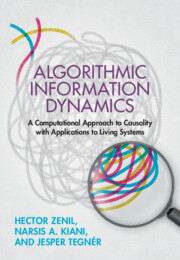
References
Published online by Cambridge University Press: 11 May 2023
Summary
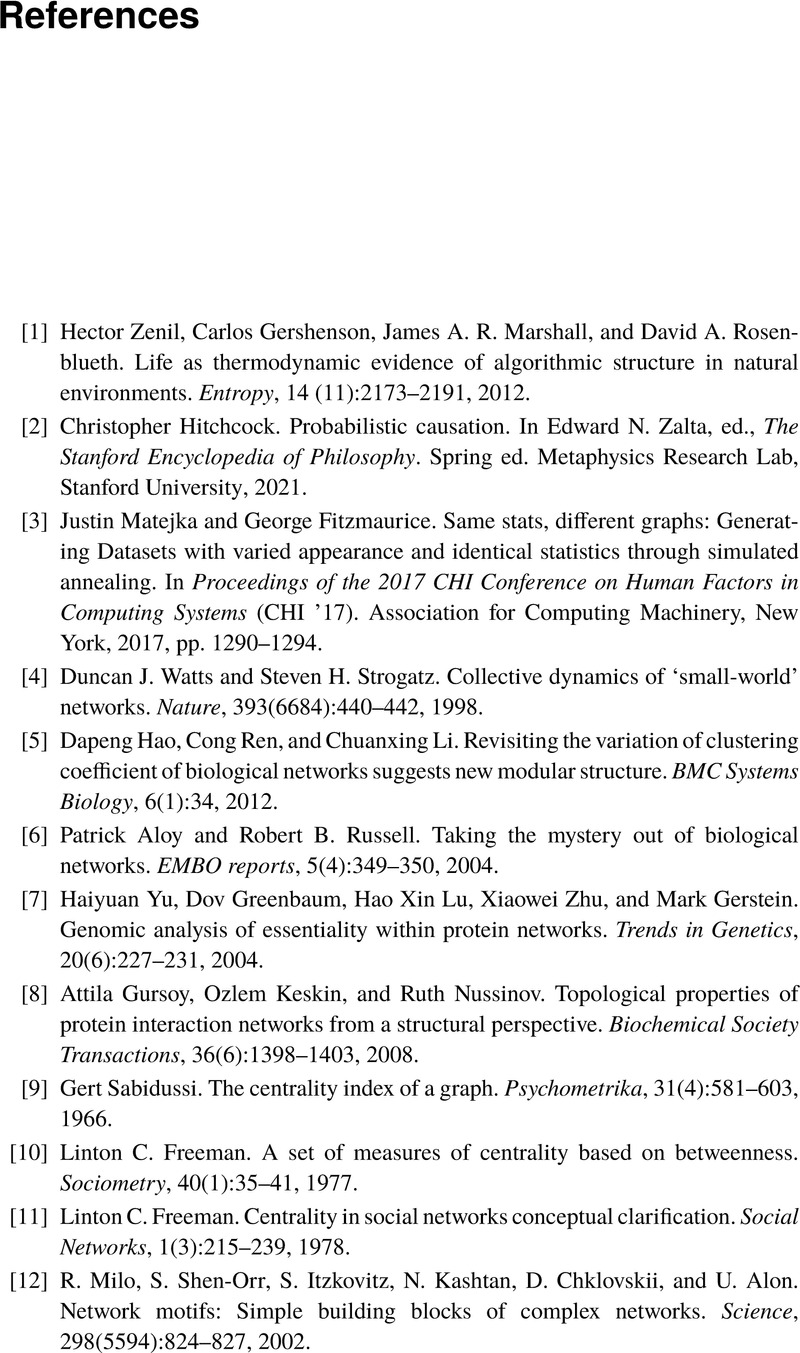
- Type
- Chapter
- Information
- Algorithmic Information DynamicsA Computational Approach to Causality with Applications to Living Systems, pp. 310 - 321Publisher: Cambridge University PressPrint publication year: 2023