Language comprehension is extremely fast. How do people manage this feat? Previous literature has shown that language processing is, to some extent, predictive in nature. Hence, listeners and readers use prior contextual information to predict upcoming semantic content, and in some cases, even specific word forms (DeLong et al., Reference DeLong, Urbach and Kutas2005; Ito et al., Reference Ito, Corley, Pickering, Martin and Nieuwland2016; Kuperberg & Jaeger, Reference Kuperberg and Jaeger2016; Luke & Christianson, Reference Luke and Christianson2016; but also see Frisson et al., Reference Frisson, Harvey and Staub2017; Huettig & Guerra, Reference Huettig and Guerra2019; Ito et al., Reference Ito, Martin and Nieuwland2017; Nieuwland et al., Reference Nieuwland, Politzer-Ahles, Heyselaar, Segaert, Darley, Kazanina, Bartolozzi, Kogan, Ito, Méziere, Barr, Rousselet, Ferguson, Busch-Moreno, Fu, Tuomainen, Kulakova, Husband and Huettig2018). Consider the sentence “He gave her a diamond necklace for her …”. Most people would probably complete this sentence with “birthday,” even though other completions such as “daughter,” “graduation,” or “neck” (even though they may be unpredictable) are plausible too. In contrast, many people will probably agree that other unpredictable nouns such as “mountain” or “liver” would not complete the sentence plausibly at all. In this study, we investigate how predictability and plausibility violations affect online language processing and longer-term memory structures. We begin by defining the linguistic concepts and reviewing the recent empirical evidence.
Definitions
Predictability indexes the likelihood of a sentence ending in a particular word and is usually measured by means of the cloze procedure (Taylor, Reference Taylor1953). Plausibility is sometimes measured by rating studies in which participants indicate how plausibly or meaningfully (given the real world) a word completes a sentence. Plausibility violations have found varying conceptualizations and labels in the literature (Brothers et al., Reference Brothers, Wlotko, Warnke and Kuperberg2020; DeLong et al., Reference DeLong, Quante and Kutas2014; Kuperberg et al., Reference Kuperberg, Brothers and Wlotko2020; Matsuki et al., Reference Matsuki, Chow, Hare, Elman, Scheepers and McRae2011; Rayner et al., Reference Rayner, Warren, Juhasz and Liversedge2004; Warren et al., Reference Warren, Milburn, Patson and Dickey2015; Warren & McConnell, Reference Warren and McConnell2007; Van de Meerendonk et al., Reference Van De Meerendonk, Kolk, Vissers and Chwilla2010). In this paper, we use the terms “deeply implausible” and “somewhat plausible” to refer to severe and mild violations of plausibility, respectively, consistent with the plausibility ratings we obtained for our items (see below; DeLong, et al., Reference DeLong, Quante and Kutas2014; Van De Meerendonk et al., Reference Van De Meerendonk, Kolk, Vissers and Chwilla2010). In order to facilitate comprehension of our review of previous studies, we use these exact same terms in reviewing the literature, even though we note that, across studies, implausible sentences varied in the properties that lead them to feel deeply or mildly implausible (being dependent on aspects such as context length, semantic similarity with preceding words, mismatch of semantic context as a whole vs word-based selectional restriction violations, among others; cf. Brouwer et al., Reference Brouwer, Delogu, Venhuizen and Crocker2021; Lau et al., Reference Lau, Namyst, Fogel and Delgado2016; Warren et al., Reference Warren, Milburn, Patson and Dickey2015).
Effects of predictability and plausibility violations on online language processing
Since predictability and plausibility are correlated (see Nieuwland et al., Reference Nieuwland, Barr, Bartolozzi, Busch-Moreno, Darley, Donaldson, Fu, Heyselaar, Huettig, Husband, Ito, Kazanina, Kogan, Kohút, Kulakova, Mézière, Politzer-Ahles, Rousselet and Von Grebmer Zu Wolfsthurn2020), it can be challenging to disentangle their separate contributions to language processing. Maybe because of this correlation, many prior studies have investigated effects of predictability and plausibility separately (e.g., Rayner et al., Reference Rayner, Warren, Juhasz and Liversedge2004; Staub et al., Reference Staub, Rayner, Pollatsek, Hyönä and Majewski2007; Warren & McConnell, Reference Warren and McConnell2007; among others). Only more recently, studies have started to examine the processing consequences of plausibility violations in conditions when predictability is low (as is done in the present study; see Brothers et al., Reference Brothers, Wlotko, Warnke and Kuperberg2020; DeLong et al., Reference DeLong, Quante and Kutas2014; Kuperberg et al., Reference Kuperberg, Brothers and Wlotko2020).
Predictability effects in sentence processing are well documented. For example, unpredictable words elicit larger N400 amplitudes, a negative-going ERP waveform that is supposed to index the semantic fit of a word with prior context (for review, see Kutas & Federmeier, Reference Kutas and Federmeier2011). Highly predictable words are more likely to be skipped in natural reading, and they normally elicit shorter and fewer fixations during initial, lexical stages of processing (for review, see Staub, Reference Staub2015) and during subsequent semantic comprehension and re-reading (Frisson et al., Reference Frisson, Harvey and Staub2017). Normally, these predictability effects are manifest as facilitation for predictable words, as opposed to slowing for unpredictable words. For example, Frisson and colleagues (Reference Frisson, Harvey and Staub2017) found that there was no processing cost for unpredictable nouns (e.g., “chair”) when they were presented in a context that strongly constrained toward a different noun (e.g., “The young nervous paratrooper jumped out of the …”), compared to when that same noun appeared in a neutral context (e.g., “The tired movie maker was sleeping in the …”).
Effects of plausibility violations on sentence processing are less clear. A series of eye-tracking studies has found that severe plausibility violations induce processing difficulties that emerge relatively early during reading (within approximately 300 ms of the eye’s first encounter with the noun or even earlier; see Veldre & Andrews, Reference Veldre and Andrews2016), often when using verb-based selectional restriction violations (e.g., “He used a pump to inflate the large carrots” or “The new principal talked to the cafeteria (manager)”; Rayner et al., Reference Rayner, Warren, Juhasz and Liversedge2004; Staub et al., Reference Staub, Rayner, Pollatsek, Hyönä and Majewski2007; Veldre & Andrews, Reference Veldre and Andrews2016; Veldre et al., Reference Veldre, Reichle, Wong and Andrews2020; Warren et al., Reference Warren, Milburn, Patson and Dickey2015; Warren & McConnell, Reference Warren and McConnell2007). The relatively early-emerging plausibility effect in these studies indicates that violations of plausibility result in immediate processing difficulties, with plausibility affecting natural reading almost as early as word frequency normally does. However, it is sometimes difficult to ascertain whether the effects in some of these studies were really driven by violations of plausibility or predictability (or indeed, a combination of the two), since predictability was not consistently controlled for.
In contrast to these findings from eye-tracking, ERP studies have shown that effects of plausibility occur somewhat later in the processing stream than those of predictability and with a different topographical distribution. For example, Nieuwland and colleagues (Reference Nieuwland, Barr, Bartolozzi, Busch-Moreno, Darley, Donaldson, Fu, Heyselaar, Huettig, Husband, Ito, Kazanina, Kogan, Kohút, Kulakova, Mézière, Politzer-Ahles, Rousselet and Von Grebmer Zu Wolfsthurn2020) demonstrated that, whereas an increase in noun predictability involved a more peaked reduction in the N400 in an earlier time window, an increase in plausibility lowered the N400 predominantly in a later and temporally more extended time window. An even later-emerging effect of plausibility violations was reported in other ERP studies, which factorially manipulated plausibility and predictability. In these studies, unpredictable nouns that violated plausibility severely (i.e., violations of animacy, for example, “They cautioned the drawer”) elicit a post-N400 positivity on posterior electrodes (Brothers et al., Reference Brothers, Wlotko, Warnke and Kuperberg2020; Brouwer et al., Reference Brouwer, Delogu, Venhuizen and Crocker2021; Brouwer et al., Reference Brouwer, Crocker, Venhuizen and Hoeks2017; DeLong et al., Reference DeLong, Quante and Kutas2014; Kuperberg et al., Reference Kuperberg, Brothers and Wlotko2020; Paczynski & Kuperberg, Reference Paczynski and Kuperberg2012; but see Vega-Mendoza et al., Reference Vega-Mendoza, Pickering and Nieuwland2021, for conflicting evidence). In contrast, unpredictable nouns that were somewhat plausible elicited a positivity with a different topographical distribution, that is frontally distributed (also see Thornhill & Van Petten, Reference Thornhill and Van Petten2012). Of note, the observed frontal and posterior positivities have been associated with diverging interpretations, in particular, regarding their consequences on memory representations, which we turn to now.
Effects of predictability and plausibility violations on learning and memory
Very little is currently known about the longer-term memory consequences of predictability and plausibility violations, because, to our knowledge, there are no studies that have investigated their joint effects systematically. The broader memory literature frequently uses the term “schema congruency” to refer to stimuli that are processed in a condition that is typical or congruent given previously established world or event knowledge (Brod et al., Reference Brod, Werkle-Bergner and Shing2013). That literature has shown that under some circumstances, schema-congruent information is remembered more distinctly (e.g., Höltje et al., Reference Höltje, Lubahn and Mecklinger2019; Packard et al., Reference Packard, Rodríguez-Fornells, Bunzeck, Nicolás, de Diego-Balaguer and Fuentemilla2017), whereas in other cases, schema-incongruent information improves memory (e.g., Corley et al., Reference Corley, MacGregor and Donaldson2007; Federmeier et al., Reference Federmeier, Wlotko, De Ochoa-Dewald and Kutas2007; Rommers & Federmeier, Reference Rommers and Federmeier2018). As our literature review will show, it is not at all clear whether schema congruency refers to a violation of predictability or plausibility (or indeed, both), which might explain the conflicting findings obtained so far.
A psycholinguistic study by Rommers and Federmeier (Reference Rommers and Federmeier2018) showed that unpredictable (but somewhat plausible)Footnote 1 words (e.g., “Jason tried to make space for others by moving his car”) elicit larger N400 repetition effects (i.e., more consistent reduction in the N400) when presented in a neutral sentence a second time, compared to the same words when they were initially processed in a predictable-plausible condition (e.g., “Alfonso started biking to work instead of using his car”). In addition, unpredictable-somewhat plausible nouns showed a later-emerging positivity, an ERP effect that is sometimes associated with explicit recollection (compared to an initial stage of implicit, gist-wise memory without conscious recollection; Rugg & Curran, Reference Rugg and Curran2007). Hence, these findings suggest that unpredictable information that is somewhat plausible boosts short-term memory maybe because of the greater semantic elaboration associated with having predictions disconfirmed (i.e., prediction error; see Haeuser & Kray, Reference Haeuser and Kray2021).
In conflict with the prediction-error perspective on memory and learning, other studies, especially from the memory domain, found that, in some cases, schema congruency drives memory. For example, Höltje and colleagues (Reference Höltje, Lubahn and Mecklinger2019) found that nouns that are initially encoded in a schema-congruent condition (e.g., preceded by a semantic category cue that semantically matches that noun: “a four-footed animal”-“dog”) showed higher hit rates in a next-day recognition task than nouns that were initially processed in an incongruent condition (“pepper”) or a low-typicality condition (“fox”). However, since low- and high-typicality endings were not matched in terms of frequency, it is difficult to critically evaluate the findings from that study. Packard and colleagues (Reference Packard, Rodríguez-Fornells, Bunzeck, Nicolás, de Diego-Balaguer and Fuentemilla2017) provided more compelling evidence on this matter by showing that recognition accuracy is improved for words (e.g., “blue,” “sofa”) that were initially read in a schema-congruent condition (e.g., preceded by the category cues “colors” or “furniture”) compared to these same words preceded by an incongruent category cue (e.g., “planets,” “continents”). Hence, this latter study suggests that high predictability (and/or plausibility, indeed) may increase recognition memory.
Maybe because of the inherent confound between predictability and plausibility, it is sometimes challenging to reconcile conflicting findings showing that schema congruency drives memory, on the one hand, and schema incongruency (i.e., prediction error) drives memory on the other hand. In addition to this, studies have shown that task- and item-related characteristics can push effects around. For example, distinctiveness of incongruent information triggers memory, such that the ratio between congruent and incongruent stimuli during encoding matters. In encoding situations when incongruent items are less frequent, they might be remembered more successfully because they stand out more, whereas in conditions where incongruent stimuli are frequent, there might be no memory advantage for such items (Reggev et al., Reference Reggev, Sharoni and Maril2018). Other studies found that speaker-related characteristics determine what language users retain from a sequence. Christianson and colleagues (Reference Christianson, Zhou, Palmer and Raizen2017), for example, demonstrated that recognition accuracy for words preceding a taboo word increased significantly when the taboo word was processed unexpectedly (i.e., uttered by a speaker who was otherwise unlikely to use taboo words).
A recent neurocognitive framework makes testable predictions specifically regarding the memory consequences of unpredictable words presented in a rich semantic context that are additionally deeply implausible (e.g., “The lifeguards received a report of sharks right near the beach. Their immediate concern was to prevent any incidents in the sea. Hence, they cautioned the drawer.”Footnote 2 ). According to Kuperberg and colleagues (Reference Kuperberg, Brothers and Wlotko2020), language users represent contextual information on three hierarchical levels, a situation model, an event level, and a semantic feature level. The highest level of the hierarchy, the situation model, is thought to hold a high-level representation of meaning that includes all events, actions, and characters that are contextually relevant in a given episode (also see Kuperberg, Reference Kuperberg2021). Unpredictable-deeply implausible words (e.g., “drawer”) should elicit restructuring and repair in this highest level of the hierarchy, the situation model, precisely because they cannot be plausibly integrated given the prior discourse context. Such restructuring should be absent when unpredictable-somewhat plausible information is processed, because that type of information violates contextual information only on lower levels (e.g., semantic features) and can still be integrated into the sentence context (is “explained away”; also see Van De Meerendonk et al., Reference Van De Meerendonk, Kolk, Vissers and Chwilla2010). Hence, this framework suggests that unpredictable words that additionally violate plausibility enhance memory.
The present study
Here, we examined how violations of predictability and plausibility affect online language comprehension, and how they impact longer-term memory and learning. We conducted an online study that consisted of a word-by-word self-paced reading (encoding) and a (surprise, i.e., unannounced) word recognition task (retrieval), administered about 15 minutes after initial sentence reading.
During self-paced reading, participants read sentences such as “Since Anne is afraid of spiders, she does not like going down into the…” which constrained expectations strongly toward a particular noun (e.g., “basement”; predictable-plausible condition), but instead were completed with unpredictable nouns that were somewhat plausible given the context (e.g., “garden”; unpredictable-somewhat plausible condition) or deeply implausible (e.g., “moon”; unpredictable-deeply implausible condition). All nouns were matched in word length and frequency. In order to distinguish effects of unpredictability from those of implausibility, we capitalized on a priori contrasts that separately tested for effects of predictability violations (i.e., contrast predictable-plausible vs unpredictable-somewhat implausible) and plausibility violations (i.e., contrast unpredictable-somewhat plausible against unpredictable-deeply implausible; see Schad et al., Reference Schad, Vasishth, Hohenstein and Kliegl2020).
In the noun recognition task, we probed participant’s memory for previously read nouns as well as new nouns, and we also asked them to indicate their confidence about their recognition judgments. According to dual-process models of recognition memory (Yonelinas, Reference Yonelinas2002), low-confidence judgments demonstrate implicit, gist-wise familiarity with an item, whereas high-confidence judgments indicate retrieval of more detailed, qualitatively more specific information about an item (Rugg & Curran, Reference Rugg and Curran2007; Yonelinas et al., Reference Yonelinas, Aly, Wang and Koen2010).
Our first research question concerned the timing of plausibility and predictability violations during initial sentence reading. If plausibility violations affect reading very early on, we would expect to find a pattern of results where plausibility violations affect reading before (Staub et al., Reference Staub, Rayner, Pollatsek, Hyönä and Majewski2007), or occur largely simultaneously with, effects elicited by predictability violations, possibly on the noun. On the other hand, if plausibility affects reading rather late, we would find a delayed plausibility effect (see e.g., Kuperberg et al., Reference Kuperberg, Brothers and Wlotko2020; Brothers et al., Reference Brothers, Wlotko, Warnke and Kuperberg2020), for example, slowed reading for words of the spill-over region after the noun. Any dissociation regarding the timing of the effects would inform models of human sentence reading (e.g., the EZ reader model; Reichle, Warren & McConnell, Reference Reichle, Warren and McConnell2009), which currently assume a two-step reading process where plausibility affects sentence reading at a post-lexical stage, that is, after word access (Abbott & Staub, Reference Abbott and Staub2015; but see Veldre et al., Reference Veldre, Reichle, Wong and Andrews2020, for simulations showing that EZ reader is able to account for very early-emerging effects of plausibility during reading). Our second question concerned the outcome of the behavioral word recognition task. If deeply implausible nouns drive memory and learning (Kuperberg et al., Reference Kuperberg, Brothers and Wlotko2020), we would find a memory difference between the two unpredictable conditions, that is, better recognition memory for unpredictable-deeply implausible compared to unpredictable-somewhat plausible items. This effect should be manifest especially in the high-confidence judgments, which we used as an index for conscious recollection (as opposed to implicit, gist-wise memory; Yonelinas, Reference Yonelinas2002). In contrast, if schema congruency boosts memory, we would expect a reversed predictability effect, that is, increased memory for predictable-plausible nouns, possibly compared to both unpredictable conditions.
Method
Participants
Eighty-threeFootnote 3 native speakers of German between the ages of 20 and 41 participated in the online experiment that lasted about one hour. All participants were psychology students and were compensated with course credit for their time. Participants had normal or corrected-to-normal vision and reported no neuropsychiatric medication and/or reading disabilities at the time of testing. Three subjects were excluded from further analysis because their average accuracy on the comprehension questions that checked for attentive reading during the experiment was below 75%. The final sample consisted of 80 participants (54 female, 25 male, 1 non-binary), with a mean age of 22 (SD = 5). Informed consent was obtained from all participants; all study procedures were in line with the Helsinki declaration on Human Subject Testing.
Materials and tasks
Materials for the self-paced reading task consisted of 45 German sentences presented in three conditions (predictable-plausible, unpredictable-somewhat plausible, unpredictable-deeply implausible); these sentence frames were taken from an earlier project conducted in our lab. The OSF Link to the online repository where all materials are uploaded is https://osf.io/t9nf3/. All sentence frames were relatively highly constraining toward a particular sentence-final noun, for example, “Kühlschrank” (“fridge”) in “In der Nachmittagshitze war der Wein warm geworden, also stellte Johanna ihn in …”; English approximation: “In the heat of the afternoon, the wine had become warm, and so Johanna put it in …”. (see Table 1, for example stimuli and literal English translations; see below for cloze probabilities). The predictable nouns from these sentence frames (i.e., “fridge”) served as the predictable-plausible condition for this study.
Table 1. Examples and English literal translations of experimental items used in the self-paced sentence reading task
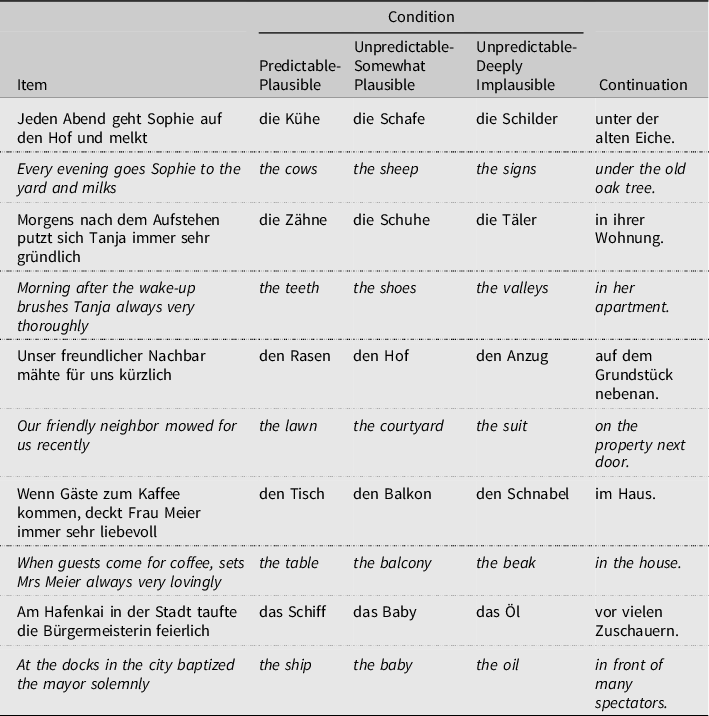
Note: Presentation rate was word-by-word (no chunking of multiple words).
To create unpredictable-somewhat plausible and unpredictable-deeply implausible continuations for each sentence frame, the experimenters then selected two further sentence-final nouns that did not occur as response in the cloze ratings and had the same grammatical gender as the predictable nouns (so that the gender-marked article preceding the nouns would not give away crucial information). These continuations were thought to be an unpredictable but somewhat plausible continuation of the sentence or an unpredictable-deeply implausible continuation (e.g., “Schatten,” “shade” vs “Spiegel,” “mirror,” respectively). The majority of deeply implausible nouns were selected so as to be incompatible with the selectional restrictions provided by the pre-nominal verb (e.g., “milk the sign,” “set the beak,” “baptize the oil,” “conduct the cereal”), if the verb was sufficiently restricting to allow for such a manipulation. In all other cases, deeply implausible nouns were selected on the basis of being impossible in the real world (e.g., it is physically impossible for a person to “put a bottle of wine into a mirror,” as much as it is physically impossible to “go into the moon”). Hence, deeply implausible nouns deviated from the rational assumption that a speaker would communicate literally about possible events in the literal world (see e.g., Brothers et al., Reference Brothers, Wlotko, Warnke and Kuperberg2020; Kuperberg et al., Reference Kuperberg, Brothers and Wlotko2020). Unpredictable-somewhat plausible nouns were chosen so as to be as plausible as possible given the sentence context, literally possible, and compatible with the selectional restrictions provided by the verb (e.g., it is literally possible and somewhat plausible to put a bottle of wine into the shade when it is too warm, as much as it is literally possible and plausible to not like going down into the garden when one is afraid of spiders).
Altogether, this yielded three conditions per sentence frame, and a total of 135 (45 times 3) unique experimental sentences (see Table 1): 45 predictable-plausible (e.g., “fridge”), 45 unpredictable-somewhat plausible (e.g., “shade”), 45 unpredictable-deeply implausible (e.g., “mirror”). For presentation during the self-paced reading task, the 135 items were arranged on 3 lists so that each experimental subject got to see one item in only one of its experimental versions (yielding a total of 45 sentences during SPR per subject).
The three sentence continuations were matched in gender. Note that German additionally marks for case on the definite article, but since case is dictated by the noun’s function in the sentence (which was identical for nouns across conditions per item), case marking on the definite article was also identical across conditions.
Nouns from the three conditions were matched pairwise with respect to word length (F(2, 132) = 1.57, p = .21; estimated marginal means, EMMs, for predictable-plausible, unpredictable-somewhat plausible, and unpredictable-deeply implausible nouns, respectively: 5.84, 6.27, 5.51). However, nouns from the three conditions differed from one another with respect to frequency (frequency estimates were based on the Zipf scale from the SUBTLEX DE database; see Brysbaert et al., Reference Brysbaert, Buchmeier, Conrad, Jacobs, Bölte and Böhl2011; F(2, 125)Footnote 4 = 3.48, p = .04), in that the predictable-plausible nouns were more frequent than the unpredictable-deeply implausible nouns (EMMs for the three conditions were 2.79, 2.51, 2.45). There were no significant differences in word frequency between deeply implausible and somewhat plausible nouns, or between somewhat plausible and predictable-plausible nouns (both p’s > .20). Note that the frequency difference between the predictable-plausible and unpredictable-deeply implausible nouns is of little concern to our investigation, not only because we included word frequency as a control variable in all models reported below but more importantly because we never directly compare these two critical conditions with one another.
Materials for the word recognition task consisted of the 45 “old” (i.e., previously seen) nouns from the self-paced reading task, and 15 additional “old” nouns that were selected from the sentence frames presented in the self-paced reading task, for example, “wine” for the item “In the heat of the afternoon, the wine had become warm, and so Johanna put it in …”. These 15 additional nouns were included as a “control” condition in order to investigate recognition effects for nouns that are initially read neither in a highly predictable nor in a highly unpredictable condition (as was the case for all predictable-plausible, and unpredictable-somewhat plausible and unpredictable-deeply implausible nouns). This yielded a total of 60 “old” nouns per subject for the word recognition task. “New” nouns (i.e., previously unseen nouns) consisted of 60 nouns that were not seen during the initial reading task of the experiment, neither in the SPR practice trials nor in fillers or in experimental items. These new nouns were selected from a custom subset of the SUBTLEX DE database, which only included words in which the first letter was capitalized (i.e., nouns; German nouns are capitalized). Post hoc t-tests showed that old and new nouns did not differ significantly from one another in word length (t(208) = 0.56, p = .60; average length in characters for old and new nouns, 5.85 vs 6.02, respectively). However, new nouns differed from old nouns with respect to frequency, in that new nouns were slightly less frequent than old nouns (t(200) = −2.50, p = .01; average count per million for old and new nouns, 46. 63 vs 16.97, respectively). Again, note that we are controlling for word frequency in all statistical analyses.
Pretest: Predictability
Cloze ratings for the predictable-plausible nouns were known based on earlier cloze ratings conducted in our lab, where 40 native speakers of German (mostly Psychology students; age range = 18–27 years; 25 female, 15 male), who did not participate in the present study, were presented with the sentence frames that were truncated before the pre-nominal definite article. Participants were asked to generate a definite article and noun that best completed the sentences. According to these ratings, predictable-plausible nouns had an average cloze probability of .77 (range = .3–1).
For unpredictable-somewhat plausible and unpredictable-deeply implausible nouns (which mostly yielded cloze probabilities of zero in prior ratings), we estimated cloze probabilities based on the procedure suggested in Lowder and colleagues (Reference Lowder, Choi, Ferreira and Henderson2018), where zero values in cloze ratings are replaced with half the value of the lowest nonzero cloze value. The lowest nonzero cloze value possible in our cloze rating study was .06, and so cloze values of zero were replaced with .03. On average, unpredictable-somewhat plausible items had a cloze probability of .03 (range = .03–.05, SD = .01), and unpredictable-deeply implausible nouns had cloze probabilities of .03 throughout (SD = .00). ANOVA showed significant differences for cloze probabilities across conditions, F(2, 132) = 792.80, p < .001. Post hoc t-tests demonstrated significant predictability differences between predictable-plausible and unpredictable-somewhat plausible nouns, and between predictable-plausible and unpredictable-deeply implausible nouns (both p’s < .001). Crucially, predictability for somewhat plausible and deeply implausible nouns did not differ from one another (p = .99).
Pretest: Plausibility
For the plausibility pretest, 44 native speakers of German (19 male, 25 female; mean age = 27 years; age range = 18–51 years), who did not participate in the main experiment or the cloze probability ratings, rated the 135 sentences for plausibility in an online questionnaire presented through the German online survey platform SoSciSurvey. Sentences were presented on three lists; each participant saw each item in only one of its experimental conditions (final number of subjects per list 1, 2, and 3: 14, 16, 14, respectively). Participants were presented with single sentences (e.g., “Since Anne is afraid of spiders, she doesn’t like going down into the garden or the washroom”) and asked to indicate, on a scale from 1 to 7, how plausibly the underlined noun completed the sentence. Prior to the ratings, they were given an example that illustrated the use of the scale and the meaning of the word “plausible” (e.g., “Every Sunday the religious widow goes to (the) church” (plausible, rating of 7), “graveyard” (somewhat plausible given the context and possible, rating of 3), “canvas” (implausible and impossible, rating of 1). Participants were instructed to use the full scale in their responses.
On average, predictable-plausible sentences received a plausibility rating of 6.56 (range = 5.75–7.00), unpredictable-somewhat plausible sentences received a rating of 3.94 (range = 1.06–6.64), and unpredictable-deeply implausible sentences received a rating of 1.41 (range = 1.00–2.71). To analyze the plausibility ratings statistically, we ran cumulative link models using custom-built contrasts for the predictor variable condition on the unaggregated plausibility ratings, as implemented in the R package ordinal. We also included random intercepts for subjects and items. In keeping with our main analysis, we specified two contrasts. The first contrast compared the unpredictable-somewhat plausible condition to the predictable-plausible condition (i.e., the predictability contrast). The second contrast compared the unpredictable-deeply implausible condition to the unpredictable-somewhat plausible condition (i.e., plausibility contrast). The results showed significant plausibility differences between unpredictable-somewhat plausible and predictable-plausible items (contrast 1: b = −3.86, z = −13.62, p < .001) and between unpredictable-deeply implausible unpredictable-somewhat plausible sentences (contrast 2: b = −3.77, z = −13.43, p < .001). Hence, plausibility differed between unpredictable-deeply implausible sentences and unpredictable-somewhat plausible sentences. However, plausibility also differed between unpredictable-somewhat plausible and predictable-plausible items. We return to this point in the General Discussion.
Procedure
The experiment was run online using the platform LabVanced; anyone with the link to the experiment could participate. The link was disseminated using an online recruitment website that advertises studies to Psychology students. The experiment consisted of three major parts. The first part was a non-cumulative word-by-word self-paced reading task (∼ 20–25 min), followed by a 10-min retention interval (in which participants completed the Digit Symbol Substitution Test; a test of processing speed, not reported here). The third part of the experiment was the word recognition task (∼15 mins).
In the self-paced reading task, participants read the experimental sentences on a screen word-by-word (each word was only displayed once and was not replaced by dashes later on, no mask). Each word was presented in the center of a white screen using Lucinda 18pt font and stayed on the screen until participants pushed the space bar, which revealed the next word in the sentence, and so on. All 135 unique sentences were arranged on three lists, so that there were 45 experimental sentences per list in the SPR task. Each list also contained an additional 33 moderately predictable filler items from the Potsdam sentence corpus, which were inserted to encourage people to generate predictions during reading, despite the large number of unpredictable sentence endings. Comprehension questions were inserted on 40% of the filler items to ensure that participants were reading for contentFootnote 5 . The order of sentence presentation on each list was pseudo-randomized, with two constraints: (1) no more than 4 unpredictable sentences in a row, (2) no more than 4 items with comprehension questions in a row. The experimental task was preceded by eight practice sentences, which were implemented to make sure that participants could get accustomed by the word-by-word reading task. All subjects were instructed to read the sentences as quickly and thoroughly as possible, and to answer all comprehension questions as accurately as possible by pushing the “S” (Yes, correct) and “L” (No, incorrect) keys on the keyboard. All sentences were separated by a fixation screen which displayed “Ready? Press the space bar to begin” until participants pressed the button.
In the word recognition task, participants saw single words (nouns) appear centrally on the screen in a Lucinda 18pt font. Participants were instructed to discriminate “old” from “new” nouns (i.e., previously seen or not seen during the sentence reading part of the experiment), and that they should additionally indicate their confidence about their judgment. This resulted in four response options per single trial: sure old, maybe old, sure new, and maybe new. Participants were instructed to put the index and middle finger of both hands on the “S,” “D” (sure new, maybe new) and “J,” “K” (maybe old, sure old) keys and to keep them there for the duration of the task. At the bottom of every trial, there was an additional figure display of the response options (SD, JK), as well as a schematic display of two hands with stretched-out index and middle fingers, overlaid over the response options. Participants were instructed to respond to each noun as accurately as possible.
Results
We report results for the word-by-word self-paced reading task and subsequent word recognition task. Data were analyzed using linear mixed effects models (Baayen et al., Reference Baayen, Davidson and Bates2008) as implemented in the lme4 library (Bates et al., Reference Bates, Mächler, Bolker and Walker2015) in R (R Core Team, 2021; version 3.6.2). Word recognition rates were analyzed using glmer, appropriate for binary dependent variables. The OSF Link to the online repository where all data frames and R analysis scripts are uploaded is https://osf.io/t9nf3/.
Since R does not provide p-values for LMER models, we used the R package lmerTest (Kuznetsova, Brockhoff, & Christensen, Reference Kuznetsova, Brockhoff and Christensen2017) to obtain p-values for the self-paced reading data (note that lmerTest estimates p-values using the Satterthwaite degrees of freedom method). All models were fit with the categorical predictor condition (three levels: predictable-plausible, unpredictable-somewhat plausible, and unpredictable-deeply implausible) and included control variables for word length and word frequency. Additional control predictors that were unique to self-paced reading and/or word recognition models are reported in the respective sections. All models included random effects for subjects and items and were initially fit with the fullest random slope structure warranted by the design (Barr et al., Reference Barr, Levy, Scheepers and Tily2013). The final fit is detailed for each model separately in the results section. All predictors were effect-coded, so that model coefficients need to be interpreted as simple effects (i.e., not ANOVA-style main effects). For the condition variable, we used sliding differences coding, to compare (in a first comparison) the unpredictable-somewhat plausible condition to the predictable-plausible condition (henceforth, predictability contrast). The second comparison for the condition variable compared the unpredictable-deeply implausible condition to the unpredictable-somewhat plausible condition (henceforth, plausibility contrast). We made use of model comparisons to check whether excluding non-significant and not trending-toward-significance control predictors improves model fit. For these comparisons, we used a likelihood ratio test and evaluated significance against the χ 2 distribution, taking as the degrees of freedom the difference in number of parameters between the two critical models.
Self-paced sentence reading: Comprehension accuracy
Accuracy on the comprehension questions was very high with 93% correct answers across all subjects (SD = 4, range = 77–100). This suggests that participants were attentive during the experiment and understood the sentences they were reading.
Self-paced sentence reading: Reading times
Models for the self-paced reading data additionally included a control predictor for trial number to offset effects of customization that normally occur during reading studies. We report our results for two regions of interest, the critical noun (e.g., “basement/garden/moon”; henceforth “early” region) and the two spill-over words following immediately after the noun (spill-over word 1 and spill-over word 2; e.g., “ or the washroom”), collapsed into one region (henceforth, “late” region). Instead of running two models (i.e., one model per region early and late), we ran one model by entering region as an interaction variable. Therefore, there were two categorical predictors in the model, region (early vs late) and condition (predictable-plausible, unpredictable-somewhat plausible, and unpredictable-deeply implausible), including their interaction. Prior to analysis, and based on visual inspection of the data, RT data were trimmed minimally and RTs outliers faster than 100 ms and slower than 2000 ms were excluded from the analysis. Altogether, this procedure affected less than 2% of all data points. Prior to analysis, all RT data were log-transformed to avoid skewness. Running identical analysesFootnote 6 to those reported below on the untransformed data did not change results. The final model that converged contained random intercepts for subjects and items and by-subject random slopes for condition. To facilitate interpretation of the analyses reported below, means and standard deviations of untransformed reading times are reported in Table 2.
Table 2. Means and standard deviations of word-by-word reading times (and SD; both in ms) in the self-paced reading task, split out by sentence condition

The model showed a significant effect for predictability (b = 0.03, SE = 0.01, t = 2.93, p < .01; see Figure 1), suggesting longer reading times for unpredictable-somewhat plausible sentences than for predictable-plausible sentences, irrespective of region. The simple effect of plausibility was not significant (b = 0.01, SE = 0.01, t = 1.28, p = .20), but there was a significant, positive-going, interaction between plausibility and region (b = 0.06, SE = 0.01, t = 4.89, p < .001), suggesting a larger plausibility effect for the late, compared to the early, region. Planned model splits that investigated RTs for the noun and the spill-over region separately showed an effect of plausibility in the late region (b = 0.07, SE = 0.01, t = 9.04, p < .001), but no such effect in the early region (i.e., on the noun; b = 0.02, SE = 0.01, t = 1.56, p = .12). Hence, predictability affected reading earlier than plausibility didFootnote 7 .

Figure 1. Coefficient Plot of Estimated Log-Transformed RTs in the Self-Paced Reading Task, Depending on Condition and Region. Statistical analyses were conducted using a binary factor for region, using noun as “early” region, and spill-over word 1 and spill-over word 2 (collapsed) as “late” region. Error bars represent 95% SE.
In order to quantify and statistically compare the size of the RT slow-down associated with predictability and plausibility violations, we computed per-subject difference scores for log-RTs for the plausibility effect (log-RT unpredictable-deeply implausible condition minus log-RT unpredictable-somewhat plausible condition) and the predictability effect (log-RT unpredictable-somewhat plausible condition minus log-RT predictable-plausible condition). This was done across regions, that is, collapsing over the noun and the two spill-over words after the noun. Repeated measures ANOVA showed a significant difference between the size of the plausibility violation and the size of the predictability violation, F(1,79) = 4.07, p < .05. Estimated marginal means were larger for the plausibility effect (M = 0.05 log-RT, M = 35.90 ms in untransformed RT) compared to predictability effect (M = 0.02 log-RT, M = 12.10 ms in untransformed RT).
In sum, predictability and plausibility violations affected reading rates both with respect to timing and size of the effect. With respect to timing, predictability affected reading rates regardless of region, in that unpredictable-somewhat plausible sentences were read more slowly than predictable-plausible items throughout. In contrast, plausibility affected reading rates only in the late region (but not in the early region), in that unpredictable-deeply implausible sentences were read more slowly than unpredictable-somewhat implausible items in the spill-over region (but not at the noun). With respect to size of the effect, the slow-down in reading rates associated with plausibility violations was more pronounced (an effect almost three times the size in raw RTs) than the one associated with predictability violations.
Word recognition
Irrespective of confidence, participants correctly recognized 71% of the “old” nouns (range = 47%–96%), and false alarmed to an average of 26% of all “new” nouns (range = 3%–53%). The larger hit rate to seen words than false alarm rate to unseen words indicates that participants were paying attention during the experiment and remembered the words. Average d’ was 1.29 (SD = 0.39, range = 0.42–2.41), which is similar in size to earlier psycholinguistic studies examining effects of predictability on word recognition (e.g., Hubbard et al., Reference Hubbard, Rommers, Jacobs and Federmeier2019; Rommers & Federmeier, Reference Rommers and Federmeier2018; Rasenberg et al., Reference Rasenberg, Rommers and Van Bergen2020). One participant was excluded from the analyses reported below because they did not use the full response scale (i.e., they only gave high-confidence responses).Footnote 8
For the main statistical analysis of the word recognition data, recognition accuracy was analyzed on unaggregated data. We chose this analysis technique over running ANOVAs on per-subject aggregated d’ scores, as per-subject ANOVAs ignore variance over items, which is statistically problematic (see Clark, Reference Clark1973; Raaijmakers et al., Reference Raaijmakers, Schrijnemakers and Gremmen1999; Raaijmakers, Reference Raaijmakers2003). Further, per-subject aggregated data do not allow for the simultaneous inclusion of per-subject and per-item control variables such as speed of initial word encoding and word frequency.
The dependent variable was recognition accuracy, coded in 0s and 1s. Fixed effects in the model were condition (contrast-coded; see below) and confidence (with two levels: low vs high), including their interaction. The model also contained control variables for word frequency, word length, and encoding RT of the respective noun (log-transformed), in order to account for the fact that longer reading times during the SPR task would likely increase memory performance in the recognition task. Average accuracy values and corresponding standard deviations per condition and confidence are shown in Table 3.
Table 3. Means and standard deviations of accuracy rates in the word recognition task, split out by condition and confidence

Results from two models are reported here. The first model compared effects for nouns initially processed in the three critical conditions in the study (i.e., predictable-plausible, unpredictable-somewhat plausible, unpredictable-deeply implausible; excluding the control nouns from the contexts). That first model used the same contrast coding as before, in that there was a predictability contrast (i.e., unpredictable-somewhat plausible vs the predictable-plausible) and a plausibility contrast (i.e., unpredictable-deeply implausible vs unpredictable-somewhat plausible). The second model was exploratory in nature and investigated memory effects for the control nouns from the sentence contexts. Recall that the control nouns were included in the memory test to probe recognition of words that are neither highly predictable nor highly unpredictable during initial reading. Therefore, the contrasts in that second model compared memory rates of control words to (1) the grand mean of unpredictable nouns (i.e., unpredictable-somewhat plausible and unpredictable-deeply implausible) and (2) the control condition to the predictable-plausible nouns.
Model 1: Predictability vs plausibility
The interactions between condition and confidence were not significant so the interaction term was removed from the model, χ 2 (2) = 2.05, p = .40. We also removed the scaled continuous predictor for noun length, χ 2 (1) = 0.02, p = .89. The final model contained per-subject random intercepts, with no other random effects specified. There was a significant effect of plausibility (b = 0.29, SE = 0.11, z = 2.74, p < .01; see Figure 2, for effects plot), indicating that recognition accuracy was better for unpredictable, deeply implausible nouns than for unpredictable, somewhat plausible nouns overall, irrespective of confidence. There was also a simple effect of confidence (b = 1.84, SE = 0.09, z = 19.76, p < .001), suggesting higher recognition accuracy for high- compared to low-confidence judgments. Notably, the simple effect of predictability failed to reach statistical significance (b = −0.01, SE = 0.10, z = −0.12, p = .90). Finally, there were significant, positive-going effects for noun RT during initial reading and for word frequency, suggesting that longer reading times and higher word frequency improved recognition overall (b = 0.29, SE = 0.13, z = 2.18, p < .05, and b = 0.29, SE = .07, z = 4.30, p < .001, respectively)Footnote 9 .

Figure 2. Coefficient Plot Illustrating the Effects of Predictability and Plausibility, as well as Confidence, on Accuracy Rates in the Noun Recognition Task. Error bars represent 95% SE.
Model 2: Control vs predictable; control vs unpredictable
There was no significant effect for the scaled continuous predictor for noun length, χ 2 (1) = 0.98, p = .32, so it was removed from the model. The final model that converged contained per-subject and per-item random intercepts and per-subject random slopes for confidence. Figure 3 shows a partial effects plot.

Figure 3. Coefficient Plot Illustrating the Recognition Accuracy Results for Control Nouns (i.e., Nouns from the Sentence Context), as well as Predictable and Unpredictable Nouns. The predictable condition represents the predictable-plausible nouns, whereas the unpredictable condition represents the grand mean of the unpredictable-deeply implausible and unpredictable-somewhat plausible nouns. Error bars represent 95% CI.
There were significant effects for confidence (b = −1.89, SE = 0.13, z = −14.97, p < .001; see Figure 3) and word frequency (b = 0.37, SE = 0.07, z = 5.16, p < .001; again, note the positive-going effect here), indicating that recognition accuracy was better for high-confidence judgments and for words with higher frequency. There was also a marginally significant interaction between confidence and the contrast control vs unpredictable (b = 0.38, SE = .19, z = 1.96, p = .05). There were no effects for the contrast control vs predictable, neither as a simple effect (b = 0.03, SE = 0.18, z = 0.14, p = .89) nor as an interaction with confidence (b = 0.08, SE = 0.22, z = 0.38, p = 0.70).
To examine the interaction between predictability and confidence more closely, we ran separate follow models in which we split items between low- and high-confidence judgments. However, both resulting models showed non-significant effects for predictability (control vs unpredictable in low-confidence judgments: b = −0.80, SE = 0.13, z = −0.62, p = .50; control vs unpredictable in high-confidence judgments: b = 0.33, SE = 0.18, z = 1.87, p = .06). Numerically though, there was a trend for unpredictable nouns to be recognized more accurately (compared to the control nouns) in high-confidence judgments.
In sum, plausibility violations improved memory performance both with respect to implicit, gist-wise memory (i.e., low-confidence memory judgments) and more conscious recollection (i.e., high-confidence memory judgments). There were no clear effects for control nouns (i.e., nouns taken from the sentence context).
Discussion
Predictability and plausibility affect language comprehension, but little is known about how they interact during online sentence processing. Recently, studies have made different predictions about the longer-term memory consequences of predictability and plausibility that exceed stages of initial processing. Crucially though, these longer-term consequences have not been investigated to date. Here, we used a self-paced sentence reading and subsequent word recognition task to investigate initial processing and longer-term memory of predictability and plausibility violations. Experimental sentences in the SPR task either involved a prediction violation without strongly violating plausibility (e.g., “Every evening Sophie goes to the yard and milks the sheep” when “cows” is expected), or they additionally violated plausibility (“… milks the signs”). In the subsequent memory task, we probed people’s recognition memory of nouns (e.g., “sheep”/ “cows”/“signs”) that they had previously read.
Our results were as follows. During initial reading of the sentences, there was an early-emerging and longer-lasting effect of predictability, beginning on the target noun, suggesting comprehension difficulties when reading unpredictable nouns that were somewhat plausible (e.g., “sheep”). These comprehension difficulties continued onto RTs of subsequent words after the critical nouns (i.e., the spill-over region). In contrast, violations of plausibility (e.g., “signs”) did not affect reading rates until after the noun, that is, on the spill-over region. Hence, effects of predictability violations preceded those of plausibility violations during initial sentence reading. Irrespective of their onset, our data also showed that comprehension difficulties associated with plausibility violations were much larger in size than those associated with mere unpredictability.
During subsequent word recognition, severe violations of plausibility (e.g., “milk the signs”) boosted memory across low- and high-confidence memory judgments, indicating that nouns previously encountered as a plausibility violations elicited not only greater implicit memory among participants but also greater conscious recollection. Memory was not increased for unpredictable but plausible nouns or for nouns that were predictable-plausible. We discuss our findings in greater detail below.
Effects of predictability and plausibility violations on sentence processing
Our data show that, when reading sentences that strongly constrained expectations toward a particular noun, comprehension difficulties associated with unpredictability emerge earlier in the processing stream than effects of implausibility; in other words, readers appreciated effects of unpredictability before those of implausibility. These results confirm prior ERP studies attesting to later-emerging effects of plausibility violations (e.g., Brothers et al., Reference Brothers, Swaab and Traxler2015; Brothers et al., Reference Brothers, Wlotko, Warnke and Kuperberg2020; Brouwer et al., Reference Brouwer, Delogu, Venhuizen and Crocker2021; DeLong et al., Reference DeLong, Quante and Kutas2014; Kuperberg et al., Reference Kuperberg, Brothers and Wlotko2020; Thornhill & Van Petten, Reference Thornhill and Van Petten2012). For example, Brouwer and colleagues (Reference Brouwer, Delogu, Venhuizen and Crocker2021) found that violations of plausibility primarily affect the P600 ERP component, whereas those of predictability are manifest in the N400 ERP component. Data obtained by Nieuwland and colleagues (Reference Nieuwland, Barr, Bartolozzi, Busch-Moreno, Darley, Donaldson, Fu, Heyselaar, Huettig, Husband, Ito, Kazanina, Kogan, Kohút, Kulakova, Mézière, Politzer-Ahles, Rousselet and Von Grebmer Zu Wolfsthurn2020) are, at least qualitatively, in line with this, as in that study, plausibility effects emerged later in the N400 amplitude than effects of predictability.
It is worth pointing out here again that not all previous literature reports later-emerging effects of plausibility violations. A series of eye-tracking studies has previously attested to relatively early-emerging effects, with plausibility affecting eye-tracking measures as early as first fixation duration, often believed to reflect early lexical access (as opposed to late semantic integration). In contrast, later-emerging effects of plausibility violations have often been reported in ERP studies. These conflicting findings are sometimes attributed to methodological differences between studies. For example, most ERP studies use fixed word-by-word presentation rates, which may push back or delay integration effects from sentence constituents onto subsequent words of the sentence (Roland et al., Reference Roland, Mauner and Hirose2021; Smith & Levy, Reference Smith and Levy2013). Similarly, late plausibility effects may be a consequence of participant instructions that force “deep” comprehension (i.e., meaningfulness judgments or plausibility ratings after sentence offset which may cause participants to adopt a more cautious or more careful processing strategy; see Brothers et al., Reference Brothers, Wlotko, Warnke and Kuperberg2020; Paczynski & Kuperberg, Reference Paczynski and Kuperberg2012), or they may result from material selection (e.g., selectional restriction violations such as “entertaining a backpack” or “inflating a carrot” often affect processing earlier than other types of violations; see Rayner et al., Reference Rayner, Warren, Juhasz and Liversedge2004; Staub et al., Reference Staub, Rayner, Pollatsek, Hyönä and Majewski2007; Warren & McConnell, Reference Warren and McConnell2007; Warren et al., Reference Warren, Milburn, Patson and Dickey2015; but see Vega-Mendoza et al., Reference Vega-Mendoza, Pickering and Nieuwland2021; for contrasting findings using ERPs).
To what extent may the data reported here be affected by such methodological aspects as well? “Deep” comprehension strategies are relatively unlikely to account for the present data since in our experiment, people were merely instructed to read for comprehension. The other methodological aspect, word-by-word presentation rate, is more difficult to account for. We cannot fully exclude the possibility that the late-emerging plausibility effect found here may have resulted from spill-over effects that are known to come to bear in experiments using word-by-word presentation of sentences. However, if this were true, it would raise the question why predictability effects emerged with no such delay. Since both manipulations in the present study were semantic in nature, we conjecture that a general methodological constraint would have presumably affected both types of manipulations, not only one. Finally, with respect to stimulus selection, we explored the possibility that the late-emerging effect of plausibility violations in the present study was primarily driven by items that did not involve verb-based selectional restriction violations, by entering the predictor “type of violation” (selectional restriction vs world- or event-based) as an additional interaction variable to our RT models. There were no effects.
On a more theoretical level, what does it mean when effects of predictability and plausibility emerge with diverging temporal footprints? One possible explanation is that the two variables, despite their obvious correlation, may be dissociable on cognitive grounds. For example, some researchers consider predictability and plausibility as equivalent to two different processing strategies that humans use to process language, that is, prediction and integration (Nieuwland et al., Reference Nieuwland, Barr, Bartolozzi, Busch-Moreno, Darley, Donaldson, Fu, Heyselaar, Huettig, Husband, Ito, Kazanina, Kogan, Kohút, Kulakova, Mézière, Politzer-Ahles, Rousselet and Von Grebmer Zu Wolfsthurn2020; DeLong et al., Reference DeLong, Urbach and Kutas2005; Nieuwland et al., Reference Nieuwland, Politzer-Ahles, Heyselaar, Segaert, Darley, Kazanina, Bartolozzi, Kogan, Ito, Méziere, Barr, Rousselet, Ferguson, Busch-Moreno, Fu, Tuomainen, Kulakova, Husband and Huettig2018). Although integration refers to the ease with which words can be combined with previous words in the sentence, prediction refers to pre-activation of words before they are processed, by means of incremental formulation of expectations (for recent review, see Bovolenta & Marsden, Reference Bovolenta and Marsden2021). Early-emerging effects of predictability are then taken to mean that prediction takes precedence in the processing stream (e.g., Brothers et al., Reference Brothers, Swaab and Traxler2015), but also that effects of prediction are truly conceptually separable from effects of integration – a view often shunned by traditional linguistics (Jackendoff, Reference Jackendoff2002) and the initial literature on sentence processing (see Van Petten & Luka, Reference Van Petten and Luka2012, for review). Others have attributed relatively late-emerging effects of plausibility violations to a staged language comprehension process (see e.g., the EZ Reader model by Reichle and colleagues, Reference Reichle, Warren and McConnell2009), where effects related to word form (such word frequency) are taken into account earlier in the processing stream than effects related to word meaning (such as plausibility).
The findings presented here align with both of these accounts. Intuitively, it would seem to make some sense that language users appreciate effects of predictability before those of plausibility. Prior research has shown that in situations where a sentence context enables very specific predictions (like the ones used here), language users may pre-activate word form (e.g., DeLong et al., Reference DeLong, Urbach and Kutas2005; Fleur et al., Reference Fleur, Flecken, Rommers and Nieuwland2020; Haeuser, Kray, & Borovsky, Reference Haeuser, Kray, Borovsky, Denison, Mack, Xu and Armstrong2020; Van Berkum et al., Reference Van Berkum, Brown, Zwitserlood, Kooijman and Hagoort2005; but see Ito et al., Reference Ito, Martin and Nieuwland2017, and Nieuwland et al., Reference Nieuwland, Politzer-Ahles, Heyselaar, Segaert, Darley, Kazanina, Bartolozzi, Kogan, Ito, Méziere, Barr, Rousselet, Ferguson, Busch-Moreno, Fu, Tuomainen, Kulakova, Husband and Huettig2018, for failed replication; see Nieuwland, Reference Nieuwland2019, for critical discussion) and orthographical features of words (e.g., Balota et al., Reference Balota, Pollatsek and Rayner1985; Kim & Lai, Reference Kim and Lai2012; Laszlo & Federmeier, Reference Laszlo and Federmeier2009; Luke & Christianson, 2012). In fact, we know that the stimuli used in this study did have such an effect on people in that they allowed for specific predictions regarding word forms (see Haeuser et al., Reference Haeuser, Kray, Borovsky, Denison, Mack, Xu and Armstrong2020; Haeuser et al., accepted; Haeuser & Kray, Reference Haeuser and Kray2021). We could speculate that, in the case of disconfirmed predictions, the early-emerging predictability effect may have reflected some early-emerging detection mechanism, signaling that the predicted word has not been encountered (cf., Kuperberg et al., Reference Kuperberg, Brothers and Wlotko2020), or that vice versa, predictable words show a boost in pre-activation compared to other words which are at baseline (Frisson et al., Reference Frisson, Harvey and Staub2017). This would additionally explain why, in our study, at the noun, reading times of the deeply implausible condition did not differ from the one of the somewhat plausible condition: In both conditions, regardless of the meaning of the noun, the word form mismatched with what people may have expected to occur.
Effects of predictability and plausibility violations on memory
Our results from the recognition task are very clear in suggesting that unpredictable, deeply implausible information increases memory performance, that is, recognition memory was enhanced for nouns previously encoded in an unpredictable-deeply implausible condition, compared to nouns encoded in an unpredictable-somewhat plausible condition (and also, numerically than nouns encoded in a predictable-plausible conditions, though our contrasts did not specifically test for this). The fact that the plausibility effect held up across low- and high-confidence judgments suggests that deep implausibility not only engendered a sense of implicit memory among participants (i.e., gist-wise memory), but also more conscious recollection (Yonelinas, Reference Yonelinas2002). In contrast, recognition memory did not differ between predictable-plausible and unpredictable-implausible nouns (i.e., there was no predictability effect).
The lack of a predictability effect is interesting because it suggests that unpredictability alone was not sufficient to drive memory. If unpredictability improved memory across the board, we would have obtained a pattern of results where not only the unpredictable-deeply implausible nouns would have been remembered accurately, but also the unpredictable-somewhat plausible nouns. Hence, the recognition data reported here tentatively suggest that only very strong conflicts (i.e., a certain magnitude of prediction error) between predicted information and actual outcome suffice to trigger episodic recollection.
Our results align with the predictions made by the sentence processing framework by Kuperberg and colleagues (Kuperberg et al., Reference Kuperberg, Brothers and Wlotko2020). According to that framework, language users represent contextual information on three hierarchical levels, with the top-level situation model representing the comprehender’s probabilistic beliefs about possible situations or real-world contingencies that could be generating a set of recently observed events. Deeply implausible events should trigger re-adjustments in this top level of the hierarchy, maybe because the only way to accommodate them would be for language users to temporarily abandon rational beliefs about real-word events. For example, specifically in reference to our stimuli, participants may start embracing the idea of a cartoon scenario where it is somewhat plausible for a person to “go into the moon” (e.g., the moon may have an entrance door that gives access to a labyrinth of corridors and rooms that people can pass through).
Therefore, violations of predictability alone are not sufficient to drive memory; instead, it requires a certain magnitude of surprisal during initial encoding to facilitate learning. This view fits with the results of studies on developmental language learning (Fazio & Marsh, Reference Fazio and Marsh2009; Stahl & Feigenson, Reference Stahl and Feigenson2017). For example, Stahl and Feigenson (Reference Stahl and Feigenson2017) showed that when children learn novel verbs in a deeply implausible condition that violates core expectations about object continuity (e.g., an object magically disappearing from one place and showing up in another), they are more likely to correctly learn the meaning of the novel word, compared to when the verb referred to an expected-plausible outcome (e.g., an object changing locations after having been moved by the experimenter). Similarly, Fazio and Marsh (Reference Fazio and Marsh2009) showed that young adults’ source memory for general-knowledge items was highest in the condition when they received feedback on an incorrect response given with high confidence, in other words, in the condition when the feedback was most surprising and therefore, most highly informative.
Together, these data can be accommodated by a Bayesian framework of learning. On such a framework, people have priors about real-word contingencies or object behavior, and they continuously observe evidence from the world to update their priors to posterior probabilities. Upon encountering events that are sufficiently surprising, learners might update their priors to a greater degree than when encountering expected or mildly surprising events. Ultimately, it is worth pointing out that our data cannot speak to the exact workings of the plausibility effects, and how precisely it drives superior word recognition (cf., Wagner et al., Reference Wagner, Koutstaal and Schacter1999). One possibility is that reading a deeply implausible noun caused readers to allocate more attention to it. Another, not mutually exclusive, possibility is that word recognition was driven by the depth with which the deeply implausible noun was processed, that is, participants reading about a person going into the moon may have been compelled to think about the action more deeply (e.g., the cartoon scenario described above). To the extent that our processing measure for the encoding task, that is, reading times, is driven by either of these two possibilities, we have no way of adjudicating between them.
One direct impact of the memory results in our study is that they inform current models on error-driven learning (reviewed in Rabagliati et al., Reference Rabagliati, Gambi and Pickering2016) which argue that prediction error is one way to explain how different types of cognitive representations are acquired, including linguistic knowledge in the L1 and L2. The recent popularity of such models in psycholinguistic research notwithstanding (see Bovolenta & Marsden, Reference Bovolenta and Marsden2021; for a review on L1 and L2 processing), the behavioral evidence attesting to a significant role of prediction error in language learning has been surprisingly mixed (Gambi et al., Reference Gambi, Pickering and Rabagliati2021; Bovolenta & Marsden, Reference Bovolenta and Marsden2021; Reuter et al., Reference Reuter, Borovsky and Lew-Williams2019). Our data offer one potential explanation: Only a certain magnitude of prediction error (as in, information that is deeply implausible given one’s world knowledge and therefore, potentially, more distinctive) will trigger learning.
One applied use of our findings would be in terms of second language learning, where teachers may be best advised to build sufficiently constraining learning environments that violate core features of objects in order to facilitate word learning in students. For example, when the goal is to learn L2 words from a semantic category (e.g., “stuff in my bathroom”), the corresponding objects and their word labels could be put into a context where the occurrence of these objects is highly unlikely and therefore informative to students (e.g., a plastic duck that normally sits in the bathtub is presented sitting on the toaster in the kitchen; see Van Kesteren et al., Reference Van Kesteren, Ruiter, Fernández and Henson2012). Similar learning mechanisms could be applied to clinical settings that treat patients with aphasia or in children with specific language impairment.
Limitations and future directions
There are questions and limitations of the present study that remain unanswered and unresolved. One remaining question is whether and if so, to what extent, other linguistic variables that are known to moderate language processing affected our results. One such variable, for example, is semantic similarity, as in the semantic association between target nouns and preceding sentence contexts. It is conceivable that the predictable-plausible nouns used in the present study, in addition to being more predictable and more plausible, were also more semantically similar to the context frames than both types of unpredictable nouns (e.g., “Having arrived at the camping site, the family started to assemble the tent/raft/glass”). Since we did not collect semantic similarity ratings for our stimuli, we have no way of ascertaining whether this variable affected our results. However, we believe that semantic similarity would have affected predominantly the predictability contrast, and not the plausibility contrast, since the latter compared both unpredictable nouns where semantic similarity should have been equally low. Hence, if semantic similarity affected our results, it would have driven primarily the predictability effect. We are not sure to what extent this would affect our conclusions at all, as there are different accounts of whether prediction refers to passively spreading activation or top-down driven and controlled activation. Under the “passive spreading” account, predictability effects may be conceptually similar to semantic similarity effects.
Another unanswered question is whether the use of deeply implausible sentences during the initial reading phase may have affected, or partially driven, the low memory results for the unexpected-somewhat plausible items. Specifically, the relatively large “oddness” of the deeply implausible sentences may have boosted their distinctiveness during initial reading, potentially impeding successful recognition memory for other unexpected items which, under these specific circumstances, failed to “stand out” in any way. One way to address this concern in future research would be to run a study that does not include deeply implausible sentences at all, and then check whether this manipulation changes the memory results for the unexpected-somewhat plausible items.
Another limitation of the present study lies in the nature of the predictability contrast, which we operationalized as the difference between the predictable-plausible condition and the unpredictable-somewhat plausible condition. Given the nature of our stimuli, however, this contrast was arguably not as pure a contrast as the plausibility contrast, because the critical conditions differed not only with respect to predictability, but also, minimally, with respect to plausibility (cf. the average plausibility ratings: Mpred_plaus = 6.6, Munpred_someplaus = 3.9). We conjecture that in highly constraining sentence contexts like the ones we used, any unpredictale noun will be rated as less plausible (see Materials and plausibility ratings in DeLong et al., Reference DeLong, Quante and Kutas2014; Nieuwland et al., Reference Nieuwland, Barr, Bartolozzi, Busch-Moreno, Darley, Donaldson, Fu, Heyselaar, Huettig, Husband, Ito, Kazanina, Kogan, Kohút, Kulakova, Mézière, Politzer-Ahles, Rousselet and Von Grebmer Zu Wolfsthurn2020; Quante et al., Reference Quante, Bölte and Zwitserlood2018; Brothers et al., Reference Brothers, Swaab and Traxler2015; also see stimuli used by Kuperberg et al., Reference Kuperberg, Brothers and Wlotko2020, even though there were no plausibility ratings for that study). One way to address this problem in future studies would be to use low-constraint sentence contexts, though it might prove difficult to obtain very high-cloze probability ratings for predictable nouns using low-constraint sentence frames.
Similar methodological limitations might apply to the two unpredictable sentence continuations used in the present study, since cloze probability ratings collected from small sample sizes have limited power to estimate really low cloze probabilities (cf. Lowder et al., Reference Lowder, Choi, Ferreira and Henderson2018). We conjecture that this could have affected the cloze probability estimates for the unpredictable-somewhat plausible items in particular, as it is conceivable that, had we collected ratings from a substantially larger amount of people (i.e., tens of thousands; see Kuperberg & Jaeger, Reference Kuperberg and Jaeger2016), the cloze probabilities for this condition would have been higher than estimated here.
During peer review of this paper, the question surfaced how our recognition memory results square with other findings in the literature suggesting that people sometimes retain incorrect information about a sentence that aligns with general world knowledge. For example, in “The man bit the dog,” people sometimes indicate that it was the dog that was doing the biting when probed for their comprehension of that sentence later on (see Bader & Meng, Reference Bader and Meng2018; Meng & Bader, Reference Meng and Bader2021). The findings presented here may be interpreted to conflict with these extant results as they predict that what should be memorized for such sentences is the correct reading of that sentence, that is, the man doing the biting (as it violates plausibility). However, we believe that these “false memory” results on thematic role reversals are difficult to compare to the findings reported here, as the present study only measured veridical (i.e., true) memory, and not false memory. In fact, we do know that unpredictable sentences (like the ones used here) induce false memory effects that are not so unlike the ones reported in the literature on thematic role reversals (i.e., people sometimes show false recognition memory for nouns initially predicted during reading, compared to new nouns that were not seen and not predicted). Hence, it is possible that the true memory results found here hold up (i.e., better memory for deeply implausible nouns) regardless of people additionally showing false memories.
Conclusions
Our results demonstrate that, when reading sentences for comprehension, readers appreciate effects of unpredictability earlier than those of deep implausibility and that deep implausibility leads to larger and more persistent comprehension difficulties. In addition, nouns initially processed as deeply implausible prediction violations boosted implicit memory and conscious recollection during later recognition. Our self-paced reading data suggest that different types of semantic information (predictability, plausibility) affect reading rates at distinct time points. Our memory results indicate that violations of plausibility cause a greater shift in updating and learning, facilitating memory for events later on.
Replication package
The OSF Link to the online repository where all data frames and R analysis scripts are uploaded is https://osf.io/t9nf3/.
Acknowledgements
The authors would like to thank four anonymous reviewers and Kiel Christianson, for their valuable comments and feedback that helped improve the paper. This work was funded by the Deutsche Forschungsgemeinschaft (DFG, German Research Foundation) – Project-ID 232722074 – SFB 1102.
Conflict of Interest
The authors declare no conflict of interest.