Introduction
Fifteen years after mapping the human genome, an individual’s health is clearly shaped not only by genomics, but also by a complex web of factors, from molecular to societal, that varies over time. Genetics are fixed at conception, though gene expression is dynamic and “genetics” of health and disease are complex and develop across the lifespan. While our understanding of the genetics of disease evolves, another medical “revolution” has been occurring. During the past several years, generation of massive quantities of data has become commonplace and excitement surrounding “big data” as well as machine learning (ML) for medicine is palpable. However, the explosion of these two fields has outpaced the evolution of theoretical and conceptual frameworks that should underlie research in these arenas. Studies that are not grounded in solid frameworks may lack reproducibility and biological plausibility. We propose that the marriage of a “life course” theoretical framework with complexity science associated with big data applications has paradigm-shifting potential for understanding health development across the lifespan. It also offers potentially significant translational science impact.
The Barker Hypothesis is often cited as an early use of the life course research lens in chronic disease epidemiology [Reference Barker1]. The landmark Dutch Famine natural experiment used a large historic cohort database, where the exogenous insult was lack of nutrition in a defined population with available health outcomes data [Reference Roseboom, Meulen, Osmond, Barker, Ravelli and Bleker2]. The study demonstrated a strong association with poor maternal nutrition and later offspring risk for obesity and diabetes, but the outcome depended on the gestational timing of the exposure this general phenomenon of early life exposures (ELEs) affecting later disease manifestation has since evolved to the more encompassing Life Course Health Development (LCHD) framework [Reference Halfon, Forrest, Lerner and Faustman3]. LCHD provides a theoretical framework for embracing complexity in health research by integrating a developmental perspective into the concept of health and describing how health development is applicable to both individuals and populations. The model is built on the recognition that although early determinants, exposures, and influences may not become phenotypically evident for years or decades, such events can lead to significant adverse later life health consequences. Health risks and disease conditions evolve over time, coexist with positive health states, and can be mitigated or exacerbated by social, physical, and biologic contexts.
Life course research is often conflated with lifespan research; however, the ideas are distinct and related to our recommendations for moving beyond theory to implementation. The focus of this article is to (1) delineate differences between lifespan and life course research, (2) articulate the importance of complex systems science as a methodological framework in the life course research toolbox to guide our research questions, (3) raise key questions that can be asked within the clinical and translational science domain utilizing this framework, and (4) provide recommendations for life course research implementation, charting the way forward.
Differentiating Lifespan from Life Course
Confusion often surrounds the terms lifespan and life course. Lifespan is a measure of longevity reflecting underlying biologic aging of an individual that occurs for everyone. Life course encompasses lifespan and is influenced by the interaction of contextual factors over time that affect health and development and vary among individuals (see Table 1 for a list of definitions). Furthermore, exposures, whether physiologic or sociologic, can have differential impact on health outcomes based on the following:
a. Dose: The amount of endogenous or exogenous exposure (such as stress or air pollution).
b. Duration: Short- or long-term accumulation of physical and psychosocial exposures throughout the life course on health and development from gamete to grave.
c. Timing: The timing of exposure in relation to human development, recognizing that some periods of development allow for more plasticity and adaptation than other periods.
Table 1. Core definitions

Matching Theory to Methods: Complex Systems Science as a Methodological Framework in the Life Course Research Toolbox to Guide Our Research Questions
A theoretical tenet of life course research is the interdependence between and dynamic nature of factors that shape health. Recent advances in high-performance computing create possibilities for the combination of data across a vast number of sources to make sense of the complex, interdependent factors that shape health. As a result of these technological advances, as with genomic medicine two decades ago, the potential for ML-based methods to solve complex disease questions has emerged [Reference Kermany4,Reference Bennett5]. While ML can identify important patterns that may not be extractable by the human mind, theoretical and methodological grounding is necessary to avoid misattributing causation to spurious associations. A hybrid approach is needed, one that connects theory to methodological frameworks to allow for scientifically rigorous and biologically plausible inquiries.
A Life Course Approach Influences New Hypotheses
Below we highlight three case examples illustrating the complex nature of human health and disease and the importance of couching questions related to the development of immunity, type 2 diabetes (T2DM), and Alzheimer’s disease (AD) within the LCHD theoretical framework using methodological tools from complexity science. This demonstrates how using a life course lens within complexity science frameworks can lead to generation of new scientific hypotheses and associated interventions that consider the high dimensionality, nonlinearity, and heterogeneity of health and disease.
Case 1: The Development of Immunity and Impact of Ubiquitous Persistent Infection
Early infancy and childhood represent windows where fundamental processes prime the immune system and development of the microbiome occurs. For example, early life acquisition of human cytomegalovirus (CMV), a ubiquitous childhood infection, can lead to enhanced responsiveness to vaccines including those for influenza [Reference Furman6,Reference Castrucci7]. Conversely, later in the life course, ongoing CMV infection may promote immunosenescence, paradoxically conferring decreased vaccine responsiveness and increased susceptibility to other infections [Reference Brodin8]. Thus, the timing of exposure to and acquisition of CMV has potentially a profound impact on immune system priming and function, and functional immunologic outcomes can clearly evolve over the life course.
However, sole examination of timing of CMV infection does not fully describe its broad influence over the immune network, which is reactive and adaptive to both heritable and nonheritable influences [Reference Brodin8]. A study by Brodin et al. used monozygotic identical twins discordant for CMV serostatus (i.e., one twin infected, the other uninfected) to demonstrate substantial differences in CD8+ cell effector function, gamma-delta T-cell populations, and differences in both cytokine levels and overall responsiveness to IL-10 and IL-6. These parameters vary with age; generally heritable factors have limited influence and nonheritable factors increase influence as cumulative environmental exposures shape the microbiome over the life course. In fact, responses to influenza vaccine were found to be entirely influenced by nonheritable factors, indicating that interactions between the microbiome and infections are complex and driven by a combination of inherited and nutritional or pathogenic factors, which vary based on timing of exposure during vulnerable windows of development. Shifts in the microbiome, in turn, affect immune system health at large, which continually adapts to environmental circumstances [Reference Belkaid and Hand9]. Social patterns of immunity and infection may also give rise to differential trajectories of exposure and illness within populations. The example of CMV infection is instructive, given that there are striking disparities in congenital infection and early life seroprevalence depending upon race, ethnicity, and socioeconomic status [Reference Fowler10,Reference Manicklal, Emery, Lazzarotto, Boppana and Gupta11].
Because the development and influence of the immune system are dependent on the timing of exposure to infections and long-term influence on immune system functioning, a life course lens leads us to different research questions that could shed light on prevention strategies and improve health across the lifespan. Research questions should be framed historically, as occurring within a specific time context, and holistically, focusing on genetic changes over time and multiple step processes to disease initiation rather than reducing the question to cause and effect of a single variable.
Case 2. Emerging Adolescent and Adult Type 2 Diabetes Mellitus (T2DM)
Individuals may be genetically predisposed to the development of T2DM, with rates of heritability ranging from 20% to 80%. To date, over 150 genetic variations that increase risk of T2DM diagnosis have been identified [Reference Prasad and Groop12]. While genetic predisposition is an important contributor, it cannot fully explain the overall risk for the development of T2DM. T2DM is a heterogeneous phenotype [Reference Tuomi, Santoro, Caprio, Cai, Weng and Groop13] that involves mechanisms at multiple levels (individual, family, community, policy) that interact dynamically, consistent with the LCHD framework. A complex set of factors throughout the life course contributes to risk of disease in adulthood, some having additive or multiplicative interactive effects and others, such as interactions between transcription/translation regulation and metabolic networks, affecting risk through feedback loops [Reference DenBraver, Lakerveld, Rutters, Schoonmade, Brug and Beulens14].
Dose, duration, and timing of exposures may also have important roles between environment and T2DM risk. The level and length of time spent in unhealthy environments (i.e., dose and duration) may be important factors to consider when linking possible exposures to T2DM. The developmental period (i.e., timing) of exposure is also an important consideration. During the prenatal period, higher maternal prepregnancy BMI, maternal hyperglycemia, and exposures to endocrine disrupting compounds are associated with later T2DM risk [Reference Hivert, Vassy and Meigs15,Reference Giulivo, Lopez de Alda, Capri and Barceló16]. In newborns, low infant birth weight and associated rapid catch-up growth in the first 6 months of life increase the risk for T2DM in adolescence and young adulthood [Reference Eriksson, Kajantie, Lampl and Osmond17]. Furthermore, the infant gut microbiota, influenced by pre-, peri-, and early postnatal factors, may also affect risk associated with adult-onset disorders such as T2DM [Reference Tamburini, Shen, Wu and Clemente18]. Childhood and young adult exposures such as poverty, adverse childhood experiences, depression, and engagement in risk behaviors (e.g., smoking, alcohol use) all further influence the risk for the development of T2DM in middle adulthood, and account for some racial and ethnic disparities observed [Reference Bancks, Kershaw, Carson, Gordon-Larsen, Schreiner and Carnethon19].
Selecting tools from complexity science allows for holistic approaches to understanding the associations and can be used to assess the relative contributions of factors that could be altered to benefit health. For example, a recent study by Frisard et al. used the Women’s Health Initiative Clinical Trials Cohort and Observational Study Cohort, both longitudinal cohorts, to investigate the association between antidepressant use over time and T2DM risk [Reference Frisard20]. The authors used marginal structural models (MSMs) to allow for predictor variables such as antidepressant use and BMI to vary across an average of 7.6 years of follow-up. A handful of other studies investigating T2DM risk used similar approaches to allow for variation in measures, such as socioeconomic status, over time [Reference Farmer21,Reference Nandi, Glymour, Kawachi and VanderWeele22]. Other approaches have incorporated multiple types of genetic and dietary information. Network analyses have been used to integrate genetic, genomic, and functional data to explore the mechanisms predisposing individuals to T2DM [Reference Fernández-Tajes23,Reference Liu24]. Random forests have been used to predict metabolic syndrome status based on dietary and genetic data [Reference Szabo de Edelenyi25].
The current literature lacks in-depth studies that combine a spectrum of data ranging from genes to environment and utilize multilevel models and methods that embrace the complexity of T2DM. Novel research questions will likely require new methods that allow for the incorporation of time and complex interactions. For example, investigating the relationship between neighborhood, diet and exercise, differential DNA methylation over time, and resulting changes in fasting glucose and insulin metabolism in individuals transitioning from normal to pre-T2DM and T2DM requires a methodological framework that can model the complexity of the relationships without ignoring changes over time. By looking through a life course lens, drawing from existing tools and the creation of new tools, identified risk factors at the individual, parental, and environmental level along with the timing and interaction of the exposures could be used to direct targeted T2DM interventions.
Case 3. Cognitive Impairment/AD in Older Ages
Cognitive decline is an example of a complex phenotype that may have multiple interrelated risk factors across the lifespan, with genetics and environment playing an important role in shaping risk. Early life risk factors, including learning disabilities, educational attainment, impaired body growth and development, and lower childhood socioeconomic status all impact adult brain structure and function, and when present, increase vulnerability to the development of cognitive impairment/ AD in later life [Reference Whalley, Dick and McNeill26–Reference McGurn, Deary and Starr30]. While AD is a major cause of dementia in Western societies, it develops asymptomatically decades before diagnosis. Genes play a role, but by themselves rarely predict when or if AD will emerge [Reference Seifan, Schelke, Obeng-Aduasare and Isaacson31], suggesting the need to consider the interactive role of environment.
Some gene–environment interactions may be expressed in epigenetic markers; several epigenetic candidates demonstrate an association with dementia later in life [Reference Sanchez-Mut and Gräff32]. This is referred to as the Latent Early Life Associated Regulation model which suggests epigenetic markers acquired from gestation to older age could modify gene expression that may induce or accelerate AD [Reference Lahiri, Zawia, Greig, Sambamurti and Maloney33]. These nonheritable factors include infections, stress, nutrition, adiposity, and exposure to toxins (such as pesticides) which may have differing effects on risk of AD development depending on the degree and timing of the exposure, as well as the interaction of these factors [Reference Miller and O’Callaghan34].
While exposures such as these in early childhood can alter brain structure, predisposing the brain to increased risk for learning and memory dysfunction throughout life, exposure at other times in life can also affect brain health and risk for neurodegeneration. Chronic medical conditions, such as hypertension and T2DM in adolescence or adulthood, are associated with later cognitive impairment. This may be the result of defects in insulin signaling and consequent activation of neuroinflammatory pathways. However, the manifestation of cognitive impairment could be affected by control of the underlying condition, with cumulative effects of dysregulation over time exacerbating the onset and timing of cognitive impairment [Reference Zilliox, Chadrasekaran, Kwan and Russell35].
Moreover, it is not just early in life that these various exposures affect whether and when cognitive impairment will emerge, but also during middle age when there are many physiological changes. One such physiologic change includes an alternation in hormone levels. As women are 50% more likely to develop dementia sometime in their lives compared with men, multiple studies have turned to examining estrogen levels and total reproductive years to understand these associations [Reference Gilsanz, Lee, Corrada, Kawas, Quesenberry and Whitmer36]. However, specific mediators of neural injury have not yet been elucidated [Reference Seifan, Schelke, Obeng-Aduasare and Isaacson31]. Women with pregnancy-related complications, such as preeclampsia, are also at increased risk of developing later life cognitive decline [Reference Fields37]. Using a complexity science lens would inform new hypotheses to look not only at estrogen levels, fertility, and pregnancy outcomes over one’s lifespan, but examine the interactions of exposures such as infections, medications, chronic illnesses (such as T2DM), early childhood brain development, and social deprivation. By examing interactions, timing of these interactions, dose of exposure, and duration over time, different types of interventions would emerge.
Another example of how this lifecourse framework could change the timing and type of interventions is the relatively new discovery that the gut microbiome mediates regulation of brain homeostasis, potentially resulting in the alteration of microglial activation and brain function in middle age. Middle-aged mice who received prebiotic diets had reversal of stress-induced immune priming and reduction in aging-related monocyte infiltration, modulating the peripheral immune response and altering neuroinflammation in middle age [Reference Boehme38]. This could redirect our thinking – it is not only the intervention type, such as providing prebiotics, but the intervention timing, during middle age, that needs to be considered.
Taken together, the complex web of family history, genetic and epigenetic influences, environment and social context, chronic medical conditions, and microbiome influence require a life course approach to truly help us understand how to prevent or delay the onset and improve treatment of cognitive impairment and AD in older age [Reference Thein, Igbineweka and Thein39,Reference Lin, Zheng and Zhang40].
Charting the Life Course Recommendations
We are not the first to note a need for a complexity science approach to the epidemiology of health and disease [Reference Galea, Riddle and Kaplan41–Reference Roux43]; however, we think new technological innovations can now enable this approach to improve population health using a life course theoretical framework. We offer recommendations to build the necessary databases (process recommendations) that would allow for application of complexity science to generate and test new hypotheses (application recommendations). These five recommendations are summarized in Fig. 1. Process recommendations include the following: (1) creating systematic processes for longitudinal integration of datasets across the lifespan representing multiple levels of exposures from physiologic to sociologic; (2) utilizing data science core resources to prepare and package integrated datasets to make them accessible for researchers to generate and test new hypotheses; and (3) developing and validating ways to model high dimensional data. Application recommendations include the following: (4) promoting and applying existing methods that fit a complexity science methodological framework; and (5) developing analytical methods that can capture multiple dimensions of time (timing, dose, and duration). Below, we elucidate next steps to act upon these recommendations.

Fig. 1. Five recommended pathways for moving transnational life course research forward.
Integrate Datasets Across the Lifespan
Prioritization for the creation of datasets that allow for investigations of the interplay between factors at the molecular level to the organ level within a single individual, the study of individual interactions with the environment, and the elucidation of how these factors vary between people and over time is necessary to move life course research forward. Large amounts of data from individuals in different contexts and across time will allow us to explore gene–environment interactions or family member to family member influences in ways that were impossible before.
It is becoming increasingly common for studies to link multiple large datasets to allow for a more in-depth examination of disease risk. For example, the Utah Population Database (UPDB) is an immense genealogical dataset that has been record-linked to many statewide datasets (including the Utah Cancer Registry and statewide medical claims from 1996 to present), with annual updates. The full dataset contains nearly 10 M people, 4 M of whom have 3–18 generations of genealogy available, and infrastructure that links distinct records for a specific person allows the UPDB to create a depiction of the life history of an individual based on medical and administrative data [Reference Skolnick, Dintelman and Mineau44,Reference Hanson45]. UPDB links EHRs data to government datasets, such as birth and death certificates, census record, geospatial data, and social determinants of health. Creating a “tapestry” of information sources allows for a wealth of measurement on factors influencing health and disease, identification of sensitive windows of exposure, and targets for prevention [Reference Corn46]. EHRs linked to government datasets, such as birth and death certificates, census records, geospatial data, and social determinants of health, as well as other large data (e.g., school, child welfare, and employment records).
Utilize Data Science Core Resources
As the amount of patient-related data linked to external sources increases, data science cores focused on the curation, cleaning, analysis, and modeling of data need developed. Data science builds upon principles and methods from across multiple disciplines, including ethics, engineering, statistics, communication, mathematics, and epidemiology. Organizing teams including experts from across these disciplines would allow for the development of innovative methods and increase interpretability of large amounts of complex data. In addition to data curation and methods development, these teams should be focused on developing ways to communicate results from the scientific community to the lay population, as well as standards for data sharing and for improving algorithm portability (such as the model adopted by the www.ohdsi.org) to enable a wider range of analysis on these curated datasets.
Develop New Ways to Model High Dimensional Data
In order to fully leverage data integrated from a vast number of sources, new ways to model high dimensional data collected serially need to be developed. Combining data across multiple sources and utilizing ML (variable selection) techniques can help us develop more accurate predictive models by identifying large-scale properties of interacting variables and enabling targeted approaches by clustering patients with similar characteristics or phenotypes. This hypothesis-generating approach will allow us to expand on traditional hypothesis-driven approaches and find innovative ways to improve population health through pattern discovery and disease prediction. ML identifies patterns that explain the data available in both supervised (trained on an outcome) or unsupervised (trained to find a pattern) approaches. Pattern discovery algorithms are generally unsupervised, and can be used to refine our definition of phenotype [Reference Hanson47, Reference Hanson47–Reference Beaulieu-Jones and Greene50]. Algorithms are developed to seek out variables and combinations of variables to predict outcomes. ML differs from traditional regression methods because it can handle large amounts of combinations of data to examine interactions and nonlinear assumptions [Reference Mullainathan and Spiess51]. However, algorithms can also overfit models and lead to false conclusions. Consequently, data generated from ML approaches need validation from different populations. Moreover, outcomes are only as good as the data input and therefore can lead to biases reflected in the datasets themselves [Reference Obermeyer and Emanuel52].
Expand Traditional Epidemiological Methods to Include Systems and Network Modeling
Complex systems assume that the functional form is nonlinear, distributions are nonnormal, individuals are heterogeneous, and health reflects multilevel structures, dynamic temporality, and interaction between factors. Traditional epidemiological methods need to expand to include systems modeling, network analyses, and machine learning. Complex systems analyses model the dynamic interactions between factors at the same level (e.g., cells, individuals) and across levels (e.g., tissue, neighborhood). Agent-based models are a commonly used approach for complex systems analyses and have been used in chronic disease epidemiology [Reference Nianogo and Arah53], health disparities [Reference Langellier54], and health behaviors research [Reference Galea, Hall and Kaplan55] to explore dynamically complex processes and strengthen understanding of causal processes. Network analyses have been very useful for unraveling the complexity of genetic pathways and their interactions in chronic diseases. Phylogeography, a form of network analysis, is a method that can be used to study spatial viral disease distribution and would be useful for COVID-19-related studies [Reference Forster, Forster, Renfrew and Forster56]. ML methods take a more agnostic approach to epidemiological discovery by learning the models that best fit the data. ML methods, therefore, can play an important role in discovering unknown predictors of disease as well as refining phenotypic definitions of disease. Pattern identification, dimension reduction, and phenotypic definitions can be used to hone the search for causal mechanisms [Reference Hanson45,Reference Hanson47]. Methods embracing complexity science have the potential to identify factors having the largest impact on disease risk, the importance of duration and dose of exposure, periods across the lifespan that might be most amenable to intervention, the dynamic nature of risk, and moderating factors that should be considered.
Improve Reproducibility with Standardized Measures
As we move forward in identifying these measures, standard ways of operationalizing measures would improve reproducibility. Progress in recommendations 1–4 will be for naught without high-quality, semantically interoperable, and structured data. The penultimate solution would be a single American healthcare system, allowing for standardized records collection and storage. Until that goal can be achieved, investigators will have to continue to derive innovative solutions to overcoming the fragmented nature of data collection and storage.
Conclusions
Health and disease evolve over time through complex interactions. New technology allows for the development of new methods and measures to effectively conduct life course research, applying a life course framework drawing from tools – from complexity science methodology to both generating and testing novel hypotheses. These recommendations are not without limitations (Table 2); however, the benefits outweigh the limitations. The field will benefit from a consistent approach to generate verifiable big data and a systematic approach to identify key methods and measures to maximize utility of that data. New statistical analytic approaches to handle the complexity of time, dose and duration of exposures, and context need to be developed and tested. These recommendations begin to chart the way forward in life course research to further develop the field and move it from theory to application.
Table 2. Examples of existing methods that can be used to investigate health across the lifespan using multiple interactions, time as a dimension, system science-based approaches, and computational approaches
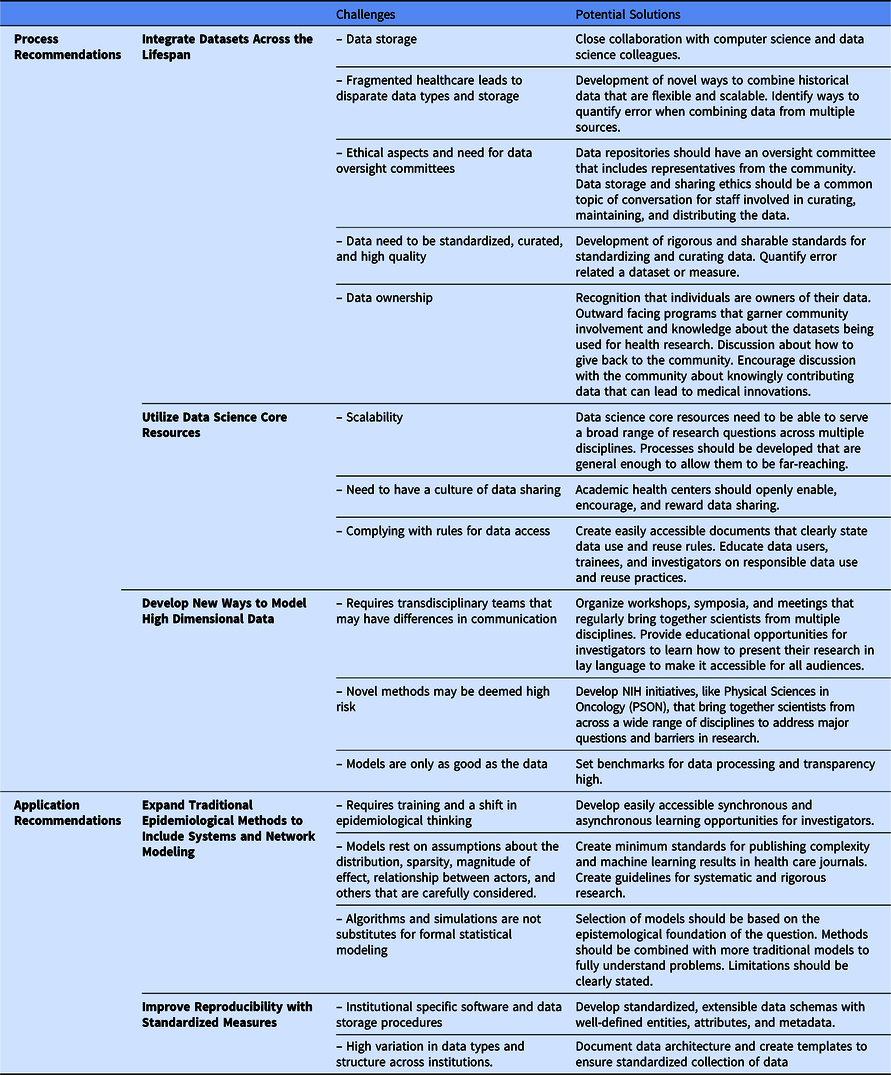
Acknowledgements
The authors would like to thank Karen Bandeen Roche, William Hay, and Rashmi Gopal-Srivastava for their valuable feedback. They would also like to thank Brenna Kelly for designing Fig. 1.
This publication was made possible by the following CTSA grants from the National Center for Advancing Translational Science (NCATS), National Institutes of Health (UL1TR001067, UL1TR002001, UL1TR001873, UL1TR001442, UL1TR001876, UL1TR002369, UL1TR001414, U01TR002004, UL1TR002494, UL1TR001086, UL1TR001866). Heidi A. Hanson is also partially supported by the National Institutes of Health K07 Award 1K07CA230150-01. Nicole G. Weiskopf is partially supported by the National Library of Medicine K01 Award LM012738. Brad H. Pollock is partially support by NIH UL1TR000002. Daniel Armstrong is partially supported by Agency for Community Living 90DDUC0031-03. Dan Cooper is partially supported by NIH R01HL110163 and P01HD048721. M Schleiss is partially supported by R01 HD079918. F. Kaskel is partially supported by NIHT32 NIDDK 5T32DK 007110-46. C Weng is also supported by the National Library of Medicine R01 LM009886 project. G Bandoli is also partially supported by the National Institutes of Health K01 Award K01 AA027811.
Disclosures
The authors declare no potential conflicts of interest.