1. Introduction
‘Digital is changing the world’ has become a mantra in academic, industrial and policy-making circles. Digital technology has indeed brought changes and disruption to many industries worldwide, and ‘digital’ corporations have now climbed to the top of the league tables, as far as market capitalization is concerned.
So, if ‘digital’ is impacting the world, it is reasonably obvious that design is being affected too. This change is probably still in its infancy. In fact, the transformation brought by ‘digital’ product representations since the 1970–1980s (as can be found first in computer-aided design (CAD) and then in Product Lifecycle Management (PLM) systems) is likely to be nothing more than a preliminary step of a much deeper and broader transformation, which is still ongoing.
However, declaring that ‘digital has changed, is changing and will change the world of design’ risks to be rhetoric and to not help in addressing any problem. Such statements do not address what working on ‘digital design’ really means, either as practitioners, academics or policy-makers.
This paper aims to provide a conceptual framework of the current and emerging challenges brought by digitalization on design and to highlight key research questions that still ought to be addressed. We believe that these research questions could lead to a better understanding of a new paradigm and to develop sound and industrially relevant tools and methods.
From a methodological point of view, the research has followed a top-down approach. In particular, three main contributions (Porter & Heppelmann Reference Porter and Heppelmann2014; Iansiti & Lakhani Reference Iansiti and Lakhani2014; Bstieler et al. Reference Bstieler, Gruen, Akdeniz, Brick, Du, Guo, Khanlari, McIllroy, O'Hern and Yalcinkaya2018) have inspired the main arguments of the paper and provided the insights to structure it. In order to broaden the vision and address the topic of digitalization in design, an extensive and interdisciplinary literature review of around 100 papers in the fields of Management (Management Science, Organization Science and Decision Sciences), Innovation Management (e.g., Journal of Product Innovation Management, R&D Management and Technovation), Operations Management (e.g., Production Engineering; International Journal of Production Research, ASME Journal of Manufacturing Science and Engineering, The International Journal of Advanced Manufacturing Technology) and Engineering Design (Journal of Engineering Design, Research in Engineering Design, Design Studies, Design Science, ASME Journal of Mechanical Design; Computers in Industry, AIEDAM) has been conducted. Some papers from the Information Technology and the Data Science Domains (e.g., Advanced Engineering Informatics, IEEE Transactions on Knowledge and Data Engineering, IEEE Big Data, International Journal of Business Research and Information Technology) were specifically included in order to allow more in-depth insights on enabling technologies.
This survey of literature has led to identifying three main streams of research for engineering design, each of which bears consequences at different levels (the detailed documentation linking each paper to each stream and consequence will not be reported in the paper but is available on request):
-
(i) Stream 1: literature shows that digitalization affects designers both at the individual level and as part of a team, and, specifically, the ways by which they work (operative (OP) consequences) and interact with other units in their firms (organizational (ORG) consequences). Furthermore, this impact appears to be different when looking at supply-side data (i.e., the data that come from the production value chain) and demand-side data (i.e., data coming from customers);
-
(ii) Stream 2: literature shows that digitalization has consequences on the development process itself since digital and agile environments both enable and force design routines and processes to change. This has not only OP and ORG consequences but also managerial consequences since these new processes have to be set up and governed;
-
(iii) Stream 3: part of the literature works on new tools that can support data-driven design processes. In the design context, this trend means investigating the field of analytics for design, whose consequences are mainly operative.
The following Table 1 summarizes these three streams of research, their impact at different levels and the section of the paper where they will be discussed. In particular, the three different levels represent the distinct consequences of implementing technology, that are operational, managerial or strategic and organizational (Cantamessa et al. Reference Cantamessa, Montagna and Neirotti2012).
Table 1. The high-level relationship between the consequences of digitalization and the main streams of research on design
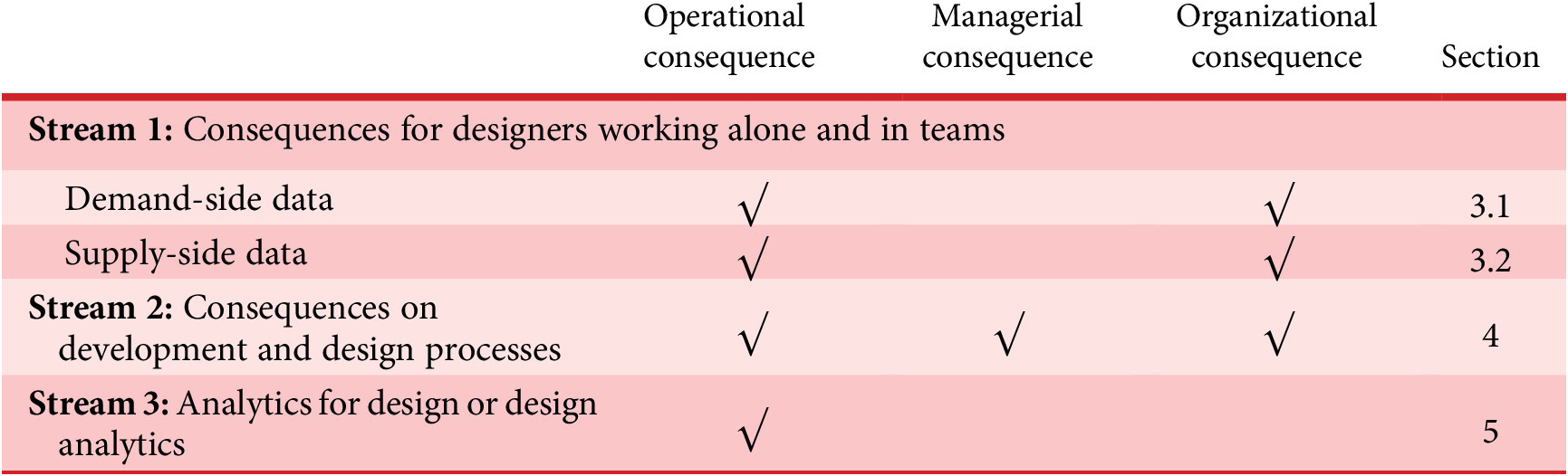
These OP, ORG and managerial consequences lead designers to operate in a world of growing complexity. To convey this complexity in clear terms, we will adopt a relational model, as first developed in Cantamessa (Reference Cantamessa2011) (see Figure 1), and adapt it to the emerging scenario.
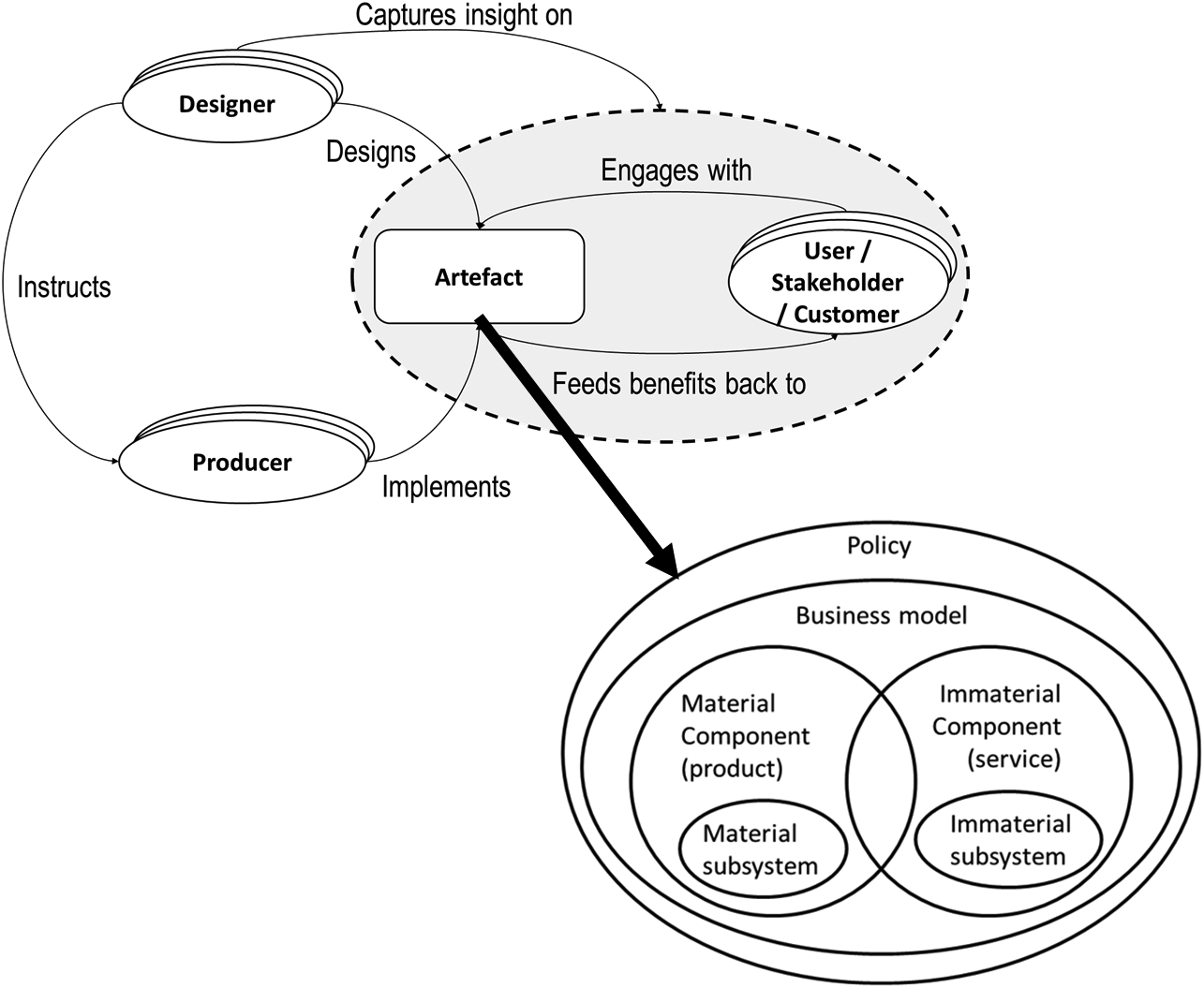
Figure 1. The relational model developed in Cantamessa (Reference Cantamessa2011).
The model, depicted in Figure 1 includes the main design paradigm elements in the 1990s. Designers were considered here either working alone or in a team and following a ‘customer-driven’ product development approach They were called to capture somehow insights about the interaction between artefacts and humans (which, depending on the role, could be users, external stakeholders or customers); based on these insights, they would aim at designing better products, at the same time, providing adequate information to the firms which would produce such artefacts. The concept of the artefact was at those times progressing beyond material ‘products’ and becoming broader, by also encompassing service components and business models. However, the approach to design was still strongly rooted in the tradition of designing physical goods.
The following discussion will adopt this relational model as a way to ‘track the changes in the design context’ (Cantamessa Reference Cantamessa2011) from the baseline situation to the emergent impact of digitalization. Compared to other macrolevel process models proposed by the literature (for a survey, see Wynn & Clarkson Reference Wynn and Clarkson2018), this representation allows to go beyond a description of the design process and shows the context in which it occurs, the actors involved and their mutual relationship. In the model, the differences brought by the emergence of ‘digital’ in designers’ activities and relations will be discussed and highlighted, looking at the supply-side and demand-side elements that have emerged (or are emerging) in recent years.
The paper is structured as follows. Section 2 will provide a concise framework description of the most relevant ‘digital’ technologies and of the way with which they are shaping industrial sectors in general. Then, Sections 3–5 will provide a detailed description of the three aforementioned streams of research and the associated research questions. The final section will attempt to draw a few conclusions on this emerging paradigm of design in the digital era.
The dissertation will develop mainly around durable goods industries. Durable goods indeed are among the most impacted by digital transformation. Contrary to disposable or soft goods, they allow a higher possibility of retrieving data and information directly from the field (something of that more complex systems, such as aeroplanes or power plants were already doing since a long time).
Examples from industry are generally provided throughout the paper to exemplify conceptual statements; however, a single case study has been predominantly chosen in order to have a common thread in the discussion. The case study is somewhat freely inspired by news and events reported from Tesla, the well-known battery-electric car producer. Tesla, in fact, easily lends itself as a poster example of transformations occurring in the design and innovation contexts following the emergence of ‘digital’ technologies. In fact, cars are the archetype of durable goods, and Tesla represents an emblem as it collects many data from everyday use and returns them to the user in the form of updates. Readers should be aware that the approach taken is not inductive since we are not using the Tesla case study as a source from which to derive the conceptual model. On the contrary, the conceptual model is built by elaborating results taken from literature, and news from Tesla are simply used as exemplifications of aspects of the model.
2. The enabling technologies of digital contexts are shaping a different world
The word ‘digital’ implies the computerization of previously ‘analogue’ products and processes. The computerization has indeed been occurring since the 1970s when computers progressively emerged and diffused. Computers have become both a new ‘digital’ product category and an enabler of a first ‘information revolution’. In the context of design, product information was traditionally relegated to ‘passive’ paper-based visualizations (i.e., a drawing could only be looked at but could not automatically lead to calculations or simulations) that were unrelated to each other (i.e., the drawings of an assembly and its constituent parts could not interoperate with one another). Thanks to that early wave of digitalization, product information migrated to digital models. This has allowed tremendous progress not only in terms of more convenience in generating and archiving product information but also because digital product information became a real ‘virtual product model’. For instance, digital models incorporated the structure and the history of the product and could support simulation under multiple perspectives (e.g., stress–strain analysis carried out with finite element methods, process analysis carried out with computer-aided manufacturing).
Nowadays, when using the word ‘digital’, the above-mentioned advances are further advanced by the following – and highly complementary – enabling fields of technology:
-
(i) Internet protocols and always faster and cheaper connectivity. In its many waves of innovation, the Internet started connecting people to contents through the World Wide Web; it then allowed unprecedented forms of communication and socialization among people through social networks. Nowadays, the Internet of Things (IoT) technology is leading to a continual connection and flow of data between people and objects and between objects and other objects;
-
(ii) Augmented and virtual reality, which allows richer representations of objects and environments, merging the real with the virtual, and vice-versa;
-
(iii) powerful and cheap IT equipment (processors, bandwidth and storage) which have enabled the virtualization of physical servers, leading to cloud computing and the possibility of storing Big Data;
-
(iv) Data mining can exploit the value hidden in massive amounts of heterogeneous data, by identifying patterns and behaviours in phenomena and occurring within systems, without requiring ex-ante theorizations;
-
(v) Machine learning allows the development of new forms of automation and decision-making, based on the re-elaboration and ‘digestion’ of large amounts of data.
These technological streams enable radical changes in products and services and beyond. Digitalization is, in fact, a paradigmatic change, in which not only the technological shift occurs but behavioural/social shifts in society can be observed, often accompanied by disruption into business models in industries and value chains. Table 2 describes the immediate effect brought by each technology, the ensuing impact on products and services and the consequences on both the side of demand (consumers and society in general) and supply (producers).
Table 2. Enabling technologies and their impacts
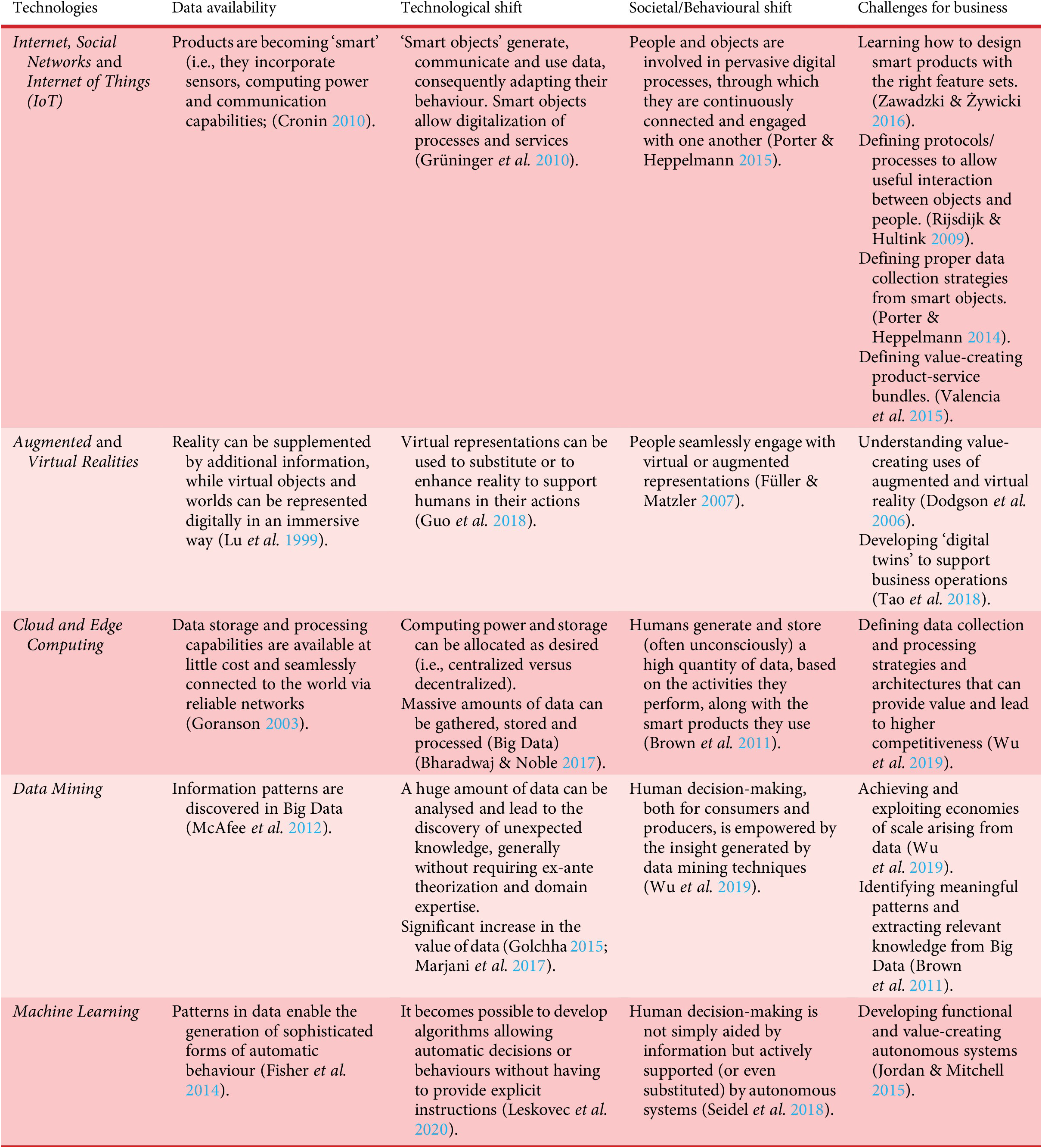
3. Stream 1: the OP and ORG consequences for designers
As shown in Table 1, the first stream looks at the impact of ‘digital’ on designers and their work, which, on the one side, includes the activities they have to carry out and the tools they can use (OP) and, on the other side, the way they operate within organizations (ORG).
Central to this discussion is the role of data. These are here classified for the analysis purposes into demand-side and supply-side since any technological paradigm ideally is fostered by supply and demand-side elements (Dosi Reference Dosi1982). In particular, data can derive both from the field (‘demand-side data’) and the side of the production/distribution value chain (‘supply-side data’). Both demand-side (D) and supply-side (S) data have OP and ORG consequences. To track these consequences throughout the paper, we will associate a ‘code’ to each consequence/challenge, by combining these labels (i.e., D-OPi represents the i-th operational consequence due to demand-side data).
3.1. Demand-side data
Demand-side data are gathered from customers. They are mainly collected by recording customer purchasing behaviour, through the observation of individual choices (Lesser et al. Reference Lesser, Mundel and Wiecha2000) as well as from stakeholders (Cantamessa et al. Reference Cantamessa, Montagna and Cascini2016) and, nowadays and increasingly in the future, from the actual use of smart products or digital services (Kagermann Reference Kagermann, Albach, Meffert, Pinkwart and Reichwald2015; Bharadwaj & Noble Reference Bharadwaj and Noble2017; Li et al. Reference Li, Roy and Saltz2019) or even from the sheer presence of smart products in the environment (e.g., a smart speaker picking up conversations). Walmart, for instance, gathers more than 2.5 petabytes of data every hour from its customer transactions (McAfee et al. Reference McAfee and Brynjolfsson2012), and Facebook or LinkedIn collect data to suggest new personal contacts (Davenport et al. Reference Davenport, Barth and Bean2012). GE has become the manufacturing industry leader in managing customer product data, to the point where it is becoming the most known developers of service offerings based on Big Data (Davenport & Dyché Reference Davenport and Dyché2013). Tesla, similarly, collects more data than most car companies and varies from vehicle’s location, car’s settings and short video clips from the car’s external cameras (Muller Reference Muller2019).
Basically, the idea is that demand-side data will allow companies to understand user needs fully. Not only companies will be able to have a real, and not simply estimated, understanding of mission profiles, but they will also be able to adapt continuously, based on market stimuli. This adaptation might consist of newly added functions identified through the analysis of data generated by product already launched (Lewis & Van Horn Reference Lewis and Van Horn2013), up to a real-time adjustment of the offering to address the evolving customer needs (Brown et al. Reference Brown, Chui and Manyika2011) and self-customized products (Porter & Heppelmann Reference Porter and Heppelmann2014). The simultaneous adaptation is to be intended as the possibility by designers to continuously update product design and consequently product itself and have operational and organizational consequences for designers:
The first operational change regards the identification of the customer segments whose needs must be addressed (Canhoto et al. Reference Canhoto, Clark and Fennemore2013; Roblek et al. Reference Roblek, Meško and Krapež2016) (D-OP1).
In a context of mature product and services, a firm usually segments the market statically, using dimensions such as demographics, usage patterns, and so on, while products are still to be diffused, firms are used to distinguishing between the segments proposed by Rogers (i.e., from innovators to laggards, Rogers Reference Rogers1962). As a result, designers are used to derive the priorities of each customer segment at each step (Canada Reference Canada, Mortensen and Patnaik2010), and any interaction with customers took place when a new segment had to be addressed.
Nowadays, two changes are occurring through data gathered directly from product: (1) customization, or even personalization, is leading to consider each customer as a ‘segment-of-one’ and as a ‘new need every time’ (Canhoto et al. Reference Canhoto, Clark and Fennemore2013); (2) companies are truly increasingly turning towards continuous and real-time interactions with customers so that designers must keep abreast with the evolving needs not only of those who have already adopted but also of those who will adopt (Roblek et al. Reference Roblek, Meško and Krapež2016). In the first case, beyond the possibilities of approaching closer to each customer, it is possible to reach customers that belong also to the tails of the sales distribution curves. Those customers have needs that are different from the ‘standard’ ones, and fitting in niche markets (Chen et al. Reference Chen, Chiang and Storey2012; Ma & Kim Reference Ma and Kim2016) can provide new needs to be addressed. In the second case, the continuous collection of upcoming needs from products already in the field both when they are ‘sold’ and – even more – when they are ‘provided as a service’, implies the redesign of products, simultaneously considering the upcoming customers who have not adopted yet and have to be acquired, as well as the existing customers, who must be kept loyal.
Tesla Model S is an example of how even the hardware of the car has been designed to offer the maximum flexibility to the changing customers’ needs, starting from a control panel that allows changes in the interface and functionalities. Those needs are the ones that emerge from the feedbacks of customers but are also deduced by the uses that car owners make of their vehicles (Lyyra & Koskinen Reference Lyyra and Koskinen2016).
The second change concerns the almost simultaneous elicitation and satisfaction of customer needs together with the validation of the corresponding product/service performance in each product development iteration (Montagna & Cantamessa Reference Montagna and Cantamessa2019) (D-OP2).
Traditionally, the management of customer requirements involves an iterative procedure to elicit requirements by users, interpret them and, finally, define product specifications (Jiao & Chen Reference Jiao and Chen2006). After that, in the postlaunch reinnovation phase, customer satisfaction is usually assessed to redesign the product (Rothwell & Gardiner Reference Rothwell and Gardiner1985). Nowadays, in agile contexts, companies continuously gather data from users after the commercial deployment of the product and use them as a basis for continuous technical improvement (Ülkü et al. Reference Ülkü, Dimofte and Schmidt2012). In more and more industries, starting from digitalized services, product-development iteration simultaneously incorporates the elicitation and satisfaction of customer needs and the validation of corresponding performance (Montagna & Cantamessa Reference Montagna and Cantamessa2019). Hence, testing and validation no longer follow the conception of the product but coexist in a continuous circle. Although today it is not possible to find this continuous circle of elicitation, satisfaction and validation in every sector, this is the direction that can be hypothesized.
For instance, as if it were producing a ‘mobile phone on wheels’, Tesla Motors exploit its data collection system connected to Tesla HQ in order to understand software bugs and errors and to release updates which can improve car performances (Walker Reference Walker2015). In 2013, for instance, three Tesla Model S vehicles caught fire because of suspension problems (Bullis Reference Bullis2014) and – instead of starting a very costly vehicle recall process – Tesla simply sent out an over-the-air (OTA) update to change the suspension settings, giving the car more clearance at high speeds.
A third operational change is linked to the possibility of having derivative innovations (Yoo et al. Reference Yoo, Boland, Lyytinen and Majchrzak2012) or more generally, the chance that designers discover implications which were not anticipated by the initial design (Gawer Reference Gawer2010) (D-OP3).
Basically, the ability to collect new needs determines new features that were not conceived initially and therefore new functions and behaviours to be designed (Van Horn & Lewis Reference Van Horn and Lewis2015; Bstieler et al. Reference Bstieler, Gruen, Akdeniz, Brick, Du, Guo, Khanlari, McIllroy, O'Hern and Yalcinkaya2018; Wang et al. Reference Wang, Sha, Huang, Contractor, Fu and Chen2018). This phenomenon is already known for socio-technical systems that usually evolve over time (Geels & Kemp Reference Geels and Kemp2007). The diffusion of Facebook in developing countries, for instance, involved several technical problems, since it was not originally designed to be supported by poor communication infrastructures. A new set of requirements and solutions was required to be developed since the access to the Internet, and the quality of the connections entailed for a ‘softer’ version of the standard application (Wyche et al. Reference Wyche, Lampe, Rangaswamy, Peters, Monroy-Hernández and Antin2014).
The ability to identify unanticipated implications can also avert or limit negative consequences in case of product defects or technological immaturity. For instance, significant diffusion of autonomous vehicles may entail significant negative impacts and externalities, and continuous and detailed observation of products operating in the field may allow firms to manage them in a timely and appropriate way.
Finally, a fourth change may involve the automation of the innovation process or at least parts of it (Bstieler et al. Reference Bstieler, Gruen, Akdeniz, Brick, Du, Guo, Khanlari, McIllroy, O'Hern and Yalcinkaya2018) (D-OP4).
Since the 1990s, a variety of efforts have been made to automate portions of the design process, for instance in idea generation (e.g., Wang et al. Reference Wang, Rao and Zhou1995; Seidel et al. Reference Seidel, Berente, Lindberg, Lyytinen and Nickerson2018). Marketing departments, among business functions, have intrinsically been spending generalized efforts in updating data collection processes from the field, and digitalization has fostered such an approach. The presence of such large amounts of data, simultaneously to the computational capability and pervasive use of computers in design (Anderson et al. Reference Anderson, Perez, Xuereb, Otto and Wood2018), is making nowadays the efforts for design automation closer to fruition. In particular, some attempts in the SW domain exist (Clarke Reference Clarke2018). Recently, Elon Musk has affirmed that ‘computers will be able to do anything a human can by 2030–2040’ (Lant Reference Lant2017). However, these possibilities are still to be explored because while some examples exist in design optimization or prototyping, it is yet to be understood whether the early, more creative and less routinary design phases may be automated and to what extent (Kwon et al. Reference Kwon, Park and Geum2018).
All these elements have consequences not only on operational processes but also on the interactions that design teams have with the other functions and departments of the firm.
A fifth change, therefore, concerns the integration of IT, marketing and NPD functions (Bstieler et al. Reference Bstieler, Gruen, Akdeniz, Brick, Du, Guo, Khanlari, McIllroy, O'Hern and Yalcinkaya2018) (D-ORG1). Various authors have shown that marketers and designers need to cooperate closely (Cooper & Kleinschmidt Reference Cooper and Kleinschmidt1986; Moore Geoffrey Reference Moore1995; McQuarrie Reference McQuarrie2014), and that information technology (IT) should be integrated into the organization at various levels (Ward & Zhou Reference Ward and Zhou2006; Barczak et al. Reference Barczak, Hultink and Sultan2008). This collaboration between marketing, the IT department and the design function is nowadays even more crucial (Bstieler et al. Reference Bstieler, Gruen, Akdeniz, Brick, Du, Guo, Khanlari, McIllroy, O'Hern and Yalcinkaya2018) if one wishes to share, structure and interpret continuously updated data throughout the product development process (Urbinati et al. Reference Urbinati, Chiaroni, Chiesa and Frattini2018). The novelty is that this collaboration rests on the ability to analyse vast and rich amounts of continuously gathered data, which very probably will require the constant support of the new professional role of data analysts (refer to Figure 1). This ongoing collaboration makes thus necessary to define and formalize the roles and competencies that designers, marketers and data analysts should have with one another.
Finally, the sixth change leads to reshaping the relationships between customers and manufacturers (Nedelcu et al. Reference Nedelcu2013; Espejo & Dominici Reference Espejo and Dominici2017) (D-ORG2). Indeed, supplying goods to previously targeted consumers has become outdated (Dominici Reference Dominici2009); consumers do not just passively buy products, they increasingly participate in the process of value creation, even by unconsciously transmitting data on their usage (Espejo & Dominici Reference Espejo and Dominici2017), at least for maintenance planning (Tao et al. Reference Tao, Cheng, Qi, Zhang, Zhang and Sui2018). Tesla, again, has created digital twins of its products to monitor their real-time use. Each car is equipped so to communicate constantly with its virtual twin located on a Tesla server, to detect product failures or suggest timely maintenance interventions (Schleich et al. Reference Schleich, Anwer, Mathieu and Wartzack2017).
In other cases, product failures and degradation patterns (e.g., material ageing mechanisms) are not only useful for preventive and predictive maintenance but also for the definition of other product mission profiles (Aggarwal & Abdelzaher Reference Aggarwal and Abdelzaher2013). Customers, therefore, become producer–consumers or ‘prosumers’ (Nedelcu et al. Reference Nedelcu2013). The relationship between customers and manufacturers therefore changes, and the ownership of this process is to be understood, whether it should be managed by marketing, design teams or even automated outright.
In light of what has just been described, the corresponding demand-side evolution of the baseline model is represented in Figure 2. The main shift is represented by the presence of data that are not only systematically, but also continuously, generated, collected and analysed. These data are collected by direct, continuous and real-time observation of interactions between customers in different market segments and the artefact (Figure 2b). This is clearly different from the traditional methods for customer observation and elicitation of customer needs typical of the previous paradigm, depicted in Figure 2a. Data become a focal element in the new design paradigm, leading to the new figure of the data analyst. Data analysts work closely with designers and other business functions, such as the marketing department, eventually allowing a tight integration between them. Data analysts and designers are intended here both as working alone and in teams, both in the sense of the work they carry out and of the competencies they must possess.

Figure 2. The shift from the old (a) paradigm to the new demand-side one (b).
These operational and organizational consequences give rise to some key research questions to be answered as shown in Table 3.
Table 3. Operational and organizational consequences of demand-side data availability

3.2. Supply-side data
In addition to demand-side data, companies collect reams of useful data from their production systems and operating environments. Data can be generated from a multitude of sources, such as production machinery, supply chain management systems and monitoring systems (Noor Reference Noor2013; Ma et al. Reference Ma, Chu, Lyu and Xue2017; Ghobakhloo Reference Ghobakhloo2020), also using systemic approaches (Alfieri et al. Reference Alfieri, Cantamessa and Montagna2012). The massive data generation and their systematic collection have been even more stressed with the revolution in production systems brought by Industry 4.0 actions.
Data from these sources are often collected and analysed with a focus on opportunities for reducing cost and increasing efficiency. Smart manufacturing, for instance, is often conceived as an environment in which a new wave of electronic sensors, microprocessors and other components allows a high degree of automation (Noor Reference Noor2013) and provides real-time warnings about potential problems or opportunities for cost reduction (Jenab et al. Reference Jenab, Staub, Moslehpour and Wu2019).
However, the effects of digitization of production processes can lead even to broader benefits, especially if this information is fed early in the development process (Schuh et al. Reference Schuh, Rudolf and Riesener2016). Tesla Model 3, for instance, turned out to be particularly critical during the assembly phase, due to its many weld points and rivets that were not suitable for heavy production automation (Welch Reference Welch2018). Data from manufacturing, in that case, provided information useful for industrialization, suggesting product architecture alternatives in view of the consequences on assembling.
The first operational challenge posed by supply-side data concerns the need of having integrated information coming from different domains, such as design, manufacturing and after-sales services (Schuh et al. Reference Schuh, Rozenfeld, Assmus and Zancul2008) (S-OP1).
These data traditionally are collected by companies through their ERP, MES and PLM systems. However, these systems are rarely integrated, and this prevents firms from having a unified view of this data and a rigorous approach to data collection (Asmae et al. Reference Asmae, Souhail, El Moukhtar and Hussein2017; Lanz & Tuokko Reference Lanz and Tuokko2017; Singh, Misra & Chan Reference Singh, Misra and Chan2020). Especially in SMEs, interoperability and data exchange issues among system architectures still provide consistency matters (Telukdarie & Sishi Reference Telukdarie and Sishi2018). For instance, product architectures and production bills of material are scantly put in relation (Altavilla & Montagna Reference Altavilla and Montagna2019; Behera et al. Reference Behera, McKay, Earl, Chau, Robinson, de Pennington and Hogg2019), current systems often fail in tracking data changes (Li et al. Reference Li, Tao, Cheng and Zhao2015) and the granularity level and detail of the information are often not shared among systems to ensure satisfactory interoperability (Cheung et al. Reference Cheung, Maropoulos and Matthews2010).
In order to disentangle this situation, firms will have to proceed to define common conceptual models and shared procedures and frameworks for data collection (Sajadfar & Ma Reference Sajadfar and Ma2015). It is no coincidence that Tesla has chosen to develop a customized ERP in-house; a highly vertically integrated environment, in fact, cannot be cut by the standard solutions provided for the automotive sector. The common use of a single system not only has avoided the need for custom adaptations to other standard systems but also has provided the common views of the data, required to ensure interoperability (Clark Reference Clark2013; Alghalith Reference Alghalith2018). In particular, unified feature-based representations of products, which contemporaneously relate to functional geometry and manufacturability, can be generated and can be used to manipulate and evaluate part designs, according to part family information, multiple period production plans and market demand fluctuations (Lee & Saitou Reference Lee and Saitou2011).
Scattered data represent a problem not only within the firm but also by firms operating in the value chain; data must be bundled and unbundled according to the necessity of the analysis carried on at each product lifecycle stage (Altavilla & Montagna Reference Altavilla and Montagna2019). The challenge for companies again lies in developing such kind of common frameworks, and while some companies are still struggling to embrace the culture of PLM, the information system designed by Tesla again is able to face the challenge. Tesla system connects not only with standard PLM tools (specifically, in 2010, Tesla has also adopted Dassault System Catia V5 and Enovia V6 solutions) but also includes its world-class e-commerce system (Clark Reference Clark2013; Alghalith Reference Alghalith2018).
Once collected, the supply-side data come from diverse data sources and in highly various formats. Therefore, such digital contexts stress the operational requirement of managing such diversity in extensive volumes of data (Li et al. Reference Li, Tao, Cheng and Zhao2015; Trunzer et al. Reference Trunzer, Calà, Leitã, Gepp, Kinghorst, Lüder, Schauerte, Reifferscheid and Vogel-Heuser2019) (S-OP2).
Other data sources of data which require appropriate management include virtual reality, which has been employed since many years by designers to better visualize structure testing and verify assembly and maintenance processes (Sivanathan et al. Reference Sivanathan, Ritchie and Lim2017), as well as rapid prototyping, which has demonstrated its effectiveness in the context of product design activities (Schuh et al. Reference Schuh, Rudolf and Riesener2016). These systems have nowadays begun to impose further requirements for data collection (Seth et al. Reference Seth, Vance and Oliver2011) and the need to avoid lack of convergence between the physical and the digital domains (Tao et al. Reference Tao, Cheng, Qi, Zhang, Zhang and Sui2018). The risk of inconsistency is inflated if the datum does not derive only from testing or verifying activities, which remain ‘local’ to the product development process, but it is constantly generated – and in large quantity – by digital twins (e.g., from robotic systems during production or in digital format from maintenance crews) (Mejía-Gutiérrez & Carvajal-Arango Reference Mejía-Gutiérrez and Carvajal-Arango2017). Tesla has defined specific partnerships (e.g., with PTC) to ensure data consistency on its product models (Schleich et al. Reference Schleich, Anwer, Mathieu and Wartzack2017), and the attention of Tesla to data consistency of its platforms is easily proved by the number of positions opened for database engineers worldwide in each of its business areas.
Finally, even if one were able to guarantee interoperability between the systems, coherence and defragmentation of the data, regardless of the source and the format, the effective use of all these data still remains, also in a digital context, a significant operational problem for designers (Zhan et al. Reference Zhan, Tan, Li and Tse2018) (S-OP3), because of a number of reasons.
First, companies already own a large amount of data which could be used to support decisions (Wu et al. Reference Wu, Zhu, Wu and Ding2013) but do not always even know what data are already available in their database and what data they are producing (Altavilla et al. Reference Altavilla, Montagna and Newnes2017; Arnarsson et al. Reference Arnarsson, Gustavsson, Malmqvist and Jirstrand2018). Second, designers often still do not know how to make the best use of these data (Arnarsson et al. Reference Arnarsson, Gustavsson, Malmqvist and Jirstrand2018). Third, the magnitude of the phenomenon could force the use of AI algorithms but the potential of these algorithms is still not clear, especially when it comes to embedding the necessary knowledge from different technical domains (Dougherty & Dunne Reference Dougherty and Dunne2012).
At the moment, apart from design optimization and prototyping, it is not clear if AI algorithms will be able to interpret performance and functional patterns correctly and what type of contribution they can provide to designers. For instance, doubts have been raised with respect to their ability to generate feasible technical alternatives either on their own or by interpreting the intentions expressed by designers (Noor Reference Noor2017). This adds – this type from supply-side data – to the previously mentioned problem of design automation (Bstieler et al. Reference Bstieler, Gruen, Akdeniz, Brick, Du, Guo, Khanlari, McIllroy, O'Hern and Yalcinkaya2018; Seidel et al. Reference Seidel, Berente, Lindberg, Lyytinen and Nickerson2018) (S-OP4).
Concerning the organizational consequences, the main effect that can be identified relates to the procrastination of collaboration between Development, IT and the other operational departments (Agostini & Filippini Reference Agostini and Filippini2019) (S-ORG1).
The data integration requirements force, today more than ever, functional integration among other operational departments, such as production, maintenance, logistics, etc. (Liao et al. Reference Liao, Chen and Deng2010). If Industry 4.0 actions have equipped production plants and operative processes with sensors and devices able to collect real-time data on process execution and companies are willing to use them not only to solve efficiency issues but also to feed design processes, the analysis of such a massive quantity of data and the interpretation of the evidences will require full functional integration, cross-domain knowledge and new competencies and skills (Agostini & Filippini Reference Agostini and Filippini2019). Also, in this case, Tesla can provide an indication. Tesla initially did not hire expert engineers from the automotive field to avoid fixation on traditional architectures and competence biases. However, it is possible that some erroneous design choices could have been prevented with proper specific knowledge (Welch Reference Welch2018). To what extent can a firm do without specific and sector knowledge? So, given that designers with prior industry experience are likely to be involved, they will be required to enrich their competencies on other technical domains, to stress collaborations with colleagues from other specialist technical domains, as well as to develop digital skills to be able to interpret the evidences arising from data analyses. Moreover, they will have to be supported by data analysts that, at their turn, have to acquire the main principles of engineering design, as well as some fundamental knowledge from the specific technical field. It is difficult to conceive such a transformation of competencies in the short term. Moreover, one should wonder who – in organizational terms – should ‘own’ (i.e., be responsible for) such data-driven processes between designers, production engineers, industrialization teams or data analysts.
Figure 3b, therefore, depicts a further step into the new design paradigm, compared to the baseline model (Figure 3a). It includes the role of the supply flow of information, which is especially relevant to the producer and the designer (represented with the long dash-dot line). The centrality of the data becomes dominant. IT systems must be adequate to accommodate real-time information from the multiple systems associated with the various operating processes. The continuous and massive flow of data must be integrated and made reliable in order to be used effectively by the data science methods and tools and the results delivered to support design activities. Designers, producers and data analysts will have to work together, mutually integrating their knowledge and competences. Moreover, the producers, operate together in a group with assemblers and distributors (and coherently are represented as joined in the figure), surpassing the idea of a linear supply chain.

Figure 3. The shift from the old (a) paradigm to the new supply-side one (b).
The research questions linked to the changes described above are summarized in Table 4.
Table 4. Operational and organizational consequences of supply-side data availability
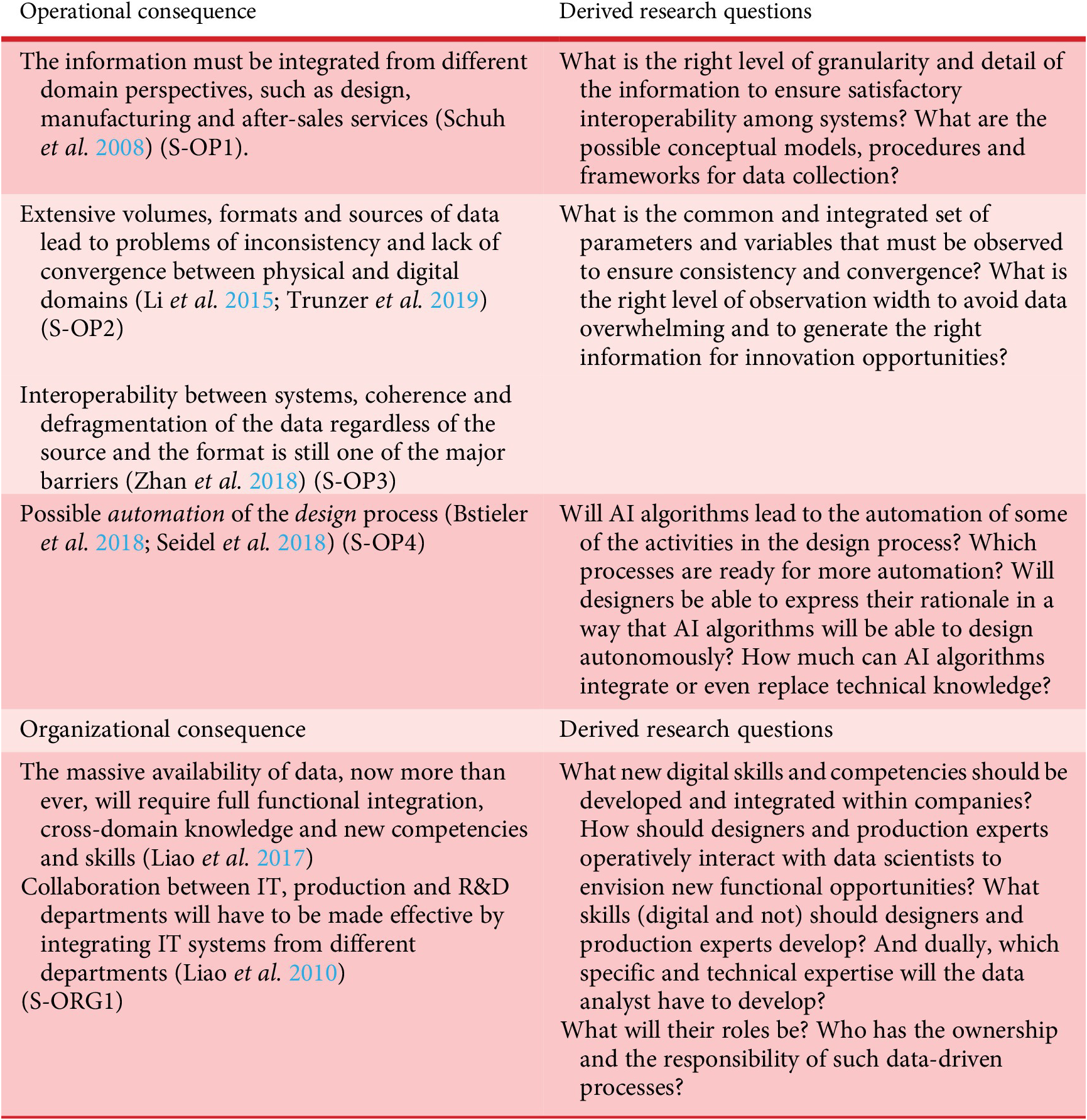
4. Stream 2: the specific consequences on development and design processes
Digitalization and its enabling technologies additionally have impacts on product development (NPD), as process itself. First, because the product development process is changing in its nature. The traditional separation between ex-ante product development and ex-post product use does not exist anymore (Montagna & Cantamessa Reference Montagna and Cantamessa2019). Second, because digital technologies themselves and new business models (i.e., the ones described in Section 2 and listed in Table 5) impose new guidelines for design (i.e., design pilots as named by Hermann Reference Hermann, Pentek and Otto2016): Interoperability, virtualization, decentralization, real-time capability, service orientation and modularity.
Table 5. The new design pilots imposed by technologies and business models

These new design pilots lead again to operative (NPD-OP) and organizational (NPD-ORG) changes. Additionally, a further consequence emerges in relation to the management of the process (NPD-MAN). These consequences are described in columns 2–4 in Table 6.
Table 6. Operational, organizational and managerial consequences on development and design processes

From an operative and process point of view, since companies will continuously adapt their value proposition, changing in some cases their business model (Trimi & Berbegal-Mirabent Reference Trimi and Berbegal-Mirabent2012), it becomes impossible – but also irrelevant – to develop a reliable and complete set of product/service specifications (Gunasekaran et al. Reference Gunasekaran, Subramanian and Ngai2019) (NPD-OP1). Designers will have to design an initial version of the product (i.e., ‘seed design’), similarly to the concept of ‘minimum viable product’, as a basis for further product improvements and extensions (NPD-MAN1). The subsequent iterations raise the need for iterative validation steps, and one may wonder to whom the responsibility and authority in deciding on these iterations should be assigned, whether to designers, marketing people or data analysts (Song Reference Song2017) (NPD-ORG1). In a way, this leads to a reissue (but also an amplification) of the problems in project management already posed by concurrent engineering practices (Krishnan et al. Reference Krishnan, Eppinger and Whitney1997) (NPD-MAN2). Activities and teams, in fact, must be managed in concurrency with an increasingly frequent exchange of information between the demand and supply-side information. Flexibility opportunities are constrained by project technical uncertainty and by the sensitivity of downstream activities, while coordination complexity is inflated for highly complex projects or when multiple parallel projects are ongoing. Attempts in this regard have been made, by integrating the Stage-Gate approach to agile principles (Ahmed-Kristensen & Daalhuizen Reference Ahmed-Kristensen and Daalhuizen2015): in particular, stage-gate modalities are used to handle high-level requirements and project progress, while the agile elements are adopted to drive development, to support teams in validation and to find more conveniently design failures (Ahmed-Kristensen & Faria Reference Ahmed-Kristensen and Faria2018). Finally, new contractual and administrative problems could arise. Indeed, without a given and fixed set of specifications, producers cannot draft a legal document describing their product but they have to keep changing and updating it, and certification processes are all to be reviewed coherently (Magnusson & Lakemond Reference Magnusson and Lakemond2017; Song Reference Song2017) (NPD-MAN3).
In order to support the development of continuously improved products and services, design modularity and platforms become key enablers, and it is not by chance that digital technologies are facilitating their renewed use in design (Porter & Heppelmann Reference Porter and Heppelmann2014; Rossit et al. Reference Rossit, Tohmé and Frutos2019) (NPD-OP2). Modularity and design platforms, now more than ever, in fact, are becoming fundamental given the multiplicity of needs led by product customization and personalization that are stressed and enabled by digitalization (Mourtzis & Doukas Reference Mourtzis and Doukas2014). The development of combinatorial innovations is instead activated, thanks to these operative design practices (Yoo et al. Reference Yoo, Boland, Lyytinen and Majchrzak2012; Marion et al. Reference Marion, Meyer and Barczak2015) (NPD-OP3). Tesla again confirms this tendency: an end-to-end workflow system (the 3DX system), heavily based on platform design, supported the ramp-up for the Model 3 and Tesla Energy in 2016.
Platform design has its counterpart and entails costs. Companies face the issue of understanding the trade-off between cost, production constraints and openness and flexibility of the product architecture (Krause et al. Reference Krause, Gebhardt, Greve, Oltmann, Schwenke and Spallek2017) (NPD_OP4) that has strategic and managerial consequences, such as significant economies of scale and lower development cost (Simpson et al. Reference Simpson, Maier and Mistree1999) (NPD_MAN4). Moreover, besides deciding which product components should be shared and which not, companies have to choose which layers of the platform they will permit other firms to extend (Yoo et al. Reference Yoo, Boland, Lyytinen and Majchrzak2012) and therefore they have to decide on vertical integration at the organization level (Cantamessa & Montagna Reference Cantamessa and Montagna2016) (NPD-ORG2).
Moreover, digitalization generates further complexity in designing and integrating digital technologies in the product (Jung & Stolterman Reference Jung and Stolterman2011). This is the problem of facing ‘design affordance’ for features and functionalities of a digital product (i.e., the problem of digital affordance seen in Oxman Reference Oxman2006; Yoo et al. Reference Yoo, Boland, Lyytinen and Majchrzak2012), which revolutionizes the traditional approach to design, based on a relatively rigid mapping between functions, behaviours and structure (Gero & Kannengiesser Reference Gero and Kannengiesser2004).
Traditionally, the function of a product was a driver for its structural and shape characteristics. The function and the component trees were coupled entirely between themselves and with respect to system behaviours so that once the set of functions was defined, this was linked to the set of the behaviours and components (that were traditionally HW by nature). The presence of digital components and the reprogrammability of digital technologies, in particular, enable instead the addition of new behaviours (not properly functions) after the product has been designed, produced and sold. This implies that an SW platform but consequently also the structure and the physical parts, must be ex-ante enabled, so to accept ex-post behaviours. It may even happen that new components, specifically SW, but not necessarily, can be ex-post added to enable behaviours, inducing new physical features (i.e., forms) that were previously unimagined. The binding of form, behaviour and function is procrastinated, and facing digital affordance requires designers to decouple ‘form’ from ‘function’ (Autio et al. Reference Autio, Nambisan, Thomas and Wright2018) (NPD-OP5). Product development managers have to control a process in which new features and behaviours are added even after the product has been designed, produced and already is in operation. An example of this is the aforementioned software update system of Tesla that has been used by the company over the years to address problems and enhance performances of the vehicles ex-post when the product was already on the market. For instance, in 2013, Tesla sent out an OTA update to change the suspension settings, giving the car more clearance at high speeds (Bullis Reference Bullis2014).
This situation may represent a means to encourage and support unpredictability in innovation processes (Austin et al. Reference Austin, Devin and Sullivan2012) (NPD-MAN5), and it is necessary to understand how to control and support creativity and serendipity behaviours in such frequently changing processes (Andriani & Cattani Reference Andriani and Cattani2016) (NPD-ORG3).
The last effect regards a further shift in design information and knowledge management. Years ago, product information was relegated to ‘passive’ visualizations and representations (i.e., a drawing on paper, which could be observed, but not operated upon with calculations or simulations), unrelated to each other. Then, digitization processes (CAD systems and simulations) through modelling helped explicate design knowledge-making codifiable what was previously done by intuition and experience (by storing design choices and verification activities through validated parameters and variable values). Paradoxically nowadays instead, the progressive transition to design automation is having the opposite effect, reporting tacitly the knowledge that a designer can afford the luxury of not having anymore, thus attributing to systems an active role in design processes. The implications of such a shift have not a secondary effect since the knowledge of individuals and the one incorporated into the organization are different. It is a matter of the individual, the team in which he works and the relationship of his/her competences within the knowledge base of the organization. If support systems automatically act for the designer and learn, they incorporate that knowledge that was previously of the individual or the design team; that knowledge, therefore, passes from the individual or team to knowledge base of the organization. Through automation, knowledge passes from its explicit to tacit form, changing the process rules and organization equilibria again since it moves from individuals to capital (NPD-ORG4).
Figure 4 adds to the baseline the specific effects of the new design pilots. Differently from the old paradigm, designers do not design the complete set of an artefact specification but start from a ‘seed design’, which will be iteratively improved and extended in a continuous platform-driven development process, where the design of form, behaviour and function may be procrastinated.
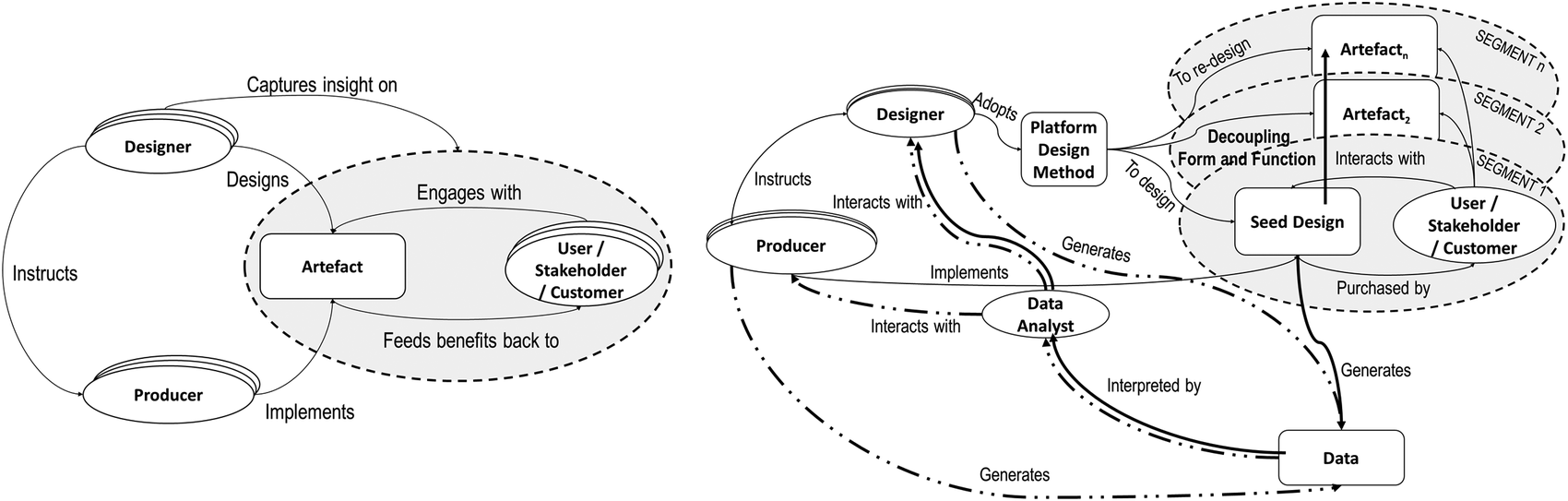
Figure 4. The shift from the old (a) paradigm to the new one (b) considering the specific consequences on development and design processes.
As a result of these changes, it is possible again to express further research questions in the last column of Table 6.
5. Stream 3: analytics for design or design analytics
The last research stream focusses on a new field, which is known as design analytics (Van Horn et al. Reference Van Horn, Olewnik and Lewis2013) and regards the processes and tools supporting the transformation of design-related data to suit design decision-making processes. Design analytics, in particular, is the process of inspecting, cleaning, transforming and modelling data to extract knowledge, which could be valuable to generate and evaluate new design solutions (King et al. Reference King, Lyu and Yang2013).
In general, data mining techniques (cluster analysis, conjoint analysis, etc.) and AI tools (e.g., neural networks and genetic algorithms, etc.) are rather well-established and widely applied to design problems (Tao et al. Reference Tao, Cheng, Qi, Zhang, Zhang and Sui2018; Tan et al. Reference Tan, Steinbach and Kumar2019). Among the AI tools, machine learning algorithms (mainly classified in supervized and unsupervized algorithms) are, instead, at their first attempts (for a review, Kotsiantis et al. Reference Kotsiantis, Zaharakis and Pintelas2007; Leskovec et al. Reference Leskovec, Rajaraman and Ullman2020). They are used both with descriptive and predictive purposes, and there are many examples of application along with the different phases of product development.
Cluster analysis, for instance, has been employed with a descriptive purpose both during the planning phase, to distinguish between potential and target customer from a large dataset (Tao et al. Reference Tao, Cheng, Qi, Zhang, Zhang and Sui2018), and during requirement elicitation (Zhang et al. Reference Zhang, Kwon, Kramer, Kim and Agogino2017; Shimomura et al. Reference Shimomura, Nemoto, Ishii and Nakamura2018). Conjoint analysis has been used, instead, in defining customer preferences and suggesting the possible functions and performances of a new design solution (Song & Kusiak Reference Song and Kusiak2009).
Prescriptive and predictive analytics often make use of AI techniques. Multiple response surfaces methodology (Jun & Suh Reference Jun and Suh2008), ordinal logistical regression (Demirtas et al. Reference Demirtas, Anagun and Koksal2009) and genetic algorithms (Hsiao & Tsai Reference Hsiao and Tsai2005; Kim & Cho Reference Kim and Cho2005) have been attempted for instance to determine the optimal settings of the design attributes that achieve maximum customer satisfaction. Case-based and neural network approaches have been used extensively during idea generation, either for leveraging decisions on previous design cases (Hu et al. Reference Hu, Ma, Feng and Peng2017) or for simulating design alternatives concerning specific performance parameters (Dering & Tucker Reference Dering and Tucker2017; As et al. Reference As, Pal and Basu2018; Babutzka et al. Reference Babutzka, Bortz, Dinges, Foltin, Hajnal, Schultze and Weiss2019). Optimization tools have been employed mainly with predictive purposes during the detail design phase. The aim, in this case, is to foresee the impact of a design change at the subsystem level into the overall system performances (e.g., Yao et al. Reference Yao, Moon and Bi2017) or design optimization (Quintana-Amate et al. Reference Quintana-Amate, Bermell-Garcia, Balcazar and Tiwari2015).
The use of design analytics techniques within the product development process also leads to operational consequences (DA-OP).
At first, each of these techniques, whether descriptive, prescriptive or predictive, has intrinsic characteristics that define the type and amount of input data it requires, as well as a propensity to generate outputs that may be more or less reliable and qualitative. The choice of the technique to adopt is contextual to the phase of the product development in which it should be applied (Van Horn et al. Reference Van Horn, Olewnik and Lewis2013; Altavilla & Montagna Reference Altavilla and Montagna2019) (DA-OP1). Therefore, it is not clear yet how and which techniques should be used in the different stages of the design and development process.
Second, the majority of analytics employed are still descriptive, while predictive and prescriptive analytics represent the next and more insightful challenges (Bstieler et al. Reference Bstieler, Gruen, Akdeniz, Brick, Du, Guo, Khanlari, McIllroy, O'Hern and Yalcinkaya2018) (DA-OP2). At the moment, the main benefit is provided by the latter in identifying optimal improvements to solutions, while a wider exploration of a solution space remains to be fully dealt with.
A final operational aspect concerns the ability of algorithms to automate the learning process based on a large amount of data obtained in input and to acquire autonomy for future data analysis processes (Tan et al. Reference Tan, Steinbach and Kumar2019) (DA-OP3). Nowadays, this characteristic is provided mostly by machine learning algorithms (Fisher et al. Reference Fisher, Pazzani and Langley2014) that go beyond data mining, and AI approaches; however, these approaches have still experienced a lack of implementation during the different design and development phases.
Also, in this last stream, Figure 5 depicts the change brought to the new design paradigm by the use of data analytics. Data analysis tools were already employed during the design process, even in the old paradigm. In particular, data mining and AI algorithms were part of those sets of tools used by designers to tackle a wide variety of design problems. Differently from the latter paradigm, the new one (depicted in Figure 5b) employs a new set of tools, and in particular, machine learning ones, that are increasingly pushed towards autonomy in learning from data and taking over part of the design process activities. This autonomy, together with the need for predictive and prescriptive analysis, and more accurate data analysis tools, is pushing them to become a separate element in the new design paradigm.

Figure 5. The shift from the old (a) paradigm to the new one (b) considering the data analytics.
Finally, Table 7 summarizes the operational consequences and research questions related to this stream of research.
Table 7. Operational consequences on the use of data analytics tools for the design and development process

6. The complete data-driven design paradigm
Based on the previous discussion, it is possible to describe the resulting data-driven design paradigm, as in Figure 6, in which one can realize an increasing relevance of tools and methods, and especially the ones pertaining to platform design and design analytics. These take on an ever more central role in guiding the designers’ interpretation and analysis activity and, therefore, in supporting design decisions. In other words, design methods are no longer to be seen as relatively weak supplements to a process that was mainly driven by human cognition and social interaction but have the potential to become dominant determinants of the outcomes of the design process. Moreover, their effectiveness will heavily rely on the existence of an appropriate IT infrastructure and organizational context.

Figure 6. The new paradigm of the data-driven design context.
The choice and appropriateness of the data analysis methods employed, as well as the methodologically rigorous use of platforms, are therefore likely to affect the quality of the solutions generated heavily.
Therefore, the choice of the methods to be used cannot be left to the designers’ inclinations; it will depend instead on the strategic choices that firms will make in dealing with IT investment and in the way they will interpret the new role of data analysis in the design process, that is, whether to internalize or outsource it; whether to create separate data analysis functions interacting at arm’s length with other functions (including design) or to ‘embed’ data analysts within each function. The degree with which designers will be trained in data analysis (i.e., fully proficiency versus being able to perform simple tasks and interacting with specialists when needed versus being minimally trained to interact with specialists versus no training at all) will constitute a relevant issue. In some way, this is a similar problem to what firms had to tackle when first dealing with Design for X (DFX) methodologies in the 1990s, that is, the amount of competence and responsibility to be assigned to designers on complementary technical domains. The strategic issue at this point becomes evident.
These new design support methods, tools and systems, moreover, undermine the relationship between tacit knowledge, traditionally placed into individuals and the explicit knowledge, conventionally considered as an asset of organizations and incorporated into capital, which also provides a very interesting area of research, which however goes beyond the scope of this paper.
The number of new elements, as well as the complexity emerging from the relational diagram in Figure 6, suggests that this is by no means an incremental change but represents a true paradigm shift. This raises many research questions for academics, with the added difficulty of finding robust empirical foundations, given the recency of the phenomenon. Finding answers to these research questions is of obvious relevance for firms as well, since they risk having to enter this new paradigm by relying on intuition and (potentially costly) trial and error. Finally, there are very significant implications on education, due to the impact on the curricula of future engineers and designers.
7. Conclusions
The paper has attempted to provide a structured and rational discussion on the ways with which digitalization and the ‘data revolution’ are impacting the world of design. Based on the recent literature and preliminary anecdotal evidence, the paper has started by identifying five main technological areas with which digitalization is leading to change in both artefacts and design processes.
This description led to the proposal of a conceptual framework, organized around three main streams, and namely the consequences on designers, the consequences on design processes and the role of methods for data analytics. Given that these three streams have implications at individual, organizational and managerial level, the impact on design can be represented at the intersections between streams and levels. For each such intersection, the authors have then proposed a list of preliminary research questions, which might be used for defining future research agendas in the field.
Moreover, a conceptual framework has been proposed to describe the changing relationships between the objects and the actors involved in the design process. The resulting relational diagrams show a picture of significantly increasing complexity, which suggests that what is happening is not a simple evolution of current design theory and practice but a real paradigmatic shift in the way with which both ‘new’ and ‘old’ artefacts are designed. This has significant consequences for researchers involved in studying the phenomenon and/or developing design support methods and tools, for practitioners, involved in managing this deep change within firms and for educators, who must understand what new skills and competencies are to be given to students. As it happens in most paradigmatic shifts, the emergence of data-driven design has clear interdisciplinary implications, and it is noteworthy that researchers from adjacent fields, such as Verganti et al. (Reference Verganti, Vendraminelli and Iansiti2020), have just recently started working on this topic as well. It is, therefore, possible that the further explorations in the new landscape of data-driven design may lead to contaminations and changes in the current boundaries between disciplines.