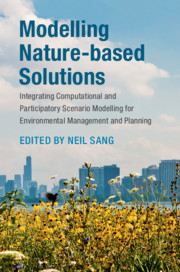
Book contents
- Modelling Nature-based Solutions
- Modelling Nature-based Solutions
- Copyright page
- Contents
- Contributors
- Foreword
- Acknowledgements
- Introduction
- 1 Landscape Modelling and Stakeholder Engagement: Participatory Approaches and Landscape Visualisation
- 2 Agent-based Models of Coupled Social and Natural Systems
- 3 Modelling Nature-based Solutions from Soil Ecosystem Services
- 4 Modelling Water Resources for Nature-based Solutions
- 5 Models at the Service of Marine Nature-based Solutions
- 6 Coastal and Freshwater Flood Models: A Review in the Context of NBS
- 7 Nature-based Solutions to Urban Microclimate Regulation
- 8 Data Mining, Machine Learning and Spatial Data Infrastructures for Scenario Modelling
- 9 Can Geodesign Be Used to Facilitate Boundary Management for Planning and Implementation of Nature-based Solutions?
- 10 Integrating Models into Practice – Recommendations
- Appendix: List of Models/Software
- Index
- Plate Section (PDF Only)
- References
8 - Data Mining, Machine Learning and Spatial Data Infrastructures for Scenario Modelling
Published online by Cambridge University Press: 13 March 2020
- Modelling Nature-based Solutions
- Modelling Nature-based Solutions
- Copyright page
- Contents
- Contributors
- Foreword
- Acknowledgements
- Introduction
- 1 Landscape Modelling and Stakeholder Engagement: Participatory Approaches and Landscape Visualisation
- 2 Agent-based Models of Coupled Social and Natural Systems
- 3 Modelling Nature-based Solutions from Soil Ecosystem Services
- 4 Modelling Water Resources for Nature-based Solutions
- 5 Models at the Service of Marine Nature-based Solutions
- 6 Coastal and Freshwater Flood Models: A Review in the Context of NBS
- 7 Nature-based Solutions to Urban Microclimate Regulation
- 8 Data Mining, Machine Learning and Spatial Data Infrastructures for Scenario Modelling
- 9 Can Geodesign Be Used to Facilitate Boundary Management for Planning and Implementation of Nature-based Solutions?
- 10 Integrating Models into Practice – Recommendations
- Appendix: List of Models/Software
- Index
- Plate Section (PDF Only)
- References
Summary
The models discussed in other chapters in this book relate (generally) to some form of simulation or representation in a formal modelling language. The range of computational or technological complexity involved is variable, but in most cases a very high degree of domain knowledge is also required with respect to the system under investigation. This presupposes that such expertise is available, and indeed that it is sufficient to understand and represent a particular system. For large coupled systems with a wide range of socio-economic, ecological and biophysical systems interacting, this may not be feasible. As with nature itself, NBS are often part of a complex web of interdependent systems so this chapter explores data mining as a pragmatic alternative/complementary approach when systems are insufficiently well-described by current theory or where domain expertise is in short supply. Examples are provided in Table 8.1.
- Type
- Chapter
- Information
- Modelling Nature-based SolutionsIntegrating Computational and Participatory Scenario Modelling for Environmental Management and Planning, pp. 276 - 304Publisher: Cambridge University PressPrint publication year: 2020
References
- 1
- Cited by