36 results
Active learning of the collision distance function for high-DOF multi-arm robot systems
- Part of
-
- Journal:
- Robotica , First View
- Published online by Cambridge University Press:
- 12 January 2024, pp. 1-15
-
- Article
-
- You have access
- Open access
- HTML
- Export citation
Teaching Greek mythology through a scenario-based game
-
- Journal:
- Journal of Classics Teaching / Volume 25 / Issue 49 / Spring 2024
- Published online by Cambridge University Press:
- 23 October 2023, pp. 22-32
-
- Article
-
- You have access
- Open access
- HTML
- Export citation
Chapter 5 - Exploring Types of Organizational Change and Differential Effects on Employee Well-Being and Personal Development
- from Part III - Change in Context
-
-
- Book:
- The Psychology of Organizational Change
- Published online:
- 28 September 2023
- Print publication:
- 12 October 2023, pp 95-119
-
- Chapter
- Export citation
3 - Note-Taking Strategies
-
- Book:
- Cognitive and Emotional Study Strategies for Students with Dyslexia in Higher Education
- Published online:
- 14 September 2023
- Print publication:
- 28 September 2023, pp 74-116
-
- Chapter
- Export citation
2 - Developing Global Management Skills
- from Part I - Foundational Skills for Global Managers
-
- Book:
- Management Across Cultures
- Published online:
- 07 September 2023
- Print publication:
- 21 September 2023, pp 25-48
-
- Chapter
- Export citation
12 - Robust Deep Regression and Active Learning
-
- Book:
- Adversarial Learning and Secure AI
- Published online:
- 07 September 2023
- Print publication:
- 31 August 2023, pp 281-293
-
- Chapter
- Export citation
Environmental sensor placement with convolutional Gaussian neural processes
-
- Journal:
- Environmental Data Science / Volume 2 / 2023
- Published online by Cambridge University Press:
- 03 August 2023, e32
-
- Article
-
- You have access
- Open access
- HTML
- Export citation
Focusing on potential named entities during active label acquisition
-
- Journal:
- Natural Language Engineering / Volume 30 / Issue 3 / May 2024
- Published online by Cambridge University Press:
- 06 June 2023, pp. 602-624
-
- Article
-
- You have access
- Open access
- HTML
- Export citation
Chapter 3 - Engagement
-
- Book:
- Handbook of Augmented Reality Training Design Principles
- Published online:
- 18 May 2023
- Print publication:
- 01 June 2023, pp 17-33
-
- Chapter
- Export citation
Active-learning-based nonintrusive model order reduction
-
- Journal:
- Data-Centric Engineering / Volume 4 / 2023
- Published online by Cambridge University Press:
- 09 January 2023, e2
-
- Article
-
- You have access
- Open access
- HTML
- Export citation
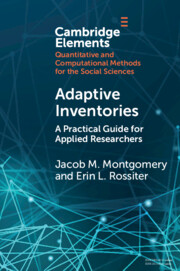
Adaptive Inventories
- A Practical Guide for Applied Researchers
-
- Published online:
- 08 July 2022
- Print publication:
- 28 July 2022
-
- Element
- Export citation
56 - Undergraduate Research in Israel
- from Part IV.2 - Africa & Middle East
-
-
- Book:
- The Cambridge Handbook of Undergraduate Research
- Published online:
- 11 August 2022
- Print publication:
- 07 July 2022, pp 516-523
-
- Chapter
- Export citation
Chapter 5 - A Look to the Future
-
- Book:
- Helping People Learn
- Published online:
- 16 June 2022
- Print publication:
- 30 June 2022, pp 114-156
-
- Chapter
- Export citation
Chapter 2 - The Invention and Use of CmapTools Software in Schools, Corporations, and Other Organizations
-
- Book:
- Helping People Learn
- Published online:
- 16 June 2022
- Print publication:
- 30 June 2022, pp 42-72
-
- Chapter
- Export citation
Chapter 4 - The Design of Better Instructional Programs
-
- Book:
- Helping People Learn
- Published online:
- 16 June 2022
- Print publication:
- 30 June 2022, pp 98-113
-
- Chapter
- Export citation
Chapter 1 - Developing the Foundations to Help People Learn
-
- Book:
- Helping People Learn
- Published online:
- 16 June 2022
- Print publication:
- 30 June 2022, pp 1-41
-
- Chapter
- Export citation
Chapter 3 - Building a Theory of Education
-
- Book:
- Helping People Learn
- Published online:
- 16 June 2022
- Print publication:
- 30 June 2022, pp 73-97
-
- Chapter
- Export citation
Chapter 4 - Searching for Information, from Infancy to Adolescence
- from Part I - What Drives Humans to Seek Information?
-
-
- Book:
- The Drive for Knowledge
- Published online:
- 19 May 2022
- Print publication:
- 02 June 2022, pp 77-98
-
- Chapter
- Export citation
Chapter 4 - The active learner: Working memory, metacognition and brain research
- from Part 1 - The science of learning and development in the twenty-first century
-
- Book:
- The Science of Learning and Development in Education
- Published online:
- 26 May 2022
- Print publication:
- 26 May 2022, pp 84-111
-
- Chapter
- Export citation