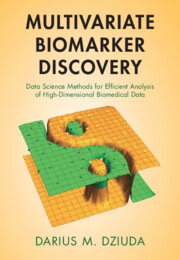
Book contents
- Multivariate Biomarker Discovery
- Multivariate Biomarker Discovery
- Copyright page
- Dedication
- Contents
- Preface
- Acknowledgments
- Part I Framework for Multivariate Biomarker Discovery
- 1 Introduction
- 2 Multivariate Analytics Based on High-Dimensional Data: Concepts and Misconceptions
- 3 Predictive Modeling for Biomarker Discovery
- 4 Evaluation of Predictive Models
- 5 Multivariate Feature Selection
- Part II Regression Methods for Estimation
- Part III Classification Methods
- Part IV Biomarker Discovery via Multistage Signal Enhancement and Identification of Essential Patterns
- Part V Multivariate Biomarker Discovery Studies
- References
- Index
5 - Multivariate Feature Selection
from Part I - Framework for Multivariate Biomarker Discovery
Published online by Cambridge University Press: 30 May 2024
- Multivariate Biomarker Discovery
- Multivariate Biomarker Discovery
- Copyright page
- Dedication
- Contents
- Preface
- Acknowledgments
- Part I Framework for Multivariate Biomarker Discovery
- 1 Introduction
- 2 Multivariate Analytics Based on High-Dimensional Data: Concepts and Misconceptions
- 3 Predictive Modeling for Biomarker Discovery
- 4 Evaluation of Predictive Models
- 5 Multivariate Feature Selection
- Part II Regression Methods for Estimation
- Part III Classification Methods
- Part IV Biomarker Discovery via Multistage Signal Enhancement and Identification of Essential Patterns
- Part V Multivariate Biomarker Discovery Studies
- References
- Index
Summary
Chapter 5 is dedicated to the most important part of predictive modeling for biomarker discovery based on high-dimensional data – multivariate feature selection. When dealing with sparse biomedical data whose dimensionality is much higher than the number of training observations, the crucial issue is to overcome the curse of dimensionality by using methods capable of elevating signal (predictive information) from the overwhelming noise. One way of doing this is to perform many (hundreds or thousands) parallel feature selection experiments based on different random subsamples of the original training data and then aggregating their results (for example, by analyzing the distribution of variables among the results of those parallel experiments). Two designs of such parallel feature selection experiments are discussed in detail: one based on recursive feature elimination, and the other on implementing the stepwise hybrid selection with T2. The chapter includes also descriptions of three evolutionary feature selection algorithms: simulated annealing, genetic algorithms, and particle swarm optimization.
Keywords
- Type
- Chapter
- Information
- Multivariate Biomarker DiscoveryData Science Methods for Efficient Analysis of High-Dimensional Biomedical Data, pp. 76 - 98Publisher: Cambridge University PressPrint publication year: 2024