INTRODUCTION
The issue of antimicrobial resistance in bacteria of veterinary origin has been a significant concern in respect of both human and animal health for many years [Reference Swann1]. Since the approval of fluoroquinolone (FQ) antibiotics for animal use in the 1990s, there has been evidence that FQ resistance of veterinary origin is appearing in human patients, particularly in cases of salmonellosis and campylobacteriosis [Reference Endtz2, Reference Threlfall3]. The issue of FQ resistance and its links to veterinary sources prompted a farm-level study of FQ resistance in thermophilic Campylobacter spp. and E. coli, of which the present report is a part.
The reported prevalence of Campylobacter excretion in slaughter-weight pigs is around 60–100% [Reference Harvey4–Reference Pezzotti6]. In neonatal piglets, diarrhoea may be seen in association with exposure to Campylobacter coli or Campylobacter jejuni [Reference Taylor7], but colonization by Campylobacter is generally asymptomatic. In most surveys, C. coli is the heavily predominant species in pigs [Reference Milnes5, Reference Payot8–Reference Thakur and Gebreyes10], although C. jejuni predominates in some American studies [Reference Harvey4, Reference Young11]. Typing studies suggest that pigs are relatively less important as a source of zoonotic C. coli when compared with other food animals, particularly poultry [Reference Pezzotti6, Reference Guevremont, Higgins and Quessy12–Reference Siemer, Nielsen and On14]. This may be due to post-slaughter treatment of pig carcases that is unfavourable to survival of Campylobacter. Rapid selection of resistance has been observed in FQ-treated pigs: C. coli readily acquires a clinically relevant level of resistance to FQ [Reference Delsol15], and generally exhibits a higher level of resistance to antimicrobials than does C. jejuni [Reference Pezzotti6, Reference Engberg16, Reference Gupta17]. FQ resistance may be found on pig units that do not use these antimicrobials [Reference Thakur and Gebreyes10], and studies of conventional slaughter-weight pigs in France have yielded FQ-resistant Campylobacter spp. at prevalences of between 11% and 24% of isolates [Reference Payot8, Reference Moore18]. Concerns have been expressed about the ecological problem of environmental contamination with such resistant organisms in animal waste [Reference Payot8, Reference Moore18].
E. coli is universally present in the intestines of pigs, and most strains are commensals which are not associated with disease [Reference Hartl and Dykhuizen19]. However, some E. coli strains which possess certain adhesion, invasion and/or toxigenic capabilities are considered to be primary porcine pathogens, capable of causing enteritis, septicaemia or oedema disease [Reference Taylor7]. Resistance to FQ in E. coli is commonly seen in human and veterinary isolates [Reference Threlfall20, Reference Webber and Piddock21], and there is evidence of the transfer of antimicrobial-resistant porcine E. coli to human intestinal flora [Reference Aarestrup and Wegener22].
The current study involved cross-sectional sampling of finisher pig and poultry meat production units for FQ resistance, focusing on Campylobacter and E. coli, to assess the level of FQ resistance in these sectors in the United Kingdom [Reference Taylor23]. The prevalence of FQ resistance was examined at the farm level. Simultaneously, data were collected to provide the basis for an exploratory risk-factor analysis. The present report describes the results of this analysis for finisher pig farms. The risk-factor analysis for poultry farms will be reported elsewhere.
MATERIALS AND METHODS
Data collection
Data were taken from farms participating in a survey to estimate farm-level prevalence of FQ-resistance [Reference Taylor23]. The target population was defined as: ‘pig finishing (breeding to finishing, or growing and finishing) farms in Great Britain, with at least 100 breeding females if breeder to finish, or 200 finisher places if specialist finishers’. There were 2650 eligible holdings according to the June 2002 Agricultural Census. A desired sample size of 330 farms had been calculated on the basis of attaining an estimate of proportion of farms ‘affected’ with a 95% confidence interval (CI) of ±5%. However, the final sample size obtained was affected by the industry's apprehension and reluctance to take part. In total, 416 pig farms, selected at random from lists provided by Quality Meat Scotland (QMS), Assured British Pigs and the National Pig Association, were contacted by their organizations and invited to join the study. Partly due to the sensitive nature of the study, only 108 farms agreed to take part. This population of sampled farms matched the geographical distribution of the national herd. The sample contained both breeder/finisher and non-breeding grower/finisher farms. Breeding farms made up 55% of the sample, compared with 59% of eligible British holdings which had breeding pigs in the June 2002 Agricultural Census.
Data about husbandry practices, performance criteria, disease and drug use were collected using detailed questionnaires completed by the farm manager and the farm's private veterinarian who was contracted to do the sampling. Information about recent usage of FQ and other prescription drugs on the farm was verified by the farm's private veterinarian using farm and practice records.
Categorization of farms for FQ resistance
The 108 participating pig farms were classified as ‘affected’ or ‘not affected’ with respect to FQ resistance in E. coli and thermophilic Campylobacter spp. following bacteriological analysis of faecal samples taken as part of a cross-sectional survey carried out between December 2002 and October 2003, the details of which are reported elsewhere [Reference Taylor23]. The sampling protocol was devised to provide a 95% confidence level for detecting affected farms assuming a minimum prevalence of 5% of animals shedding resistant bacteria and a 90% sensitivity of laboratory detection.
Briefly, measured aliquots of eight pools of ⩾8 fresh faecal samples from finishing pigs were cultured on semi-selective media (Chromagar ECC, CM956, Oxoid Ltd, Basingstoke, UK, for E. coli and BASAC [Reference Shreeve24] for thermophilic Campylobacter) containing 1 mg/l ciprofloxacin before and after broth enrichment. The identities of putative E. coli and Campylobacter colonies were confirmed using standard biochemical tests. The growth of colonies typical of E. coli or Campylobacter spp. on ciprofloxacin-containing media was taken to indicate that FQ-resistant bacteria were present in the faecal samples and therefore the farm of origin was classed as ‘affected’. Minimum inhibitory concentrations of ciprofloxacin for the FQ-resistant bacteria were estimated based on an agar doubling dilution methodology [25, Reference Randall26] and all were shown to be ⩾2 mg/l with 90% ⩾8 mg/l. To demonstrate the presence or absence of Campylobacter on the farm in the case of no growth on ciprofloxacin-containing media, swabs from pooled faecal samples were also plated on ciprofloxacin-free BASAC after enrichment in Exeter broth.
Statistical analysis
The questionnaire generated a huge number of variables. In an initial exploration of the data, univariate analyses were performed for potential risk factors with respect to odds of occurrence of FQ-resistant E. coli and FQ-resistant Campylobacter. These analyses were performed using exact conditional logistic regression, using the exact statement of SAS proc logistic in SAS version 8.2 [27] in order to provide exact 95% confidence intervals. Following the recommendation of Agresti [Reference Agresti28] inference based on the mid-P value was used to alleviate conservativeness in the exact confidence intervals.
As a preliminary to the final regression analyses, variables were further rationalized using correlation and cluster analyses, also in SAS [27]. The questionnaire data were first broken up into blocks according to subject matter (e.g. farm characteristics, farm hygiene, biosecurity, drug usage). The analytical process then examined each block in turn, before combining data from all blocks in a final analysis. The approach taken was as follows.
Step 1
Each block of data contained variables that could be expected to be related: for example, the provision and use of site-dedicated boots on farms and the use of site-dedicated overalls, or the provision of a toilet for staff and the provision of a washbasin. In a regression model the inclusion of one of a group of related variables would ‘stand for’ all variables in that group. Screening of variables within each block was performed to identify groups of related variables. Using 1−r 2 as a distance measure between all possible pairs of variables within a block (where r=Spearman's correlation coefficient [Reference Sprent and Smeaton29]), Ward's minimum variance cluster analysis [Reference Everitt30, Reference Ward31] was performed to generate graphic representations of variable grouping in the form of dendrograms.
Step 2
The groups identified in step 1 were examined in order to assess which variables should represent each group and be put forward as possible explanatory variables (risk factors) in the multiple regression. Decisions were based on epidemiological grounds, but also took into account the variability of the candidate factor in the population and data completeness (variables with more variability and more data-points were favoured). The aim was to include at least one variable from each group of related variables in the regression, so that every group would be represented in the analysis.
Step 3
The key variables identified in step 2 were tried as explanatory (independent) variables in logistic regression modelling [27] within each block, using the farm result for FQ-resistant E. coli (affected or not affected) or, similarly, FQ-resistant Campylobacter as the outcome (dependent) variables. In order to extract the most value from the data, despite the smaller than ideal sample size, an exploratory approach using a non-automatic variable selection procedure, as recommended by Collet [Reference Collett32] was used. Briefly, both forwards and backwards manual stepwise modelling was performed and the sets of variables that made the best epidemiological sense were chosen as the basis for final modelling. At the next stage, models were fitted using the maximum data available and variables originally dropped by the stepwise procedures were manually added to the base models one by one. This process often resulted in further variables being found to be significant within the model. The manual fitting process was repeated until no further variables could be added or dropped based on their statistical significance and scientific relevance. Following the recommendation of Collet [Reference Collett32], the criteria for significance, on which variables were included in the models, were P⩽0·1 to enter and P>0·1 to exit. This process identified a small number of ‘candidate’ risk-factor variables from each block.
Step 4
Variables from different blocks could be related: for example, presence of sows, buying-in of gilts and buying-in of growers (block F) are all connected with the type of farm (breeding to finish or finish only – block B). Therefore, as with variables within subject-matter blocks, all ‘candidate’ risk-factor variables identified in step 3 were screened for correlations and clustering regardless of their originating data blocks, using the statistical procedures of step 1. Variables were omitted from the next step if there were strong correlations with other variables. For example the ‘buying-in of growers’ variable was used to stand for farm type, including presence or absence of sows.
Step 5
The retained ‘candidate’ variables from all blocks were tried together in logistic regression modelling. The modelling procedures and criteria for significance were as described for step 3. Having fitted main effects, interactions were checked for but inclusion of these in the regression models always resulted in estimates for some odds ratios approaching infinity or zero. This was considered to be the result of small sample sizes, such that inclusion of too many effects, notably the interactions, produced models that were ‘over-fitted’, as described by Collet [Reference Collett32]. To avoid the possibility of over-fitting and implausible interpretations, models were finalized without interactions.
The analysis resulted in identification of a ‘final’ list of risk factors for occurrence of FQ resistance in each bacterial species, and quantified the effects of these risk factors in terms of adjusted odds ratios.
The results of the final regression modelling are presented as tables showing the variables included as risk factors, estimates of coefficients with P values, and the estimated adjusted odds ratios with 95% confidence intervals. The confidence intervals for the odds ratios were calculated using the exact statement in logistic regression [27] to adjust for the problem of small sample sizes in the strata. As in the univariate analyses, inference based on the mid-P value was used to alleviate conservativeness in the exact confidence intervals.
An r 2 value, which estimates the proportion of variation in the data explained by the model, was calculated for each model, according to the method of Nagelkerke [Reference Nagelkerke33] as recommended by Collet [Reference Collett32].
RESULTS
Of the 416 farms invited to take part in the survey, 345 responded. Of these, 78 were ineligible for the survey (had stopped keeping pigs or had too few pigs). Of the remaining 267, 159 declined to take part: 34 gave reasons relating to lack of time and money; 24 gave a variety of other reasons and 101 gave no reason. Thus 108 farms took part in the survey.
FQ-resistant E. coli were isolated from 63/108 farms. FQ-resistant Campylobacter were isolated from 81/108 farms; no Campylobacter were detected on 20/108 farms and Campylobacter were isolated from a further 7/108 farms on which no FQ-resistant Campylobacter had been isolated.
Use of FQ antibiotics and risk of resistance on the surveyed farms
In relation to the use of FQ on farms, the questionnaire response options were: ‘within 12 months’, ‘between 1 and 2 years ago’, ‘over 2 years ago’, and ‘never’. Table 1 shows the breakdown of responses. Use of FQ was reported on 71 (66%) of 108 farms in the survey. Almost half of all farms had used FQ in the last year and more detail about the usage was available for these farms. The most common conditions treated with FQ were reported to be ‘enteric problems’ or ‘scouring’. The most common formulation recently employed was 5% or 10% injectable solution, used on 50/53 farms that had used FQ within the last year. An oral FQ piglet doser had been used on 12 farms, three of which had not additionally used an injectable form. FQ had been used in all classes of pig, but use was most commonly reported in piglets, in 36/53 farms.
Table 1. Detection of fluoroquinolone (FQ)-resistant bacteria on finisher pig farms in Great Britain during 2002–2003, according to the timing of the most recent use of FQ

* Thirteen of these 18 reported most recent use >2 years ago.
FQ-resistant E. coli and/or Campylobacter spp. were detected on 96% of the farms that had a reported history of FQ use. The proportions of farms affected by FQ-resistant E. coli and Campylobacter were lower in farms where the most recent use of FQ had been >1 year ago than in those using FQ within the last year (Table 1). However, these differences were not statistically significant (Fisher's two-tailed exact test: E. coli, P=0·1837; Campylobacter, P=0·4339). For this reason, in further analyses, farms where any FQ use had been reported were grouped together as having used FQ within the recent past, for comparison with those farms reporting that they had never used FQ.
Modelling of risk factors for the occurrence of FQ resistance on finisher pig farms
Tables 2 and 3 list the variables that were selected from the questionnaire data for analysis. Univariate odds ratios for the association between each variable and farm status with respect to resistance are shown, with exact mid-P confidence intervals. The numbers of farms exposed or not exposed to each putative risk factor and positive for resistance (R+) or negative for resistance (R−) are shown. Data sparsity is indicated in Tables 2 and 3 by bold italic wherever the number of farms in any one exposed/resistant category was ⩽5.
Table 2. List of variables selected from the finisher pig farm survey, Great Britain 2002–2003, with univariate odds ratios for the occurrence of fluoroquinolone (FQ)-resistant E. coli
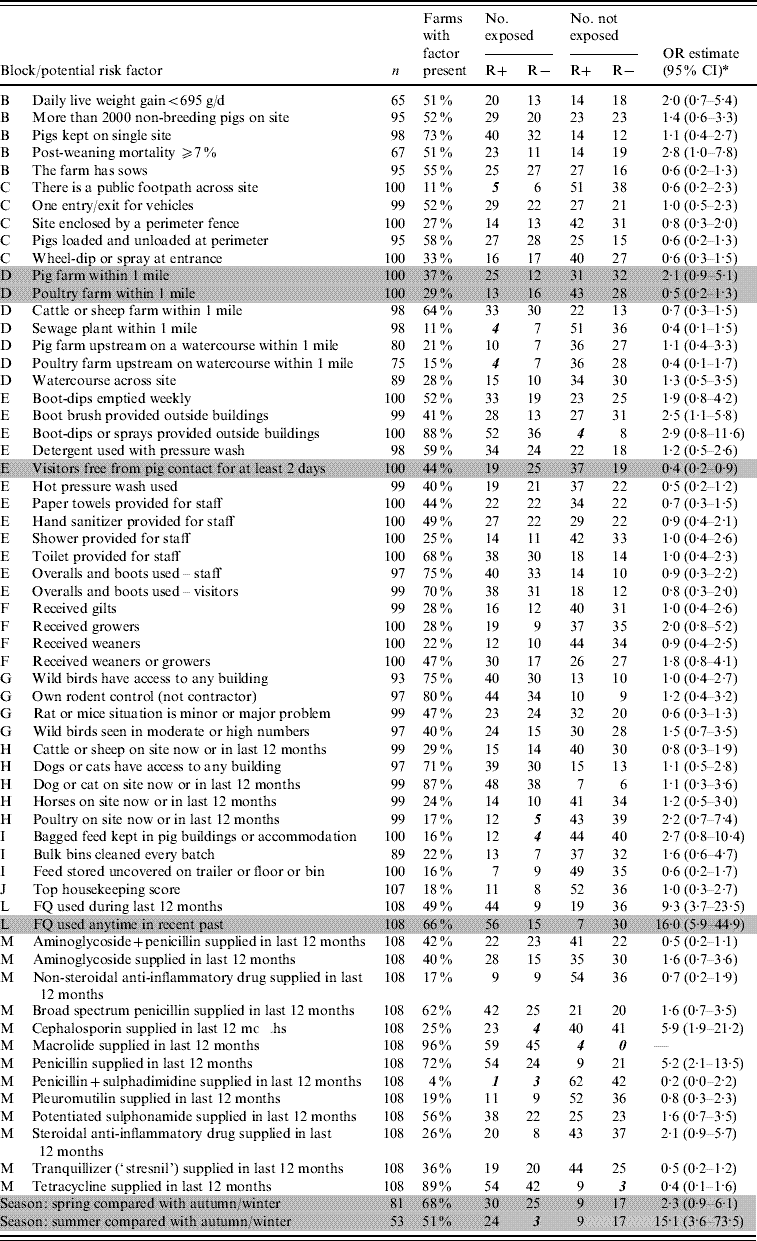
R+, Number of farms positive for FQ-resistant E. coli; R−, number of farms negative for FQ-resistant E. coli; OR, odds ratio; CI, confidence interval.
Shaded rows indicate the variables included in the final multivariate model (see Table 4).
Bold italic values indicate data sparsity wherever the number of farms in any one exposed/resistant category is ⩽5.
Block B, general characteristics of farm; block C, perimeter security; block D, possible sources of bacteria around the farm; block E, farm biosecurity and hygiene; block F, pigs moved onto the farm; block G, vermin around the site; block H, other animals around the site; block I, handling of pig feed; block J, housekeeping score judged by the vet filling in the questionnaire (this factor contains a ‘bundle’ of details related to maintenance, cleaning and biosecurity on the farm); block L, recent use of FQ; block M, use of drugs, other than FQ antibiotics, in the last 12 months.
* Confidence intervals based on mid-P inference using exact conditional logistic regression.
Table 3. List of variables selected from the finisher pig farm survey, Great Britain 2002–2003, with univariate odds ratios for the occurrence of fluoroquinolone (FQ)-resistant Campylobacter

R+, Number of farms positive for FQ-resistant Campylobacter; R−, number of farms negative for FQ-resistant Campylobacter; OR, odds ratio; CI, confidence interval.
Shaded rows indicate the variables included in the final multivariate model (see Table 5).
Bold italic values indicate data sparsity wherever the number of farms in any one exposed/resistant category is ⩽5.
Block B, general characteristics of farm; block C, perimeter security; block D, possible sources of bacteria around the farm; block E, farm biosecurity and hygiene; block F, pigs moved onto the farm; block G, vermin around the site; block H, other animals around the site; block I, handling of pig feed; block J, housekeeping score judged by the vet filling in the questionnaire (this factor contains a ‘bundle’ of detail related to maintenance, cleaning and biosecurity on the farm); block L, recent use of FQ; block M, use of drugs, other than FQ antibiotics, in the last 12 months.
* Confidence intervals based on mid-P inference using exact conditional logistic regression.
Correlation and clustering analysis revealed that several of the variables were highly correlated with each other. Specifically:
• The existence of sows on the farm (breeding farm) was positively correlated with unloading pigs at the perimeter, the requirement for visitors to be free of pig contact for at least 2 days, the buying-in of gilts and the recognition of a rodent problem; it was negatively correlated with the buying-in of growers and weaners.
• The buying-in of growers was negatively correlated with the unloading of pigs at the perimeter of the farm.
• The existence of a pig farm within 1 mile was positively correlated with the existence of a poultry farm within 1 mile.
• In this particular sample of pig farms, the variable ‘provision of a boot brush’ was connected with several other, seemingly unrelated, hygiene and biosecurity variables; it was negatively correlated with requirement for visitors to be free of pig contact for at least 2 days, provision of wash basin, toilet and soap and provision of boots and overalls for visitors.
Table 4 provides a summary of the factors included in the final fitted logistic regression model for the risk of occurrence of FQ-resistant E. coli. Three variables are included with P values between 0·07 and 0·1. The only model in which all variables have P values ⩽0·05 is a model containing only the variables related to use of fluoroquinolone and season. Inclusion of the three further variables in the model does not greatly change the P values or the estimated odds ratios of these two factors and provides more complete epidemiological information. Factors associated with increased risk are: the use of FQ in the recent past, the existence of another pig farm within 1 mile of the site, and sampling carried out in the spring and especially summer seasons in comparison with autumn and winter. Factors associated with decreased risk are: the existence of a poultry farm within 1 mile of the site and the requirement for farm visitors to be free from pig contact for at least 2 days. The r 2 value is >50%, indicating that the model provides a good explanation of factors affecting the occurrence of FQ-resistant E. coli.
Table 4. Estimated adjusted odds ratios of variables included as risk factors in the final multiple logistic regression model for the detection of fluoroquinolone (FQ)-resistant E. coli on finisher pig farms in Great Britain during 2002–2003

n=100.
Maximum re-scaled r 2=56·0%.
* P value is based on likelihood ratio test, apart from specific comparisons involving season which are based on the Wald test.
† Exact (mid-P) confidence intervals.
Table 5 provides an equivalent summary for FQ-resistant Campylobacter. Three of the variables were included with P values between 0·06 and 0·07. However, all of these variables had P⩽0·05 when one of the others was dropped from the model. Two candidate models were found with all variables having P⩽0·05, but no justification could be found for choosing one of these models above the other. A more informative epidemiological impression is therefore given by presenting the model containing all these variables. Factors associated with increased risk are: the use of FQ in recent past, the buying-in of growers and the provision of boot brushes outside buildings. Factors associated with decreased risk are: a requirement for farm visitors to be free from pig contact for at least 2 days and a top housekeeping score given by the veterinary surgeon completing the questionnaire. This last factor contained a ‘bundle’ of details related to maintenance, cleaning and biosecurity on the farm and is interpreted as an indicator of generally superior management of the farm. It is also important to remember that provision of a boot brush was associated, in this sample of farms, with lack of provision of wash basin, toilet and soap for staff and boots and overalls for visitors. The r 2 value is just <50%, indicating that the model provides a reasonable explanation of factors affecting the occurrence of FQ-resistant Campylobacter. However, there could be other important unidentified factors.
Table 5. Estimated adjusted odds ratios of variables included as risk factors in the final multiple logistic regression model for the detection of fluoroquinolone (FQ)-resistant Campylobacter spp. on finisher pig farms in Great Britain during 2002–2003

n=98.
Maximum re-scaled r 2=46·5%.
* P value is based on likelihood ratio test.
† Exact (mid-P) confidence intervals.
DISCUSSION
The present survey and risk-factor analysis was designed to identify factors that increase or decrease the risk of FQ resistance occurring on farms. The farm sample size obtained was restricted by farmers' reluctance to take part. The fact that only 26% of farms originally contacted took part could be a source of selection bias in the sample. The British pig industry was undergoing a significant contraction during the period of the study and many farmers were leaving the industry. Seventy-eight farmers out of 159 who gave detailed reasons for not taking part ruled themselves out for the reason that they no longer kept pigs, or kept too few. It cannot be known how many of the 172 farmers who gave no reason for not wishing to participate, or who did not respond at all, might also have recently stopped keeping pigs, but there might have been a high number in this group.
The present study was just one of three being carried out concurrently in the same sample of farms, so farmers did not necessarily decline from the study for reasons that could be related to the use of FQ antimicrobials. Indeed, within the sample, the proportion of farms reporting FQ use does not appear unusually low. The population of sampled farms matched the geographical distribution of the national herd and the sample contained proportions of breeder/finisher and non-breeding grower/finisher farms close to that revealed in the June 2002 Agricultural Census. The survey has provided a snapshot of biosecurity, hygiene and related practices on pig finisher farms. Tables 2 and 3 summarize the proportions of farms that have particular characteristics, use a variety of husbandry practices and use a variety of drugs. Our impression is that the sample of farms was not biased towards what might be called either ‘good practices’ or ‘bad practices’. Therefore, whilst caution is advised in view of the potential for selection bias, we feel that the results reported here are valid as an initial indication of what might be important risk factors for occurrence of FQ-resistant organisms on pig farms.
Many of the variables on biosecurity, hygiene and associated practices were related, indicating that farmers often adopt, or do not adopt, bundles of practices as a package, or that variables are linked because of the way a farm is operated. For example, breeding farms tended to practise better boundary biosecurity control than non-breeding farms. The use of Ward's minimum variance cluster analysis proved to be a convenient way of identifying groups of variables that were clustered in this way, allowing epidemiologically meaningful analysis and interpretation to be carried out. A major advantage of this approach, over carrying out pairwise association tests such as χ2 tests, between variables, is that Ward's analysis can handle many variables at one time and produces graphical output in the form of dendrograms that identify clustered groupings of variables.
At the outset, it was anticipated that FQ-resistant E. coli or Campylobacter would be found on a small percentage of farms, that there would be farms using FQ that were not affected and there would be very few farms not using FQ that were affected. It was found that FQ-resistant E. coli and/or Campylobacter were detected on a very high proportion (96%) of farms that had used FQ in the past. Indeed, the use of FQ was by far the most important factor influencing the occurrence of FQ-resistant bacteria on farms, having the highest odds ratios in the logistic regression models. A separate analysis showed that there were no statistically significant differences in risk between farms where the most recent use of FQ had been within the previous year and those using FQ more than 1 year previously. However, FQ-resistant bacteria were also detected on a substantial proportion of farms that reported never having used FQ, particularly in the case of Campylobacter.
Using the results of the current surveys alone, it is impossible to explain fully the factors influencing the occurrence of FQ resistance on non-FQ-using farms. The small sample size and data sparsity, as indicated in Tables 2 and 3, have precluded the identification of a full set of statistically significant and strongly associated risk factors and the calculated 95% confidence intervals for the odds ratio estimates in the final models (Tables 4 and 5) are wide as a result. Caution is advised in interpreting the results, which should be seen as indicating some epidemiologically plausible factors that warrant further investigation, rather than robust evidence of causal association.
The list of variables presented in Tables 2 and 3 has been reduced from the complete set of variables extracted from the questionnaire. This reduction was made on the basis that variables within subject-matter blocks can be related and act as proxies for each other. Similarly, variables in different blocks were found to be related: thus, although there are several variables in Tables 2 and 3 with statistically significant univariate odds ratios, only one or two of these were included in the final multivariate models: for example, the use of some types of antibiotic other than FQ was often associated with use of FQ, therefore these variables were not required in the multivariate model. It is also important to remember that a variable in the final model may actually be representing the epidemiological impact of several factors.
It appears from the present data that resistant organisms can be spread between farms, leading to resistance on premises where FQ have never been used. The establishment and persistence of such organisms in the absence of the selective pressure of FQ use can be explained by the observations that mutations conferring resistance to antimicrobials often confer little or no fitness cost [Reference Sander34], and that so-called compensatory evolution of resistant mutants can ameliorate any such cost, or even confer a fitness advantage [Reference Schrag, Perrot and Levin35]. FQ resistance in particular does not appear to confer a heavy fitness burden in Campylobacter [Reference Zhang, Lin and Pereira36]. It is logical to assume that FQ-resistant bacteria may be introduced to, and established in, farm animal populations through the buying-in of animals carrying resistant bacteria or by carriage of the resistant bacteria onto the farm by fomites. The factors affecting this spread are those that would be associated with the spread of any bacterial agent. However, there are differences in the relative importance of various factors between E. coli and Campylobacter.
The results of this analysis support the conclusion that biosecurity is important for avoidance of FQ resistance in both bacterial species examined. Requiring visitors to the farm to be free of pig contact for at least 2 days may have had a protective effect. This emphasizes the importance of hygiene barriers and is consistent with the possibility of resistant E. coli being brought on farm by various ‘overland’ fomites such as people and wild animals. E. coli are robust, may persist for months or years in the environment [Reference LeJeune, Besser and Hancock37, Reference Sjogren38] and be transferred by many fomites such as workers' or animals' feet and vehicle tyres. Therefore, barrier biosecurity measures such as separation from other farms and requirements for visitors are important. For E. coli, the existence nearby of other pig farms, which may use FQ and therefore have FQ-resistant E. coli, appeared to be associated with increased risk. Interestingly, having a poultry farm nearby was protective, the reasons for which are uncertain but it is possible that the local environment may have been seeded with avian strains of E. coli which may have had a reduced ability to colonize pigs. During the statistical modelling, the two variables related to nearby pig and poultry farms operated as a pair: the P value for either one was very high unless the other was also included. These variables were also positively correlated, reflecting the fact that both types of farms tend to be established in similar areas of the country (predominantly in the east). That these two variables affect the risk of occurrence of FQ-resistant E. coli on the pig farms in opposite ways might be explained by the fact that where there are poultry farms within 1 mile of the pig farm, even though this increases the likelihood that there are also pig farms in the area, the poultry farms ‘dilute’ the density of nearby pig farms. It may be hypothesized that this dilution is associated with a lower exposure to exogenous porcine-adapted strains of E. coli, which would otherwise be more likely to persist in exposed pigs.
A notably high proportion (54%) of farms that had never used FQ had FQ-resistant Campylobacter, which strongly suggests that biosecurity breaches introducing FQ-resistant Campylobacter spp., which subsequently persist for long periods, may be a common occurrence on pig farms. An important element of this may be the buying-in of pre-exposed animals, i.e. growers, and the analysis suggested the buying-in of growers may be an important risk factor. Growers were generally bought-in by farms that did not maintain breeding sows and therefore would buy-in young pigs from other farms, where they may well have been exposed to FQ. Campylobacter on pig farms generally show a diversity of strains persisting in parallel [Reference Keller and Perreten39, Reference Weijtens40], and the acquisition of Campylobacter infections as young piglets appears to be an important determinant of strain types in older animals, more so than any inter-animal transfer or environmental exposure in growers or adults [Reference Weijtens41, Reference Weijtens42]. This implies that FQ-resistant strains brought in with growers will tend to persist in that group but may not readily spread to other pigs, unless uninfected young piglets are exposed to FQ-resistant strains from older animals. A requirement for visitors to have been free from pig contact for at least 2 days may be protective for resistance in Campylobacter, suggesting that FQ-resistant Campylobacter may be carried between farms by people and/or vehicles and can become established in new premises by this route. The low fitness cost of FQ resistance in Campylobacter implies that there will be little or no negative selective pressure on brought-in resistant strains in the absence of FQ use.
FQ-resistant E. coli were detected on a higher proportion of farms that were sampled in summer than on farms sampled in other seasons. The increase may possibly coincide with the seasonality of enteric disease in pigs, for which FQ treatment may have been used previously in sucking or weaned pigs. If so, then FQ-resistant strains appear to decline fairly rapidly, at least initially, following the cessation of FQ use. This may simply reflect the normal strain turnover rate in pigs rather than a particular competitive advantage of non-resistant strains. In a farm-level risk analysis of E. coli resistance to non-FQ antimicrobials in pigs at slaughter [Reference Dunlop43], it was concluded that the routine treatment only of young piglets with antimicrobials did not affect the levels of resistance seen at slaughter, in comparison to units not using antimicrobials routinely at any stage of production. This suggests that pigs may also exhibit rapid declines in E. coli resistant to a range of antimicrobials other than FQ, following removal of the relevant selective pressure. The relative contributions to this effect of competitive disadvantage and natural strain turnover probably vary between antimicrobials. However, the situation with regard to the decline of resistance with time is likely to be more complicated than the foregoing scenario because, whilst season produced a significant difference in resistance frequency, there was no significant difference in the frequency of affected farms between those using FQ in the last year and those using them more than a year ago. Therefore, seasonal factors potentially affecting the sensitivity of detection, for example the environmental temperature, may also have played a role in the patterns observed. This would have to be confirmed by repeat studies to confirm true seasonality and a link with meteorological trends.
Farm hygiene appears to be particularly important for resistance in Campylobacter spp. The variable ‘provision of a boot brush’ was associated with an increased risk of detection of FQ-resistant Campylobacter. On the farms sampled, this variable was connected with several other, superficially unrelated, hygiene variables such as negative correlations with the provision of wash basins, toilets, soap, and of boots and overalls for visitors. These connections are not readily explained, but the result is that the ‘boot brush’ variable acts as an indicator for a complex combination of variables generally associated with poor hygiene facilities. It may itself also be a direct indicator of a dirty farm, where boot brushes would be needed because boots become heavily soiled. The odds ratio associated with the provision of a boot brush can therefore be seen as a measure of the effect of a package of factors, rather than of the provision of a boot brush alone. The attainment of the top ‘farm housekeeping score’ is also associated with a reduced risk of detection of FQ-resistant Campylobacter, and indicates generally good performance in a package of farm maintenance, hygiene and biosecurity criteria.
Schuppers et al. [Reference Schuppers9] examined herd-level risk factors for resistance to antibiotics including FQ (ciprofloxacin) in C. coli on Swiss pig farms, using a similar approach to the present study. There was more emphasis on herd health variables, including assessments by clinical examinations, but data were not sufficient to take account of previous antimicrobial use on farms. Biosecurity and ‘good housekeeping’ factors were found to be important in this study also. An increased risk of finding multiple antimicrobial resistance was associated with a lack of ‘all-in-all-out’ batch management and with some indicators of poor herd health.
The high proportion of FQ-using farms that had detectable resistance and the strong association of resistance with recent use of FQ indicate that it is difficult for farms that use FQ to avoid resistance, although a very low proportion of the bacterial population may be resistant. This was shown in related studies where the resistant proportion of the E. coli population in faecal samples from pigs on seven affected pig farms ranged between 0·008% and 53% and the resistant proportion of the Campylobacter population on five affected pig farms varied from <10% to 100% [Reference Taylor23]. It seems that the greatest scope for avoiding the development of FQ resistance may lie with those farms that have never used FQ, but which on the present evidence appear still to be at substantial risk of acquiring such resistance. Given the above findings, it can be suggested that to avoid FQ resistance, control measures should be focussd on minimizing the likelihood that FQ will be needed by maintaining a clean and healthy farm environment; preventing entry of resistant bacteria from outside by strengthening and maintaining biosecurity measures and preventing carry-over of resistant bacteria between groups of animals by applying high standards of farm hygiene and cleaning and disinfection between batches. This is in line with guidelines produced by the UK ‘Responsible Use of Medicines in Agriculture Alliance’ (RUMA; http://www.ruma.org.uk), which stress that the use of antimicrobials should be seen as complementing good management, vaccination and site hygiene. Bayer Healthcare, the major manufacturer of veterinary FQ, also states in its current guidelines for the use of quinolones in veterinary medicine [44] that ‘strategies aimed at reducing the need for antibiotics in disease control should be encouraged’. Pathogen eradication programmes plus present and projected advances in vaccinations may reduce the already limited need for FQ use.
ACKNOWLEDGEMENTS
This work was funded by the UK Veterinary Medicines Directorate and Department for Environment, Food and Rural Affairs (Defra), under project VM02101. The authors thank Mr A. Cook and Mr A. Miller for collaboration on sampling of pig farms within Defra project OZ0316; Mr J. Gallagher for statistical support and the veterinary surgeons, farmers and companies without whose support this project could not have been conducted.
DECLARATION OF INTEREST
None.