76 results
Do we need a more individualised approach to the management of comorbid depression and diabetes?
- Part of
-
- Journal:
- BJPsych Advances / Volume 30 / Issue 5 / September 2024
- Published online by Cambridge University Press:
- 30 July 2024, pp. 267-270
- Print publication:
- September 2024
-
- Article
-
- You have access
- HTML
- Export citation
Estimators for Topic-Sampling Designs
-
- Journal:
- Political Analysis / Volume 32 / Issue 4 / October 2024
- Published online by Cambridge University Press:
- 13 May 2024, pp. 431-444
-
- Article
-
- You have access
- Open access
- HTML
- Export citation
Audit Experiments of Racial Discrimination and the Importance of Symmetry in Exposure to Cues
-
- Journal:
- Political Analysis / Volume 32 / Issue 4 / October 2024
- Published online by Cambridge University Press:
- 06 May 2024, pp. 445-462
-
- Article
-
- You have access
- HTML
- Export citation
Trial by YouTube: effects of expert psychiatric witness testimony on viewers' opinions of Amber Heard and Johnny Depp
-
- Journal:
- BJPsych Bulletin , FirstView
- Published online by Cambridge University Press:
- 22 April 2024, pp. 1-3
-
- Article
-
- You have access
- Open access
- HTML
- Export citation
5 - Use Productive Research Approaches
-
- Book:
- Be a More Productive Scholar
- Published online:
- 28 March 2024
- Print publication:
- 04 April 2024, pp 91-124
-
- Chapter
- Export citation
Methods matter: the influence of method on infection estimates of the bumblebee parasite Crithidia bombi
-
- Journal:
- Parasitology / Volume 150 / Issue 13 / November 2023
- Published online by Cambridge University Press:
- 20 October 2023, pp. 1236-1241
-
- Article
-
- You have access
- Open access
- HTML
- Export citation
Improving precision through design and analysis in experiments with noncompliance
-
- Journal:
- Political Science Research and Methods / Volume 12 / Issue 3 / July 2024
- Published online by Cambridge University Press:
- 01 September 2023, pp. 557-572
-
- Article
-
- You have access
- Open access
- HTML
- Export citation
5 - Science in Practice
-
- Book:
- The Philosophy and Practice of Science
- Published online:
- 17 September 2023
- Print publication:
- 31 August 2023, pp 138-301
-
- Chapter
- Export citation
16 - Experimental Methods
- from Part III - Data Collection
-
-
- Book:
- The Cambridge Handbook of Research Methods and Statistics for the Social and Behavioral Sciences
- Published online:
- 25 May 2023
- Print publication:
- 08 June 2023, pp 333-356
-
- Chapter
- Export citation
9 - Participant Recruitment
- from Part II - The Building Blocks of a Study
-
-
- Book:
- The Cambridge Handbook of Research Methods and Statistics for the Social and Behavioral Sciences
- Published online:
- 25 May 2023
- Print publication:
- 08 June 2023, pp 179-201
-
- Chapter
- Export citation
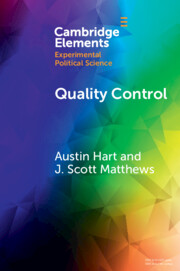
Quality Control
- Experiments on the Microfoundations of Retrospective Voting
-
- Published online:
- 26 May 2023
- Print publication:
- 31 August 2023
-
- Element
- Export citation
Testing for Uni-Dimensional Scaling of Stimuli Used in Preference Experiments
-
- Journal:
- Animal Welfare / Volume 3 / Issue 1 / February 1994
- Published online by Cambridge University Press:
- 11 January 2023, pp. 45-49
-
- Article
-
- You have access
- Export citation
Why is There no Simple Way of Measuring AnimalWelfare?
-
- Journal:
- Animal Welfare / Volume 2 / Issue 4 / November 1993
- Published online by Cambridge University Press:
- 11 January 2023, pp. 301-319
-
- Article
-
- You have access
- Export citation
The decision paradoxes motivating Prospect Theory: The prevalence of the paradoxes increases with numerical ability
-
- Journal:
- Judgment and Decision Making / Volume 14 / Issue 4 / July 2019
- Published online by Cambridge University Press:
- 01 January 2023, pp. 513-533
-
- Article
-
- You have access
- Open access
- HTML
- Export citation
One, two, three: portable sample size in agricultural research
-
- Journal:
- The Journal of Agricultural Science / Volume 160 / Issue 6 / December 2022
- Published online by Cambridge University Press:
- 25 August 2022, pp. 459-482
-
- Article
-
- You have access
- Open access
- HTML
- Export citation
6 - Designing “Good” Experiments
-
- Book:
- Experimental Thinking
- Published online:
- 12 May 2022
- Print publication:
- 12 May 2022, pp 160-168
-
- Chapter
- Export citation
A unified framework for the analysis of germination, emergence, and other time-to-event data in weed science
-
- Journal:
- Weed Science / Volume 70 / Issue 3 / May 2022
- Published online by Cambridge University Press:
- 07 February 2022, pp. 259-271
-
- Article
-
- You have access
- Open access
- HTML
- Export citation
20 - Causal Inference Approaches to Studying Recovery from Alcohol Use Disorder
- from Part III - Macro Level
-
-
- Book:
- Dynamic Pathways to Recovery from Alcohol Use Disorder
- Published online:
- 23 December 2021
- Print publication:
- 06 January 2022, pp 364-379
-
- Chapter
- Export citation
Chapter 10 - Field Experiments and Routine Dynamics
- from Part II - Methodological Issues in Routine Dynamics Research
-
-
- Book:
- Cambridge Handbook of Routine Dynamics
- Published online:
- 11 December 2021
- Print publication:
- 16 December 2021, pp 147-158
-
- Chapter
- Export citation