1. Introduction
Our society today is faced with significant challenges, one of which is the lack of effective communication through multiple languages. The challenge is further exacerbated by the outbreak of the Covid-19 pandemic that requires people to practice ‘social distancing’ and avoid ‘social interaction.’ Social distancing is fundamentally against human nature, and the prolonged practice has created not only economic hardships and cognitive disturbances, but also difficulties in language learning for both children and adults.Footnote 1 Thanks in large part to the pervasive use of digital technologies, we have dealt with some of the difficulties under the pandemic, from video conferencing to online teaching to virtual gathering. In the last decade, digital technologies have also developed alongside advances in artificial intelligence (AI) and big data analytics. These developments have changed human behavior in all aspects of our lives including how we learn a new language. Digital language learning (DLL) has emerged against this backdrop both as an educational practice and as a field of scientific study.
DLL can be used broadly to refer to digital technology-based or technology-enhanced language learning platforms or tools, or the practices of learning using such platforms or tools. In this paper, we use DLL in this broad sense to reflect the new developments through technology-driven methodologies, with second language (L2) learning as our focus. Although a DLL approach covers similar techniques encompassed by computer-assisted language learning (CALL), DLL focuses on more recent tools and platforms enabled by the latest developments in digital technologies such as mobile computing, virtual reality (from desktop 3D to augmented/mixed reality), and digital games, attempting to explore the potential of technologies for cultivating self-directed, exploratory, and autonomous learning.
Riding on the tide of rapidly developing digital technologies, L2 learners and teachers have delved into DLL and its applications. Indeed, DLL-based L2 platforms and tools have emerged so quickly in the past decade that we can no longer count them by our fingers. At the same time, however, it is unclear whether some of the commercial products (e.g., Babbel, Duolingo, Rosetta Stone) are always validated scientifically or empirically (see van Deusen-Scholl, Reference Van Deusen-Scholl2015 for discussion). It is also unclear how we might go about assessing these tools against each other and against their bold commercial claims about their effectiveness, when no randomized control studies could be performed (which is a problem with some reports out there, e.g., Vesselinov & Grego, Reference Vesselinov and Grego2012, Reference Vesselinov and Grego2016). Further, significant gaps exist between the DLL tools that the tech companies develop and the needs that learners and instructors have. It is clear, though, that the industry does not always have in mind learner-specific characteristics or the assessment of learning success, as will be discussed in this article. Technology developers are mostly interested in making their products available (and gaining profits), whereas educators are interested in using the technologies to enhance learning outcomes; unfortunately, these two do not always match. Such gaps are further complicated by the fact that even educators/instructors may not necessarily know what environmental and learner characteristics are relevant and critical without in-depth research efforts. Thus, insights from scientific studies of behavior, cognition, and the brain would be crucial.
The societal challenges, the advances in digital technologies, the gaps between DLL tools and their fit to learner characteristics, and the impacts of DLL on brain and behavior, form the bases of our discussion in this article. The purpose of this article is not to provide a comprehensive review of the literature; many such reviews already exist as discussed below, including special volumes (e.g., Chapelle & Sauro, Reference Chapelle and Sauro2017; Levy & Stockwell, Reference Levy and Stockwell2006). Our goal in this article is to provide a theoretical synthesis and analytical framework with respect to DLL's current promises, theoretical and pedagogical implications, and future directions.
2. CALL in the past and DLL in the new era
To learn a new language in addition to one's first language (L1) is always challenging. It takes time, effort, attention, motivation, and sustained involvement. The ability to use a language for communication and social interaction is a critical competence needed by everyone in the 21st century. Technology has played a significant role in helping today's learners to acquire a language. In this article, we intend to examine DLL in the views of a wide range of methods and platforms enabled by new digital technologies such as mobile computing, VR, and digital games. CALL has dominated the field for over 30 years since computers became popular (see Otto, Reference Otto, Chapelle and Sauro2017 for a general overview of the history of technology and L2 learning). Many of the methods used by earlier CALL are still widely adopted as the standard methods today (e.g., gap-filling/cloze tests, multiple choices, flashcards, and sentence reordering, both in L2 classrooms and on the web), but fundamental differences exist between the earlier CALL efforts and today's massively interactive, web-based, app-based, and mobile-enabled DLL methods (see Presson, Davy, & MacWhinney, Reference Presson, Davy and MacWhinney2013 for an earlier argument in this regard).
Shifts in the use of technology for language learning and teaching, as with the general trends in education, can be observed in terms of different emphases and focuses of the time based on different theoretical foundations, technological development, and educational paradigms. As described by Warschauer (Reference Warschauer, Fotos and Brown2004), between the 1970s and the 1980s, the behaviorist paradigm had dominated language learning and computer-assisted teaching – that is, the entire CALL field; during this period, the computer-learner were treated in a stimulus-response relationship due to behaviorism, and drill-and-practice remained the main method. The cognitive approach rejected behaviorism for language learning in the 1980s and the 1990s, although the actual paradigm shift from behaviorism to cognitivism occurred two decades earlier (see Gardner, Reference Gardner1984). During this period communicative exercises were emphasized, and fluency, rather than language analyses and grammar, was the major focus of language teaching. CALL software and language games also began to flourish during this period. Next, in the 2000s, the authentic context of learning and social interaction was highlighted (see Otto, Reference Otto, Chapelle and Sauro2017) and social-cognitive dimensions of learning shed light on language education and research. These developments also grew alongside the increasing popularity of social media and multimedia technologies (e.g., videos that can incorporate text, graphics, audio, and animations; Mayer, Reference Mayer and Mayer2005).
Based on Warschauer's (Reference Warschauer, Fotos and Brown2004) perspective, Chun (Reference Chun2019) expanded the framework by adding to the focus of DLL in the 2010s seamless digital technologies, technologies that have extended language learning spaces and blurred the boundaries of formal and informal learning. Learning is no longer isolated from the environment; instead, it is embedded in the context in which authentic learning takes place. This development goes hand-in-hand with today's focus on e-learning, blended learning, and multimedia learning, aided significantly by ubiquitous computing, mobile apps, and wearable devices. Such technological advances have greatly promoted multimedia and multimodal learning in all subject areas, and in the last year due to the pandemic, the pace of development has been further accelerated.
With these paradigm shifts for language learning in the past decades, we predict that DLL in the third decade of the 21st century will further focus on new approaches. In particular, big data and AI are impacting every aspect of our lives and our society, from the environment (energy, climate, ecosystem, space) to human behavior (aging, health, education). AI technologies, such as machine learning, automatic speech recognition, and natural language processing (NLP), no doubt also have profound implications for education (Luan, Geczy, Lai, Gobert, Yang, Ogata, Baltes, Guerra, Li & Tsai, Reference Luan, Geczy, Lai, Gobert, Yang, Ogata, Baltes, Guerra, Li and Tsai2020). Language learning is no exception in this regard (see details in Section 5). We have seen an unprecedented increase in the integration of AI and language applications: for example, mobile apps with image recognition and NLP turn the real world into a language learning setting; automatic evaluation systems analyze the errors in L2 learners’ writings (Al-Ahdal, Reference Al-Ahdal2020) and provide instant feedback on correct grammar and hints on best writing (e.g., the popular Grammarly software; see some earliest efforts discussed in Grosjean, Reference Grosjean2019, Chapter 7); the combination of VR and intelligent agents creates immersive and authentic contexts allowing language learners to have social interaction in real-life like situations (e.g., Nicolaidou, Pissas & Boglou, Reference Nicolaidou, Pissas and Boglou2021); and virtual agents through interactive dialogues can enhance learners’ language performance (e.g., Graesser, Chipman, Haynes & Olney, Reference Graesser, Chipman, Haynes and Olney2005; Junaidi, Hamuddin, Julita, Rahman & Derin, Reference Junaidi, Hamuddin, Julita, Rahman and Derin2020; Tai & Chen, Reference Tai and Chen2020); these are only a few of the many examples in recent years.
To truly take advantage of the AI technologies, we must also make use of the big data readily available during language learning, along with the relevant data analytic tools. For example, in a smart learning environment, the entire learning process can be logged on a key-stroke or step-wise level, and the learner data can be automatically analyzed and visualized. Based on such analytic results, a personalized learning plan can be recommended and the learning materials that fit individual learning profiles can be appropriately provided (see 3.1; Kokoç, Akçapınar & Hasnine, Reference Kokoç, Akçapınar and Hasnine2021; Yang, Chen & Ogata, Reference Yang, Chen and Ogata2021). Better still, such personalized feedback can be provided in real time, providing instant information to allow learners to adjust their pace as they learn, to see their up-to-the-point achievements, weaknesses, and learning behavior patterns. For the learner, learning opportunities are available anywhere and anytime (Pikhart, Reference Pikhart2020); for the educator and researcher, making use of the data generated in such environments would guide the design and implementation of precise and personalized education (Godwin-Jones, Reference Godwin-Jones, Chapelle and Sauro2017; Lan, Reference Lan, Spector, Lockee and Childress2016; Yang, Reference Yang2021).
While all these developments are exciting, we must caution that learning successes through DLL are not automatically guaranteed. As pointed out by Godwin-Jones (Reference Godwin-Jones2019), the ability to conduct self-regulated, self-directed, and self-reflective learning is essential to learners’ language acquisition. Furthermore, learning outcomes obtained in a DLL environment cannot be precisely evaluated simply by traditional achievement tests. Multifaceted evidence should be leveraged to correctly evaluate the effects of DLL, which may include data-driven analyses of learning patterns and behaviors. Based on this consideration, it is also important to understand characteristics of learning (e.g., what make up a better or worse DLL environment) and individual differences of the learner (e.g., cognitive ability, language aptitude, and learning strategies). Finally, we have shown that learning a new language through innovative technologies brings about positive changes in the learner's brain structure and function (e.g., Legault, Fang, Lan & Li, Reference Legault, Fang, Lan and Li2019b) but so far we have only limited knowledge in this regard. To study how L1 vs. L2 neural representations emerge as a function of DLL will surely be a new exciting direction (see 4.4).
3. New developments in DLL: MALL, VR, and game-based language learning
In this section, we highlight recent developments in digital technologies and their applications in DLL – in particular, mobile-assisted language learning (MALL), virtual reality (VR), and digital game-based language learning (GBLL). Shadiev and Yang (Reference Shadiev and Yang2020) listed 19 different technologies that have been used for language learning and teaching, many of which are based on the latest digital technologies. There is a tendency to name all technology-enabled language learning as CALL, but we think this does not do justice to a field that is so rapidly developing and that rides on the successes of new emerging technologies. In our view, ‘computer-assisted’ methods are being replaced, both in practice and in theory by emerging technologies and fields of studies (e.g., multimedia learning, blended learning, situated/embodied learning, and social learning). Furthermore, a new industry has joined hands with educational technology in designing popular digital tools and platforms for language learning. Several highly commercialized products attract millions of users to learn new languages (e.g., Babbel, Duolingo, Rosetta Stone), although their scope, languages covered, and functionality vary widely. As discussed earlier, technological innovations can drive pedagogical paradigm shifts, and in the case of language education, shifts are occurring from the classroom-based, instruction-oriented, and teacher-centered approaches to student-centered teaching and learning, as in other areas of education. Covid-19 has brought a ‘new normal’, and digital technologies play an even more critical role now than ever before. DLL rides on this tide to move from the ‘computer-assisted’ ideas and methods to the massively socially connected, web-based, and app-based MALL, VR, GBLL tools and platforms, enabling contextualized and embodied language learning to occur in the real or simulated real world.
3.1. Mobile-Assisted Language Learning (MALL)
The popularity of MALL has increased dramatically as mobile devices such as smartphones and tablets become indispensable in our daily lives. Ubiquitous as they are, mobile technologies now provide convenient platforms to support L2 learning anytime and anywhere, overcoming the limitations imposed by physical classrooms. Importantly, MALL allows the learner to acquire a new language through real-life exploration, effectively turning the real world into a learning context. Situated learning (Anderson, Reder & Simon, Reference Anderson, Reder and Simon1996; Dede, Reference Dede2009; Dede, Jacobson & Richards, Reference Dede, Jacobson, Richards, Liu, Dede, Huang and Richards2017) is a concept referring to learning taking place in real-world or real-world like (simulated) situations, which can be implemented on mobile devices or through immersive technologies (see 3.2). Such situated MALL platforms can connect L2 learning with real-life events and contexts (Lin & Lin, Reference Lin and Lin2019; Lin, Lin, Liu, Kou, Kulikova & Lin, Reference Lin, Lin, Liu, Kou, Kulikova and Lin2020; Shadiev, Hwang & Huang, Reference Shadiev, Hwang and Huang2017), and many MALL applications also involve game playing which promotes language learning through self-exploratory and knowledge construction processes. An additional new direction has been to integrate MALL with data-driven and AI-inspired methodologies, such as automatic speech recognition, NLP, and image recognition, resulting in many new web-based tools or apps (e.g., Chen, Yang & Lai, Reference Chen, Yang and Lai2020; Shadiev, Zhang, Wu & Huang, Reference Shadiev, Zhang, Wu and Huang2020).
Drawing from Kearney, Schuck, Burden and Aubusson's (Reference Kearney, Schuck, Burden and Aubusson2012) framework for mobile learning, Lai and Zheng (Reference Lai and Zheng2018) identified three key features that make MALL significant for L2 learning: personalization, authenticity, and connectivity. By surveying many college students with follow-up interviews, the authors found that the students used MALL mostly for their personal learning purposes, and less for authentic language learning or social connection. In a more recent review, Tu, Zou and Zhang (Reference Tu, Zou and Zhang2020) expanded on these features to include portability, real-time interaction, and situated learning, but also reviewed the negative aspects of MALL such as limited screen space and users’ short attention span for learning. Some commercial products such as Google Translate provide situated learning through instant phone camera translations while Instagram and WhatsApp enable social networking groups to learn L2 and chat with native speakers on the phone. Tu et al. also articulated an evaluation framework for MALL apps designed for vocabulary learning in terms of factors such as content quality, multimodal presentation, engagement, and usability. While most MALL applications are designed for young adults, Puebla, Fievet, Tsopanidi and Clahsen (Reference Puebla, Fievet, Tsopanidi and Clahsen2021) conducted a web-based survey with over 200 participants and further in-depth interviews to see whether older adults are open to using MALL for learning L2. The authors found that older adults, unlike younger generations, are more resistant to adopting MALL applications, mainly because they dislike personal interactions that are not face-to-face. MALL and DLL in general have so far been focused on young adults or college students, and their application and use for older adults thus require further examination (see also Wang & Christiansen, Reference Wang and Christiansen2019 who tested a population with a mean age of 51, which may be too young to count as ‘older adults’).
It has become popular for MALL applications to use QR codes attached to real objects to enable mobile phones to display L2 sounds and labels (e.g., Chinese characters). Liu, Chen and Hwang (Reference Liu, Chen and Hwang2018) developed such a context-aware system for improving L2 English learners’ listening comprehension. It allowed learners to scan QR codes attached to exercise machines in a fitness center to learn exercise-related vocabulary collaboratively. Other than vocabulary, higher-level language skills, such as conversational interactions and writing, can also benefit from mobile technology-based language tasks (e.g., Gharehblagh & Nasri, Reference Gharehblagh and Nasri2020; Lan & Lin, Reference Lan and Lin2016). Previous work has found that students using MALL outperform their peers without MALL support; for example, in oral communication (Lan & Lin, Reference Lan and Lin2016) and in English writing (Gharehblagh & Nasri, Reference Gharehblagh and Nasri2020). Even at the non-linguistic level, Lee, Lo and Chin (Reference Lee, Lo and Chin2021) showed that mobile technologies support the integration of multimodal information and social interaction, which can trigger intercultural learning and increase multicultural awareness. Lomicka and Ducate (Reference Lomicka and Ducate2021) also encouraged students to work with peers collaboratively, and through posts at Padlet, a social networking app, the students could share ideas and knowledge about culture and cultural experiences. Given the tight-knit relationships among sociocultural adaptation, intercultural learning, and L2 proficiency (Ward & Kennedy, Reference Ward, Kennedy, Pandey, Sinha and Bhawuk1996), MALL can play a significant role in enhancing both communicative competence and intercultural interaction.
A novel use of the MALL technology is to combine it with adaptive learning algorithms to enable the design of learning material to better fit student profiles (see Section 5 for further discussion). Sandberg, Maris and Hoogendoorn's (Reference Sandberg, Maris and Hoogendoorn2014) adaptive model is an example of this: they weighted the 120 learning target words by different linguistic characteristics and derived a level of initial difficulty for each word, adjusting the level as student learning progressed. This way the MALL platform could create a dynamic student model that considers the learner's developing level of knowledge. Similarly, Stockwell (Reference Stockwell2007) described an intelligent vocabulary MALL system in which learners’ access and performance information was tracked, and new exercises were automatically generated to fit the level of individual learners. Pandarova, Schmidt, Hartig, Boubekki, Jones and Brefeld (Reference Pandarova, Schmidt, Hartig, Boubekki, Jones and Brefeld2019) further extended this approach to grammar learning, although not on MALL platforms. Theoretically, these approaches are also consistent with the ‘input hypothesis’ of second language theory (Krashen, Reference Krashen1988), according to which the target input for learning should be one level higher beyond the learner's current level of knowledge.
The evidence on MALL's overall effectiveness for L2 learning, as compared with other methods, remains mixed (Chen, Tseng & Hsiao, Reference Chen, Tseng and Hsiao2018; Loewen, Crowther, Isbell, Kim, Maloney, Miller & Rawal, Reference Loewen, Crowther, Isbell, Kim, Maloney, Miller and Rawal2019). For example, learning based on mobile apps, compared with CALL or in-person teaching, produced similar results for high school students (e.g., Peterson, Reference Peterson2010). It may be that high schoolers are a group of users with extremely high frequency of use of mobile phones for social networking, and this has negatively impacted their ability to make use of MALL effectively for content-based language learning. Recent behavioral and brain imaging data suggest that young people's excessive use of electronic devices including mobile phones may have adverse effects on scientific knowledge integration (Hsu, Clariana, Schloss & Li, Reference Hsu, Clariana, Schloss and Li2019) and on Chinese literacy development (Tan & Xu, Reference Tan and Xu2020). Another significant limitation of mobile devices on learning is their small screens, which may increase learners’ cognitive load, especially when the processing of rich and multi-page information is necessary. In addition, older adults may find the screen's small size a particular weakness of MALL when they need face-to-face interaction (see Puebla et al., Reference Puebla, Fievet, Tsopanidi and Clahsen2021). Finally, except a few recent studies most MALL applications remain limited to basic skills such as vocabulary learning (Lai & Zheng, Reference Lai and Zheng2018; Lin & Lin, Reference Lin and Lin2019). Given this limitation, some authors (e.g., Hannibal Jensen, Reference Hannibal Jensen2019; Presson et al., Reference Presson, Davy and MacWhinney2013; Sykes, Reference Sykes, Chapelle and Sauro2017) called for the use of extended mobile technologies to include videos, social media, and Google maps to enhance not just vocabulary learning but also other communicative skills.
3.2. Virtual Reality (VR)
VR has emerged as an important technology for education in the last two decades because of its significant potential and impact on student learning in many educational contexts (see Li, Legault, Klippel & Zhao, Reference Li, Legault, Klippel and Zhao2020; Liu, Dede, Huang & Richards, Reference Liu, Dede, Huang and Richards2017). The role of VR in student learning has received much attention, but its application in experimental studies of L2 learning has been more recent (see Legault, Zhao, Chi, Chen, Klippel & Li, Reference Legault, Zhao, Chi, Chen, Klippel and Li2019a; Li et al., Reference Li, Legault, Klippel and Zhao2020). The term VR can be used to cover a wide range of virtual environments and tools including: dynamic 3D displays projected on computer monitors (desktop or tablet virtual environments; VE); on large screens/walls in amphitheaters, rooms, or specialized cubicles outfitted for 3D images (e.g., CAVE systems); on head-mounted displays (HMD); through devices that show digital image enhancements (‘augmented reality’ or AR); and through a blend of virtual and real-world objects projected onto HMDs (‘mixed reality’ or MR). This broad range of VE, VR, AR, and MR vary in immersion (e.g., 360-degree views vs. limited wide-angle views), interactivity (extent of action and movement), social presence (whether there is feeling of being there), and ultimately realism (how realistically VR simulates the real world).
Broadly speaking, VR can be categorized into two types (Robertson, Card & Mackinlay, Reference Robertson, Card and Mackinlay1993): immersive VR (iVR) and non-immersive VR. Both types of VR aim at creating authentic (i.e., real-world like) environments to enable learning through active and self-exploratory discovery in the virtual environments (Dede, Reference Dede2009). Among the many innovative applications of VR, social interaction through simulated immersion seems to be the most important for L2 learning (see 4.2-4.4). As argued by many theories of L2 acquisition, meaningful social interaction is one of the most significant processes that lead to the success of L2 acquisition (e.g., Ellis, Reference Ellis2019; Lantolf, Reference Lantolf2006; Mackey, Abbuhl & Gass, Reference Mackey, Abbuhl, Gass, Gass and Mackey2012). Another significant advantage of VR, to educators and researchers alike, is its flexibility in designing learning contexts that can vary systematically in environmental characteristics (Casasanto & Jasmin, Reference Casasanto, Jasmin, de Groot and Hagoort2018). A real-world situation contains too many variables or noises that may confound a study, but VR enables modification and manipulation of virtual environments with rigorous control. In other words, VR provides both ‘high ecological validity’ and ‘high experimental control’, thereby lending researchers an excellent tool to study naturalistic events in the lab (Peeters, Reference Peeters2019).
Language learning in VR is contextualized and interaction-oriented. Like MALL, VR fulfills three essential components of successful L2 learning – that is, authentic contexts, learners’ active involvement, and meaningful social interaction (see Lan, Reference Lan2014; Legault et al., Reference Legault, Zhao, Chi, Chen, Klippel and Li2019a; Sadler, Reference Sadler, Chapelle and Sauro2017 for reviews). Sadler (Reference Sadler, Chapelle and Sauro2017) provided a brief history of L2 applications of virtual worlds including platforms such as Second Life. Lan (Reference Lan2020a) suggested that current L2 applications of VR learning can be classified into five categories based on different pedagogical purposes: (1) expanding L2 learners’ visual experience, (2) learning by operating or manipulating virtual objects, (3) learning by creation, (4) creating a joyful learning process, and (5) building a social network. First, the L2 learner can have enhanced visual experiences, particularly in immersive VR contexts. Such experiences may not only match with our visual experiences in the physical world, but also expand our experiences to transcend boundaries in time and space, such as attending a 17th-century drama play in Shakespeare's time, observing creatures under the sea, and walking in outer space, experiences not possible in the real world (Dede et al., Reference Dede, Jacobson, Richards, Liu, Dede, Huang and Richards2017; Mohsen, Reference Mohsen2016). For L2 learning, the student can easily be ‘transported to’ or immersed in regions where the target language is used, along with the relevant cultural artifacts and environmental characteristics. Second, they can manipulate or operate on the virtual objects as in the real environment, sometimes even with enhanced capabilities. For example, in Lan, Fang, Legault and Li (Reference Lan, Fang, Legault and Li2015) and Legault et al. (Reference Legault, Zhao, Chi, Chen, Klippel and Li2019a), L2 learners can move spoons, cups, teapots, and other kitchenware in the VR Kitchen, experiencing the tactile and motoric aspects of the objects when learning the L2 words/labels. The learners could also walk along a path to see the animals in a VR Zoo. This type of tactile, sensory, motoric learning allows learners to contextualize the acquired labels – that is, to represent them in an embodied manner, closely matching with what the child does during L1 learning (see 4.1). Third, in addition to exploring the virtual worlds, sharing one's 3D creation before and during learning is also an innovative VR application (e.g., Yeh & Lan, Reference Yeh and Lan2018). Learning by creation strengthens learners’ ownership and consequently promotes their learning autonomy (Lan, Hsiao, Fang & Chen, Reference Lan, Hsiao, Fang and Chen2018). Fourth, getting immersed in VR worlds is a joyful experience for many users, allowing for learners’ exploration of an unknown environment. In this regard, many studies have indicated that VR motivates students’ positive attitudes towards learning (see Lan, Reference Lan2015, Reference Lan2020b for reviews). Fifth, VR enhances interpersonal interaction through multi-user platforms such as Second Life, allowing L2 learners to create a social community and interact with each other from around the globe. Such a social community also enables L2 learners to perform ‘role playing’ during language learning.
Verbal and non-verbal skills, from vocabulary to listening and from spoken conversation to interpersonal communication, can all be enhanced by VR, given VR's specific features of immersion, interactivity, and enabling of imagination and innovation (Lan, Reference Lan2020a; Li et al., Reference Li, Legault, Klippel and Zhao2020). Further, Chen (Reference Chen2016) showed that virtual environments could enhance students’ engagement and promote collaboration in communication. Even in studies that used basic desktop 3D virtual environments, researchers have found VR to help enhance learning outcomes. For example, Lan et al. (Reference Lan, Fang, Legault and Li2015) constructed Second Life environments to train American students to learn Mandarin Chinese vocabulary. The authors showed that learning in Second Life needed only about half the number of exposures to attain the same level of accuracy as learning via computer-based picture-word paired associations; in addition, students showed faster acceleration of learning in the second phase of training. Such differences between VR learning and non-VR learning were further observed in immersive VR environments in Legault et al. (Reference Legault, Zhao, Chi, Chen, Klippel and Li2019a).
As VR becomes more accessible and portable, more computational resources and tools are also available (e.g., Turbosquid 3D models and Unity development tools), which enables educators to develop real-life like environments more easily (e.g., garden, kitchen, library, MTR station, school, shopping mall, street, supermarket, and zoo). However, there remain a number of limitations of current VR-based applications for L2 learning: (a) sample sizes are small in most studies, limiting the generalizability of findings; (b) descriptive results, rather than statistically tested findings, are usually reported (see Wang, Lan, Tseng, Lin & Kao, Reference Wang, Lan, Tseng, Lin and Kao2020 for a discussion); (c) popular VR applications (and DLL tools in general) such as House of Language VR (Oculus Gear) remain limited in their scope of coverage and number of languages; (d) most of the popular VR headsets (e.g., HTC Vive) remain bulky, and may be unsuitable for younger users. These limitations, we believe, can be overcome in future large-scale studies with future technological developments that make VR more portable and easier to use.
3.3. Game-Based Language Learning (GBLL)
Young people are game lovers, especially the Millennials and the Generation Z who are the ‘digital natives’ growing up with smartphones, tablets, and online games. In the past decades, a significant amount of research interest has been directed to games for education (Mayer, Reference Mayer2016). Against this context, GBLL has become particularly popular in recent years. Although many of the CALL, MALL, and VR platforms discussed above are also game-based, researchers have treated GBLL as a separate methodological approach probably because games have had a longer tradition and wider usage than digital learning.Footnote 2 The idea here is that like other ‘serious games’, GBLL games are not just for fun or entertainment, but are explicitly structured with educational purposes and goals (e.g., learning L2 vocabulary). So far, most GBLL research has focused on learning English as an L2 (over 90% of the studies) and has used video gaming or immersive gaming platforms for single users and role-playing games for multi-users (for reviews see Hung, Yang, Hwang, Chu & Wang, Reference Hung, Yang, Hwang, Chu and Wang2018 and Reinhardt, Reference Reinhardt, Chapelle and Sauro2017).
It is not yet clear how much gaming experience (e.g., frequency/amount of time, and proficiency in playing computer games) can affect the success of L2 learning. Hung et al. (Reference Hung, Yang, Hwang, Chu and Wang2018) reviewed several studies that indicate a potential relationship between experience in digital games and the learner's L2 proficiency, particularly for male students (e.g., Smith, Li, Drobisz, Park, Kim & Smith, Reference Smith, Li, Drobisz, Park, Kim and Smith2013; Sundqvist & Sylvén, Reference Sundqvist, Sylvén and Reinders2012). However, the evidence so far is mixed regarding GBLL's effectiveness as compared with traditional methods of language learning (e.g., deHaan, Michael Reed & Kuwada, Reference deHaan, Michael Reed and Kuwada2010; Sundqvist & Wikström, Reference Sundqvist and Wikström2015). For example, Rachels and Rockinson-Szapkiw (Reference Rachels and Rockinson-Szapkiw2018) found that Spanish L2 learning using Duolingo and traditional teacher-student instruction did not make a difference; similarly, Loewen et al. (Reference Loewen, Crowther, Isbell, Kim, Maloney, Miller and Rawal2019) found that students learning Turkish as L2 with Duolingo had shown limited gains, calling into question the overstated claims on Duolingo's efficacy (Vesselinov & Grego, Reference Vesselinov and Grego2012). Some meta-analyses (e.g., Cerezo, Baralt, Suh & Leow, Reference Cerezo, Baralt, Suh and Leow2014; Grgurović, Chapelle & Shelley, Reference Grgurović, Chapelle and Shelley2013) also indicated mixed results, with some showing an overall advantage of GBLL, while others showing similar performances with both GBLL and non-game based learning. Further, there may be individual differences, as Hung, Young and Lin (Reference Hung, Young and Lin2015) showed that, for high-achieving students, gaming vs. non-gaming conditions did not make a difference, whereas for low-achieving students GBLL was more effective (see also Legault et al., Reference Legault, Zhao, Chi, Chen, Klippel and Li2019a for a similar pattern in VR vs. non-VR learning). Yu (Reference Yu2018) found that, for male more than female students, GBLL led to better English L2 learning than traditional approaches. The good news is that GBLL generally produces positive learning outcomes (e.g., Foomani & Hedayati, Reference Foomani and Hedayati2016; Sato, Murase & Burden, Reference Sato, Murase and Burden2015; Shi, Luo & He, Reference Shi, Luo and He2017), although this positive learning effect might be more evident for vocabulary than for other aspects (grammar, pronunciation, pragmatics; Hung et al., Reference Hung, Yang, Hwang, Chu and Wang2018; Tsai & Tsai, Reference Tsai and Tsai2018; Zou, Huang, & Xie, Reference Zou and Xie2019).
Acquah and Katz (Reference Acquah and Katz2020) suggested six important features that make GBLL particularly appealing for language learning: ease of use, challenging, reward-and-feedback, control/autonomy, goal-directedness, and interactivity. Previous work has indicated that games may activate the user's intrinsic motivation and provide learners with a sense of autonomy or control (e.g., Peterson, Reference Peterson2010). Like MALL and VR, GBLL engages attention, activates prior knowledge, and is often situated in real-life contexts. Acquah and Katz further pointed out that not all six features equally influence language learning; for example, challenging games can increase motivation, but not necessarily improve learning outcomes. Another feature not discussed by the authors is the adaptivity of games (as in MALL, see 3.1), and the extant evidence points to positive effects of adaptive educational games on learning achievement and engagement in general; see Liu, Moon, Kim, and Dai (Reference Liu, Moon, Kim and Dai2020) for a recent review.
Gaming itself is a social process that involves multiple users/parties. While many GBLL platforms have been developed for L2 learners to play on a ‘one-on-one’ basis, multiplayer environments – specifically, the ‘massively multiplayer online role-playing games’ (MMORPGs; Peterson, Reference Peterson2010) – have become important for language learning. Unlike single-user games, MMORPGs operate on connected networks, in real-time, and engage many people simultaneously in the same gaming environment or learning process (e.g., the most popular gaming platform World of Warcraft). According to Wimmer (Reference Wimmer and Carpentier2008), we should identify the important elements for ‘dynamic interaction’ in MMORPGs – including, at least, the learners, the environments, the objects in the environments, and the results of interactions among these elements. Peterson (Reference Peterson2016) further extended these to include other features of MMORPGs: large number of users, use of personal avatars, real-time interaction, immersion in virtual worlds, game-embedded quests, and extensive user-created contents, which all may be highly relevant to language learning. From a cognitive perspective, unlike other GBLL tools, MMORPG games are particularly facilitative to L2 production, because the learner needs to develop a communicative ability by holding dialogues with other players in the language of the game (e.g., Reinders & Wattana, Reference Reinders and Wattana2014; Suh, Kim & Kim, Reference Suh, Kim and Kim2010). From a sociocultural perspective, MMORPGs provide learning environments that are conducive to socialization through language use, and help to develop a positive learner attitude (Peterson, Reference Peterson2016).
4. How does DLL matter? Insights from multiple dimensions
The new developments in DLL as discussed above indicate the arrival of an exciting era but also a crossroad for digital technology and language learning. Significant gaps remain both theoretically and empirically in the understanding of how digital technologies may be leveraged to enhance student performance, not just for language learning but for all domains of learning. Previously we discussed several important features/affordances of digital learning, including interactivity and autonomy/control, but, without a theoretical understanding of the roles of these affordances in learning, we will remain unclear about why and how DLL can benefit students and teachers. For example, what features in DLL environments are critical and conducive to L2 learning, and what empirical evidence is there? Does DLL learning lead to deeper cognitive processing and better L2 achievement than the traditional learning methods? Can DLL learning enable direct mappings between L2 and concepts and hence promote embodied representation in the L2? Are joint social attention and affective-emotional processing similarly important for adult L2 learning as for child L1 learning? What positive brain changes might we expect as a function of DLL, and what neural networks underlie DLL versus traditional L2 learning? And, finally, what emerging technologies in AI and big data analytics can we incorporate into DLL for personalized L2 learning? These are the kinds of questions that we as educators and researchers must tackle, and the answers may also have implications for better pedagogical practices and DLL product designs.
To address these questions, we must not only focus on the cognitive and social aspects of DLL, as already suggested by Peterson (Reference Peterson2016; see 3.3). We must also study other dimensions of learning that may be critical for successful L2 learning. Below we discuss four such dimensions – namely, cognitive, social, affective, and neural – with respect to DLL.
4.1.Cognitive dimensions
An important area of study in cognitive science in the last decades has been embodied cognition. According to the embodied cognition theory (Barsalou, Reference Barsalou, Semin and Smith2008; Glenberg, Sato, Cattaneo, Riggio, Palumbo & Buccino, Reference Glenberg, Sato, Cattaneo, Riggio, Palumbo and Buccino2008; Willems & Casasanto, Reference Willems and Casasanto2011), our mental representations consist of not just symbolic abstractions, as assumed in classic cognitive theories, but conceptual properties that are deeply grounded in our body and our perceptions/actions in the physical world. Such theories highlight the “interaction between perception, action, the body and the environment” (Barsalou, Reference Barsalou, Semin and Smith2008), and how body-specific (e.g., head, hands, feet) and modality-specific (e.g., auditory, visual, tactile) experiences are embedded in our mental representations. An embodied representation of a ‘spoon’ is not just its curved shape, the spelling of the letters, the fact it is used for eating, but an integrated memory of activity/eating with a spoon, the texture and size of a spoon, the fact it appears together with a plate or bowl, and that it is usually in a kitchen or restaurant, all of which form the conceptual representation of spoon – that is, the schema for ‘spoon.’ Furthermore, such embodied representations can activate the brain's visual and sensorimotor regions when the concept is retrieved, due to the way the concept has been encoded via perception and action.
The embodied cognition hypothesis allows us to see why DLL is fundamentally different from traditional classroom-based, translation-based, and teacher-centered L2 learning. In classroom-based vocabulary learning, for example, the teacher provides a list of foreign language words, and asks the student to learn by associating the list with the corresponding L1 word list, most likely through L2-to-L1 word translations; in traditional CALL, such translation-based associations can be implemented through digital flashcards, so that the correct associations can be tallied electronically. Learning in this way can be highly effective in the short term, but might result in the so-called ‘parasitic’ L2-on-L1 representation (Hernandez, Li & MacWhinney, Reference Hernandez, Li and MacWhinney2005) or stronger L2-to-L1 links (Kroll & Stewart, Reference Kroll and Stewart1994). This contrasts with the situation in which the child learns the L1 words; for example, the child acquires an embodied representation of ‘spoon’ through using the spoon in the kitchen, feeling its shape and texture, eating with it with a bowl, and often with a parent/adult around. Such perception-action features are absent in the classroom during adult L2 learning of the Spanish word ‘la cuchara’ through translation/association with its L1 equivalent ‘spoon.’ DLL can help to remedy this situation through technologies such as VR and simulated actions within VR that the learner can perform, as illustrated in Figure 1: the L2 learner can see, point to, pick up, and move kitchen objects associated with the L2 word/label, or even simulate the corresponding action (e.g., drinking with a cup, squatting to pick up a broom). Thus, DLL enables a child-like learning process, which may be critically important for building an embodied representation in the L2: the learner encodes the L2 word by making direct contact with the concept without the mediation of L1, unlike in L2-to-L1 translation/association learning.
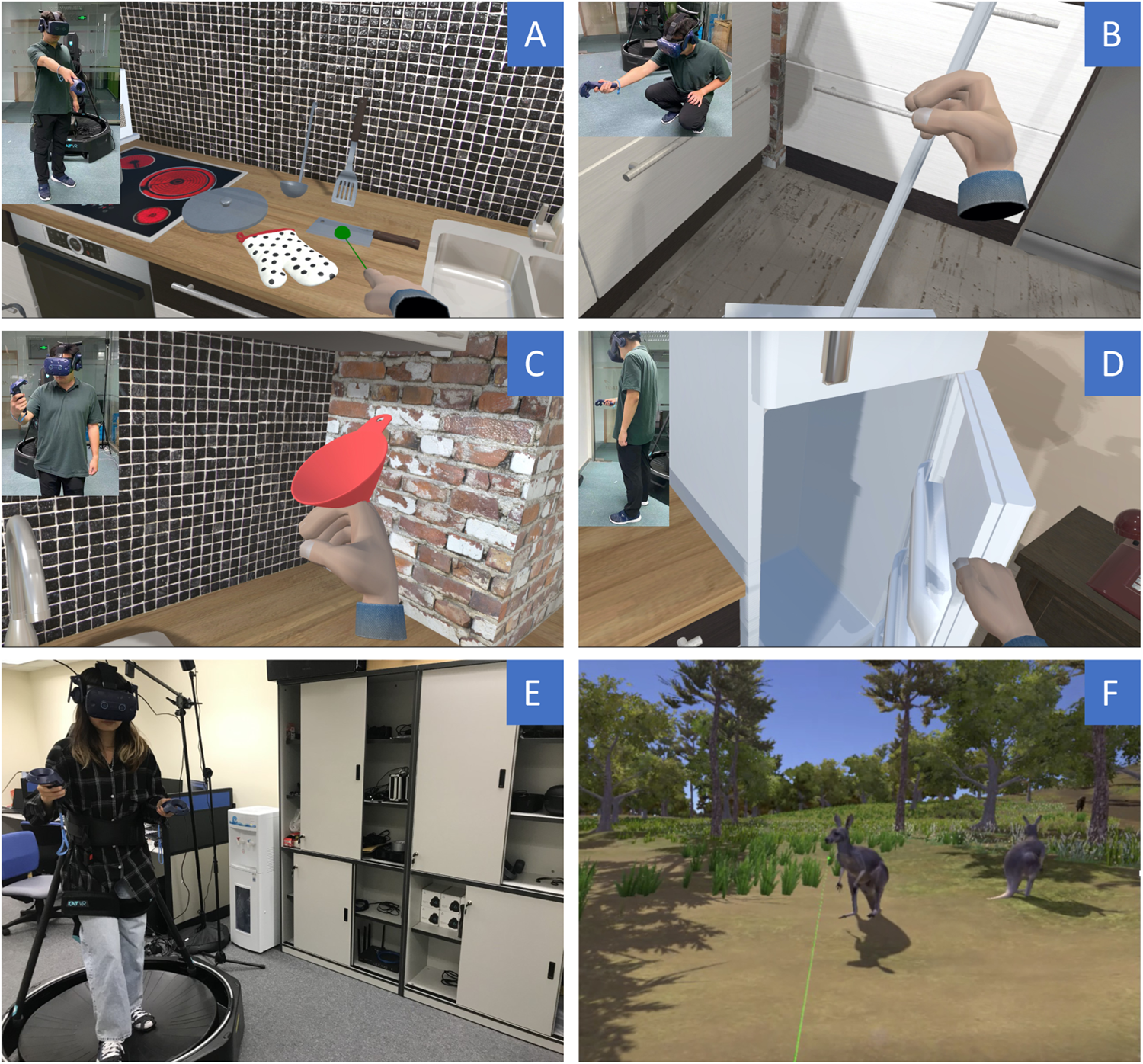
Figure 1. Perception and action in immersive VR. (A) The L2 learner uses the handset to point to any item in the VR kitchen, which triggers the sound of the corresponding L2 word, in this example, ‘dao’ (knife in Chinese); (B) the learner virtually picks up and moves any object by pressing a trigger button with the index finger, in this example, a broom; (C) the learner holds a funnel to move it around; (D) the learner opens the refrigerator; (E) the learner uses a VR treadmill to navigate a virtual zoo; and (F) kangaroos in the virtual zoo, and as in (A) the learner uses the handset to point to the animal to trigger the L2 sound.
Relevant to the discussion here is the question of what type of perception and action will be most conducive to the establishment of embodied representations. According to the National Academies of Sciences, Engineering, and Medicine (2018), learning technologies offer ‘affordances’ (features or properties of objects that present a given object in a particular way when being used), and consideration of the affordances of a given technology is important for understanding student learning. Interactivity, adaptivity, feedback, linked representations, and communication with others are among the key affordances of today's digital technologies. Software designers as well as researchers should consider these affordances when developing or examining DLL products. For example, interactivity can be achieved in MALL, VR, and GBLL through user-to-user, user-to-object, or user-to-context interactions, and can be simulated with or without actual bodily actions (e.g., on the desktop computer, with a smartphone, or through Microsoft Kinect; see Lan et al., Reference Lan, Hsiao, Fang and Chen2018). We will further analyze these affordances in the remainder of this article.
Learner characteristics are significant to our discussion of the cognitive dimensions, too. Identifying the cognitive abilities of the learner will enable us to understand how these abilities may be brought to bear on the L2 learning task. Specifically, two kinds of cognitive abilities have been implicated in technology-based learning: spatial abilities and executive function abilities (particularly working memory). Spatial abilities refer to an individual's ability to analyze spatial features of an environment, to navigate a complex landscape, and to construct a mental map. Various studies have shown that spatial abilities are essential for academic performance in a variety of science subjects (e.g., Kozhevnikov, Motes & Hegarty, Reference Kozhevnikov, Motes and Hegarty2007; Pani, Chariker & Naaz, Reference Pani, Chariker and Naaz2013). For example, Naaz, Chariker and Pani (Reference Naaz, Chariker and Pani2014) found that students who scored higher on mental rotation tasks (Vandenberg & Kuse, Reference Vandenberg and Kuse1978) also performed better on learning brain anatomy in a 3D dynamic environment. The abilities to mentally analyze and represent spatial features and relations are also highly relevant to language learning: the child learns L1 in a natural context with rich spatial cues, such as in environments of house, kitchen, and zoo, all involving a spatial layout with object locations relative to one another. DLL provides an authentic learning context for adult L2 comparable to that for child L1, aiming at grounding L2 learning in simulated or real environments (see Li & Jeong, Reference Li and Jeong2020). Hsiao, Lan, Kao, and Li (Reference Hsiao, Lan, Kao and Li2017) showed that, given the same DLL virtual spatial layout, L2 learners perform differently, both in the use of learning strategies (e.g., more self-exploratory roaming vs. sequential learning) and in the learning outcomes (high- vs. low-achieving). Such differences may stem from learner characteristics, including spatial analytic abilities. Legault et al. (Reference Legault, Zhao, Chi, Chen, Klippel and Li2019a) also showed that learners with higher spatial abilities performed better when learning in a VR zoo environment (where there is spatial navigation) than in a VR kitchen environment (where there is no spatial navigation). The authors further showed that, for highly successful learners, learning in VR vs. non-VR conditions did not matter, whereas, for the struggling learners, VR significantly promoted learning, a pattern consistent with data from GBLL-based research (see 3.3). Interestingly, such effects interacted with simulated action embodiment, such that, in general, kitchenware L2 names were learned better than animal names, perhaps due to the learner's ability to perform more action-based manipulations of objects in the virtual kitchen (where the learner can pick up and move objects around, which is not possible in the VR zoo). Figure 2 illustrates these differences based on Legault et al.'s (Reference Legault, Zhao, Chi, Chen, Klippel and Li2019a) findings.

Figure 2. Effects of learning context, category, and individual differences. (A) There was an overall significant difference between immersive VR (iVR) vs. non-VR associative learning (WW, word-to-word association); (B) there was a significant difference between learning in Kitchen vs. learning in Zoo (both in iVR conditions); (C) there was no significant effect of learning context for Successful Learners; and (D) there was a significant effect of learning context for Less Successful Learners, with significantly higher accuracy in the iVR compared to the WW condition. Error bars indicate 95% confidence intervals and * indicates significant effect (based on Legault et al., Reference Legault, Zhao, Chi, Chen, Klippel and Li2019a).
There has been ample evidence on the role of executive function, particularly working memory, in L2 learning (Baddeley, Reference Baddeley2003; Miyake & Friedman, Reference Miyake, Friedman, Healy and Bourne1998; Wen, Biedrón & Skehan, Reference Wen, Biedrón and Skehan2017). However, it is so far unclear how working memory might play its role in DLL. Legault et al. (Reference Legault, Fang, Lan and Li2019b) reported preliminary data regarding the neural correlates (see 4.4) of working memory for VR learning. These data suggested that working memory may be more important for DLL learners when the learning environment has many details and distractions (e.g., in a VR zoo), where the learner needs to attend to and monitor L2 target material while ignoring/inhibiting irrelevant information in the virtual environment. In such situations, the successful learner not only conducts more self-exploratory learning, but also dynamically keeps track of upcoming information using working memory and executive function.
Finally, previous work has indicated that DLL, as compared with traditional methods, can lead to deeper cognitive processing (e.g., Erhel & Jamet, Reference Erhel and Jamet2013). In human memory research, the well-known ‘encoding-specificity principle’ (Tulving & Thomson, Reference Tulving and Thomson1973) suggests that, if the encoding and retrieval contexts match, people learn better; for example, a word list encoded underwater would be retrieved better underwater than on dry land (Godden & Baddeley, Reference Godden and Baddeley1975). Further, it is well established that deeper and more elaborative processing of that information (e.g., relating to semantic content of a word) leads to better long-term memory retention and retrieval, as compared to shallow processing (e.g., counting the number of letters in a word), supporting the classic cognitive theory of ‘levels of processing’ (Craik & Lockhart, Reference Craik and Lockhart1972). Deeper processing may also involve multimodal processing, i.e., encoding of multiple sources of information (e.g., reading, writing, and hearing the same word). This ‘multimodal advantage’ is a central premise of the multimedia learning theory (Mayer, Reference Mayer2014), which suggests that students learn and remember better with words and pictures together than with words alone (see Liu, Wang, Li, Ding, Yang, & Li, Reference Liu, Moon, Kim and Dai2020 for recent fMRI evidence). Multimedia platforms give the learner a chance to select, organize, and integrate diverse information, and mobile-based apps, VR, and game-based learning all take into consideration how auditory, visual, and tactile information may be leveraged simultaneously for successful L2 learning.
4.2. Social dimensions
DLL's role in promoting contextualized, situated, and embodied L2 representations is highlighted, by the above-discussed cognitive theories, cognitive abilities, and multimodal information processing. DLL, in essence, attempts to equate, with the help of technologies, conditions of adult L2 learning with those of child L1 learning by grounding the learning process in the context in which language is used. In learning the word ‘spoon’, the child abstracts a representation through repeated ‘episodes’ of interactions associated with using a spoon in the context; the same can be done through simulations in VR or games when adults acquire the corresponding L2 representation. The Unified Competition Model (MacWhinney, Reference MacWhinney, Gass and Mackey2012) postulates that there are no fundamentally different principles underlying L1 learning vs. L2 learning, but the processes and contexts under which these two types of learning take place are different. If L1 and L2 learning conditions can be equated, L2 learners can fend off the ‘risk factors’ such as thinking in L1 (as opposed to using L2 for inner speech) and social isolation (as opposed to integrating socially and culturally with the L2 community). Recently, Caldwell-Harris and MacWhinney (Reference Caldwell-Harris and MacWhinney2021) further expanded this view in an emergentist account of the age effect, focusing on how environmental support, cognitive abilities, and motivational factors change over time in children, adolescents, and adults.
The idea of action-based interactive learning is not new and has long been accepted in child language research (Meltzoff, Kuhl, Movellan & Sejnowski, Reference Meltzoff, Kuhl, Movellan and Sejnowski2009). For children, decontextualized situations (e.g., watching DVDs) do not induce learning; from the earliest stages infants already depend on social interaction, joint attention, shared intentionality, and eye-hand-body coordination for learning success (Kuhl, Reference Kuhl2007; Tomasello, Reference Tomasello2000; Yu & Smith, Reference Yu and Smith2016). Researchers have realized that social interaction and joint attention may also be critical for L2 learning. Verga and Kotz (Reference Verga and Kotz2017) showed that in simulated social learning in the lab, joint attention between the participant and the experimenter helps to orient the learner's attention to the correct meaning among competing alternatives. Caldwell-Harris, Goodwin, Chu and Dahlen (Reference Caldwell-Harris, Goodwin, Chu and Dahlen2014) compared adult L2 learning from live instructors versus that from videos and found that the physical/social presence of the teacher leads to better learning than when the teacher appears only in videos (consistent with findings from Kuhl, Tsao & Liu's Reference Kuhl, Tsao and Liu2003 infant study). These perspectives are highly consistent with both historical and recent trends in language acquisition and L2 learning, from sociocultural theory (Lantolf, Reference Lantolf2006; Vygotsky, Reference Vygotsky1978) to usage-based language learning and processing (Tomasello, Reference Tomasello2000, Reference Tomasello2003), to input and interaction hypotheses (Krashen, Reference Krashen1988; Long, Reference Long1981), all of which highlight the properties and conditions in the learning environment, the linguistic input/output, and the interaction between these properties and learner-specific characteristics and cognitive profiles (see Ellis, Reference Ellis2019 and Mackey et al., Reference Mackey, Abbuhl, Gass, Gass and Mackey2012 for reviews and perspectives). A recent formulation of this interaction has been proposed by Claussenius-Kalman, Hernandez and Li (Reference Claussenius-Kalman, Hernandez and Li2021) in terms of the 3E framework, Ecosystem, Expertise, and Emergentism, which postulates that the emergent patterns of bilingual representation and cognitive processing reflect the dynamic interactions among the complex learning environment, the genotype of the individual, and the developing cognitive abilities of the learner.
On the basis of these data and theories, Li and Jeong (Reference Li and Jeong2020) proposed the ‘social L2 learning’ (SL2) hypothesis, according to which child L1-like representations can be achieved in L2 even for late adults through ‘social learning’ – learning that is perception and action-based, interactive, involving multimodal processing of information relevant to the target L2 environment, either through real-world or simulated contexts. One important SL2 hypothesis is that social learning can promote embodied L2 representations, because of the rich perceptual, sensorimotor, and affective-emotional processes that are embedded in the learning experience. Such experiences engage multimodal information integration, social reasoning, and motoric action or simulation, all of which reinforce long-term memory retention and facilitate retrieval. SL2 also provides a way for adult L2 learners to decouple the L2-to-L1 link that would otherwise be characteristic of late age of acquisition (the ‘parasitic’ representation; Hernandez et al., Reference Hernandez, Li and MacWhinney2005; Li & Zhao, Reference Li and Zhao2013). Moreover, such SL2 learning will necessarily recruit the brain's corresponding key regions that handle perception, action, and emotion, in both hemispheres (see 4.4). Given the social-affective as well as perception/action-based cues, social learning of L2 provides a genuine natural context comparable to that of L1 learning. Not surprisingly, the DLL platforms, most notably MALL, VR, and GBLL, all attempt to make the best use of such social cues for L2 learning. These cues may be analyzed with regard to ‘affordances’, important features that make the context be conducive to learning. Here we focus on two, interactivity and autonomy.
‘Interactivity’ in DLL means that the technology allows the learner to actively interact with the digital environment presented by the DLL platforms (e.g., with a virtual agent or avatar). For example, the learner can assume a specific role in a MMORPG gaming environment or have dialogues with a virtual agent in an immersive VR environment (e.g., Mondly™ relies on this method). Interactivity can also more broadly refer to any visual, manual, or bodily interactions with digital objects; for example, the learner can manipulate objects through hand movements (e.g., picking up a virtual cup in a kitchen) or bodily movements and locomotion (e.g., navigating a virtual town; see Figure 1E-F). Such interactivity is not social interaction in the strict sense but does engage perception/action-based learning in the context, in a way very different from reciting a list of word translations in an L2 classroom. To the degree that a given digital technology enables interactivity, the technology offers different affordances and may consequently have different impacts on learning (e.g., desktop video games do not allow the user to conduct full-body movement during playing or learning, whereas immersive VR does).
In social learning, ‘autonomy’ (sometimes also called ‘agency’; see Mayer, Reference Mayer2014) is another important affordance, implying that the learner is empowered to explore the learning environment, discover facts, control their own learning process and pace, and decide on what and how learning should proceed. This notion of learner autonomy has become particularly popular today, as the emphasis on student-centered learning has gradually taken center stage in education. In the L1 literature, there is evidence that even 9-month-old infants learn better when they have control of the presentation of speech materials for learning (Lytle, Garcia-Sierra & Kuhl, Reference Lytle, Garcia-Sierra and Kuhl2018). In traditional classrooms, the teacher provides the learning target and method; in flipped classrooms, the teacher serves as a facilitator and provides feedback; and in DLL learning, the learner decides on the learning goals (Egbert, Chao & Hanson-Smith, Reference Egbert, Chao, Hanson-Smith, Egbert and Hanson-Smith2007), along with the order, time, and frequency with which the material will be acquired. The student will also have control of how he or she moves around in the digital environment (see the trajectory pattern analyses by Hsiao et al, Reference Hsiao, Lan, Kao and Li2017). The advantages conferred by autonomy in DLL are considerable, and the data derived from learner autonomy often provide information about learner characteristics, learning strategies, and L2 achievement outcomes that are otherwise unavailable (see Section 5).
4.3. Affective dimensions
As compared with research on the cognitive and social dimensions, relatively little work has been done to study the affective dimensions of DLL. However, it is clear from child L1 learning that affective processing, especially emotionality, is equally important for successful language learning. Lytle et al. (Reference Lytle, Garcia-Sierra and Kuhl2018) argued that when children are learning with peers in the same environment, they show heightened social and emotional arousal, which motivates their learning and leads to better performance. Yu and Smith (Reference Yu and Smith2016) identified a positive correlation between child-parent joint attention to objects in the environment and the child's sustained attention, pointing to social interaction as the underlying factor that supports this correlation. It is important that social interactions involve a reciprocal affective relation: the child pays more attention to the object that the adult focuses on, the adult also provides a contingent response to the child's attention, which in turn increases the child's attention (i.e., sustained attention). Without such contingent responses and reciprocal interactions, there will be no role for social interaction to play in learning. Indeed, today's pandemic-induced online learning mode (e.g., through Zoom or Microsoft Teams) often lacks joint attention, contingent response, and reciprocal interaction between the students and the instructor. Sustained attention to the learning content is difficult to maintain in such a setting.
The SL2 hypothesis of Li and Jeong (Reference Li and Jeong2020) argues that lessons learned from child L1 are directly relevant to our understanding of adult learning of L2. As shown by Verga and Kotz (Reference Verga and Kotz2017), even in L2, joint attention is important, but the underlying affective and emotional mechanisms, however, have not been fully explored. Our hypothesis is that social-affective cues could activate the learner's emotional responses as well as deeper cognitive processing, thereby facilitating learning and enhancing the quality of L2 representation. An important component of social learning is about how to better connect with others, both cognitively and emotionally, using joint attention and contingent responses. For example, eye contacts, facial cues, emotional expressions, hand and body gestures, are all human signals on top of textual and verbal information, serving as feedback, appraisal, and interests for continued engagement (or lack therefore); these are crucial to a regular face-to-face social interaction, as in child L1 learning, but are not usually available to classroom-based adult L2 learning. In particular, human faces serve a social function, carrying significant affective information: slight movements of our eyes, eyebrows, nose, lips, mouth, cheekbones, and chins can indicate subtle but important emotional states and convey meanings of happiness, anger, indifference, ignorance, or disgust. More recent studies have also shown that the perceived emotions from the instructor's face can serve as priming to the learner's positive or negative responses during learning (e.g., Lawson, Mayer, Adamo-Villani, Benes, Lei & Cheng, Reference Lawson, Mayer, Adamo-Villani, Benes, Lei and Cheng2020; Pi, Chen, Zhu, Yang & Hu, Reference Pi, Chen, Zhu, Yang and Hu2020). The study of human facial expression has now become a burgeoning field in psychology and cognitive science (Calvo & Nummenmaa, Reference Calvo and Nummenmaa2016).
Given such significant affective functions of human faces, it is clear that under today's pandemic both the student and the instructor suffer when no reciprocal facial expressions are available in learning or teaching. It is also no surprise that the lack of affective processing in traditional L2 instruction may have led to the lack of affective representations of the acquired L2 material. In contrast to previous empirical emphases on how L2 learner's anxiety impedes learning, bilingual representation studies (see Dewaele, Reference Dewaele, Schiewer, Altarriba and Ng2021, for a review) have shown that affective-specific feelings by emotion-laden words (words for affection, taboo words, swearwords, etc.) are more strongly evoked in L1 than in L2. This pattern could be due to the different contexts in which L1 vs. L2 is learned (in natural environments vs. in L2 classrooms) and the resulting semantic representation of emotions in L1 vs. L2 words. Importantly, such L1-vs.-L2 emotionality differences have been found most reliable when the L2 is a later-learned or less proficient/dominant language, showing that late adult L2 representations cannot easily incorporate the rich affective/emotional features that are typical of L1 representations (Caldwell-Harris, Reference Caldwell-Harris2015). Pavlenko (Reference Pavlenko2012) specifically linked L2 representation's weak emotionality to the decontextualized nature of traditional L2 classrooms where few opportunities are offered for integrating multimodal and multisensory information and where disembodied L2 representation results (see also 4.1).
DLL tools and platforms could potentially remedy the lack of L2 affective processing and emotionality differences through automatic feedback in MALL apps, avatars with emotional expressions in VR, and performance-contingent rewards in GBLL (Graesser et al., Reference Graesser, Chipman, Leeming, Biedenbach, Ritterfeld, Cody and Vorderer2009; Park, Kim, Kim & Yi, Reference Park, Kim, Kim and Yi2019). Intelligent tutors or agents can also be built into DLL platforms using automatic speech recognition and AI, such that joint attention and contingent responses can be simulated (see D'Mello & Graesser, Reference D'Mello and Graesser2012 for incorporating human-like facial expressions in intelligent tutoring systems). However, simply providing the instructor's face images on a screen as in today's online teaching might not be sufficient: Resnik and Dewaele (Reference Resnik and Dewaele2021) concluded in a recent study that the projection of the tiny 2D thumb-sized faces of teachers and peers on the screen does not convey the same emotional impact as do real human faces in student-teacher interactions. The Image Principle of the multimedia learning theory also states that “people do not necessarily learn more deeply from a multimedia lesson when the speaker's image is added to the screen” (Mayer, Reference Mayer2014, p. 360).Footnote 3 Finally, whether real human faces and cartoonlike characters (‘pedagogical agents’; see Section 5) make a difference to student learning is an active topic of investigation. Much work is needed in this area.
4.4. Neural dimensions
Our discussion has made it amply clear that DLL, due to its features/affordances on cognitive, social, and affective dimensions, enables L1-like representations in the L2, through the use of interactive and socially relevant contexts and multimodal/multisensory information. If there are such advantages of DLL, how does the brain reflect them? Despite much work in the study of the bilingual brain, we have so far very limited knowledge about how DLL tools and practices impact brain function and structure in L2 learning. Here we predict that the DLL methods will directly impact the L2 learning brain, and this prediction is based on converging evidence from two related literatures: a) action video game playing can enhance attentional control and cognitive resource allocation, leading to neuroplasticity in the central executive network (Bavelier, Green, Pouget & Schrater, Reference Bavelier, Green, Pouget and Schrater2012; Nahum & Bavelier, Reference Nahum, Bavelier, Ramsey and Millan2020); b) bilingual experience can increase executive function including attentional control, leading to brain changes also in the central executive network (Abutalebi & Green, Reference Abutalebi and Green2007; Bialystok, Craik & Luk, Reference Bialystok, Craik and Luk2012; Li, Legault & Litcofsky, Reference Li, Legault and Litcofsky2014). There has also been recent neural evidence that game-based learning, as compared with non-game-based learning of the same material, leads to higher levels of activation in the brain's emotional and reward processing systems (Kober, Wood, Kiili, Moeller & Ninaus, Reference Kober, Wood, Kiili, Moeller and Ninaus2020). Understanding the neural substrates of DLL will not only provide further evidence on the impacts of DLL, but also a window into how brain changes might result from the cognitive, social, and affective dimensions of DLL.
New evidence indicates that the brain can directly reflect the L1 vs. L2 difference with regard to embodied semantic representation: an integrated brain network that connects key language areas with semantic and sensorimotor regions is evoked when semantic processing is performed in L1, whereas such a network is absent or weakly configured for L2 processing (Zhang, Yang, Wang & Li, Reference Zhang, Yang, Wang and Li2020). In the sensorimotor integration hypothesis of Hernandez and Li (Reference Hernandez and Li2007), this difference results from the different ages of acquisition (AoA, early for L1 and late for L2). In the views of the declarative/procedural model of Ullman (Reference Ullman2001), such difference is argued to be the result of procedural learning of L1 and declarative learning of L2. But according to the recent hypothesis of Li and Jeong (Reference Li and Jeong2020), such L1-L2 contrast is best seen as reflecting social learning for child L1 and association/translation learning for adult L2. There is already evidence in the literature that social learning in adult L2 can have a positive impact on the brain, measurable through functional and structural magnetic resonance imaging (MRI; see Stein, Winkler, Kaiser & Dierks, Reference Stein, Winkler, Kaiser and Dierks2014 for an earlier review). For example, Jeong, Sugiura, Sassa, Wakusawa, Horie, Sato and Kawashima (Reference Jeong, Sugiura, Sassa, Wakusawa, Horie, Sato and Kawashima2010) and Jeong, Li, Suzuki, Sugiura and Kawashima (Reference Jeong, Li, Suzuki, Sugiura and Kawashima2021) showed that words learned through videos of social interaction produced more activity in the right supramarginal gyrus (SMG) and angular gyrus (AG), whereas words learned through translation produced more activity in the left frontal gyrus (LFG). Verga and Kotz (Reference Verga and Kotz2017) also showed that simulated partner interaction in L2 learning led to more brain activities in SMG and areas involved in visuospatial learning and sensorimotor processing.
However, there is so far little work focusing on the neural substrates of DLL in this direction. Hong et al. (Reference Hong, Han, Kim, Bae, Kim and Renshaw2017) provided some preliminary evidence that child L2 English learners showed increased resting-state functional connectivity in Broca's and Wernicke's areas after a 12-week game-based training, but the study suffered from a small sample size and lack of a control group. A more recent study by Legault et al. (Reference Legault, Fang, Lan and Li2019b) analyzed the structural MRI data from Lan et al. (Reference Lan, Fang, Legault and Li2015), showing that L2 Chinese learners in the VR condition had a positive correlation between learning performance and brain structure in the right inferior parietal lobule (IPL), where brain structure was measured using cortical thickness. IPL has been regarded as a key hub for vocabulary learning and for multimodal information integration (Binder & Desai, Reference Binder and Desai2011; Mechelli et al., Reference Mechelli, Crinion, Noppeney, O'Doherty, Ashburner, Frackowiak and Price2004). By contrast, the learners in the non-VR condition (word-to-picture association) showed no such correlation.
Enabled by digital technology, DLL makes social-affective cues available to adult L2 learners that are normally only available to L1 learners. In other words, DLL enables social learning without putting the L2 learner in the physical social environment such as in immigration or study-abroad situations. The consequence is that DLL learners, as compared with translation/association-based learners, will necessarily engage a broader brain network in cortical, subcortical, and limbic systems, in both the left and right hemispheres, for effectively analyzing linguistic and non-linguistic perceptual information. This broadened brain network leads to enhanced cognitive processing, increased social-affective response, higher levels of motivation, better long-term memory retention, and faster memory retrieval. Figure 3 is an illustration of what such a network might look like.
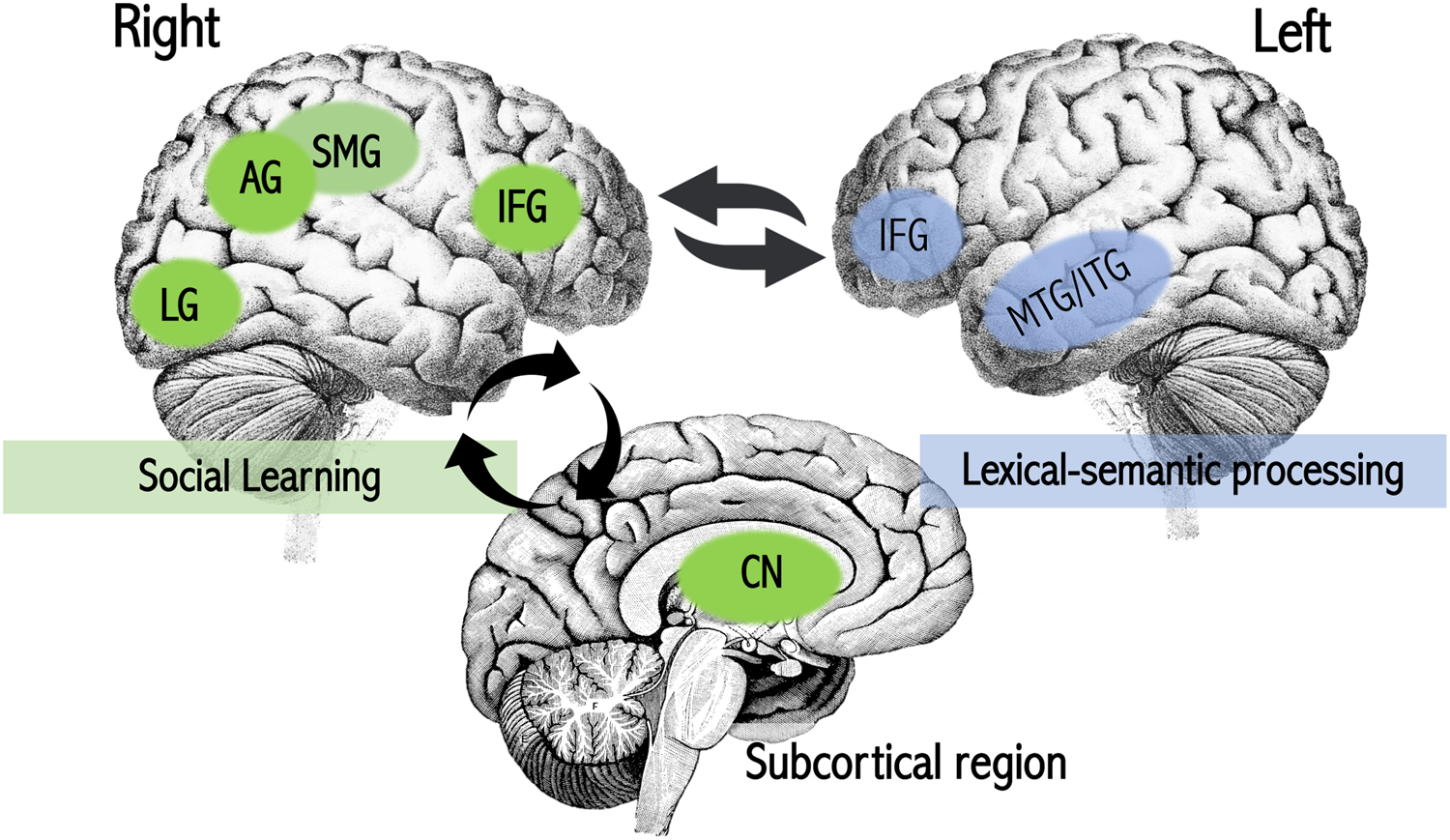
Figure 3. Brain network that supports lexical learning and social learning in both hemispheres. The figure illustrates a typical left-hemisphere lexical learning (blue) and a right-hemisphere social learning (green) system. The latter involves a right-heavy network that connects key regions in both hemispheres for visual processing (LG) and cognitive and linguistic processing (IFG, AG, SMG, MTG) with the subcortical region (CN for sequence learning). AG: angular gyrus; IFG: inferior frontal gyrus; SMG: supramarginal gyrus; LG: lingual gyrus; CN: caudate nucleus; MTG/ITG: middle/inferior temporal gyrus. (from Li & Jeong, Reference Li and Jeong2020; with permission from Springer Nature)
This figure highlights the contribution of the right hemisphere to the learning of L2, contrasting the traditional left-hemisphere dominant language/lexical processes. It has become increasingly clear that the right hemisphere plays a much more important role than previously thought in adult L2 learning (see Qi & Legault, Reference Qi and Legault2020, for a recent review). It is our hypothesis that DLL can enable the learner to establish direct and strong links between new L2 forms and social-affective features of the environment, leading to richly contextualized and embodied semantic representations. Much work needs to be done to identify such representations clearly in the L2 brain. We will need to rely on recent advances in network science (e.g., Bassett & Sporns, Reference Bassett and Sporns2017) to delineate the specific connections, dynamic pathways, and overall organizations among the key brain regions, as well as the cooperation between the left and right hemispheres; in the case of DLL, we need to identify the particular impacts that MALL, VR, and GBLL may have on the structural brain change and functional connectivity due to L2 learning (see Li et al., Reference Li, Legault and Litcofsky2014; Yang & Li, Reference Yang and Li2019; Zhang et al., Reference Zhang, Yang, Wang and Li2020).
Given this perspective, future directions should also include the study of neural networks underlying social learning and their interactions with the extended language network (see Ferstl, Neumann, Bogler & Von Cramon, Reference Ferstl, Neumann, Bogler and Von Cramon2008; Hagoort, Reference Hagoort2019; Meltzoff et al., Reference Meltzoff, Kuhl, Movellan and Sejnowski2009). For example, the learner may participate in a process of ‘social reasoning’, engaging the so-called ‘theory of mind’ (ToM; Frith & Frith, Reference Frith and Frith2012; Saxe, Reference Saxe2006). ToM activates the brain's mentalizing network, including medial prefrontal and bilateral temporoparietal junction regions, when thinking about other people's beliefs, desires, emotions, and intentions. In the case of language, this network may be engaged when the individual is trying to make inferences or take another person's perspective, which is highly relevant to the acquisition of L2 pragmatics that can also be aided by DLL (Sykes, Reference Sykes, Chapelle and Sauro2017). Thus, we need to understand how our brain's linguistic system, memory system, emotional system, and theory of mind all work together as an integrated network to facilitate L2 learning and bilingual representation.
5. Emerging technologies and DLL: AI, Big Data, and personalized learning
The study of language learning has become a highly interdisciplinary enterprise due to its interaction with psychology, education, neuroscience, and now with machine learning. Meltzoff et al. (Reference Meltzoff, Kuhl, Movellan and Sejnowski2009) used child language learning as a bona fide example to illustrate key principles for a ‘New Science of Learning’, in that language learning fulfills three premises simultaneously: (a) learning is a computational process, (b) learning is a socially interactive process, and (c) learning is supported by a dynamic neural circuitry linking perception and action. We believe that adult L2 learning can be equally positioned, if we adopt the DLL approach illustrated in this article. DLL follows the theoretical and methodological advances in education, cognitive science, and neuroscience, as discussed above. Moreover, DLL depends heavily on the latest technologies from mobile computing and VR to digital games. In this section, we discuss how emerging new technologies could further expand the impacts of DLL for the future.
Recent years have witnessed rapid developments and applications in AI and big data analytics. These developments have had profound impacts on all aspects of our lives. Although AI and data-driven language learning technologies are still at an early stage, learning with digital tools and platforms has become the norm as DLL attests, and it generates a vast amount of data in a short period of time (the so-called ‘data deluge’) which quickly exceeds the capacity of traditional data analytic methods. For example, in MALL, the apps can record each click as learning progresses; in VR, a student may traverse a virtual environment and every activity or movement may be recorded as a learning event (e.g., the activities depicted by Figure 1A-E); and in game-based learning, playing a game with multi-users could involve rapid interactive dialogues, resulting in many words and utterances in seconds. Further, cutting-edge immersive technologies such as VR-Eye integration and VR-EEG integration have enabled the collection of large-scale, multi-dimensional, and continuous data as learning occurs in real time, which include not only behavioral patterns but also eye gazes, electrophysiological, and neurocognitive responses during learning. Even learner's emotional and affective states/responses can be automatically captured through sensors and wearables (e.g., HTC Vive Facial Tracker, eye-trackers) or other experimentally designed tools (e.g., body posture measurement system, see D'Mello & Graesser, Reference D'Mello and Graesser2012). Such rich data provide, on a moment-by-moment basis, details about the object features that learners attend to, about learners' attention and cognitive spans, and about their spatial movements and navigation patterns in terms of time, speed, accuracy, and frequency. These complex multimodal and multimedia data differ significantly from traditional data collected after learning (answers to questionnaires and interviews, multiple choices, etc.), and lend themselves readily to data-intensive analytics based on advanced statistics, machine learning, and AI techniques.
One important question to ask is whether we can make use of the data deluge and data analytics from DLL to identify, predict, and adapt to individual differences in light of different learner characteristics. This is the idea of ‘personalized learning’ or ‘precision education’: educators take into consideration learner-specific characteristics, abilities, and strategies/styles of learning when developing curricula and pedagogies to fit the cognitive, social, and affective profiles and demands of different learners so as to optimize learning (Hawk & Shah, Reference Hawk and Shah2007; see Luan et al., Reference Luan, Geczy, Lai, Gobert, Yang, Ogata, Baltes, Guerra, Li and Tsai2020 for a recent white paper on AI and big data in education). For example, corpus linguistic research has led to a large amount of word and text corpora, often open-access, with very detailed information about linguistic properties and usages, such as in the databases or corpora of WordNet (Fellbaum, Reference Fellbaum1998; Miller, Reference Miller1995), BNC (BNC Consortium, 2007), and COCA (Davies, Reference Davies2008). However, DLL tools and platforms have yet to seamlessly incorporate such information (e.g., lexical concordances) for intelligent L2 learning and teaching (see Ma, Reference Ma, Chapelle and Sauro2017 for a discussion).
To effectively design personalized learning, we need to understand both the internal characteristics of the learner (e.g., cognitive abilities, affective states, learning styles) and external characteristics of the environment (e.g., affordances of the learning context), and how the two interact (e.g., learning strategies in the context). In 4.1 we pointed out that individual differences in working memory may be particularly important for VR learning, but how working memory interacts with affordances of VR environments for L2 learning remains to be understood in the perspective of personalized learning. For example, Hsiao et al. (Reference Hsiao, Lan, Kao and Li2017) showed how we could use advanced statistical analyses and computational models to identify the relations between navigation patterns of learners and their L2 learning strategies, and to predict their language learning success. In addition, using methods developed in other fields (e.g., ‘roaming entropy’ used to measure rat movements in maze running; Freund et al., Reference Freund, Brandmaier, Lewejohann, Kirste, Kritzler, Kruger, Sachser, Lindenberger and Kempermann2013), we can also identify learners’ traversing patterns within the digital environment. Such analyses indicated that the self-explorers (‘high roamers’) vs. the sequential learners (‘low roamers’) differed in learning outcome, high-achieving vs. low-achieving, respectively. Further, individuals with higher working memory, when facing a complex virtual environment, may be more able to keep track of the continuously updating visual scenes and ignore or inhibit irrelevant information, and therefore they are the ones more likely to adopt self-exploratory learning.
The next question to ask is if we might be able to modify and adapt the digital environment or virtual context to optimize individualized learning; for example, some distracting or ‘seductive’ details not directly relevant to the learning task can be simplified or eliminated in the virtual environment, such that individuals who have a lower working memory may more effectively focus on the L2 targets without getting distracted (see 4.1). This would make much sense in light of the ‘cognitive load’ theory (Mayer & Moreno, Reference Mayer and Moreno2003; Sweller, Reference Sweller1994), according to which irrelevant audio-visual details (e.g., illustrations, images, faces), even if appearing highly attractive, can present increased demands on the learner's cognitive processing resources. However, we need to understand what audio-visual materials might be more distracting from learning versus more conducive to learning, and what kinds of learners might benefit more or less from them. As mentioned earlier, to design effective DLL tools and platforms, we must separate technological features from human characteristics and learner abilities, which will in turn help us better understand the efficacy of technological products. We need a greater synergy between technology and human characteristics – nowhere more than in education – and we must make our technologies be adaptive to individuals’ cognitive, social, affective, and linguistic abilities and profiles.
How can we best combine the power of digital technology and that of AI and machine learning for developing personalized L2 education? Preliminary evidence suggests that we can indeed develop learner-specific models and materials through data-driven methods to enhance personalized vocabulary learning; for example, by analyzing detailed individual learning logs (e.g., Zou & Xie, Reference Zou and Xie2018). One critical aspect, in addition to the key affordances of digital technologies discussed above, is feedback, which has been extensively examined in the multimedia learning literature generally (e.g., Moreno & Mayer, Reference Moreno and Mayer2004; Moreno & Valdez, Reference Moreno and Valdez2005) and in second language acquisition research specifically (Mackey et al., Reference Mackey, Abbuhl, Gass, Gass and Mackey2012; Presson et al., Reference Presson, Davy and MacWhinney2013). Feedback has been shown to contribute positively to learner motivation, cognitive processing, memory retention, and learner's enjoyment/feeling of rewards (e.g., Erhel & Jamet, Reference Erhel and Jamet2013; Sweetser & Wyeth, Reference Sweetser and Wyeth2005). In this respect, an exciting domain inspired by AI and big data analytics is the development of intelligent tutoring systems (ITS, such as AutoTutor; see Graesser et al., Reference Graesser, Chipman, Haynes and Olney2005; Nye, Graesser & Hu, Reference Nye, Graesser and Hu2014). ITS incorporates AI and machine learning algorithms to provide the learner with direct, immediate, and to-the-point feedback, not simply in the form of right or wrong answers. Like a human instructor, ITS can give feedback containing detailed, content-based corrections, comments, and suggestions, in response to and tailor-made to the individual's learning behavior and outcome.
Feedback represents a key affordance for digital technology to be both personal and humanistic – personal because it considers learner-specific patterns and humanistic because it incorporates other human-relevant features in the learning environment. In human face-to-face tutoring, the learner has social-affective-emotional cues including facial expressions, eye gazes, and body and manual gestures. ITS systems aim to incorporate, in addition to content-based feedback, such personal features through the design of animated ‘pedagogical agents’, the anthropomorphic animated human-like characters, to serve as virtual tutors. Johnson, Rickel and Lester (Reference Johnson, Rickel and Lester2000) and Johnson and Lester (Reference Johnson and Lester2016) suggested that pedagogical agents should possess these social and affective-emotional features to qualify them as effective agents for guiding learning in interactive/immersive environments. Most important among these features, in our view, are the pedagogical agent's abilities to provide performance-contingent verbal and nonverbal feedback and to respond to affect and emotions in real time; hence, being socially intelligent (e.g., D'Mello & Graesser, Reference D'Mello and Graesser2012; Louwerse, Graesser, Lu & Mitchell, Reference Louwerse, Graesser, Lu and Mitchell2005).
Such features are particularly important for language learning (see also 4.3): without the ability to provide immediate feedback and affective responses, DLL tools will remain to be socially and emotionally distant to learners (and instructors). Unfortunately, existing ‘intelligent language tutors’ (ILTs) do not meet the standards yet (see Godwin-Jones, Reference Godwin-Jones, Chapelle and Sauro2017 for a review), particularly given ILT's current focuses on providing corrective feedback on writing or giving text-based evaluations (see Shadiev & Yang, Reference Shadiev and Yang2020). As an example, the popular VR software for L2 learning Mondly™ relies on a static stern-faced pedagogical agent responding to correct-vs.-wrong answers. Nevertheless, we see great potential in this domain given the significant advances in recent years in NLP (Hirschberg & Manning, Reference Hirschberg and Manning2015), automatic speech recognition (Golonka, Bowles, Frank, Richardson & Freynik, Reference Golonka, Bowles, Frank, Richardson and Freynik2014; Li, Deng, Haeb-Umbach & Gong, Reference Li, Deng, Haeb-Umbach and Gong2015), affective computing (D'Mello & Graesser, Reference D'Mello and Graesser2010; Picard, Reference Picard, Calvo, D'Mello, Gratch and Kappas2015), and deep learning neural networks (LeCun, Bengio & Hinton, Reference LeCun, Bengio and Hinton2015). For example, automatic voice recognition can be built into the system to assess the learner's pronunciation accuracy and provide real-time feedback to the learner, which is already being explored by some commercial products (e.g., Rosetta Stone). We predict that AI-based tools will be further improved in the next few years, and be readily incorporated into or interfaced with MALL, VR, and GBLL to expand the utility and power of DLL.
In summary, there exist many opportunities and promises in leveraging AI and big data to make DLL more effective and personalized when we integrate the properties of the learning context including those from the environment, the tutor, and the learner. This integration will in turn facilitate the application of AI and big data analytics for better pedagogical design and language education. DLL represents an exciting interdisciplinary field where technology interfaces with human studies, and where theories and practices from cognitive science, neuroscience, and educational technology converge.
6. Conclusions
Language learning has entered a new era of pervasive digital applications. In light of the rapid developments in technology-enhanced education and AI-inspired innovations, DLL has become an exemplary interdisciplinary area of study and a gateway connecting language science, the society, and the industry. In this article, we have charted an overall picture of what DLL has evolved into, what impacts it has created, and what future promises it may hold. We have also attempted to provide theoretical perspectives from psychology, education, linguistics, and neuroscience to understand the cognitive, social, affective, and neural dimensions of DLL. DLL has enormous potential given the new generations of ‘digital natives’ and the interests in digital applications and blended learning in the foreseeable future. But significant work remains to be done to understand the mechanisms under which DLL might simulate language learning in its natural, authentic context and consequently enhance its learning success. There are also significant gaps that exist between our academic knowledge of student learning and the industry's commercial product design. We need quick knowledge transfer from academia to the industry, which is currently hindered by many factors, including bureaucracies at different levels, and such problems are exacerbated by the different paces adopted by the academia versus the industry. To mend such gaps, we need the academics to work more closely with the industry and with policy makers, which will facilitate and accelerate the development of both knowledge discovery and knowledge transfer (see Luan et al., Reference Luan, Geczy, Lai, Gobert, Yang, Ogata, Baltes, Guerra, Li and Tsai2020 for a discussion). We hope that integration of the emerging technologies with the science of learning will allow us to address not only the theoretical and practical problems associated with second language learning, but also unpredictable and long-term challenges posed by disruptive societal events such as the Covid-19 pandemic.
Acknowledgments
Preparation of this article has been partially supported by a grant from the Hong Kong Research Grants Council (Project # PolyU15601520) and a Research Startup Fund from the Hong Kong Polytechnic University to PL, and grants from the Ministry of Science and Technology of Taiwan (#MOST110-2511-H-003-038-MY3 and #MOST 109-2511-H-003-026-) to YJL. The authors wish to express gratitude to Chan-yuan Gu, Zhexiao Guo, Jennifer Legault, Yingying Peng, Jing Wang, Jiayan Zhao and other members in the Brain, Language, and Computation Lab for their assistance in the relevant projects, to Hyeonjeong Jeong and Brian MacWhinney for their helpful discussions, and to Sean McMinn for his comments on an earlier draft of the article.