The scientific community has become increasingly interested in the overall quality of diets rather than in single food-based or single nutrient-based approaches to examine diet–disease relationships( Reference Hu 1 ). Many different indices of healthy eating have been developed over the last three decades; most of these have been developed to reflect adherence to the national dietary guidelines and diversity of healthy choices within core food groups( Reference Wirt and Collins 2 ). These indices are used to examine diets for several attributes concurrently; therefore, they are able to provide a measure of overall diet quality, which is not possible when only single nutrients or foods are examined( Reference Arvaniti and Panagiotakos 3 ). Although every model of healthy eating has its own advantages and limitations, the general purpose of an index is to synthesise a large amount of dietary information as a single indicator useful for assessing putative risk factor–disease relationships( Reference Patterson, Haines and Popkin 4 ).
Numerous indices have been developed based on the American Dietary Guidelines( Reference Kennedy, Ohls and Carlson 5 – Reference Guenther, Reedy and Krebs-Smith 9 ), while others have been developed with some adaptation to reflect the dietary guidelines of other countries in Europe( Reference Alkerwi, Sauvageot and Nau 10 – Reference Estaquio, Kesse-Guyot and Deschamps 12 ), Canada( Reference Shatenstein, Nadon and Godin 13 ) and Australia( Reference McNaughton, Ball and Crawford 14 ). Some indices have been developed around specific dietary patterns, such as the Mediterranean diet( Reference Trichopoulou, Costacou and Bamia 15 ) and the Dietary Approaches to Stop Hypertension (DASH) diet( Reference Fung, Chiuve and McCullough 16 ), while an international diet quality index has been developed for a cross-national comparison of diet quality( Reference Kim, Haines and Siega-Riz 17 ) and an index has been developed based on the available literature on various dietary components and inflammatory indices( Reference Shivappa, Steck and Hurley 18 ).
Despite the plethora of indices used to measure diet quality, there still exist questions as to which of these can best predict health outcomes. Several systematic reviews on the differences in existing diet quality indices and their limitations have been published previously( Reference Wirt and Collins 2 , Reference Arvaniti and Panagiotakos 3 , Reference Fransen and Ocke 19 – Reference Kant 21 ), suggesting the need to assess the effectiveness of these tools in predicting health status. The construct validity of an index depends on the extent to which it is able to distinguish between individuals on certain relevant health-related intermediate markers, its association with health outcomes, and its capacity to predict the risk of chronic diseases or mortality( Reference Alkerwi 22 ). Although several attempts have been made to validate individual dietary indicators( Reference Coulston 23 , Reference Hann, Rock and King 24 ), very few studies have compared existing diet quality indices with regard to the biomarkers of diet and disease( Reference Kant and Graubard 25 , Reference Lassale, Fezeu and Andreeva 26 ) and most of these have focused on the Mediterranean Diet Score (MDS)( Reference Bach-Faig, Geleva and Carrasco 27 , Reference Fung, Hu and Wu 28 ).
The objective of the present study was to compare the ability of five different diet quality indices, namely the Recommendation Compliance Index (RCI), Diet Quality Index-International (DQI-I), DASH score, MDS and Dietary Inflammatory Index (DII), to detect changes in chronic disease risk biomarkers. These diet quality indices were selected according to the fundamental diversity of how they were conceived and built, including their evidence-based scoring algorithms, attributes and adaptability to widespread (if not universal) application. They were developed by different groups of researchers and in diverse settings (Northern European, North American and Mediterranean populations). A deeper understanding of how well these diet quality indices relate to a set of risk markers could improve the success of public health messages and fulfil the demand of many nutrition researchers for a suitable measure of diet quality. As this is exploratory research, we did not advance a hypothesis of any index preference.
Materials and methods
Study population
The study population consisted of 1352 participants of the ORISCAV-LUX (Observation of Cardiovascular Risk Factors in Luxembourg) study, a nationwide cross-sectional population-based survey of cardiovascular risk factors in Luxembourg. The sample size was based on a theoretically expected participation rate of 32·2 %( Reference Alkerwi, Sauvageot and Couffignal 29 ). The data collection procedures, sample design and representativeness of the ORISCAV-LUX study have been described in detail elsewhere( Reference Alkerwi, Sauvageot and Couffignal 29 , Reference Alkerwi, Sauvageot and Donneau 30 ). In short, the stratified random sample was identified from the national insurance registry of non-institutionalised individuals aged 18–69 years. The participants were recruited between November 2007 and January 2009 following an invitation letter and phone contact inviting prospective participants to visit the study centre. Trained research staff provided the participants with detailed instructions on how to complete the self-administered questionnaire, assisted them in completing the dietary information, and then checked the correctness of responses.
Dietary intake was assessed by means of a validated 134-item semi-quantitative FFQ( Reference Sauvageot, Alkerwi and Albert 31 , Reference Sauvageot, Alkerwi and Adelin 32 ). Food intakes were calculated by multiplying the self-reported food portion by the frequency of consumption. Energy and nutrient intake data were compiled using the French Composition Table( Reference Hercberg 33 ). Information regarding demographic, socio-economic and lifestyle characteristics was collected as well as direct blood pressure (BP) and anthropometric measurements were taken. For the present study, data from 1352 participants were available after eliminating those with missing data on dietary habits.
The ORISCAV-LUX study was conducted according to the guidelines laid down in the Declaration of Helsinki. All procedures involving human subjects were approved by the National Research Ethics Committee and the National Commission for Private Data Protection. Written informed consent was obtained from all participants.
Diet quality indices
FFQ-based dietary intakes were used to calculate adherence to the five diet quality indices. The calculation of these indices is summarised in online Appendix 1a–e; however, detailed information can be found in the respective publications pertaining to each index. Briefly, the RCI( Reference Alkerwi, Sauvageot and Nau 10 ) has been developed previously to measure the degree of adherence to Luxembourg national dietary recommendations, which are consistent with the key prevailing European and international dietary guidelines. It is a food- and nutrient-based composite of thirteen components and ranges between − 0·5 (due to a negative half point for excessive salt intake) and 14 (2 points for more daily fruit and vegetable servings) points, where a higher degree of adherence results in higher scores.
To include a more comprehensive tool describing and comparing the diversity of consumption between countries, the DQI-I suggested by Kim et al. ( Reference Kim, Haines and Siega-Riz 17 ), which is based on the North American dietary guidelines, was selected. This index focuses on four major components: variety; adequacy; moderation; overall balance. Similarly, it incorporates both nutrients and foods from the assessed diet. The total DQI-I score ranges from 0 to 100, where a score of ‘0’ reflects a poorer-quality diet and a score of ‘100’ reflects a high-quality diet. In the present study, meat and poultry were considered as one group to calculate the within-group variety component, as it was not possible to distinguish the intake according to the FFQ.
As a clinical profile-targeted index, the DASH score was calculated as originally designed by Fung et al. ( Reference Fung, Chiuve and McCullough 16 ) for BP reduction. Based on both foods and nutrients, the DASH score focuses on eight beneficial and detrimental items of the diet. For each of the items, the participants were classified into quintiles according to their intake ranking. Component scores for beneficial items (vegetables, fruits and nuts, legumes, low-fat dairy products and whole grains) were assigned a value of 1 for quintile 1 and a value of 5 for quintile 5. Reverse scores were assigned for detrimental items (Na, red and processed meats, and sweetened beverages). The component scores were summed to obtain an overall DASH score for each participant ranging from 8 to 40 points.
In contrast to other indices, a region-specific diet quality index, the modified version of the MDS, as described by Trichopoulou et al. ( Reference Trichopoulou, Costacou and Bamia 15 ), was chosen to measure the degree of adherence to the traditional Mediterranean diet. It is characterised by nine food and nutrient components: high consumption of vegetables, fruits and nuts, pulses, cereals, and fish; high ratio of monounsaturated fat:saturated fat; low consumption of meat and dairy products; moderate consumption of alcohol. For beneficial components (vegetables, legumes, fruits and nuts, cereal and fish), consumption above the median was assigned a value of 1; otherwise a value of 0 was assigned. For components presumed to be detrimental (meat, poultry and dairy products), consumption equal to or above the median was assigned a value of 0; otherwise a value of 1 was assigned. For alcohol consumption, a value of 1 was assigned if the intake was between 10 and 50 g/d for men and a value of 1 was assigned if the intake was between 5 and 25 g/d for women. The total MDS ranged from 0 (minimal adherence to the traditional Mediterranean diet) to 9 (maximal adherence).
As a novel non-recommendation-based index selected for comparison, the DII focuses on the inflammatory properties of the diet. It is a literature-based index, involving the review of nearly 2000 articles published through 2010 on the effect of forty-five food parameters on six inflammatory biomarkers (IL-1β, IL-4, IL-6, IL-10, TNF-α and C-reactive protein). It is scored according to whether the dietary parameter increased (+1), decreased ( − 1) or had no (0) effect on each of the six inflammatory biomarkers( Reference Shivappa, Steck and Hurley 18 ). In contrast to the other diet quality indices, higher values of the DII indicate a pro-inflammatory (i.e. less healthy) dietary profile, whereas lower values indicate an anti-inflammatory (i.e. more healthy) dietary profile.
Assessment of risk markers
Several anthropometric, clinical and plasma markers were examined, including glycaemic biomarkers (plasma glucose, serum insulin and glycated Hb (HbA1c) and homeostasis model assessment of insulin resistance (HOMA-IR)); lipid biomarkers (total cholesterol, LDL-cholesterol, HDL-cholesterol, TAG, apo A1 and apo B); cardiometabolic markers (BMI, waist circumference, systolic BP, diastolic blood pressure (DBP) and high-sensitivity serum C-reactive protein); hepatic function biomarkers (serum γ-glutamyl-transpeptidase (γ-GT), serum glutamate oxaloacetate transaminase (GOT) and serum glutamate-pyruvate transaminase (GPT)); renal function biomarkers (creatinine and uric acid); and anaemic biomarkers (Hb and haematocrit).
BMI was calculated as weight in kg divided by height in m2 (kg/mReference Wirt and Collins 2 ). BP was measured using an Omron® MX3 Plus automated oscillometric blood pressure monitor (O-HEM-742-E). Measurements were taken at least three times with the participants in a seated position and with a minimum interval of 5 min between each measurement. The average of the last two readings was used in the analysis. HOMA-IR was calculated as follows:

All the biomarkers were measured according to ORISCAV-LUX standardised protocols and have been described in detail in previous publications( Reference Alkerwi, Sauvageot and Nau 10 , Reference Alkerwi, Sauvageot and Donneau 30 , Reference Alkerwi, Donneau and Sauvageot 34 ).
Assessment of other variables
Self-reported time spent engaging in both moderate and intense physical activity was obtained from the International Physical Activity Questionnaire( Reference Hagstromer, Oja and Sjostrom 35 ), from which physical activity in metabolic equivalents-h/week was calculated. Detailed information regarding smoking status was obtained from the health questionnaire, and each participant was classified as a smoker or a non-smoker.
Statistical analyses
For descriptive purposes, the five diet quality indices were divided into quartiles (Q). The Q1 and Q4 of each index are reported to compare the demographic, socio-economic and lifestyle characteristics of the participants. The number and percentage of participants are reported for categorical variables and means and standard errors are reported for continuous symmetrically distributed variables; otherwise medians and interquartile ranges are reported. The P values were computed from the χ2 test, one-way ANOVA and Kruskal–Wallis test.
The bivariate Spearman correlation method was used to assess the inter-correlation between the diet quality indices and their correlations with several micronutrients and vitamins, as this method is not influenced by the outlying values and results are therefore more robust in meeting normality assumptions.
As the diet quality indices have disparate scoring systems, raw scores of each diet quality index were standardised into z-scores, to enable meaningful cross-index comparisons.
To assess the independent linear associations between each diet quality index and risk biomarkers, multiple linear regression analyses were conducted to calculate β-regression coefficients for each 1 − z score difference across the diet quality indices. Each diet quality index (explanatory variable) was modelled to examine the independent associations with different biomarkers (outcome), after adjustment for a number of potential covariates: age; sex; education level (primary, secondary or tertiary), smoking status (current, former or non-smoker); physical activity (metabolic equivalents-h/week); total energy intake (kcal/d). As total energy intake is included in the construction of the DII, this variable was not included in the respective analyses. These covariates were identified as major known determinants of health based on the literature. As several of the outcomes were not normally distributed, including BMI, systolic BP, C-reactive protein, TAG, γ-GT, GOT, GPT, all glycaemic biomarkers, and lipid biomarkers, except total cholesterol, generalised linear models with a γ-distribution and logarithm as the link function were used. Otherwise ANCOVA models were fit with special attention being paid to the homogeneity of variance and covariance assumptions.
Of the initial sample of 1352 participants, those who reported dieting for weight loss were excluded (n 199) from all the multivariable models. Participants taking antidiabetic (n 43), hypotensive (n 187) and lipid-lowering (n 128) medications were excluded from the following models, respectively: glucose; systolic BP and DBP; lipid biomarkers. Non-fasting participants (n 26) were excluded from the glycaemic and lipid biomarker models. Hence, the final sample size used in the multivariable analyses varied between 1007 and 1153 participants.
Several sensitivity analyses were conducted to establish the robustness of the study results. First, the interaction terms for age × diet quality scores and sex × diet quality scores were tested. Analyses stratified by sex (male and female) and age group ( < 50 and ≥ 50 years) were conducted (results summarised below). Second, controlling for self-reported health awareness on the importance of eating a balanced diet did not change the findings. Similarly, results were similar when participants taking medications and dieting for weight loss were included in the analyses.
The results were considered significant at the 5 % critical level (P< 0·05, two-sided). All statistical analyses were conducted using PASW© for Windows© version 21.0 software (formerly SPSS Statistics, Inc.).
Results
Characteristics of participants according to diet quality indices
Table 1 summarises the characteristics of participants according to the quartile of each diet quality index, where Q1 reflects the lowest adherence and Q4 reflects the highest adherence to each specific dietary pattern, except for the DII, for which a reverse pattern exists (i.e. it ranges from a more anti-inflammatory (Q1) profile to a more pro-inflammatory profile (Q4)). Participants with better diet quality scores on all the indices were significantly older. A significant sex difference was observed for the DQI-I and DASH patterns, with women having higher scores than men. Globally, the five diet quality indices varied remarkably with regard to lifestyle behaviours. Participants with better diet quality scores were less likely to smoke and engaged in more physical activity, with the exception of the DQI-I. Across the five diet quality indices, participants exhibiting higher adherence tended to be more aware of the importance of balanced meals.
Table 1 Characteristics of the participants by quartiles† (Q) of five different diet quality indices, ORISCAV-LUX (Observation of Cardiovascular Risk Factors in Luxembourg) survey, 2007–8 (Number and percentage of participants; mean values and standard deviations; median values and interquartile ranges (IQR))
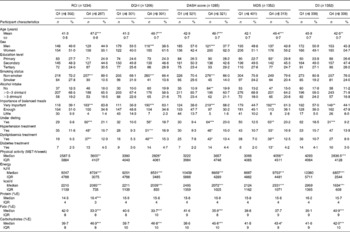
RCI, Recommendation Compliance Index; DQI-I, Diet Quality Index-International; DASH, Dietary Approaches to Stop Hypertension; MDS, Mediterranean Diet Score; DII, Dietary Inflammatory Index; MET, metabolic equivalents; %E, percentage of total daily energy intake.
Values were significantly different from that for Q1: * P< 0·05, ** P< 0·01, *** P< 0·001 (χ2 test for categorical variables and one-way ANOVA for continuous normally distributed variables; otherwise from the Kruskal–Wallis test).
† Only Q1 and Q4 of each diet quality index are given in the table.
‡ Difference in the number of cases is related to missing values for several variables.
Participants with higher diet quality scores reported lower total energy intakes, except for the Mediterranean dietary pattern. With increasing scores, the percentage of total energy intake per d derived from fat decreased, but that derived from carbohydrates increased (with the exception of the DII). Energy intake derived from protein did not differ considerably according to dietary pattern.
Correlations between diet quality indices and micronutrients
The correlations of the five diet quality indices with one another and with micronutrients are given in Table 2. The five diet quality indices were significantly correlated with one another (ρ values ranged between |0·28| and |0·65| and all P< 0·0001). As expected, negative correlations were observed between the DII and the other four indices due to the reverse DII scoring. All the indices were strongly correlated with the intakes of the three types of fatty acids and to the intakes of most of the vitamins and minerals, with the strongest correlations being observed between nutrient intakes and the DII.
Table 2 Spearman's correlation coefficients (r) among the diet quality indices and essential macronutrients and micronutrients, ORISCAV-LUX (Observation of Cardiovascular Risk Factors in Luxembourg) study, 2007–8
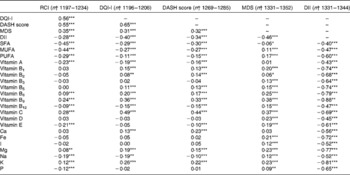
RCI, Recommendation Compliance Index; DQI-I, Diet Quality Index-International; DASH, Dietary Approaches to Stop Hypertension; MDS, Mediterranean Diet Score; DII, Dietary Inflammatory Index.
Values were significantly different: * P< 0·05, ** P< 0·01, *** P< 0·001.
† Difference in the number of cases is related to missing values for several variables.
Associations between diet quality indices and risk biomarkers
Table 3 summarises the independent associations between the selected diet quality indices and risk biomarkers.
Table 3 Multivariable linear regression of the associations between diet quality indices and risk markers, ORISCAV-LUX (Observation of Cardiovascular Risk Factors in Luxembourg) study, 2007–8† (β-Coefficients and P values)
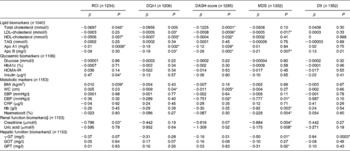
RCI, Recommendation Compliance Index; DQI-I, Diet Quality Index-International; DASH, Dietary Approaches to Stop Hypertension; MDS, Mediterranean Diet Score; DII, Dietary Inflammatory Index; HbA1c, glycated Hb; HOMA-IR, index of insulin resistance and sensitivity calculated according to homeostasis model assessment; WC, waist circumference; SBP, systolic blood pressure; DBP, diastolic blood pressure; CRP, C-reactive protein; γ-GT, γ-glutamyl-transpeptidase; GOT, glutamate oxaloacetate transaminase; GPT, glutamate-pyruvate transaminase.
* P< 0·05.
† Non-fasting participants were excluded from the glycaemic and lipid biomarker models. Participants using antidiabetic, hypotensive and lipid-lowering medications were also excluded from the following outcome models, respectively: glucose; SBP and DBP; lipid biomarkers. Participants who were dieting for weigh loss were excluded from all the models. All the models (RCI, DQI-I, DASH and MDS) were adjusted for age (continuous), sex, education level (primary, secondary or tertiary), smoking status (smoker or non-smoker), physical activity in metabolic equivalents-h/week, and total daily energy intake (kJ/d). The DII models were adjusted for the same covariates except total energy intake.
‡ n 1007 participants, after exclusion of those using hypotensive medications.
§ The models were additionally controlled for BMI as a continuous variable.
∥ The models were additionally controlled for alcohol intake (none, < 0–3 and < 3 drinks/d).
Lipid biomarkers
The DQI-I and DASH scores exhibited a very similar pattern of significant inverse associations with lipid biomarkers. Both were inversely associated with total cholesterol, LDL-cholesterol, HDL-cholesterol, apo A1 and apo B (all P< 0·05). The RCI was inversely associated with total cholesterol, HDL-cholesterol and apo A1. The MDS was inversely associated with LDL-cholesterol and apo B.
Glycaemic biomarkers
Of the five selected diet quality indices, none exhibited an association with the glycaemic risk biomarkers after multivariate adjustment, with the exception of the RCI, which exhibited a positive association with insulin (P< 0·05).
Metabolic markers
With increasing DQI-I and DASH scores, there was a significant linear decrease in central obesity as measured by waist circumference (β = − 0·009, P= 0·036 and β = − 0·011, P= 0·005, respectively). The DASH score and MDS were inversely associated with DBP (β = − 0·751, P= 0·019 and β = − 0·777 and P= 0·014, respectively). The MDS exhibited inverse associations with anaemic biomarkers. Participants with higher MDS had significantly lower levels of Hb and haematocrit.
Renal and hepatic function biomarkers
Concerning hepatic function as measured by liver enzymes (serum GOT, serum GPT and γ-GT), there were significant negative linear trends with the MDS, after additional statistical controlling for alcohol intake. Similarly, the Mediterranean dietary pattern was associated with significant linear decreases in creatinine and uric acid levels, irrespective of BMI. The RCI was inversely associated with creatinine levels.
Stratification analyses
Sex-specific stratification analyses showed that the observed associations of lipid biomarkers with the DQI-I and DASH scores were more significant in men than in women. The DII was inversely associated with plasma glucose, HbA1c, serum insulin and HOMA-IR in men aged >50 years. The DQI-I was also inversely associated with plasma glucose and HbA1c in only women (data not shown).
Discussion
Nutrition scientists have applied the diet quality concept to investigate the association between foods and nutrients and chronic disease incidence and mortality, based on prevailing hypotheses about the role of dietary factors in disease prevention( Reference Kant 36 ). In the present nationwide cross-sectional study, the performance of five diet quality indices, based on their ability to detect significant independent variations in a range of chronic disease risk markers, was compared. Among the five selected diet quality indices, the MDS exhibited the strongest associations with numerous risk markers. Higher adherence to the Mediterranean dietary pattern was significantly associated with lower levels of LDL-cholesterol, apo B, DBP, traditional indicators of renal function (creatinine and uric acid) and liver enzymes (serum γ-GT and GPT), irrespective of age, sex, education level, smoking status, physical activity and daily energy intake.
Consistent with our findings, the health benefits of following a Mediterranean dietary pattern have been widely reported, in terms of increased survival( Reference Trichopoulou, Costacou and Bamia 15 ) and decreased risk of CVD( Reference Martinez-Gonzalez and Sanchez-Villegas 37 ), diabetes( Reference Martinez-Gonzalez, de la Fuente-Arrillaga and Nunez-Cordoba 38 ), obesity and inflammation( Reference Sofi, Cesari and Abbate 39 – Reference Owen, Giacosa and Hull 41 ). The advantage of the MDS is probably related to the expedient scoring method (i.e. points awarded to a sufficient number of beneficial components) and better fit to our data by capturing dietary variation related to cardiometabolic risk. In addition, the MDS is characterised by specific food and nutrient composition. High consumption of olive oil and nuts is a key contributor of the healthy aspects attributed to the Mediterranean diet( Reference Tur, Romaguera and Pons 42 ). This vegetable-based oil contains a high proportion of oleic acid (a MUFA, vitamin E, and antioxidant phenolic compounds( Reference Owen, Giacosa and Hull 43 ), which have been linked to a reduced risk of CVD( Reference Manson, Gaziano and Jonas 44 )). Fish is an integral part of the Mediterranean diet, which has been reported to be inversely associated with CHD mortality( Reference Hu, Bronner and Willett 45 ). In addition, the Mediterranean dietary pattern is characterised by moderate consumption of meat (particularly red processed meat), which may potentially affect plasma creatinine levels( Reference Jacobsen, Christensen and Mogensen 46 , Reference Nair, O'Brien and Hayden 47 ). Serum creatinine and uric acid are simple measures and the most commonly used indicators of renal function in clinical practice. It must also be noted that the Mediterranean dietary pattern might be expected to fit another European population better than one in another part of the world where the ingredients would be scarce.
Both the DQI-I and DASH scores were inversely associated with LDL-cholesterol and total cholesterol and apo A1 and apo B. These associations with lipid biomarkers were compatible with the expectation, as dietary fat and SFA are common components in these diet quality scores. Kant & Graubard( Reference Kant and Graubard 25 ) have reported similar findings in comparing three dietary indices (Healthy Eating Index, Recommended Foods Score and Dietary Diversity Score for recommended foods) to predict cardiovascular risk. It is notable that the DQI-I and DASH scores were also inversely associated with HDL-cholesterol, which has also been observed in previous studies( Reference Fung, Chiuve and McCullough 16 , Reference Obarzanek, Sacks and Vollmer 48 ). The probable explanation is that these scores were based on dietary recommendations targeting lower dietary fat intakes, hence reduced HDL-cholesterol levels in addition to total cholesterol and LDL-cholesterol levels, notably in those with higher baseline HDL-cholesterol levels( Reference Obarzanek, Sacks and Vollmer 48 ). The observation that reduced-fat diets lower HDL levels has led to controversy over the advisability of following lower-fat, higher-carbohydrate diets( Reference Katan, Grundy and Willett 49 ).
Predictably, both the DQI-I and DASH diets were associated with a lower waist circumference, a relevant measure of abdominal obesity, and with decreased DBP. Our findings are consistent with previous literature documenting greater improvements in hypertension, cholesterol levels and weight loss in subjects following a DASH dietary pattern( Reference Fung, Chiuve and McCullough 16 , Reference Fitzgerald, Chiuve and Buring 50 ).
No specific diet quality index was associated with glycaemic biomarkers in multivariable analyses controlling for age, sex and other potential confounders. The 20-year prospective Coronary Artery Risk Development in Young Adults (CARDIA) study also did not find any association between a diet consistent with the key American Dietary Guidelines and reduced risk of type 2 diabetes( Reference Zamora, Gordon-Larsen and He 51 ), suggesting that most of the individual American Dietary Guideline recommendations have not been proven to reduce the risk of diabetes. Several diet quality scores, including MDS and DASH score, have been reported to be associated with a lower risk of type 2 diabetes in overweight subjects( Reference de Koning, Chiuve and Fung 52 ). In the present study population, age- and sex-specific stratification analyses showed that the DII was inversely associated with plasma glucose, HbA1c, serum insulin and HOMA-IR in men aged >50 years (data not shown). The DQI-I was also inversely associated with plasma glucose and HbA1c in only women. This sex-specific difference in cardiovascular risk prediction has been found in previous studies using different indices( Reference Lassale, Fezeu and Andreeva 26 , Reference McCullough, Feskanich and Stampfer 53 , Reference Nicklas, O'Neil and Fulgoni 54 ). The absence of a significant association of diet quality indices with glycaemic biomarkers is probably explained by the relatively low prevalence of diabetes in the study population( Reference Alkerwi, Sauvageot and Donneau 30 ); the small sample size did not allow to detect potentially significant changes in the risk of type 2 diabetes.
On the whole, the five diet quality indices were highly correlated in the study population, probably because most of these indices focus on dietary patterns rich in fruits and vegetables, whole grains, nuts and fish but poor in high-salt processed meats. The highest correlation was observed between the DASH and DQI-I scores, which confirms our findings with respect to the similarity in their ability to detect changes in lipid biomarkers. The inverse correlations between the DII and other diet quality indices are probably due to the fundamental difference in the conceptual background to construct this diet quality index, focusing on the inflammatory profile of the diet. Although these indices, in general, share the same beneficial and detrimental food or nutrient components, the observed variations in relation to biomarkers associated with disease risk are probably due to differences in their constructs and scoring criteria.
The RCI, DQI-I and DASH scores showed no direct correlations with important micronutrients such as vitamin D, Fe and I. This suggests that adherence to the respective dietary guidelines would be insufficient for preventing vitamin and mineral deficiencies, although other factors may influence the absorption; for example, vitamin D is not solely a food-based nutrient. Furthermore, it is possible that the poor correlations arise from the inability of the FFQ to precisely assess the intake of these nutrients.
By contrast, the DII was strongly negatively correlated with virtually all the nutrients. Although the MDS was significantly correlated with daily Fe intake, finding low serum Fe and haematocrit levels among participants matching those observed on following a Mediterranean dietary pattern should be examined in other populations to determine whether the diet is really of poor Fe quality or whether further modifications and adaptations are required to be made in the MDS scoring system.
Surprisingly, the Luxembourg diet quality index measuring the compliance to the national dietary recommendations (RCI) selected in the present study was associated with higher levels of serum insulin and BMI, but with lower levels of HDL and apo A1. The possibility of reverse causality, i.e. obese individuals and subjects with poor health status may have improved their dietary habits after being informed of a medical condition, is minimal, because these correlations were observed in a population sample relatively free from chronic diseases. Furthermore, participants taking medications or dieting for weight loss were excluded. In addition, further controlling for health awareness as expressed by the importance of eating a balanced diet did not change the results (data not shown). However, a bidirectional causal relationship between diet and health is possible, as diet quality has a positive effect on health and health influences diet quality and food choices. These unexpected findings indicate that adherence to the current national dietary guidelines may be insufficient to avoid cardiometabolic risk and disease.
Although the RCI helped to identify the major determinants of good compliance( Reference Alkerwi, Sauvageot and Nau 10 ), it is probably a poor indicator of risk prediction and not the ideal tool to reflect a good-quality healthy diet. The lack of consistent associations with risk biomarkers may be explained by the non-specificity of the type of protein and carbohydrate. The RCI emphasises a broad inclusion of many foods but not of micronutrients. Apart from population adherence to the national dietary guidelines, its ability to predict diet-related risk is questionable, and future modification and improvement of this indicator are required. In our national context, it is important to validate a summary nutritional indicator to serve as a useful tool for rapid screening, thereby aiding public health authorities to develop effective nutrition promotion messages to the general public( Reference Kennedy, Ohls and Carlson 5 , Reference McCabe-Sellers, Bowman and Stuff 55 ).
Similar to most of the nutrition population-based studies, potential limitations include factors related to the cross-sectional design, which precludes inferences regarding causal relationships. In addition, an optimum dietary intake assessment strategy is still challenging in nutrition research( Reference Kristal, Peters and Potter 56 ). The list of foods in a FFQ is a crucial element needed to capture the variability of dietary habits. The calculation of the selected diet quality indices relied on the 132-item FFQ. This tool has been shown to be sufficiently convenient and inexpensive to use in large-scale, population-based studies( Reference Schatzkin, Kipnis and Carroll 57 ), compared with a high-cost 24 h recall, which must be repeated to avoid under-reporting( Reference Ma, Olendzki and Pagoto 58 ), although both methods rely on cognitive processes such as memory and perception. It is worth mentioning that most of the selected indices were developed using the FFQ, except the DQI-I, which was originally designed using 24 h recalls. The short-term dietary intake assessment method was considered a weak point because food variety among the categories included in the DQI-I would have been prone to a misclassification error( Reference Kim, Haines and Siega-Riz 17 ). The ability of the DII to predict inflammation-related outcomes has been demonstrated using both recall methods and structured questionnaires( Reference Shivappa, Steck and Hurley 59 ); however, the FFQ used in the present study seemed to be not ideal, as the unavailability of specific foods and nutrients probably contributed to the poor performance of the DII in the present study and, hence, hampered the detection of significant associations with risk biomarkers.
The present study has several strong points. The data were derived from a recent nationwide sample of the general adult population. This is one of the few population-based studies that have directly compared alternative diet quality measures to determine whether one index can best predict the risk of chronic diseases. Previous attempts have been limited to comparison of different Mediterranean or American recommendation dietary scores( Reference McCullough, Feskanich and Stampfer 53 ) with respect to specific health outcomes( Reference Lassale, Fezeu and Andreeva 26 , Reference de Koning, Chiuve and Fung 52 ). Applying these scores, developed in different settings, to a single European population is problematic, as it could be inadequate to assess the quality of the diet of one population based on the recommendations developed for another, especially when their eating habits differ substantially( Reference Dubois, Girard and Bergeron 60 ). The DII was empirically derived from a search of the literature that involved reading and scoring nearly 2000 articles that dealt with the relationship between dietary parameters and six inflammatory markers. The remaining three indices are based on dietary recommendations that, while generally sensible, are also culture bound. Nonetheless, the observed associations of each dietary pattern with various markers of chronic diseases provide evidence of their reproducibility and validity to a different extent.
In summary, the present study provides evidence that in the study population, the Mediterranean diet was associated with the most favourable biomarker risk profile, which supports the epidemiological application of the MDS in the future to evaluate diet–disease risk relationships. The Mediterranean diet serves potentially as a universal guideline for healthful food consumption, as it presents a healthy eating pattern associated with reduced levels of cardiometabolic, hepatic and renal biomarkers in an adult population. Considering the high multiple risk profile of the study population( Reference Alkerwi, Sauvageot and Donneau 30 ), our findings provide insights to determine whether the current dietary guidelines are truly helpful in the fight against chronic diseases.
Supplementary material
To view supplementary material for this article, please visit http://dx.doi.org/10.1017/S0007114514003456
Acknowledgements
A. A. was supported by a grant from the FNR (Fond National de Recherche) for the project (DIQUA-LUX, 5870404), Luxembourg. J. R. H. was supported by an Established Investigator Award in Cancer Prevention and Control from the Cancer Training Branch of the US National Cancer Institute (K05 CA136975). These funders had no role in the design and analysis of the study or in the writing of this article or in the interpretation of the results.
The authors' contributions are as follows: A. A. was involved in the conception and design of the ORISCAV-LUX survey, coordinated field data collection, conceived the present study, contributed to the statistical analyses and drafted the first version of the manuscript; C. V. performed the data analyses and contributed to the statistical analyses and data interpretation; G. E. C., N. Sauvageot and N. Shivappa contributed to the calculation of the diet quality indices and to the critical revision of the manuscript; J. R. H. provided expertise and oversight throughout the study. All authors approved the final version of the manuscript.
None of the authors has any conflicts of interest to declare.