439 results
Self-supervised contrastive learning of radio data for source detection, classification and peculiar object discovery
-
- Journal:
- Publications of the Astronomical Society of Australia / Volume 41 / 2024
- Published online by Cambridge University Press:
- 05 November 2024, e085
-
- Article
-
- You have access
- HTML
- Export citation
The case for eliminating excessive worry as a requirement for generalized anxiety disorder: a cross-national investigation
-
- Journal:
- Psychological Medicine / Volume 54 / Issue 12 / September 2024
- Published online by Cambridge University Press:
- 04 October 2024, pp. 3447-3458
-
- Article
-
- You have access
- Open access
- HTML
- Export citation
Disappearance and dissemination of sleep symptoms: the importance of sleep medicine expertise for psychiatry. A comment on Forbes et al.
-
- Journal:
- Psychological Medicine / Volume 54 / Issue 12 / September 2024
- Published online by Cambridge University Press:
- 26 September 2024, pp. 3562-3564
-
- Article
-
- You have access
- Open access
- HTML
- Export citation
Chapter 16 - Machine learning
- from Part II - Applications, tools, and tasks
-
- Book:
- Working with Network Data
- Published online:
- 06 June 2024
- Print publication:
- 13 June 2024, pp 251-278
-
- Chapter
- Export citation
3 - Predictive Modeling for Biomarker Discovery
- from Part I - Framework for Multivariate Biomarker Discovery
-
- Book:
- Multivariate Biomarker Discovery
- Published online:
- 30 May 2024
- Print publication:
- 06 June 2024, pp 25-43
-
- Chapter
- Export citation
10 - Classification with Random Forests
- from Part III - Classification Methods
-
- Book:
- Multivariate Biomarker Discovery
- Published online:
- 30 May 2024
- Print publication:
- 06 June 2024, pp 149-157
-
- Chapter
- Export citation
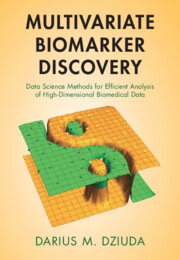
Multivariate Biomarker Discovery
- Data Science Methods for Efficient Analysis of High-Dimensional Biomedical Data
-
- Published online:
- 30 May 2024
- Print publication:
- 06 June 2024
Moving to continuous classifications of bilingualism through machine learning trained on language production
-
- Journal:
- Bilingualism: Language and Cognition , First View
- Published online by Cambridge University Press:
- 24 May 2024, pp. 1-9
-
- Article
-
- You have access
- Open access
- HTML
- Export citation
Chapter 17 - Goethe the Collector
- from Part III - Art
-
-
- Book:
- Goethe in Context
- Published online:
- 16 May 2024
- Print publication:
- 23 May 2024, pp 166-174
-
- Chapter
- Export citation
Chapter 2 - The Classification of Mood Disorders and the Unipolar/Bipolar Dichotomy
-
-
- Book:
- Clinical Textbook of Mood Disorders
- Published online:
- 16 May 2024
- Print publication:
- 23 May 2024, pp 10-36
-
- Chapter
- Export citation
Chapter 14 - Phenomenological Psychopathology of Mood Disorders
-
-
- Book:
- Clinical Textbook of Mood Disorders
- Published online:
- 16 May 2024
- Print publication:
- 23 May 2024, pp 141-150
-
- Chapter
- Export citation
Chapter 8 - Mood and Psychosis: Limits and Overlapping between Psychotic Disorders and Mood Disorders
-
-
- Book:
- Clinical Textbook of Mood Disorders
- Published online:
- 16 May 2024
- Print publication:
- 23 May 2024, pp 82-94
-
- Chapter
- Export citation
7 - Low-Rank Approximation and Multidimensional Scaling
-
- Book:
- Linear Algebra for Data Science, Machine Learning, and Signal Processing
- Published online:
- 01 November 2024
- Print publication:
- 16 May 2024, pp 238-282
-
- Chapter
- Export citation
Automated Sentinel-1 sea ice type mapping and in-situ validation during the CIRFA-22 cruise
-
- Journal:
- Annals of Glaciology , First View
- Published online by Cambridge University Press:
- 13 May 2024, pp. 1-12
-
- Article
-
- You have access
- Open access
- HTML
- Export citation
WinClbclas, a Windows program for columbite-supergroup minerals
-
- Journal:
- Mineralogical Magazine / Volume 88 / Issue 4 / August 2024
- Published online by Cambridge University Press:
- 02 May 2024, pp. 439-450
-
- Article
-
- You have access
- HTML
- Export citation
Dialectometry-based classification of the Central–Southern Italian dialects
-
- Journal:
- Journal of Linguistic Geography / Volume 12 / Issue 1 / April 2024
- Published online by Cambridge University Press:
- 29 April 2024, pp. 13-23
-
- Article
-
- You have access
- Open access
- HTML
- Export citation
Chapter 7.1 - Clinical Features and Implications of New Classification of Personality Disorders
-
-
- Book:
- Seminars in General Adult Psychiatry
- Published online:
- 04 April 2024
- Print publication:
- 18 April 2024, pp 404-413
-
- Chapter
- Export citation
Chapter 3.1 - Clinical Features of Depressive Disorders
-
-
- Book:
- Seminars in General Adult Psychiatry
- Published online:
- 04 April 2024
- Print publication:
- 18 April 2024, pp 64-88
-
- Chapter
-
- You have access
- HTML
- Export citation
Ultrasound diagnosis of cystic echinococcosis: updates and implications for clinical management
- Part of
-
- Journal:
- Journal of Helminthology / Volume 98 / 2024
- Published online by Cambridge University Press:
- 17 April 2024, e34
-
- Article
-
- You have access
- HTML
- Export citation
3 - Data-Driven Platform as Service
- from Part II - Driving Datafication
-
- Book:
- International Economic Law in the Era of Datafication
- Published online:
- 28 March 2024
- Print publication:
- 04 April 2024, pp 79-107
-
- Chapter
- Export citation