In 2016, nearly 18 million deaths were caused by CVD, accounting for one-third of the global deaths. In the last decade, the impact of the increasing prevalence of non-communicable diseases has been observed in low- and middle-income countries, where approximately 75 % of the global deaths related to CVD occurred(1, 2). Hypertension (HT) and type 2 diabetes mellitus (T2DM) are the strongest modifiable risk factors for CVD and the subsequent mortality(3). HT is the main risk factor for CVD(Reference Roth, Nguyen and Forouzanfar4), which accounts for more than two-thirds of the total deaths associated with non-communicable diseases. On the other hand, $US 827 billion are expended annually in patients with T2DM worldwide(Reference Seuring, Archangelidi and Suhrcke5).
A common major risk factor for CVD is obesity, with a global prevalence among adults of 13 % in 2016(6). The WHO defines obesity as an excess of body fat and recommends using BMI to assess it, as BMI is a simple tool to use in routine clinical examinations(Reference Green and Woodson7). However, BMI does not differentiate fat percentage from lean mass. Therefore, the obesity diagnosis obtained by it is not necessarily correlated with body fat percentage (BF%), which is associated with CVD or metabolic disturbances(Reference Su, Amiri and Mohd Hairi8, Reference Herrera, Casas and Miranda9). Waist circumference (WC) is another anthropometric marker with a demonstrated association with T2DM and HT and represents the abdominal fat and android distribution of fat(Reference Walker, Marzullo and Ricotti10). The gold standards to quantify BF% as well as describe its distribution are dual-energy X-ray absorptiometry and skinfold thickness assessment(Reference Heymsfield, Martin-Nguyen and Fong11). Skinfold thickness, being one of the recommended standards for excess of BF %, remains understudied in its relationship with major cardiometabolic risk factors such as T2DM and HT. In terms of longitudinal evaluations, only two studies from developing countries have explored the association between skinfold thickness results and developing T2DM, and only one of these considered HT as an additional outcome(Reference Mensink, Feskens and Kruijshoop12, Reference Chei, Iso and Yamagishi13).
Accordingly, we aimed to determine the association between excess of body fat, estimated by skinfold thickness using four different regions (i.e. bicipital, tricipital, subscapular, suprailiac), and the risk of developing T2DM and HT using information of the PERU MIGRANT Cohort Study. We hypothesized that excess of BF % according to skinfold thickness would demonstrate a greater association with T2DM and HT than that observed with traditional anthropometric markers.
Methods
Study design
We analysed data from the PERU MIGRANT Study, a prospective ongoing cohort for which baseline assessment was conducted in 2007–2008. Two follow-ups were carried out, the first one in 2012–2013 and the other during 2015–2016(Reference Miranda, Gilman and García14, Reference Carrillo-Larco, Ruiz-Alejos and Bernabé-Ortiz15).
Settings and participants
At baseline, individuals aged ≥30 years who reported living permanently in the selected rural and urban areas were invited to participate in the study after a single-stage random sampling method. Rural dwellers were recruited from the district of San Jose de Secce in Huanta, Ayacucho. Rural-to-urban migrants and urban dwellers were selected from Las Pampas de San Juan de Miraflores, Lima. Participants unable to consent and pregnant women were excluded(Reference Miranda, Gilman and García14).
Enrolment at baseline was made through a single-stage random sampling technique. In San Jose de Secce, the rural site, a census was conducted in 2007. In the case of Lima, the urban area, a local census was updated in that year. Those who were born and lived permanently in Las Pampas de San Juan de Miraflores, Lima, were included in the urban group, whereas those who reported to permanently live in Las Pampas de San Juan de Miraflores at the time of the baseline assessment but were born in Ayacucho were considered in the migrant group. A face-to-face administered questionnaire as well as clinical and laboratory assessments were conducted to obtain data related to CVD risk profile and sociodemographic characteristics. Only those with completed information were included in the cohort baseline, resulting in an overall response rate of 61·6 % (989/1606)(Reference Miranda, Gilman and García14). Participants were re-evaluated during follow-up assessments by contacting them in the settings where they were originally enrolled in the study.
Definition of variables
Outcome variables
The two outcomes of interest were T2DM and HT. T2DM cases were defined as: a fasting glucose level ≥126 mg/dl obtained within 8 and 12 h of fasting, self-reported T2DM diagnosis made by a physician, or a current record that the participant is on antidiabetic medication. In the follow-up assessment, new cases of T2DM were considered only when the participant accomplished the first two diagnostic criteria we used at baseline. The laboratory method used for plasma glucose measurement was the enzymatic colorimetric (GOD-PAP, Modular P-E/Roche-Cobas, Grenzach-Whylen, Germany) for 5 ml of whole blood.
At baseline, all cases of HT were defined by: the average of the last two of three measurements of systolic blood pressure (≥140 mmHg) or diastolic blood pressure (≥90 mmHg) in the clinical assessment, the participant’s self-report of HT diagnosis done by a physician, or currently receiving antihypertensive medication. New cases of HT identified in the first and second follow-up were determined by using only the first two criteria, as the antihypertensive medication data did not provide more information.
Exposure variables
Excess body fat, measured only at baseline, was defined as the exposure variable according to each skinfold measurement. Four different areas of the body (i.e. bicipital, tricipital, subscapular, suprailiac) were measured in triplicate in millimetres and assessed by the same fieldworker using a Holtain Tanner/Whitehouse Skinfold Caliper calibrated to the nearest 0·2 mm. The average of the three measures for each skinfold was calculated and utilized for further estimations. The Durnin–Womersley density formula was used to estimate the body density according to each skinfold measurement as well as the overall fat obesity, defined as the total sum of all the four skinfolds(Reference Durnin and Womersley16). Then, the SIRI equation was used to calculate BF% for each skinfold parameter(Reference Martín Moreno, Gómez Gandoy and Antoranz González17). Sex-specific cut-off points (>25 % for males and 33 % for females) established by the Spanish Society for Obesity Studies were used(18). For comparison, obesity, defined by BMI (≥30 kg/m2), WC (cut-off point ≥80 cm for women and ≥90 cm for men), waist-to-hip ratio (WHR; cut-off point ≥0·85 for women and ≥0·95 for men) and waist-to-height ratio (WHtR; cut-off point ≥0·5), were considered as other exposure variables of interest(18, Reference Ashwell, Gunn and Gibson19). Anthropometric assessments were conducted following standardized procedures by trained fieldworkers.
Other variables
The following sociodemographic variables, assessed at baseline, were also included in the analysis as potential confounders: sex (female v. male), age (<50 v. ≥50 years), education level (non/some primary, complete primary, secondary or more), and socio-economic status based on a wealth index and split into tertiles. Additionally, non-healthy behavioural risk factors were also assessed. Physical activity was assessed through the International Physical Activity Questionnaire (IPAQ). Levels in MET-min/week were categorized into low and medium/high (where MET is metabolic equivalent of task). Heavy drinking was defined as either the self-report of having a hangover or having ≥6 drinks on the same occasion at least once per month. Finally, tobacco consumption was defined as current daily smoking.
Statistical analysis
The statistical software package Stata version 13 for Windows was used for analyses. Initially, the description of the study population by study groups (rural, rural-to-urban migrant and urban) defined at baseline was conducted. Means and sd, as well as proportions were calculated and presented according to the population subgroups. Comparisons were performed using the one-way ANOVA and χ 2 tests as required.
For the incidence analysis, we excluded those participants who were diagnosed with HT or T2DM at baseline accordingly. Incidence rates and associated 95 % CI were estimated in person-years by adding the follow-up times of re-contacted participants. However, for those with a new diagnosis of T2DM or HT, only half of the time between their baseline and follow-up assessment was used in this sum, since the actual date of diagnosis was unknown (actuarial method). Since T2DM and HT diagnosis status were not available for those dead and lost to follow-up, information of the last follow-up was used if possible.
To determine the associations of interest, Poisson regression models, using link log and robust se to account for the cluster effect, were utilized to report risk ratios and 95 % CI as the risk of death was assumed constant over time. Two regression models adjusted by different confounders were developed. Model 1 adjusted for sex, age, education, assets index, population group, heavy drinking, currently daily smoking and physical activity. Model 2 adjusted for everything in model 1 plus BMI. In addition, population attributable fraction (PAF) was also calculated using the ‘punaf’ command in Stata(Reference Laaksonen, Härkänen and Knekt20, Reference Flegal, Panagiotou and Graubard21) and compared between models.
Results
Characteristics of the study population at baseline
At the baseline enrolment, 988 participants completed the study. Overall age was 48·0 (sd 12·0) years and 47 % were male. Baseline prevalence of T2DM and HT was 4·1 and 16·1 %, respectively, being higher in the urban group (Table 1). The mean of all skinfold measurements, as well as the sum of them, was significantly higher in the both urban and migrant groups, compared with those who were rural dwellers (P < 0·001, Table 1).
Table 1 Characteristics of the sample at baseline by population group; PERU MIGRANT Cohort Study, 2007–2008

N, number of observations; n, frequency; WC, waist circumference; WHR, waist-to-hip ratio; WHtR, waist-to-height ratio; HT, hypertension; T2DM, type 2 diabetes mellitus.
Values are presented as N, n and % for categorical variables or N, mean and SD for continuous variables.
* The χ 2 test, t test and ANOVA were used to assess differences between groups according to the type of variable.
Skinfold measurements and incidence and risk of type 2 diabetes mellitus
A total of 106 (10·7 %) participants were lost-to-follow up, and fifty-seven (5·8 %) died. For the T2DM incidence, we excluded those who had T2DM diagnosis at baseline (n 40; see online supplementary material, Supplemental Fig. S1). The mean follow-up was 7·6 (sd 1·3) years, leading to 6068 person-years of follow-up. We identified sixty-one new T2DM cases, resulting in an overall incidence of 1·0 (95 % CI 0·8, 1·3) per 100 person-years. T2DM incidences according to the different obesity approaches are described in Table 2.
Table 2 Incidence and risk of type 2 diabetes mellitus according to obesity defined by skinfolds; PERU MIGRANT Cohort Study, 2007–2016
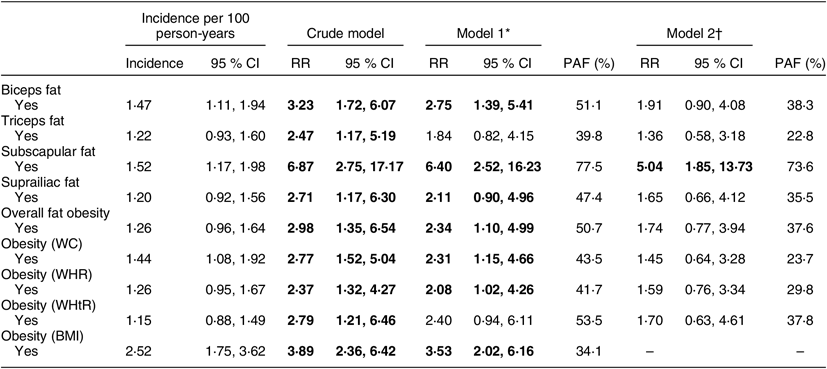
RR, risk ratio; PAF, population attributable fraction; WC, waist circumference; WHR, waist-to-hip ratio; WHtR, waist-to-height ratio.
Bold indicates significant results (P < 0·05) according to 95 % CI.
* Model 1 adjusted by age, sex, education, assets index, current daily smoking, heavy drinking, population group and physical activity level.
† Model 2 adjusted by age, sex, education, assets index, current daily smoking, heavy drinking, population group, physical activity level and BMI (in categories).
Overall fat obesity was associated with a 2·3-fold risk for T2DM. Bicipital and subscapular excess of BF% were the measurements strongly associated with higher risk of T2DM, with almost 3-fold and 6-fold risk, respectively. PAF due to subscapular fat was greater than for other common anthropometric measurements such as BMI, WC and WHtR (Table 2). After controlling for BMI, results showed a strong association between subscapular skinfold thickness and the risk of T2DM with a small variation in PAF.
Skinfold measurements and incidence and risk of hypertension
In the case of HT, we excluded 160 participants diagnosed at baseline (see online supplementary material, Supplemental Fig. S2). The mean follow-up was 6·5 (sd 2·5) years leading to 4850 person-years of follow-up. A total of 128 new cases of HT were identified, which resulted in an incidence of 2·6 (95 % CI 2·2, 3·1) per 100 person-years. HT incidences according to the different obesity approaches are described in Table 3.
Table 3 Incidence and risk of hypertension according to obesity defined by skinfolds; PERU MIGRANT Cohort Study, 2007–2016

RR, risk ratio; PAF, population attributable fraction; WC, waist circumference; WHR, waist-to-hip ratio; WHtR, waist-to-height ratio.
Bold indicates significant results (P < 0·05) according to 95 % CI.
* Model 1 adjusted by age, sex, education, assets index, current daily smoking, heavy drinking, population group and physical activity level.
† Model 2 adjusted by age, sex, education, assets index, current daily smoking, heavy drinking, population group, physical activity level and BMI (in categories).
Obesity-defined subscapular and suprailiac fat, as well as the sum of all measurements, were significantly associated with higher risk of developing HT. Although almost all of the obesity anthropometric markers were associated with the risk of developing HT, the sum of all skinfold measurements, suprailiac fat, WHtR and subscapular fat showed higher PAF (52·2, 47·7, 46·5 and 42·1 %, respectively; Table 3). After controlling for BMI, associations and their respective PAF were similar to those obtained by models without that adjustment.
Discussion
We found a strong association between BF%, assessed by skinfold thickness, and the risk of developing T2DM and HT even after adjusting for several confounders, including BMI. Obesity defined by BF% in the subscapular area was the strongest anthropometric marker associated with the risk of T2DM, whereas overall fat obesity was most greatly associated with developing HT.
Skinfold thickness measurements as predictor of type 2 diabetes mellitus
The impact of obesity, assessed by BMI, WC and WHtR, on the development of T2DM has been largely described in large cohort prospective studies(Reference Wang, Li and Xue22, Reference Bell, Kivimaki and Hamer23). However, there is still very limited prospective information assessing the association of skinfold thickness with incident T2DM. To our knowledge, there is no specific recommendation about the use of an anthropometric measurement as a marker for T2DM risk prediction besides BMI and WC(Reference Xiao, Liu and Sun24, Reference Taylor, Ebrahim and Ben-Shlomo25). Our findings provide evidence favouring the utility of measuring skinfolds as a laboratory-free marker for the risk of T2DM in non-specialist and low-resource settings.
Upper-body fat, known as truncal fat, has been associated with an increased insulin resistance among men(Reference Abate, Garg and Peshock26). Many markers of insulin secretion and its metabolism have been studied according to fat distribution. These studies have indicated that visceral and upper-body fat are more related to the release of NEFA and their accumulation in the liver, which subsequently leads to glucose metabolism impairment and even cardiovascular mortality(Reference Edelstein, Knowler and Bain27–Reference Jensen29). Few observational studies have approached the relationship between subscapular skinfold thickness and the risk of developing T2DM. Of these studies, only three were prospective in nature(Reference Mensink, Feskens and Kruijshoop12, Reference Chei, Iso and Yamagishi13, Reference Sosenko, Kato and Soto30). Mensik et al. reported that a greater subscapular skinfold thickness was more strongly associated with the risk of transient and persistent impaired glucose tolerance after 4 months of follow-up compared with BMI, WC and the sum of all skinfold measurements(Reference Mensink, Feskens and Kruijshoop12). However, Chei et al. questioned this association, as only a marginal significant risk for T2DM and HT was found(Reference Chei, Iso and Yamagishi13). Data regarding physical activity and sociodemographic characteristics were not available in this latter report, leaving room to better assess the association by including further confounders as we did herein. Sosenko et al. noted a strong association between subscapular skinfold thickness and non-insulin-dependent diabetes. Compared with BMI and WHtR, subscapular skinfold thickness was superiorly associated with T2DM, even after controlling for sex(Reference Sosenko, Kato and Soto30).
Some studies suggest that the relationship between insulin secretion and high-carbohydrate diets, which are particularly common in Peru and other Latin American countries(Reference Mayén, Marques-Vidal and Paccaud31), varies according to phenotype and body fat distribution. The positive association between abdominal obesity and T2DM is clear and seems to be stronger when other criteria of the metabolic syndrome (e.g. high cholesterol) are present(Reference Heianza, Kato and Kodama32–Reference Freemantle, Holmes and Hockey34). Moreover, in patients with a history of vascular disease, WC has a positive association with T2DM risk(Reference Wassink, van der Graaf and van Haeften35). A diet rich in carbohydrates would increase the abdominal perimeter, leading to high levels of inflammation markers related to T2DM, such as IL-6, complement factor C3 and C-reactive protein(Reference Hermsdorff, Zulet and Puchau36). In Asian populations, WC has been associated not only with T2DM but also with diabetic retinopathy(Reference Klein and Klein37). Among Hispanics, data are limited. Mexican populations had greater levels of insulin secretion after oral glucose intake unlike to white counterparts(Reference Haffner, D’Agostino and Saad38). Finally, truncal fat is linked to parental history of T2DM, which is also an established risk factor for T2DM(Reference Surendar, Indulekha and Deepa39). Overall, the assessment of body fat distribution could play an important role in T2DM screening and follow-up.
Skinfold thickness measurements as predictor of hypertension
Data regarding the association between skinfold thickness and HT are even more limited. To our knowledge, there is only one study addressing this association prospectively. The Kaunas Cardiovascular Cohort Risk Study conducted in Lithuania showed that an increased skinfold thickness measured in childhood was not associated with HT in adulthood, after 35 years of follow-up(Reference Martín, Dávila-Batista and Castilla40). There are chemical changes during adolescence that may explain why skinfold thickness assessed during childhood might not reflect final fat distribution in adulthood(Reference Lohman41). In addition, studies in twins have reported that BMI and skinfolds vary with age and sex independently of heritability, and that skinfold thickness measurements performed in childhood actually overestimate BF%(Reference Koziel, Nowak and Malina42). Our study focused only on adults and demonstrated the long-term effect of body fat distribution on blood pressure outcomes.
A few cross-sectional studies, mainly in children, have considered skinfold thickness measurements as exposure variables while assessing HT or blood pressure as outcomes. Khadilkar et al. found a relevant association between higher blood pressure and tricipital skinfold thickness in children aged 5–17 years(Reference Khadilkar, Mandlik and Chiplonkar43). Ying-Xiu et al. assessed upper-body fat as the sum of tricipital and subscapular skinfold thicknesses, obtaining similar results in 12-year-old children(Reference Ying-Xiu, Zhao-Xia and Ling44). Okosun et al. found that truncal obesity, abdominal obesity and their co-occurrence had higher odds of HT, especially in adult women(Reference Okosun, Boltri and Hepburn45). Our longitudinal study confirms these associations. A cross-sectional analysis in young Mexican women (mean age = 29·6 (sd 5·4) years) reported that the sum of all skinfold thicknesses was almost equally efficient in predicting HT compared with BMI and WC(Reference Neufeld, Jones-Smith and García46); however, in our prospective analysis, BMI had the weakest association and lowest PAF. In that sense, our results are physiologically compatible with previous studies where subscapular, suprailiac and tricipital skinfold thickness had associations with standard indicators of organ damage due to HT(Reference Selcuk, Bulucu and Kalafat47).
Our findings suggest that body fat located in the subscapular area is a risk factor for developing T2DM and HT. Thus, subscapular skinfold thickness measurement could be used as an individual potential tool or part of a risk score to identify individuals at high risk for developing T2DM and HT. In addition to BMI, WHtR and overall fat obesity could be also used as a screening tool for HT. In resource-limited settings, where laboratory analysis is not always included in the minimal health insurance package, these anthropometric parameters could provide a cost-effective and individualized approach identify and monitor patients at risk for developing either of these two conditions.
Regardless of the setting, inserting some skinfold parameters may improve the surveillance and screening of CVD risk factors by identifying individuals at high risk for developing T2DM and HT. The strong association and high PAF percentages from subscapular skinfold thickness support the utility of using this anthropometric marker as part of the general clinical evaluation in adults.
Strengths and limitations
The PERU MIGRANT Cohort Study has three well-defined sociodemographic groups, controlling our models by urbanization exposure as in previous studies(Reference Bernabe-Ortiz, Sanchez and Carrillo-Larco48, Reference Zheng, Lamoureux and Ikram49). In addition, our regression analysis allows us to pursue PAF of all the anthropometric measurements and compare them.
Nevertheless, our study remained with limitations that should be declared. First, potential misclassification of T2DM cases can arise because oral glucose tolerance was not used as diagnostic method(Reference Miranda, Gilman and García14). However, our main findings will not change since the under-diagnosis of T2DM would have a negative impact in the strength of the associations, which implies that risk of developing T2DM would be even greater. Second, selection bias might arise due to difference in baseline response rates among population groups, which was higher among rural dwellers(Reference Miranda, Gilman and García14). Third, skinfold thickness measurement has its own limitations such as variance among measurers and calliper types, ethnic phenotypes, etc. In order to minimize the effect of these variations, we standardized the skinfold thickness measurements at baseline. Nevertheless, we recognize potential additional bias due to variation of body fat during the observation period, which was not assessed periodically by skinfold thickness. Finally, data regarding diet were not collected, representing a potential but minimal bias because of its relationship with body fat distribution according to the intake of simple and complex carbohydrates and saturated fat, which typically accumulates in WC. Therefore, further studies are needed to address the impact of diet patterns.
Conclusions
There is evidence of a strong association between subscapular and overall fat obesity assessed through skinfold thickness and the risk of developing T2DM and HT. Moreover, after adjusting for obesity according to BMI, which is used the most in daily clinical practice, subscapular obesity remained as one of the best predictors for T2DM. We remark that BMI and WC, commonly used anthropometric measurements, had lower PAF, particularly for HT. Our findings suggest that skinfold thickness, if used as part of routine assessments, might improve the detection process of T2DM and HT in settings without clinical specialists or accessible laboratory support. Nevertheless, considering the limitations of the skinfold thickness measurements, implementation-type studies are needed in order to disentangle the impact of subscapular skinfold thickness measurement as part of the routine clinical examination in elderly or overweight patients.
Acknowledgements
Acknowledgements: The authors are in high debt to the participants from Ayacucho and Lima, who kindly agreed and completed their participation in the study. In addition, they want to recognize and thank the commitment and hard work of all the fieldworkers, especially Lilia Cabrera, Rosa Salirrosas and Marco Varela. Financial support: This study was funded by the National Heart, Lung, and Blood Institute, National Institutes of Health, through the GloCal Health Fellowship Program from the University of California Global Health Institute and partly under the contract number HHSN268200900033 C. A.R.-A. is supported by the GloCal Health Fellowship Program. A.B.-O. (103994/Z/14/Z), J.J.M. (205177/Z/16/Z) and L.S. (098504/Z/12/Z) are supported by the Wellcome Trust. R.M.C.-L. has received support by the Inter-American Institute for Global Change Research (IAI CRN3036). The funders had no role in the design, analysis or writing of this article. Conflict of interest: The authors declare neither competing nor conflicts of interests. Authorship: J.J.M., A.B.-O., L.S. and R.H.G. conceived the original idea of the PERU MIGRANT Cohort Study including the further studies obtained with the follow-ups. The authors were part of the design and supervision. A.R.-A. coordinated and supervised fieldwork activities during the second follow-up conducted between 2015 and 2016. A.B.-O., A.R.-A. and J.J.M. developed the idea of this manuscript. A.B.-O. led the statistical analysis supported by R.M.C.-L. and A.R.-A. A.R.-A. drafted the first version of the article with great input of A.B.-O., R.M.C.-L. and J.J.M. All authors participated in writing the manuscript, provided key intellectual content and gave their final approval of the version submitted for publication. Ethics of human subject participation: This study was conducted according to the guidelines laid down in the Declaration of Helsinki and all procedures involving human subjects were approved by the Ethics Committees of Universidad Peruana Cayetano Heredia (UPCH) in Peru and the London School of Hygiene and Tropical Medicine in the UK at baseline. Further follow-up assessments were approved by the Ethics Committee at UPCH. Written or verbal informed consent were obtained from all subjects. When verbal consent was obtained it was witnessed and formally recorded.
Supplementary material
To view supplementary material for this article, please visit https://doi.org/10.1017/S1368980019001307
Author ORCID
Antonio Bernabé-Ortiz, 0000-0002-6834-1376.