that may benefit from developments in NLP.
3.1.2 The Role of NLP in Legal Tech
NLP, as used here, refers to a set of methods that allow computers to process and extract information from human language. NLP constitutes an important building block for various programming tasks, including programs enabling computers to act on commands issued in spoken languageFootnote 27 and translate text between different languages.Footnote 28 Like much other AI research, NLP has been a topic of inquiry (in one form or another) since at least the 1950s. In earlier decades, and in line with the then-governing paradigm guiding much research in AI, NLP operated on the basis of a “top down” approach: Computers extracted information from texts following complex sets of hand-coded rules drawn up by linguists and other experts.Footnote 29 Since the 1990s, NLP has undergone a profound transition that mirrors similar developments in other areas of AI. Today, most NLP follows a “bottom up” approach, relying heavily on ML and similar statistical techniques. These approaches allow computers to “learn” how to process language on the basis of large amounts of training data.Footnote 30
On a very general level, NLP techniques hold the potential to automate an activity that plays a central role in many tasks performed by lawyers: the extraction and processing of information from unstructured text, either spoken or written. By unstructured text, we mean any text that is not organized in a manner that readily maps onto predefined categories known to be relevant for the task at hand. Most information encountered by lawyers in their daily work takes the form of unstructured text. Examples include the text of judicial opinions, statutes, legal briefs or written agreements, or written witness testimony. In contrast, the information provided by a client in response to an intake questionnaire that allows for only a predefined set of responses to each question does not constitute unstructured text, and NLP is usually not needed to process this kind of information.
To illustrate the central role of information extraction from unstructured text, consider again the example of outcome prediction. An attorney trying to predict whether a case presented to her by a client will succeed in court will usually require at least two types of information: first, information about the facts of the case and, second, information about how courts treat cases like the one at hand. The latter category includes, in particular, information about the applicable legal rules.Footnote 31 Both types of information will primarily be available in the form of unstructured text. Information about the facts of the case often comes in the form of written or spoken statements by the client, potentially combined with additional documents such as contracts; it can also involve depositions of witnesses and background research. Information about the law will typically take the form of statutes, regulations, and other cases, plus (depending on the jurisdiction) secondary sources of law such as legal treatises. Only after obtaining information about both the facts and the law (and potentially other factors that might influence the decision) will the attorney be able to determine the chances to succeed in trial.
This example is not an outlier. Instead, the processing of unstructured text plays a central role in many tasks performed by lawyers. A lawyer conducting document review reads documents to determine whether they fall into certain predefined categories (relevant to the case at hand, privileged, etc.) A lawyer drafting an agreement not only researches the law to establish the legal framework against which the contract is set, but also consults templates or examples of similar agreements she or her colleagues have drafted in the past.
Because of the central role that the extraction and processing of information from unstructured text plays in the work of lawyers, NLP is a potential key component of many actual or hypothesized legal tech applications.Footnote 32
NLP will likely be particularly important in applications that seek to increase access to legal services to those who cannot afford a lawyer. In all the examples described above, before even starting their work on a case, lawyers need to decode unstructured language (often in the form of spoken text) to understand their clients’ goals. Insofar as legal tech applications seek to interact directly with clients on the basis of unstructured language descriptions provided by the latter, NLP will also be needed to automate the task of translating these narratives into legally relevant units of information.Footnote 33 In addition, NLP can play an important role in communicating the results of the work performed by a legal tech application to a client in language that she can understand.
3.2. The NLP Prediction Pipeline
Typically, the process of creating predictions from text consists of two steps. In the first step, a language model converts text into vectors. In a second step, these vectors are used as an input into a ML classifier in order to create relevant predictions.Footnote 34 We discuss each step in turn.
3.2.1 Step 1: Converting Text into Vectors Using Language Models
Available language models differ in terms of their complexity. At the lower end of the spectrum are simple language models such as the “Bag-of-Words” (BoW) model.Footnote 35 These models do not involve a formal training process. Instead, each word is assigned its own, unique vector representation. Take, for instance, the following three sentences:
Sentence 1: We protect your data
Sentence 2: We safeguard your data
Sentence 3: We expose your data
To represent these sentences, a BoW model creates three vectors, one for each sentence. The vectors have as many dimensions as there are unique words (here 6). The elements of the vectors indicate whether a word is present or not. In this case, the column vectors for the three sentences are given in Table 3.1.
Table 3.1 Sample vectors in a BoW model
Word | Sentence 1 | Sentence 2 | Sentence 3 |
---|---|---|---|
we | 1 | 1 | 1 |
protect | 1 | 0 | 0 |
safeguard | 0 | 1 | 0 |
expose | 0 | 0 | 1 |
your | 1 | 1 | 1 |
data | 1 | 1 | 1 |
The above representation illustrates two important aspects of the BoW model: First, there is a lot of overlap between the vector representations of the sentences. Each vector has a 1 in the first, fifth, and sixth position. Hence, this simple language model accurately suggests that all three sentences are somewhat related, in the sense that they all talk about how another party handles the addressee’s data. At the same time, however, the BoW representation has a significant shortcoming. In particular, the overlap between Sentence 1 and Sentence 2 is the same as the overlap between Sentence 1 and Sentence 3. However, any human reader would attest that the words protect and safeguard are semantically close, whereas protect and expose have almost polar opposite meanings. In this sense, a simple BoW model may be able to capture some semantic similarity between texts. However, since it simply represents word occurrence, it does not encode information about the semantic meaning of text. If we were to use the output of this BoW in a downstream task, it could quickly lead to incorrect results, particularly for nuanced tasks in which every single word carries significance.
To address this problem, current language models seek to identify numerical representations that are more faithful to the semantic meaning of a word or sentence. Under these models, Sentence 1 and Sentence 2 would be represented by vectors with similar elements, whereas Sentence 1 and Sentence 3 are represented in a way that is faithful to the difference in their semantic meaning. The details on how these models are able to achieve their goal differ, but what all modern language models have in common is a reliance on distributional semantics. The intuition behind distributional semantics is that linguistic items with similar semantic meanings are also distributed similarly. Indeed, John Firth famously said that “a word is characterized by the company it keeps,”Footnote 36 a linguistic notion similarly supported by Wittgenstein, who argued that the meaning of a word can only be understood by learning how said word is used in context.Footnote 37 We can easily see that this assumption is often reasonable. Assume, for instance, that we encounter a new word spelled quari. Without context, it would be difficult to tell what this word means. But now assume we encounter the word being used in practice. Perhaps we encounter sentences such as “I took my quari to the mechanic yesterday,” or “I was driving fast with my quari on the highway.” At this point, we could start forming expectations that a quari is a form of vehicle, because it is used in a way that is similar to how we use words such as car or automobile. At some point, we may even start using quari as a synonym for car.
The objective on which modern language models are trained imitates this type of learning from distributional properties. For instance, Google’s BERT and OpenAI’s GPT-3 – two recent large language models that have been hailed as breakthrough innovations for NLP – are trained simply by repeatedly guessing which word is most likely to occur next in a sequence of words.Footnote 38 The idea behind this training objective is straightforward: If a model is optimized to predict the next word in a sentence, it inherently learns linguistic properties that correspond to semantic meaning. For instance, a model learning that it is likely that the next word in the sentence “I was driving fast with my … ” is car or motorcycle but not cat implicitly learns that a motorcycle is closer in meaning to car than it is to cat. And it turns out that next word prediction at scale is able to implicitly encode much more than just semantic meaning. For instance, by repeatedly solving next word prediction tasks, language models can “learn” grammatical rulesFootnote 39 and encode world and limited legal knowledge.Footnote 40
3.2.2 Step 2: Downstream Prediction Tasks
Once a language model has been trained, it allows for the conversion of text into vectors that encode the semantic meaning of a word, sentence, or document. These vectors can then be used in a ML model tasked with generating predictions that can vary with the objective of the legal tech application.
Like all ML models, those used in the context of NLP applications require at least two different types of data: training data and input data.Footnote 41 Training data refers to the information that is used to “calibrate” the statistical models forming the core of the ML tools. In other words, the training data allows the algorithm to “learn” about the relationship between some input and the desired prediction. For many language-based legal tech applications, training data consists of texts alongside labels that reflect certain information contained in the text. During training, the algorithm will learn which textual cues are strongly associated with the individual labels.Footnote 42 For example, a legal tech application assisting in electronic discovery may seek to automatically distinguish between documents that do and do not contain privileged information. In order to achieve that goal, the creator of the legal tech application would begin by hand-labeling a training corpus of documents for whether they contain privileged information or not. She would then use a language model to transform the content of the training documents into numerical vectors and would feed these vectors into a ML algorithm that learns the relationship between the different elements of the vectors and the human annotation (privileged/non-privileged).
Input data is the data that is fed into the machine to generate the predictions or other results that the user of the legal tech application is interested in. Notably, and in contrast to the training data, input data can be largely unlabeled. In the context of document review, the input data usually consists of the unlabeled documents that were not included in the training dataset. After the ML algorithm has been trained, it can generate predictions for these documents at scale.
3.2.3 Two Use Cases
To illustrate both the utility and limitation of NLP in legal tech, we focus on two applications – document review and case outcome prediction. Both tasks lie at opposite ends of the spectrum of legal cognitions and thus bring some of the problems of NLP in sharp relief. At the same time, we are conscious of the fact that the full spectrum of legal tech implementations encompasses a much larger set of applications and that implications we draw here might apply differently in different use cases.
In the case of document review, the standard NLP pipeline described above performs well.Footnote 43 This is in part due to the fact that document review closely resembles information extraction, a standard linguistic prediction task that these models are particularly designed for. For instance, a typical exercise in NLP research is to predict the sentiment of social media posts on the basis of the posts’ texts and a labeled training dataset.Footnote 44 Similarly, as described above, document review can be understood as an exercise in predicting labels associated with a document (e.g., privileged/non-privileged) on the basis of the text of the document.
Other tasks, however, are much more difficult to perform, irrespective of how sophisticated the language model is. One such task is the prediction of the outcome of a legal dispute. Legal outcome prediction using expert-generated systems has a long tradition.Footnote 45 However, here, we focus on the automatic prediction of legal outcomes without any human intervention.Footnote 46
To illustrate the difficulties associated with legal outcome prediction, consider how the standard NLP pipeline might be implemented for this task.Footnote 47 At first, the process may appear to be straightforward: A language model could transform textual descriptions of fact patterns into vectors, with each vector in the training dataset accompanied by a label indicating whether the plaintiff or the defendant won the case.Footnote 48 The ML algorithm would then generate predictions for the input dataset depending on the textual features of the document. The approach may sound simple in theory. And yet, to date, NLP-based attempts at predicting the outcome of legal cases using the standardized process have failed to produce reliable results.Footnote 49 But what is it that differentiates legal outcome prediction tasks from other exercises, such as classifying documents into relevant and non-relevant?
At its core, the difference lies in the quantity of linguistic markers that influence the prediction task. In legal outcome prediction, the set of informative linguistic markers is potentially infinite, whereas in document review, there typically is a small set of linguistic cues the algorithm is required to pick up on. To illustrate, imagine that there was a universal speed limit of fifty miles per hour. If we trained a model to predict the outcome of a dispute over a citation, it would quickly pick up on the fact that the most important linguistic cue for the prediction of the outcome is the description of the car’s speed. If that description contains phrases such as “sixty mph,” it would predict that a legal challenge to the citation is unlikely to succeed, whereas phrases such as “thirty mph” would lead the algorithm to predict a higher success rate. Perhaps the model would alter its prediction based on whether the description indicates that a radar was used or whether the speed was measured through pacing. But overall, the possible combinations of types of fact patterns that are relevant to the prediction are finite and small, and it is likely that (almost) every type of fact pattern relevant to the prediction could be sufficiently represented in a large enough corpus. Importantly, note that the classifier could achieve a high performance without ever being told what the specific rule is. Indeed, given a large enough training sample, the algorithm would automatically identify that “fifty mph” appears to be the discontinuity at which the probability of success changes significantly.
However, most legal prediction tasks, and certainly most of the law applicable to disputes, are not as simple. Consider, for instance, a negligence tort dispute. In contrast to the clear fifty mph rule in the narrow context of driving on public roads, negligence is a vague standard that may trigger liability in an unquantifiable number of contexts. The indefinite number of variations in fact patterns comes with a similarly indefinite number of potential linguistic cues that are relevant to the prediction of the outcome. When designing a legal technology app, it is impossible to collect a training dataset that contains all potential fact patterns that may or may not lead to liability under a negligence regime. In this scenario, a language model does not have the opportunity to detect and assess the relevance of all these linguistic cues that might be relevant to the success rate of the plaintiff. In order to make accurate predictions, the algorithm can no longer rely on comparing the linguistic and distributional patterns of one document to other documents it has encountered during training. Rather, in order to be successful, the algorithm has to perform the equivalent of legal reasoning. In other words, to fully automate the process without significant human intervention, an algorithm would have to be able to extract from the texts a representation of legal concepts and their interconnections (a “legal ontology”). With such a legal ontology, the algorithm would then be able to draw accurate inferences from precedent, even if it had never encountered the particular fact pattern in the past. For instance, if an algorithm was able to infer that fact patterns describing a small burden and a large probability of a significant loss increase the chances for the plaintiff to prevail in a negligence suit, it could use this knowledge to inform its predictions without reliance on the particular words used in the description of facts.
Against this background, one might ask whether the impressive trajectory of modern language models in recent years also translates into an ability to reconstruct such legal ontologies from text.Footnote 50 Unfortunately, the results to date are not promising. In fact, existing algorithms are notoriously bad at extracting abstract rules from text, and legal ontologies are no exception. Even if a researcher feeds the text of the rules directly into the model, current language models are not able to accurately process that information. For instance, Holzenberger and colleaguesFootnote 51 task a language model with predicting how much tax is owed by an individual, using as input a description of facts. They show that, even though the relevant ontology can be defined manually by an expert and used to compute the exact tax rate, language models perform poorly at this task. But perhaps more importantly, the authors point out that model performance does not increase even after supplementing the description of facts in the training data with the text of the relevant statutes. This suggests that language models have difficulties deriving a legal ontology from text without human assistance.
To corroborate these intuitions, we turned to GPT-3, a state-of-the-art language model that has achieved extraordinary results on a number of language tasks.Footnote 52 Among others, it has demonstrated its ability to translate a description of a computer program from ordinary language into a working software application,Footnote 53 and it has written blog posts that fooled thousands of people into thinking they were authored by a human.Footnote 54 The legal literature has begun to study the utility of GPT-3 for tasks such as contract analysis in the consumer context, with at times positive results.Footnote 55
To assess the capabilities of GPT-3 for a simple task involving legal reasoning, we first submitted the following query:Footnote 56
Are liquidated damages clauses enforceable?
The (correct) response:
Liquidated damages clauses are generally enforceable unless the sum stipulated is exorbitant or unconscionable.
However, we then submitted the following query:
X and Y contract over the sale of a Toyota Corolla. Their contract specifies that X has to pay Y one million dollars in damages if X does not deliver the car on time. X does not deliver the car on time. Q: Is Y entitled to damages? If so, in what amount?
GPT-3’s response:
Yes, Y is entitled to one million dollars in damages. Damages are meant to compensate the injured party for the loss suffered due to the breach. Y has suffered a loss of one million dollars because X has not delivered the car on time.
GPT-3 has been trained on millions of documents including almost half a trillion words. And while it was able to correctly extract and restate the legal rule based on the documents it encountered during training, it was not able to convert this rule into a legal ontology that would enable it to correctly infer the invalidity of the contractual penalty.
Next, we explicitly specified that the stipulated damages were “exorbitant.” We also fed the relevant rule that GPT-3 itself recovered back into the model, resulting in the following query:
X and Y contract over the sale of a Toyota Corolla. Their contract specifies that X has to pay Y the exorbitant amount of one million dollars in damages if X does not deliver the car on time. X does not deliver the car on time. The law requires that liquidated damages clauses are enforced unless the sum stipulated is exorbitant or unconscionable. Q: Is Y entitled to damages? If so, in what amount?
GPT-3’s response:
Yes, Y is entitled to the liquidated damages. The amount in this case is not exorbitant since the price was set by the parties themselves.
Again, the model ignores the legal rules and produces an incorrect prediction. To be sure, we emphasize that our queries are not meant to scientifically explore the limitations of GPT-3. Instead, our application is meant to exemplify a more general point. As we pointed out above, the training objective of language models causes them to encode information that is included in the distributional properties of written language, such as word and sentence order. Language models are thus able to excel at tasks that require accessing distributional information. For instance, in order to write a text that is indistinguishable from a text written by a five-year-old human, most of the relevant information is contained in the grammatical structure and frequency distribution of words.Footnote 57 However, language understanding in general, and legal reasoning in particular, requires processing of information that is not a mere reflection of linguistic patterns. Instead, lawyering requires a “dizzying array of analytic moves,”Footnote 58 including an ability to apply rules to facts, inferring regularities from existing case law, distinguishing new fact patterns from precedent, and using logical reasoning and creativity to craft new legal arguments. Modern language models do not even begin to achieve performance that is close to that of human professionals. Perhaps even more importantly, the performance of these language models does not appear to significantly increase with the sophistication or complexity of the language model.Footnote 59 This suggests that the current trajectory of developments in NLP, which relies heavily on distributional properties, may run orthogonal to the kinds of models that would be needed to create accurate predictions of legal disputes.
Together, the current developments point toward a more limited role for NLP in applications involving legal reasoning: It appears that the task of building, dissecting, and understanding the law in order to assess a novel set of facts will continue to rely primarily on domain experts.Footnote 60 Creators of legal tech applications will have to rely on these experts to define a knowledge system that determines the relevant factors under the law, as well as how these factors influence the likely outcome. This does not mean that NLP will be irrelevant for such applications. It could still be used in more narrowly defined information extraction tasks with the aim of determining whether the factors that matter for the outcome of a dispute are present in a case at hand.
3.3. Other Key Challenges
The challenge of automating the creation of legal ontologies makes it difficult to predict whether, and when, technology will be able to completely replace the prominent and essential role humans – and, in particular, human lawyers – play in creating a functioning legal regime. Of course, it is famously difficult to predict the capabilities of future AI systems in many different contexts. However, one thing we do know is that recent advancements in NLP, although in many ways impressive, did not move us significantly closer in automating many of the core tasks that lawyers perform on a daily basis. It may well be the case that the full automation of most legal services is not only years, but decades away. But this is not all. At least four further challenges will impose hurdles on the development of legal tech tools and shape the field’s future trajectory.
3.4.1 Document Structure and Segmentation
Before working with a text corpus, it needs to be broken up into coherent, informative units of analysis. This process is also referred to as “document segmentation” and is an important step in the NLP pipeline that can have significant consequences both for training a language model as well as for other downstream prediction tasks. In principle, the investigator is free to break text up at varying levels of granularity, such as the individual word, sentence, paragraph, section, or entire writing (e.g., a contract or statute).Footnote 61 However, this process can be complicated by the fact that many legal documents do not follow a strict template, as is the case for most judicial opinions. But even if the structure is relatively consistent across documents, choosing the right level of granularity requires balancing of competing factors. On one hand, if the segmentation is too coarse, algorithmic training is inefficient and more training data is required. In addition, modern language models simply do not work for very long text sequences, because their computational complexity increases with the length of the document at an exponential rate.Footnote 62 On the other hand, many more complex classification tasks require a sufficiently large context to give a complete and accurate answer. Therefore, if the segmentation is too granular, performance may suffer. Accurate segmenting can thus pose a significant challenge to NLP research.
When lawyers are working with legal texts, these challenges are exacerbated, because the most informative unit of analysis is often inconsistent and can vary widely from one document to the next. To illustrate, assume that an algorithm is trained to automatically determine whether a court in a contracts dispute has personal jurisdiction over the defendant.Footnote 63 As input, we may provide a description of facts and the text of the agreement between the parties. However, choice-of-forum provisions can come in very different forms. Sometimes, they are contained in a single sentence. At other times, they may span multiple paragraphs. The agreement may even contain multiple dispute settlement provisions for different types of disputes. Thus, whether the appropriate unit of analysis is a sentence, paragraph, or section may vary from one document to the next, and this variation can significantly decrease classification performance and/or efficiency. To be sure, researchers have proposed deep-learning classifiers that are able to retain information as they move from one segment of the document to the next, thus ameliorating some of the concerns of inappropriate text segmentation.Footnote 64 However, because the computational complexity of these approaches increases exponentially in the length of the text sequence, they cannot feasibly be used to examine long legal documents.Footnote 65 Although researchers are beginning to examine ways around this limitation,Footnote 66 we are still far from finding workable solutions for long texts that obviate the need of segmentation.
In addition to document segmentation, identifying and processing the appropriate document structure complicates efforts to automate legal analysis. Many legal documents are highly contextual in that they rely on internal and external references in order to give meaning to their words or phrases. For example, M&A agreements typically include elaborate “Definitions” sections that define phrases such as “bankruptcy event” or “material adverse event,” which are then used throughout the contract. Similarly, regulations and statutes often rely on definitions that are not contained in the text of the document itself and thus can only be interpreted accurately by turning to the referenced text.Footnote 67 There may further be a hierarchical structure to legal documents, by which a definition only applies to parts of the document that are lower in the hierarchy. For instance, a definition contained in a statutory text may define words or phrases at the chapter level, but could then be modified by exceptions at the section level.
Current language models are ill-equipped to recognize and appropriately process such structural idiosyncrasies with relevant precision. Although recent research is beginning to develop a promising methodology to try to accommodate legal document structure, the existing approaches are still highly domain-specific, and it is unclear whether they can be generalized.Footnote 68
3.4.2 Availability of Training Data
A second key challenge is data availability. As discussed above, most ML models need access to large amounts of training data. In the context of legal tech applications based on NLP technology, this means access to large numbers of documents that are representative of the input data used in the application.
Whether data access is a potential problem depends on the nature of the legal tech application. One reason for this is that the creators and users of legal tech applications can create training data for some applications, while the same is not true for others. As a general matter, the creation of training data is usually possible when the “labels” that are to be predicted can be generated by the users of the legal tech application themselves. As an example, consider document review. For many document review tasks, training data can be created by tasking human coders (in many cases, lawyers and paralegals) with manually labeling a subset of documents according to a coding scheme that is later replicated by the ML algorithm.Footnote 69 By contrast, in outcome prediction tasks, labels (the outcomes of disputes) are the product of a complex interaction between a multitude of actors including judges and litigants. A party interested in predicting the outcome of a dispute cannot on its own generate additional cases to serve as training data. Rather, they are limited to the cases generated in the context of past disputes.
Creators of applications that depend on existing data often face the problem that suitable training data from past cases is not widely available. There are various reasons for this. One potential problem is that legal texts are oftentimes hidden behind paywalls (either erected by private database providers, for example Westlaw,Footnote 70 or public players such as PACERFootnote 71). Even more important, however, is the problem that many relevant documents are never collected by any central entity that would be in a position to make them available to potential creators of legal tech applications. For example, while it would be desirable to create outcome prediction tools that can use early information about a dispute as input data, such information is almost never collected in a systematic way.
Selective availability of information is problematic not only because it raises questions about the availability of sufficiently sized training datasets to achieve high levels of predictive accuracy. It also gives rise to concerns about biased results. In the context of outcome prediction, because there are (virtually) no data repositories that systematically collect texts related to legal disputes prior to litigation, whether information about a dispute will become available at some point often depends on whether the dispute ends up in court or not. This is a problem because cases that do not result in litigation arguably differ in important ways from those that are litigated.Footnote 72 For instance, if there is clear, established precedent or otherwise little ambiguity in the rules that apply to a dispute, litigation is unlikely to occur. But this means that the available textual data is not an accurate representation of all potential disputes. Instead, textual data regarding disputes that end up in litigation will be heavily overrepresented. In the extreme, an outcome prediction tool that never encounters an “easy” case during the training process and is instead only trained on “hard” cases will have a tendency to abstain from making strong predictions even if a trained lawyer would be able to predict the outcome with certainty.
3.4.3 No Benchmark Data
The final limitation we discuss is the absence of domain-specific benchmark data that would allow creators of legal tech applications to compare the efficacy of newly developed methodologies. NLP is a research field that develops at a rapid pace. Indeed, the number of yearly submissions to ACL Anthology, the largest source of NLP papers, increased from about 1,000 in the 2000s to more than 4,000 new submissions by 2019.Footnote 73 In order to identify breakthrough contributions, the NLP community relies heavily on the concept of benchmarking. Benchmarks such as SuperGLUEFootnote 74 or the Stanford Question Answering Dataset (SQuAD)Footnote 75 contain hundreds of thousands of observations corresponding to various non-legal tasks, such as question answering, causal reasoning, and reading comprehension. The benchmarks have easily accessible leaderboardsFootnote 76 and achieving state of the art (or SOTA) results is the main way in which important innovations are identified and adopted.
However, although these benchmarks aim to capture language understanding of NLP models, they are designed for an ordinary/natural use of language. As mentioned above, legal language differs from ordinary language in several important aspects. Whether a higher score at SuperGLUE or SQuAD corresponds to better performance at legal interpretation remains unknown – and, indeed, there are many reasons to doubt that the correlation is close to perfect. This suggests that we currently lack the means to gauge how well language models work in the legal domain. To remedy this problem would require the availability of benchmark datasets specifically designed to resemble legal language understanding and reasoning tasks. However, such datasets can be exceedingly resource-intensive to design and maintain.Footnote 77 Indeed, it appears likely that significant progress can be made only if there is a shared commitment toward advancing and improving the use of natural language processing in law. Naturally, there appear to be various barriers to such a collaborative process. For instance, it stands to reason that law firms would be in a particularly good position to make available representative samples of legal documents (such as contracts). At the same time, law firms generally lack incentives to make these documents available to a general audience for benchmarking. To be sure, there are some efforts to try to overcome these barriers. For instance, the Atticus Project has made publicly available a dataset of annotated contracts with the stated goal of establishing a reliable reference corpus that could be used to improve the use of AI in contract analysis.Footnote 78 However, with a corpus of 510 agreements, the dataset is still far from providing a representative sample of commercial contracting that is comparable to those available to benchmark natural language models. It remains to be seen whether efforts like the Atticus Project can establish themselves as reliable benchmarks in the legal domain.
3.5. Conclusion and Outlook
NLP, a set of computational techniques that automate the extraction and processing of information from unstructured text, is considered a key ingredient in many legal tech applications. However, the performance of these techniques might not always live up to some commentators’ high expectations. In particular, as this chapter argues, current NLP techniques are ill-equipped to distill legal concepts from texts, which imposes severe limitations to their use in legal tech applications that need to perform the equivalent of legal reasoning. Further, creators of NLP-assisted legal tech need to grapple with several additional challenges, including a dearth of training data and the absence of benchmark datasets.
This result suggests that, in the short to medium term, NLP can be employed most fruitfully in tasks that do not significantly rely on legal reasoning. In contrast, the automation of tasks that rely heavily on legal reasoning will remain out of reach for the foreseeable future, save for any significant changes in how language models operate. This is because, for automated legal reasoning to succeed, it is not sufficient for an algorithm to process language in a literal sense. Instead, legal reasoning requires building, understanding, and processing the legal ontology that the language reflects. In other words, what is needed is a particularly complex form of Natural Language Understanding.Footnote 79
How, then, might we identify tasks that are more reliant on legal reasoning and less amenable to automation? Perhaps the most obvious examples are those applications that require not just a high performance in predicting outcomes, but also need to explain how they arrived at the prediction.Footnote 80 But concerns about explainability are not the only reason why the ability of algorithms to perform the equivalent of legal reasoning matters. This ability is also a crucial determinant of an algorithm’s predictive performance in those tasks for which large enough amounts of representative training data are not available.
If such training data is available, prediction tasks reduce to an inference problem: The algorithm must simply identify the predictors of a legal outcome while filtering out the noise. This can be the case, for instance, for outcome prediction tasks in run-of-the-mill cases that are based on relatively homogenous fact patterns.
However, if representative training data does not exist, accurate predictions are more heavily reliant on the availability of a representation of the general principles underlying previous case law. Without these, algorithms will likely fail to generate accurate predictions for previously unseen fact patterns. Because of current NLP techniques’ limitations in recovering legal ontologies, NLP-based automation will likely face the greatest technological hurdles in dynamic areas of law where rules frequently change (e.g., those characterized by significant regulatory activity, such as environmental law), in areas that are multi-faceted and complex, and in areas in which the data is scarce (e.g., in litigation areas where settlement rates are particularly high). Similarly, settings that produce many new, previously unforeseen legal constellations will prove to be particularly challenging (e.g., appellate court decisions).